Where ML Is Used
Machine learning (ML) is constantly advancing and becoming more prevalent in various industries. From healthcare to marketing, ML is being utilized to automate processes, improve decision-making, and enhance overall efficiency. This article explores some key areas where ML is currently used and discusses its impact on different sectors.
Key Takeaways:
- ML is used in a wide range of industries, including healthcare, finance, retail, and transportation.
- ML applications include chatbots, predictive analytics, fraud detection, and personalized recommendations.
- ML algorithms learn from large volumes of data to make accurate predictions and automate tasks.
Healthcare
ML has tremendous potential in the healthcare industry. It is being used for diagnosis and early detection of diseases, predictive analytics for patient outcomes, and drug discovery. *For example, ML algorithms can analyze vast amounts of medical data to identify patterns and trends that are not easily recognizable to humans.*
In addition, ML can assist in personalized medicine by considering an individual’s genetic information, lifestyle, and medical history to tailor treatment plans. This can greatly improve patient care and outcomes.
Finance
ML is an essential tool in the finance industry. It is used for credit scoring, fraud detection, and algorithmic trading. *By analyzing historical data, ML algorithms can accurately predict creditworthiness and assess the risk associated with loans or credit applications.*
Furthermore, ML can help detect fraudulent activities by identifying patterns and anomalies in large volumes of financial transactions. This enables financial institutions to take immediate action, preventing considerable losses.
Retail
Retail companies are increasingly using ML to enhance their operations and provide a better customer experience. ML-powered recommendation systems personalize product suggestions based on a customer’s browsing and purchase history. *By analyzing user behavior, ML algorithms can suggest relevant and engaging content, increasing customer satisfaction and driving sales.*
In addition, ML is used for inventory management, demand forecasting, and supply chain optimization. This ensures that retailers can keep their products in stock and minimize waste, ultimately improving profit margins.
Transportation
In the transportation industry, ML plays a vital role in various applications such as autonomous vehicles and traffic prediction. ML algorithms enable vehicles to process and interpret sensor data for safer and more efficient driving. *By continuously learning from real-time data, autonomous vehicles can make informed decisions and adapt to changing road conditions.*
Moreover, ML can analyze historical traffic data to predict congestion patterns, enabling transportation authorities to optimize traffic flow and reduce commuting time.
Interesting Data Points
Industry | ML Application | Impact |
---|---|---|
Healthcare | Diagnosis and early detection | Improved accuracy and faster diagnoses |
Finance | Credit scoring | Efficient risk assessment and better loan decisions |
Retail | Recommendation systems | Increased sales and improved customer satisfaction |
Industry | ML Application | Impact |
---|---|---|
Healthcare | Predictive analytics | Improved patient outcomes and efficient healthcare planning |
Finance | Fraud detection | Prevention of financial losses and enhanced security |
Retail | Inventory management | Optimized stock levels and reduced wastage |
Industry | ML Application | Impact |
---|---|---|
Healthcare | Drug discovery | Accelerated research and development process |
Transportation | Autonomous vehicles | Improved road safety and efficient transportation |
Transportation | Traffic prediction | Reduced commuting time and optimized traffic flow |
In conclusion, ML is revolutionizing various industries by automating processes, enabling accurate predictions, and improving overall efficiency. With its ability to learn from large datasets, ML is transforming healthcare, finance, retail, and transportation. The continuous development of ML algorithms and their integration into everyday systems holds immense promise for the future.
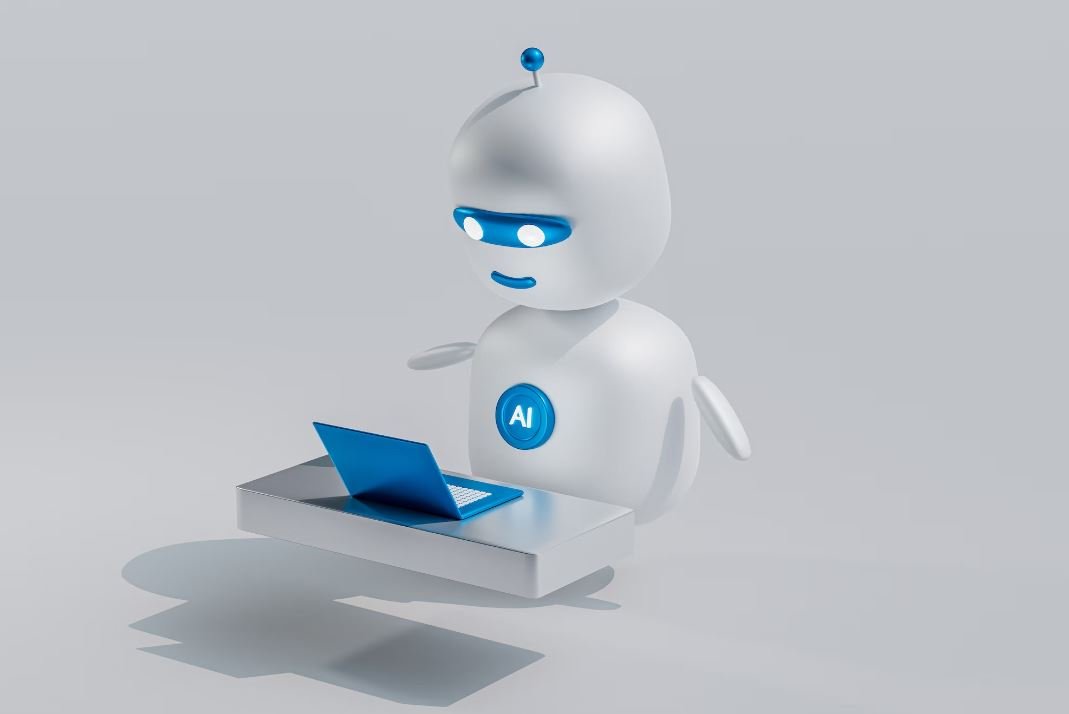
Common Misconceptions
1. Machine Learning is only used in advanced technologies
One common misconception about machine learning (ML) is that it is only applicable in advanced technologies such as self-driving cars or robotics. However, ML finds its use in various other fields as well.
- ML is used in online shopping platforms to recommend personalized products to customers.
- ML is used in healthcare to detect diseases from medical images such as MRIs and X-rays.
- ML is used in finance to detect fraudulent transactions.
2. Machine Learning replaces human intelligence
Some people believe that ML will replace human intelligence and take over jobs completely. However, ML is designed to augment human intelligence and enhance productivity, rather than replacing it entirely.
- ML helps automate repetitive tasks, allowing humans to focus on more complex and creative aspects of their work.
- ML aids in decision-making by providing valuable insights based on data analysis.
- ML can free up time and resources, enabling humans to tackle more meaningful and impactful projects.
3. Machine Learning is always accurate
Another misconception surrounding ML is that it always delivers accurate results. However, ML models are not perfect and can be influenced by biased or incomplete data, leading to less accurate predictions.
- ML models require high-quality and diverse datasets to improve accuracy.
- ML models need continuous monitoring and updates to adapt to changing patterns and ensure accuracy.
- ML models are only as reliable as the data on which they are trained, so data quality is crucial.
4. Machine Learning is too complex for non-experts
Many believe that ML is an extremely complex field that can only be understood and utilized by experts. However, there are user-friendly tools and libraries available that make it accessible to non-experts as well.
- ML platforms like TensorFlow and PyTorch provide user-friendly interfaces for building ML models.
- Many online courses and tutorials give non-experts the opportunity to learn and apply ML techniques.
- ML-as-a-service (MLaaS) providers offer pre-trained models and APIs that can be easily integrated into applications.
5. Machine Learning is only for large organizations
Some believe that ML is a resource-intensive process that is only beneficial for large organizations with massive amounts of data. However, ML can be equally valuable for businesses of all sizes.
- Small businesses can use ML to make data-driven decisions, improve customer experience, and optimize their operations.
- Cloud-based ML solutions eliminate the need for extensive infrastructure, making it accessible for organizations with limited resources.
- ML algorithms can be trained on smaller datasets or pre-trained models can be employed for specific tasks, eliminating the need for large amounts of data.
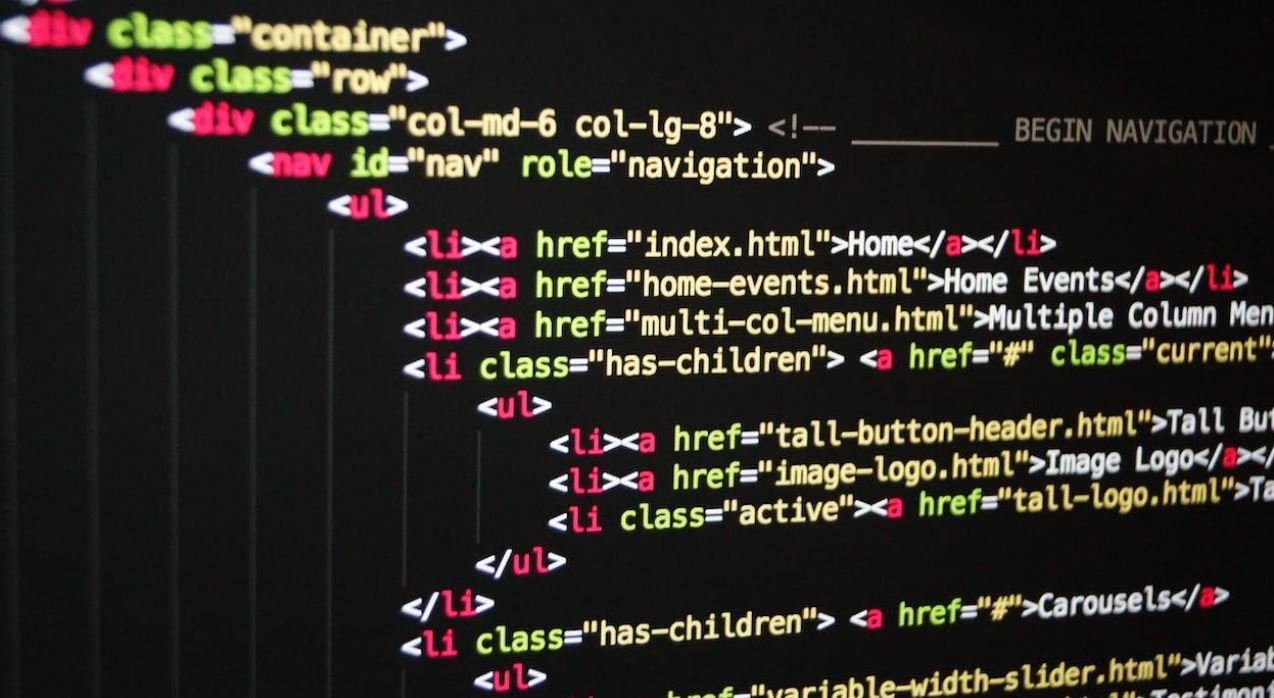
1. The Impact of ML in Healthcare
In recent years, machine learning (ML) has revolutionized the healthcare industry by enhancing diagnosis, treatment, and patient care. This table highlights the different applications of ML in healthcare:
Application | Description |
---|---|
Medical Imaging | ML algorithms can analyze medical images to identify diseases like cancer, speeding up diagnosis. |
Drug Discovery | ML models help pharmaceutical companies analyze vast amounts of data to identify potential new drugs and streamline the discovery process. |
Personalized Medicine | ML algorithms can analyze a patient’s genetic makeup and medical history to tailor treatment plans for optimal outcomes. |
Health Monitoring | Wearable devices and ML algorithms can track vital signs, detect anomalies, and provide real-time health monitoring. |
2. ML Applications in Finance
Machine learning has disrupted the finance industry, allowing for more efficient processes and better risk management. This table showcases the diverse applications of ML in finance:
Application | Description |
---|---|
Fraud Detection | ML models can analyze patterns and anomalies in financial transactions to identify and prevent fraudulent activities. |
Algorithmic Trading | ML algorithms analyze complex market data to make informed trading decisions in real-time, increasing efficiency and profitability. |
Risk Assessment | ML models can assess creditworthiness, predict loan defaults, and evaluate investment risks more accurately. |
Portfolio Optimization | ML algorithms help investors construct optimal portfolios based on risk preferences, expected returns, and market conditions. |
3. Machine Learning in Transportation
The transportation industry has been transformed by machine learning, leading to smarter systems and improved safety. Explore the following applications:
Application | Description |
---|---|
Autonomous Vehicles | ML allows self-driving cars to perceive their surroundings, make decisions, and navigate without human intervention. |
Traffic Management | ML algorithms can analyze traffic patterns, predict congestion, and optimize traffic signal timings, reducing travel time. |
Predictive Maintenance | ML models can predict potential equipment failures, enabling proactive maintenance and reducing downtime. |
Ride-Sharing Optimization | ML algorithms can match riders and drivers in real-time, optimizing routes and reducing overall travel distance. |
4. Machine Learning Applications in Retail
Machine learning technologies are transforming the retail industry, providing personalized experiences and boosting sales. Here are some notable applications:
Application | Description |
---|---|
Recommendation Systems | ML algorithms analyze customer behavior and preferences to provide personalized product recommendations, increasing sales and customer satisfaction. |
Inventory Management | ML models use historical sales data and external factors to optimize inventory levels, minimizing stockouts and excess inventory. |
Price Optimization | ML algorithms can dynamically adjust prices based on demand, competition, and other factors to maximize revenue and profitability. |
Fraud Detection | ML models can detect fraudulent transactions and activities in real-time, protecting both customers and businesses. |
5. ML in Entertainment and Media
The entertainment and media industries leverage machine learning to enhance content creation, recommendation, and distribution. Discover the following ML applications:
Application | Description |
---|---|
Content Recommendation | ML algorithms analyze user preferences and behaviors to provide personalized content recommendations, leading to increased engagement. |
Speech and Image Recognition | ML enables accurate speech-to-text transcriptions and can automatically tag images based on their content. |
Video and Music Classification | ML models can classify and tag videos based on their content, allowing for better organization and searchability. |
Streaming Quality Optimization | ML algorithms can adapt video quality based on network conditions, ensuring smooth playback and a seamless viewing experience. |
6. Machine Learning in Agriculture
Machine learning plays a crucial role in modern agriculture, helping farmers make data-driven decisions to increase productivity and sustainability. Here are notable ML applications:
Application | Description |
---|---|
Crop Yield Prediction | ML models analyze historical data on weather, soil conditions, and crop performance to predict future yields, aiding in planning and resource allocation. |
Pest Detection and Control | ML algorithms can identify pests and diseases early, enabling targeted interventions and reducing crop losses. |
Smart Irrigation | ML models use sensor data and weather forecasts to optimize irrigation schedules, improving water usage efficiency. |
Livestock Monitoring | ML-powered systems can monitor and analyze animal behavior, health, and productivity, facilitating early detection of issues. |
7. ML Applications in Education
Machine learning has the potential to transform education by enhancing teaching methods and personalizing learning experiences. Explore the following ML applications:
Application | Description |
---|---|
Personalized Learning | ML algorithms adapt learning materials and content to individual students based on their abilities, knowledge, and learning pace. |
Intelligent Tutoring Systems | ML-powered systems provide interactive and adaptive tutoring, offering personalized guidance and feedback to students. |
Automated Grading | ML models can automatically assess and provide feedback on student work, saving teachers time and providing prompt evaluations. |
Early Warning Systems | ML algorithms identify students at risk of academic difficulties based on their performance data, enabling timely interventions. |
8. Machine Learning in Cybersecurity
Machine learning plays a vital role in combating cybersecurity threats, mitigating risks, and protecting sensitive data. Check out the following ML applications:
Application | Description |
---|---|
Anomaly Detection | ML algorithms identify abnormal behaviors and patterns in network traffic, aiding in the detection of cyber attacks. |
Malware Detection | ML models can identify and classify malicious software, helping organizations defend against cyber threats. |
User Authentication | ML algorithms analyze user behavior and biometrics to detect unauthorized access attempts and strengthen authentication systems. |
Threat Intelligence | ML-powered systems analyze vast amounts of data from various sources to identify emerging cyber threats and enhance proactive defenses. |
9. Machine Learning in Human Resources
Machine learning has found applications in human resource management, streamlining processes, and improving talent acquisition. Explore the following ML applications:
Application | Description |
---|---|
Resume Screening | ML algorithms can automatically analyze resumes and match them to job descriptions, saving time and improving the candidate selection process. |
Employee Retention | ML models can predict employee turnover by analyzing various factors, guiding HR actions to improve retention rates. |
Performance Evaluation | ML algorithms help assess employee performance by analyzing various metrics and feedback data, aiding in fair and unbiased evaluations. |
Skills Gap Analysis | ML-powered systems analyze employee skills and compare them to job requirements, identifying skill gaps and suggesting targeted training. |
10. ML Applications in Energy
Machine learning technologies have made significant contributions to the energy sector, optimizing operations and promoting sustainability. Explore the following ML applications:
Application | Description |
---|---|
Energy Consumption Forecasting | ML models analyze historical consumption data, weather patterns, and other variables to predict future energy demand, aiding in efficient resource allocation. |
Smart Grid Optimization | ML algorithms optimize the distribution and management of electricity, enabling better grid stability and energy efficiency. |
Fault Detection | ML models can detect anomalies and malfunctioning equipment in power plants, helping prevent failures and improving productivity. |
Renewable Energy Integration | ML algorithms optimize the integration and distribution of renewable energy sources, maximizing their utilization in the overall energy grid. |
Machine learning has undoubtedly revolutionized multiple industries, solving complex problems and enabling data-driven decision-making. With its widespread adoption, businesses and sectors continue to benefit from the power of ML, improving efficiency, accuracy, and innovation.
Frequently Asked Questions
Where ML Is Used
What is machine learning?
How is machine learning different from traditional programming?
Where is machine learning used?
What are some examples of machine learning in healthcare?
How does machine learning contribute to finance?
Can machine learning be used in marketing?
How does machine learning enable autonomous vehicles?
How is machine learning used in cybersecurity?
What are the ethical considerations in machine learning?
How can I learn machine learning?