Which Tool Is Best for Data Analysis?
With the growing importance of data analysis in today‘s business landscape, selecting the right tool to analyze your data is crucial. Different tools offer various capabilities and features, making it challenging to determine which one is the best fit for your needs. In this article, we will explore some popular data analysis tools and highlight their strengths and weaknesses.
Key Takeaways
- Choosing the right data analysis tool is essential for obtaining meaningful insights from your data.
- Each tool has its own unique features and capabilities.
- Consider factors like your specific requirements, data complexity, and user-friendliness when selecting a tool.
1. Excel
Excel is one of the most widely used data analysis tools, known for its flexibility and familiarity. It offers a range of functions and formulas to manipulate and analyze data easily. With its powerful pivot table feature, Excel allows you to summarize and visualize data efficiently.
*Interesting fact: Excel was first released in 1985 and has since become a cornerstone of data analysis for professionals across various industries.
2. Python
Python, a versatile programming language, is gaining popularity among data analysts due to its extensive libraries such as Pandas and NumPy. Python provides a wide array of statistical and data manipulation functions, making it suitable for complex analyses. Its customization options and ability to integrate with other tools are additional advantages.
*Interesting fact: Python’s popularity has surged in recent years, and it has become one of the top programming languages for data analysis and machine learning.
3. R
R is a statistical programming language specifically designed for data analysis and visualization. It offers a vast collection of packages and libraries for advanced statistical modeling and graphics. R excels in handling large datasets and complex statistical algorithms, making it a preferred choice for statisticians and researchers.
*Interesting fact: R was initially developed by Ross Ihaka and Robert Gentleman at the University of Auckland, New Zealand.
Data Analysis Tools Comparison
Tool | Strengths | Weaknesses |
---|---|---|
Excel |
|
|
Python |
|
|
R |
|
|
Factors to Consider
When selecting a data analysis tool, several factors should influence your decision:
- Your specific requirements: Consider the nature of your data and the type of analyses you need to perform.
- Data complexity: If you are working with large datasets or complex statistical models, choose a tool that can handle the size and complexity effectively.
- User-friendliness: Consider your own familiarity with the tool and how easy it is to learn and use.
- Integration possibilities: Evaluate whether the tool can seamlessly integrate with other tools or systems you are using.
Which Tool Should You Choose?
There is no one-size-fits-all answer to this question. The choice of data analysis tool depends on your specific needs and preferences. If you prefer a user-friendly and familiar interface, Excel might be the right choice. For more advanced analytics and customization options, Python or R might be better suited. Ultimately, it is essential to evaluate and test different tools to find the one that aligns best with your requirements.
*Interesting fact: The process of selecting a data analysis tool is similar to choosing the perfect tool for a specific job – you need to consider the right fit and functionality.
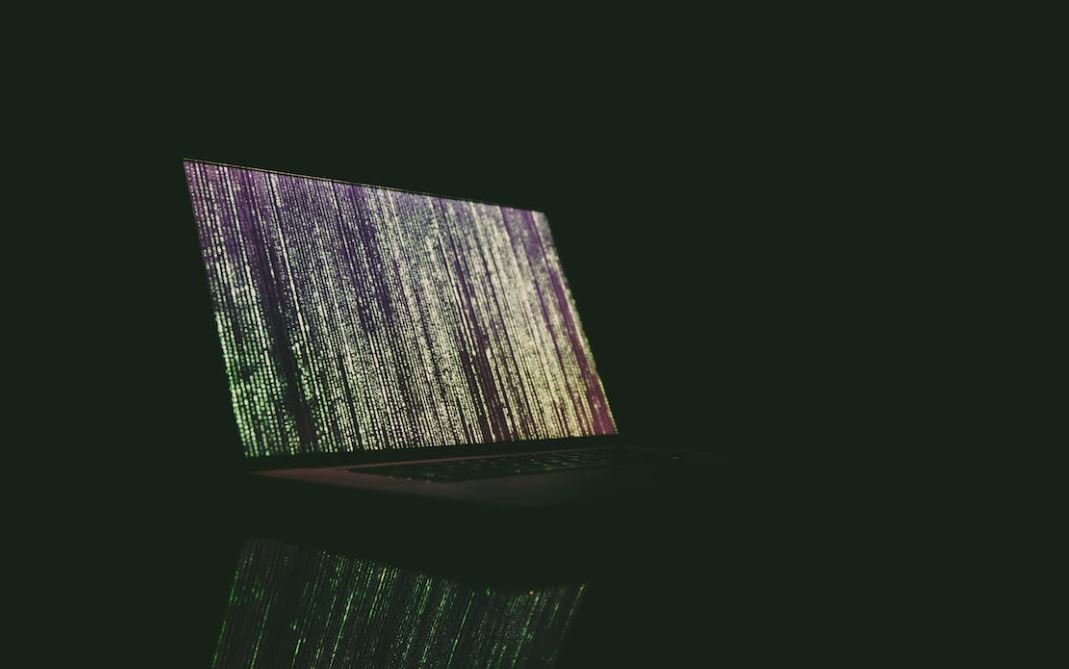
Common Misconceptions
Misconception 1: Spreadsheet software is the ultimate tool for data analysis
Many people believe that spreadsheet software, such as Microsoft Excel or Google Sheets, is the best and only tool for data analysis. While spreadsheets are widely used and offer basic data manipulation and calculation capabilities, they have limitations when it comes to handling large and complex datasets.
- Spreadsheets can become slow and prone to crashing when dealing with big data.
- They lack advanced statistical analysis functions, which limits their ability to uncover complex patterns and relationships in the data.
- Collaboration and reproducibility are harder to achieve in spreadsheets compared to specialized data analysis tools.
Misconception 2: Programming languages are too technical for data analysis
Another common misconception is that data analysis requires extensive programming skills, making it inaccessible to non-technical individuals. While programming languages like Python or R are indeed powerful tools for data analysis, they are not the only options available, and one doesn’t need to be a coding expert to perform basic data analysis tasks.
- Many user-friendly data analysis tools provide a visual interface that doesn’t require coding knowledge.
- Programming languages have extensive online communities and support resources, making it easier to learn and troubleshoot.
- Basic data analysis tasks, such as data cleaning and visualization, can be accomplished through drag-and-drop interfaces without writing any code.
Misconception 3: Data analysis tools are only for statisticians and data scientists
Some people believe that data analysis tools are only meant for statisticians and data scientists, and ordinary individuals or business professionals without a technical background should steer clear of them. However, data analysis tools have evolved to be more user-friendly and accessible to a wider range of users with diverse skill sets and objectives.
- Data analysis tools often offer pre-built analysis templates and guided workflows that simplify the process for non-experts.
- They provide visualizations and reports that are easy to understand and communicate to stakeholders.
- Business professionals can benefit from data analysis tools to gain insights, make data-driven decisions, and improve overall performance.
Misconception 4: Online data analysis tools compromise data security
Concerns around data security often lead to the misconception that using online data analysis tools can compromise the confidentiality and integrity of sensitive data. However, reputable online data analysis tools prioritize data security and employ robust measures to protect user information.
- Many online data analysis platforms adhere to strict data protection regulations and have secure infrastructure in place.
- Encryption and access controls ensure that only authorized users have access to the data.
- Users can choose to work with anonymized or aggregated data to maintain privacy while still deriving insights.
Misconception 5: Data analysis tools automate the entire analysis process
Some people mistakenly believe that data analysis tools can solely automate the entire analysis process, eliminating the need for human involvement. While data analysis tools can automate repetitive tasks and help speed up the analysis process, they still require human input for data interpretation, critical thinking, and contextual understanding.
- Data analysis tools provide the means to process and analyze data efficiently, but human expertise is vital for formulating the right questions and interpreting the results.
- Sound judgment and domain knowledge are essential to ensure the accuracy and relevance of the analysis.
- Data analysis tools are tools for humans, empowering them with efficient ways to derive insights from data.
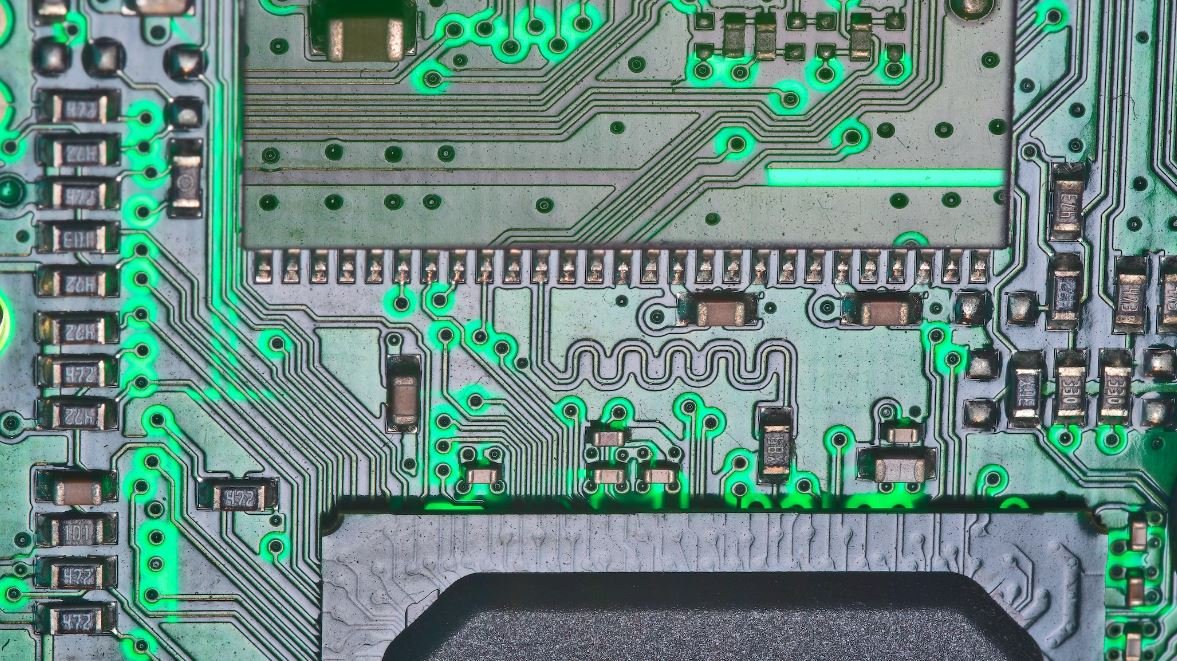
Table: Average Annual Salaries by Profession
According to the latest data, this table shows the average annual salaries of various professions. These figures serve as a reference point for individuals interested in the financial aspects of different career paths.
Profession | Average Annual Salary ($) |
---|---|
Software Engineer | 95,000 |
Data Scientist | 105,000 |
Architect | 87,500 |
Accountant | 65,000 |
Marketing Manager | 85,000 |
Table: Mobile Operating System Market Share
This table displays the market share of various mobile operating systems as of the most recent survey. It portrays which platforms dominate the industry, aiding in decisions related to app development and targeting specific user bases.
Operating System | Market Share (%) |
---|---|
Android | 73.26 |
iOS | 25.85 |
Windows Phone | 0.85 |
BlackBerry | 0.04 |
Other | 0.00 |
Table: Global Carbon Dioxide Emissions by Country
This table exhibits the total carbon dioxide emissions, in million metric tons, attributed to different countries worldwide. It highlights the environmental impact of nations and can aid in understanding the distribution of pollution sources.
Country | Carbon Dioxide Emissions (million metric tons) |
---|---|
China | 10,064 |
United States | 5,416 |
India | 2,654 |
Russia | 1,711 |
Japan | 1,162 |
Table: Online Sales Distribution by Age Group
This table represents the distribution of online sales by age group, allowing businesses to understand their target demographic, create effective marketing strategies, and optimize user experiences for different age cohorts.
Age Group | Percentage of Online Sales (%) |
---|---|
18-24 | 15 |
25-34 | 31 |
35-44 | 25 |
45-54 | 19 |
55+ | 10 |
Table: Top 5 Most Populated Cities Worldwide
This table showcases the five most populated cities across the globe. It offers insights into urbanization trends and assists in understanding population distribution.
City | Population |
---|---|
Tokyo, Japan | 37.4 million |
Delhi, India | 31.4 million |
Shanghai, China | 27 million |
Sao Paulo, Brazil | 22.1 million |
Mumbai, India | 20.7 million |
Table: Importance of Social Media Platforms for Businesses
This table delineates the perception of the importance of social media platforms for businesses. It aids in crafting effective digital marketing strategies and allocating resources accordingly.
Social Media Platform | Importance Rating (out of 10) |
---|---|
8 | |
7 | |
6 | |
9 | |
YouTube | 7 |
Table: Gender Distribution in Engineering Fields
This table presents the gender distribution within different engineering disciplines. It highlights the need for increased diversity in STEM fields and the progress made in achieving gender equality.
Engineering Field | Male (%) | Female (%) |
---|---|---|
Civil Engineering | 82 | 18 |
Chemical Engineering | 75 | 25 |
Electrical Engineering | 80 | 20 |
Mechanical Engineering | 88 | 12 |
Computer Engineering | 70 | 30 |
Table: Smartphone Penetration in Different Countries
This table displays the level of smartphone penetration in various countries, indicating the extent to which mobile phone users have transitioned to smartphones. It provides insights into market opportunities and the adoption of advanced technologies.
Country | Penetration (%) |
---|---|
South Korea | 95 |
United States | 85 |
Germany | 80 |
Brazil | 75 |
India | 60 |
Table: Household Electricity Consumption by Appliance
This table demonstrates the percentage of household electricity consumed by different appliances, allowing users to understand energy usage patterns and identify areas for potential energy conservation.
Appliance | Electricity Consumption (%) |
---|---|
Refrigerator | 13 |
Lighting | 15 |
Air Conditioning | 20 |
Television | 10 |
Washing Machine | 7 |
Conclusion
Choosing the most suitable data analysis tool is vital for researchers, analysts, and organizations aiming to extract insights from data. The tables presented in this article showcase various aspects relevant to decision-making processes. From average salaries across professions to environmental and technological data, these tables provide valuable insights into different fields. By thoroughly understanding and utilizing such data, individuals and organizations can make informed choices, improve strategies, and stay ahead in their respective industries.
Which Tool Is Best for Data Analysis – Frequently Asked Questions
FAQs
What are the top data analysis tools?
Is Python a good tool for data analysis?
Why is R a popular choice for data analysis?
What are the advantages of using Excel for data analysis?
How is Tableau useful for data analysis?
What makes Power BI a popular choice for data analysis?
Is SAS still relevant for data analysis?
What are the key features of Apache Hadoop for data analysis?
How does Apache Spark enhance data analysis?
What are the benefits of using Alteryx for data analysis?