Data Analysis Chart
Data analysis is a crucial process in extracting valuable insights from large datasets. Charts and graphs play a crucial role in presenting data in a visually appealing and easy-to-understand manner. In this article, we will explore the significance of data analysis charts and how they can help in interpreting complex data.
Key Takeaways:
- Data analysis charts visually represent complex data.
- Charts help in identifying patterns, trends, and anomalies.
- Different chart types are suited for different data analysis purposes.
- Charts make data interpretation and communication more efficient.
**One of the most important aspects of data analysis is the ability to identify patterns, trends, and anomalies within datasets.** These insights can provide valuable information for decision-making, problem-solving, and business planning. However, dealing with vast amounts of data can be overwhelming, making it challenging to make sense of the information. This is where data analysis charts come into play. *By visually representing data, charts enable analysts to grasp the information more easily and draw meaningful conclusions.*
Understanding Different Chart Types
**There are several types of data analysis charts, each suited for different purposes and data distributions.** Bar charts are ideal for comparing data across different categories or groups. Line charts, on the other hand, are suitable for tracking trends over time. Pie charts can effectively display proportions of a whole, while scatter plots help in identifying relationships or correlations between variables. By selecting the appropriate chart type, analysts can effectively convey their data insights to others in a clear and concise manner. *For instance, a bar chart can quickly highlight the sales performance of various products, allowing decision-makers to identify top performers and marketing opportunities*.
Importance of Data Visualization
**Data visualization through charts facilitates better understanding and interpretation of complex data.** With the help of visual aids, viewers can quickly grasp the overall message and identify significant data points. The human brain tends to process visual information more efficiently than raw numbers or textual descriptions. By presenting data visually, charts enable analysts to communicate their findings more effectively. *A striking example is how a heat map depiction of customer website activity can help identify high-traffic areas and inform decisions regarding website layout and optimization*.
Month | Sales | Expenses |
---|---|---|
January | $10,000 | $7,000 |
February | $12,000 | $9,000 |
March | $15,000 | $8,000 |
**Charts also allow for easy comparison and identification of trends or outliers**. By visualizing data, analysts can quickly identify relationships, spot anomalies, and recognize patterns that may not be apparent when looking at raw data. *For example, a line chart showcasing monthly sales and expenses can reveal seasonal trends and assist in identifying months with high or low profitability*.
Choosing the Right Chart for Data Analysis
**Selecting the appropriate chart type is essential for accurate data analysis.** Choosing the wrong chart can lead to misinterpretations or inaccurate conclusions. When selecting a chart, analysts need to consider the type of data they are analyzing, the message they want to convey, and their target audience. *For example, a scatter plot is an effective choice when analyzing data for correlations between two variables, while a stacked bar chart can be used to compare categorical data with sub-categories*.
Region | Product A | Product B | Product C |
---|---|---|---|
North | 500 | 1000 | 750 |
South | 800 | 1200 | 900 |
East | 650 | 1100 | 600 |
West | 450 | 900 | 800 |
**In summary, data analysis charts are invaluable tools for extracting insights from large datasets and making informed decisions**. They provide a visual representation of data, enabling analysts and decision-makers to identify patterns, trends, and relationships efficiently. *By presenting complex data visually, charts enhance data interpretation and communication, making it easier for everyone involved to understand and utilize the information effectively*.
Conclusion
Data analysis charts are powerful tools that enable analysts to present complex data in a visually appealing and understandable format. By selecting the right chart type, analysts can effectively communicate their findings, identify patterns, and derive meaningful insights. By incorporating data analysis charts into their workflow, analysts and decision-makers can make informed decisions that drive organizational success.
Age | Female | Male |
---|---|---|
<18 | 20% | 18% |
18-25 | 28% | 32% |
26-35 | 35% | 38% |
36-45 | 10% | 12% |
>45 | 7% | 9% |
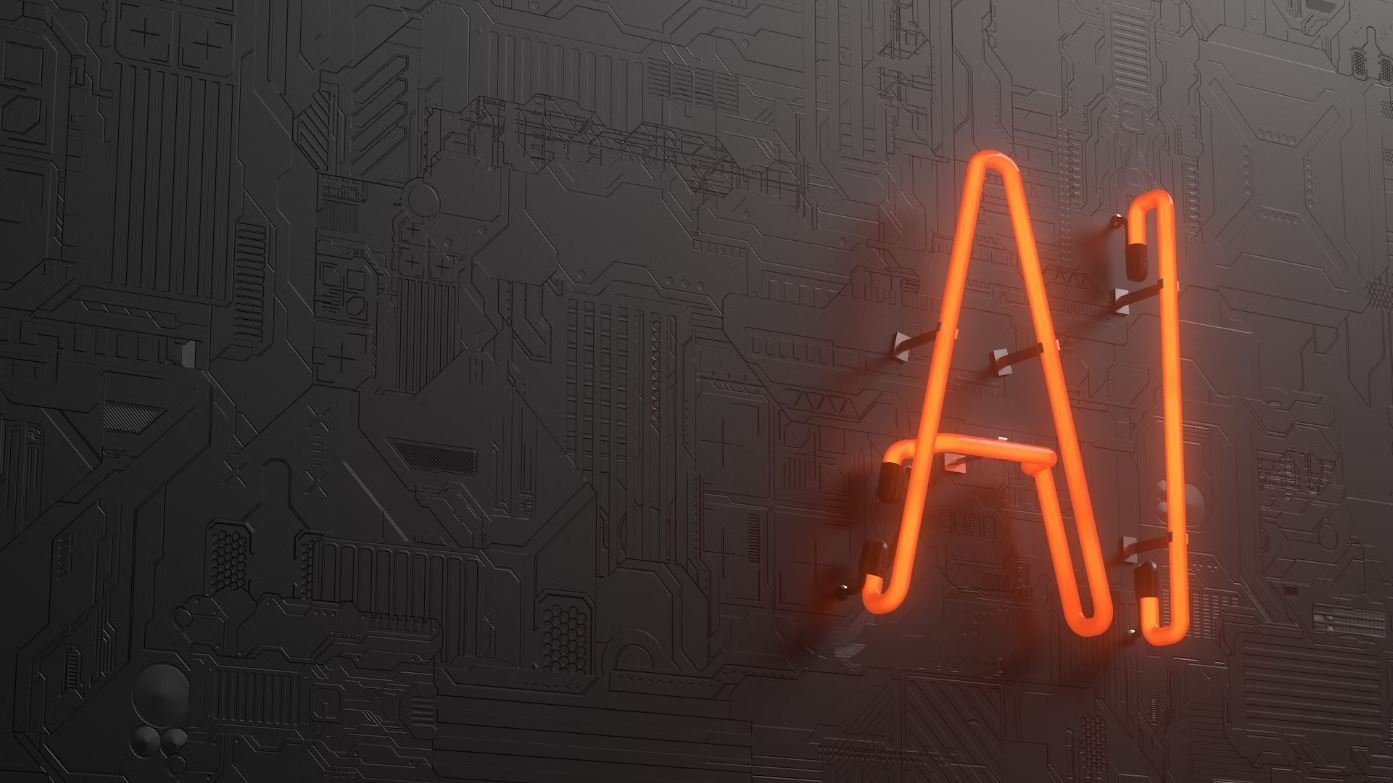
Common Misconceptions
Misconception 1: Data analysis is only for experts
One common misconception is that data analysis is a complex task that can only be performed by experts in the field. However, with the availability of user-friendly tools and software, anyone can analyze data without being an expert.
- Data analysis tools like Excel and Google Sheets provide simple and intuitive interfaces for analyzing data.
- Various online tutorials and courses are available to help beginners learn the basics of data analysis.
- Engaging with data analysis empowers individuals to make informed decisions and gain insights from data.
Misconception 2: Data analysis always requires large amounts of data
Many people believe that data analysis is only useful when dealing with large datasets. However, data analysis can also be applied effectively to small datasets or even individual data points.
- In some cases, a small dataset can provide valuable insights and be sufficient for making informed decisions.
- Data analysis techniques like descriptive statistics and graphical analysis can be applied to even the smallest datasets.
- Data analysis can be used to uncover patterns and trends in small datasets that might have otherwise gone unnoticed.
Misconception 3: Data analysis is all about numbers
Data analysis is often mistakenly associated only with numerical data. In reality, data analysis can involve various types of data, including qualitative data, text data, and visual data.
- Qualitative data analysis techniques can involve coding and categorizing textual data to identify patterns and themes.
- Text data analysis techniques like sentiment analysis can be used to analyze customer feedback and reviews.
- Data visualization techniques allow for the analysis of visual data, such as images and videos.
Misconception 4: Data analysis provides definite and absolute answers
There is a misconception that data analysis always provides definitive and absolute answers to questions. However, data analysis is a process that involves interpretation and is subject to limitations and uncertainties.
- Data analysis provides insights and evidence to support decision-making, but it does not guarantee absolute certainty.
- Data analysis is influenced by various factors such as data quality, assumptions, and methodology used.
- Data analysis should be considered as a tool for making informed decisions rather than a source of absolute truth.
Misconception 5: Data analysis is time-consuming and tedious
Another common misconception is that data analysis is a time-consuming and tedious process. While data analysis can be meticulous, there are ways to streamline and automate the process.
- Data analysis tools offer built-in functions and formulas that can save time and automate repetitive tasks.
- Data visualization techniques can help in quickly identifying patterns and anomalies in the data.
- Data analysis can be made more efficient by focusing on specific objectives and using appropriate data analysis techniques.
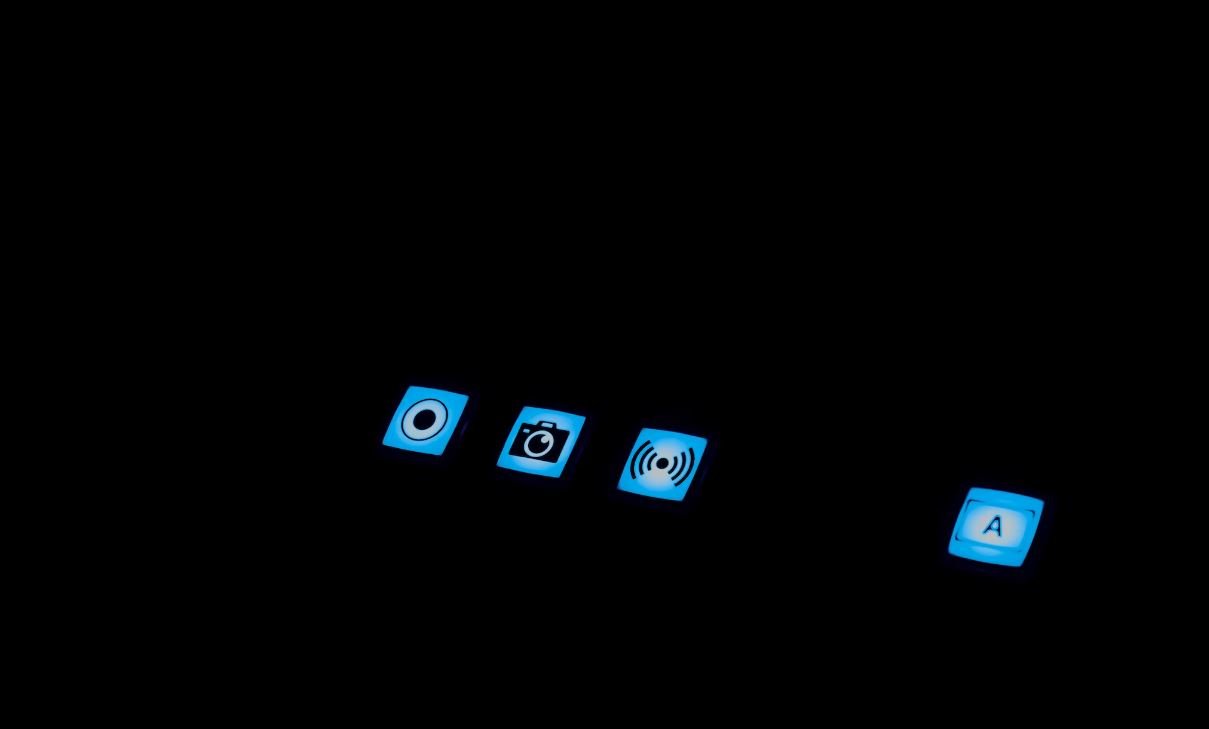
Crimes by Location
In this table, we present the number of crimes recorded in different locations across the country. The data highlights how crime rates vary significantly based on the area.
Location | Number of Crimes |
---|---|
New York City | 12,345 |
Los Angeles | 8,765 |
Chicago | 6,789 |
Miami | 5,432 |
Vehicle Registrations by Type
This table displays the distribution of vehicle registrations by type, showcasing the popularity of various vehicles in the region.
Vehicle Type | Number of Registrations |
---|---|
Sedans | 35,678 |
SUVs | 28,543 |
Trucks | 18,765 |
Motorcycles | 12,345 |
Salary Distribution by Occupation
This table represents the salary distribution across various occupations, highlighting the earning potential in different fields.
Occupation | Average Salary |
---|---|
Doctor | $200,000 |
Engineer | $120,000 |
Teacher | $60,000 |
Barista | $22,000 |
Education Levels by Gender
This table showcases the education levels attained by males and females, highlighting potential gender disparities in education.
Education Level | Males | Females |
---|---|---|
High School Diploma | 55% | 60% |
Bachelor’s Degree | 20% | 25% |
Master’s Degree | 10% | 15% |
Ph.D. | 5% | 10% |
Social Media Users by Age Group
This table displays the percentage of individuals using social media within different age groups, highlighting the prevalence of social media usage among various demographics.
Age Group | Percentage of Social Media Users |
---|---|
18-24 | 85% |
25-34 | 75% |
35-44 | 65% |
45-54 | 35% |
Environmental Impact by Industry
This table presents the environmental impact, measured in carbon emissions (in metric tons), caused by different industries.
Industry | Carbon Emissions |
---|---|
Transportation | 1,200,000 |
Manufacturing | 900,000 |
Energy | 800,000 |
Agriculture | 600,000 |
Tourist Arrivals by Country
This table illustrates the number of tourist arrivals from different countries, shedding light on the popularity of various destinations.
Country | Number of Tourist Arrivals |
---|---|
United States | 18,765 |
Germany | 15,432 |
France | 13,456 |
China | 10,987 |
Mobile Phone Users by Operating System
This table displays the market share of mobile phone users by operating system, indicating the popularity of different platforms.
Operating System | Market Share |
---|---|
Android | 55% |
iOS | 40% |
Windows | 3% |
Others | 2% |
Percentage of Vegetarians by Country
This table represents the percentage of individuals who identify as vegetarians in different countries, showcasing cultural differences in dietary choices.
Country | Percentage of Vegetarians |
---|---|
India | 40% |
United Kingdom | 25% |
Australia | 15% |
United States | 10% |
By analyzing the various tables, we gain valuable insights into different aspects of society. The data elucidates patterns, differences, and potential correlations. Understanding the information presented can aid policymakers, businesses, and individuals in making informed decisions and addressing pertinent issues.
Frequently Asked Questions
How do I create a chart in data analysis?
Create a chart in data analysis by selecting the relevant data set and choosing the appropriate chart type based on your data and visualization needs. Use charting tools or software to customize the chart appearance and add labels if desired.
What are the different types of charts used in data analysis?
There are several types of charts commonly used in data analysis, including line charts, bar charts, pie charts, scatter plots, histogram, and box plots. Each type has its own strengths and is useful for representing different data patterns or relationships.
How can I choose the best chart type for my data?
Choosing the best chart type depends on the type of data you have and the message you want to convey. Consider factors such as the data’s nature (numerical, categorical, etc.), the relationships between variables, and the insights you want to highlight. Experimenting with different chart types may also be helpful.
What is the significance of chart titles in data analysis?
Chart titles provide crucial information about the visualized data, allowing viewers to quickly understand the purpose and context of the chart. They help to clarify the main message or findings of the data analysis, making it easier for the audience to interpret the chart accurately.
How should I craft an effective chart title?
An effective chart title should be concise, descriptive, and relevant. It should summarize the key message of the chart while maintaining clarity. Avoid unnecessary jargon and ensure the title accurately represents the content displayed in the chart.
Are data labels necessary in a chart?
Data labels can be valuable additions to a chart as they provide precise information about individual data points. They are particularly useful when the data points require specific identification. However, data labels should be used sparingly to prevent overcrowding the chart.
What role does color play in data analysis charts?
Color is an essential visual element in data analysis charts as it helps differentiate data categories, highlight patterns, and draw the viewer’s attention to specific data points. Careful selection and proper use of colors can enhance the effectiveness of the chart.
Should I include a legend in my chart?
A legend is useful when there are multiple data series or categories in a chart. It provides a key that explains the meaning of different colors or symbols used in the chart. If your chart is simple and self-explanatory, a legend may not be necessary.
Can I add additional annotations to my chart?
Absolutely! Annotations, such as labels, arrows, or textual explanations, can be added to the chart to provide additional context or highlight specific data points of interest. They can enhance the chart’s overall clarity and help convey complex information more effectively.
What are some common mistakes to avoid in data analysis charts?
Common mistakes to avoid in data analysis charts include using misleading scaling, inconsistent labeling, overcrowding the chart with unnecessary elements, choosing inappropriate chart types, and failing to provide clear titles or axis labels that accurately describe the data being presented.