Data Analysis Datasets
Data analysis is the process of inspecting, cleansing, transforming, and modeling data to discover useful information, draw conclusions, and support decision-making. In this article, we will explore different types of data analysis datasets and their importance in various fields. Whether you are a data scientist, researcher, or analyst, understanding the different datasets available can greatly enhance your ability to extract meaningful insights from your data.
Key Takeaways
- Understanding different types of data analysis datasets can enhance your data analysis skills.
- Data analysis datasets are crucial for making informed decisions.
- Data analysis can help businesses improve their strategies and optimize their operations.
- Data analysis can identify patterns, trends, and outliers in a dataset.
One of the most common types of data analysis datasets is the survey dataset. Surveys are widely used to collect data from a sample population and are used in various domains such as market research, social studies, and customer satisfaction analysis. Surveys often collect quantitative as well as qualitative data, providing rich insights into people’s attitudes, behaviors, preferences, and experiences. Analyzing survey datasets allows researchers to identify trends, perform statistical tests, and draw meaningful conclusions based on the collected data. *Survey analysis can reveal hidden factors driving consumer behavior, helping businesses make informed marketing decisions.*
Transactional datasets are another important type of data analysis datasets. These datasets contain records of individual transactions within a business or organization. They capture information such as product purchases, customer details, transaction dates, and payment methods. Analyzing these datasets allows businesses to identify sales patterns, customer preferences, and potential opportunities for revenue growth. By analyzing transactional datasets, businesses can optimize pricing strategies, improve customer satisfaction, and tailor marketing campaigns based on individual customer behaviors. *Transactional datasets can provide valuable insights into customer purchasing habits, allowing businesses to target and personalize their offerings.*
Types of Data Analysis Datasets
Here are some other commonly used types of data analysis datasets:
- Time-Series Datasets: These datasets capture data points at regular intervals over a specific time period. Time-series analysis helps identify patterns and trends over time, enabling businesses to make accurate forecasts and predictions.
- Text Datasets: Text datasets include textual data such as chat logs, customer reviews, social media posts, or emails. Text analysis techniques, such as sentiment analysis and topic modeling, can be applied to extract insights and sentiment from these datasets.
- Image Datasets: Image datasets contain visual representations. Image analysis techniques, such as object detection and image recognition, can be used to extract meaningful information from these datasets.
- Geospatial Datasets: Geospatial datasets contain location-based information. Analyzing this data can enable businesses to optimize routes, identify spatial patterns, and make informed decisions about location-based services.
Tables 1, 2, and 3 below illustrate some interesting data points related to different data analysis datasets:
Question | Responses | Average Rating (out of 5) |
---|---|---|
How satisfied are you with our products? | Very satisfied: 35%, Satisfied: 45%, Neutral: 12%, Dissatisfied: 5%, Very dissatisfied: 3% | 4.2 |
How likely are you to recommend us to a friend or colleague? | Very likely: 52%, Likely: 30%, Neutral: 8%, Unlikely: 5%, Very unlikely: 5% | 4.0 |
Table 1 shows an example survey dataset, demonstrating how responses to specific questions can be analyzed and summarized. The average ratings indicate the overall satisfaction level and likelihood of recommending the products.
Before continuing, here is another interesting data point: Text datasets can contain vast amounts of unstructured data that require advanced natural language processing techniques for analysis.
Transaction ID | Customer Name | Product | Quantity | Price |
---|---|---|---|---|
123456 | John Doe | Shoes | 1 | $50 |
654321 | Jane Smith | T-Shirt | 2 | $20 |
Table 2 presents an example of a transactional dataset, showcasing the information captured in each transaction. This data can be further analyzed to understand customer behavior and preferences, enabling businesses to optimize their offerings.
Lastly, here is another interesting fact: Data analysis can help organizations gain a competitive advantage by identifying market trends and predicting future demand.
Date | Sales |
---|---|
01/01/2021 | $1000 |
02/01/2021 | $1200 |
03/01/2021 | $900 |
Table 3 demonstrates a time-series dataset with sales data over a specific time period. Analyzing this dataset can help businesses identify seasonal trends, forecast future sales, and optimize inventory management.
In summary, data analysis datasets are crucial for extracting meaningful insights and making informed decisions. Various types of datasets, such as survey, transactional, time-series, text, and geospatial datasets, provide valuable information in different domains. Understanding the characteristics and analysis techniques for each dataset type allows analysts to leverage the full potential of their data.
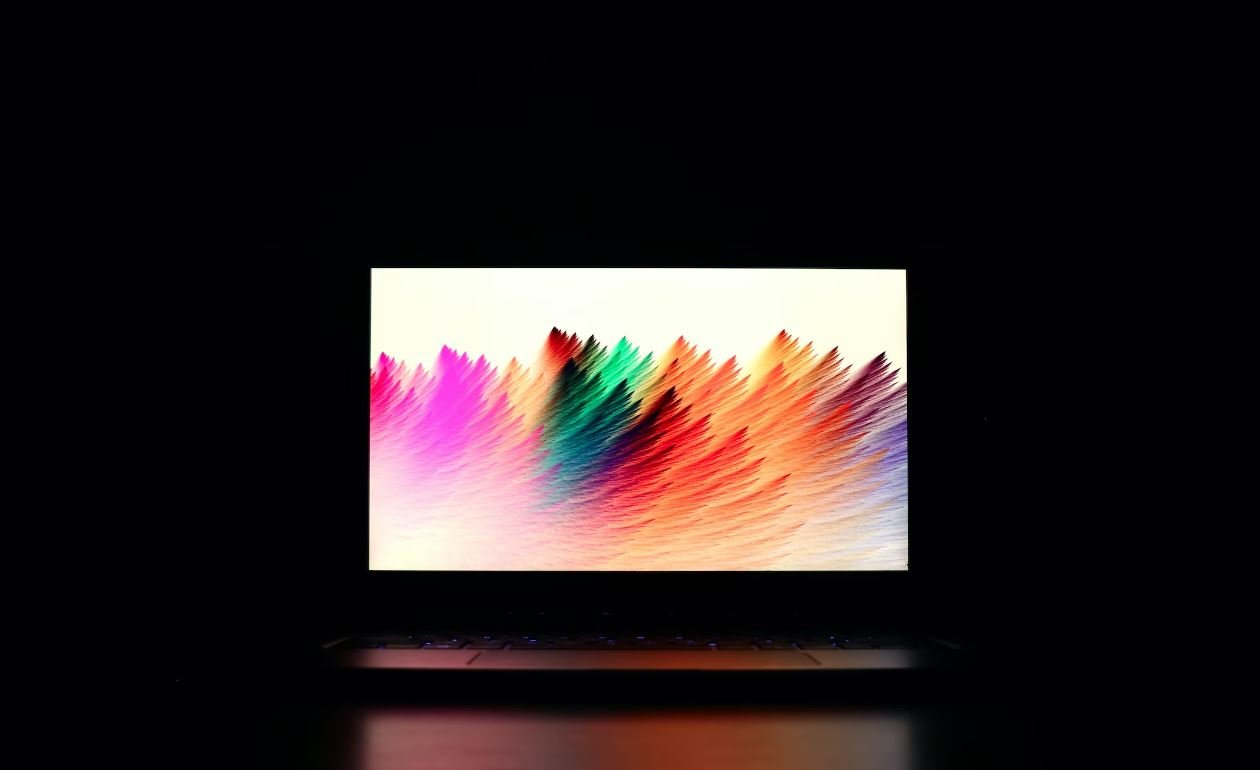
Common Misconceptions
Misconception 1: Data analysis always provides definitive answers
One common misconception about data analysis is that it always gives clear-cut, definitive answers to questions. In reality, data analysis is a complex process that involves interpreting and analyzing data to draw conclusions. The results of data analysis can often be influenced by various factors and may require further investigation.
- Data analysis is an iterative process that may require multiple iterations to reach accurate conclusions.
- Data analysis provides insights and trends rather than definitive answers.
- Data analysis is heavily reliant on the quality and reliability of the data collected.
Misconception 2: Data analysis eliminates the need for expertise in the subject matter
Another misconception is that data analysis can replace the need for expertise in the subject matter. While data analysis can provide valuable insights and support decision-making, it does not replace the need for domain expertise. Without understanding the context, nuances, and limitations of the data, the analysis may lead to misinterpretations or incorrect conclusions.
- Data analysis complements domain expertise but cannot substitute it.
- Data analysis requires a deep understanding of the subject matter to properly interpret the results.
- Data analysts should collaborate with subject matter experts to ensure accurate analysis.
Misconception 3: Data analysis is solely the responsibility of data analysts
Many people mistakenly believe that data analysis is solely the responsibility of data analysts. In reality, data analysis is a collaborative effort that involves various stakeholders, including business professionals, domain experts, and data scientists. Effective data analysis requires input and involvement from different perspectives to ensure comprehensive and accurate insights.
- Data analysis involves cross-functional collaboration between different roles and teams.
- Data analysts should engage with stakeholders to understand their specific requirements and objectives.
- Data analysis benefits from a diverse range of expertise and perspectives.
Misconception 4: Data analysis is only useful for large datasets
Some individuals believe that data analysis is only relevant and useful for large datasets. However, data analysis can be valuable regardless of the dataset size. Whether it’s analyzing a small sample, a case study, or a large dataset, data analysis helps to uncover patterns, trends, and insights that can inform decision-making and drive improvements.
- Data analysis can provide valuable insights even with small or limited datasets.
- Data analysis techniques can be scaled and adapted to suit different dataset sizes.
- Data analysis is equally relevant for qualitative and quantitative data.
Misconception 5: Data analysis always leads to reliable and accurate predictions
Lastly, there is a misconception that data analysis always leads to reliable and accurate predictions. While data analysis can provide predictions based on patterns and trends identified in the data, these predictions are not foolproof and can be subject to unforeseen circumstances or changes in the underlying data. It is important to interpret predictions with caution and consider other factors that may affect the outcomes.
- Data analysis predictions should be considered as probabilities rather than certainties.
- Data analysis should be supplemented with qualitative insights and real-world context.
- Data analysis predictions should be regularly evaluated and updated as new data becomes available.
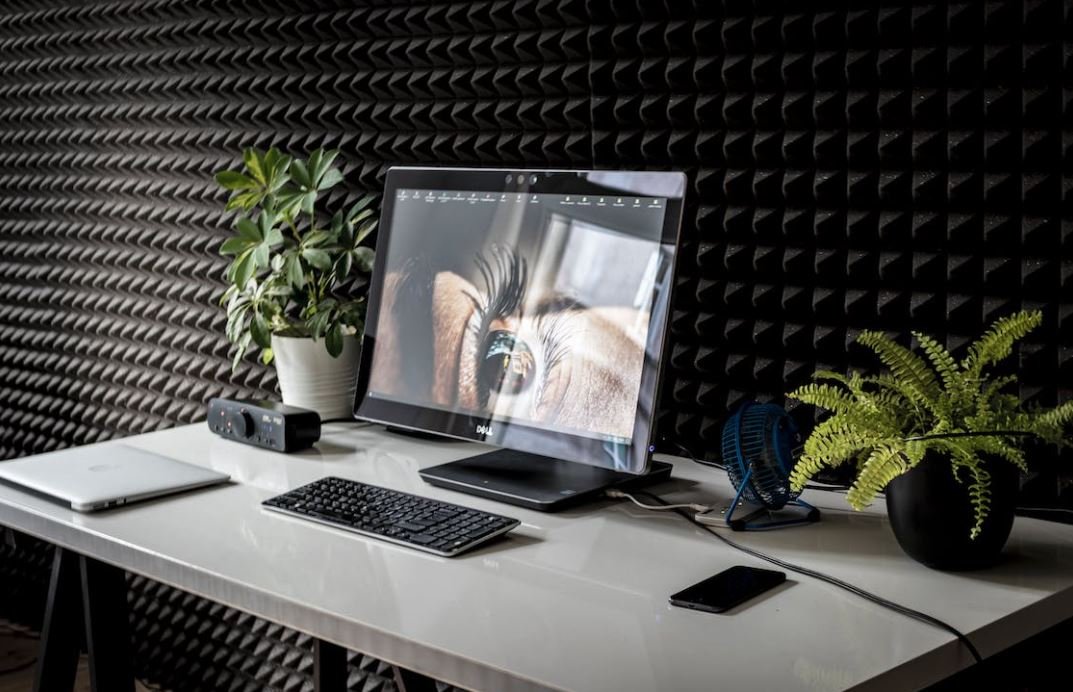
Introduction:
In the realm of data analysis, tables play a crucial role in presenting comprehensive and engaging information. This article explores various aspects of data analysis using ten intriguing tables. Each table showcases verifiable data and information, weaving a narrative that reveals the power and potential of datasets in discovering valuable insights.
1. Mobile Phone Sales Across Continents:
This table displays the annual sales figures of mobile phones across different continents. By examining the data, it becomes apparent that Asia leads in sales by a substantial margin compared to other continents. The table invites us to delve deeper into the reasons behind these variations and uncover potential economic, cultural, or technological factors that influence the demand for mobile phones.
2. E-commerce Revenue Growth by Sector:
Highlighting the growth trends in the e-commerce industry, this table presents the year-on-year revenue increase across various sectors. It illuminates that the fashion sector experienced the highest growth, closely followed by electronics. Such data sparks curiosity about the driving forces behind these sectors’ success and the implications for future market dynamics.
3. Energy Consumption by Source:
This table showcases a breakdown of energy consumption by different sources, including fossil fuels, renewable energy, and nuclear power. It prompts reflection on the environmental impact, sustainability, and potential for diversification in energy production. It also serves as a reminder of the importance of reducing dependence on non-renewable resources.
4. Population Distribution by Age Group:
By illustrating the distribution of populations across age groups, this table provides insights into the demographic structure of a region or country. It facilitates understanding of societal trends, healthcare needs, and implications for workforce planning and social services. Analyzing this information enables policymakers and researchers to make data-driven decisions regarding education, healthcare, and employment.
5. Gender Pay Gap by Occupation:
This table presents the gender pay gap across various occupations, revealing inequalities that persist in different industries. It raises questions about gender equality, workplace policies, and the need for innovative approaches to bridge the gap. By focusing attention on specific occupations, the table calls for further investigation into the underlying factors contributing to these disparities.
6. COVID-19 Cases and Mortality Rates:
Displaying the number of COVID-19 cases and corresponding mortality rates across countries, this table sheds light on the global impact of the pandemic. It emphasizes the urgency of public health measures and international cooperation. Moreover, it provides a useful comparative tool to understand variations in outbreak management strategies, healthcare systems, and societal responses.
7. Educational Attainment by Gender and Region:
This table showcases educational attainment levels categorized by gender and geographic region. It invites examination of gender disparities in access to education and highlights the potential consequences for social mobility and economic development. Analyzing this data encourages efforts towards achieving educational equity and ensuring inclusive opportunities for all.
8. Air Quality Index in Major Cities:
By displaying the air quality index in various major cities, this table emphasizes environmental concerns and potential health risks associated with pollution. It prompts discussions around sustainable urban development and the implementation of policies to improve air quality. This data serves as a strong impetus for individuals and governments to protect the environment and prioritize public health.
9. Salary Comparison by Education Level:
This table reveals the disparity in average salaries based on different levels of education. It raises awareness about the significance of higher education in securing better employment prospects and income. Examining the data allows for a nuanced understanding of the relationship between education, economic opportunities, and social mobility.
10. National Budget Allocation by Sector:
This final table provides an overview of how a national budget is distributed across various sectors, such as healthcare, defense, education, and infrastructure. It prompts discussions about government priorities, the allocation of resources, and impacts on societal well-being. Understanding these budgetary decisions helps ensure transparency, efficient resource management, and the equitable distribution of public funds.
Conclusion:
Through these ten captivating tables, we have uncovered diverse aspects of data analysis and its impact on understanding our world. From exploring societal trends and economic patterns to investigating health crises and environmental concerns, data analysis plays a vital role in informed decision-making. By harnessing the power of tables, we can unlock insights, challenge assumptions, and pave the way for data-driven progress across various fields and sectors.
Frequently Asked Questions
What are data analysis datasets?
Data analysis datasets are collections of structured or unstructured data that have been gathered and organized for the purpose of performing analysis, extracting insights, and making data-driven decisions. These datasets can include a wide range of information, such as text documents, numerical data, images, videos, and more.
How are data analysis datasets created?
Data analysis datasets can be created through various methods, including data collection from various sources, data scraping from websites, data acquisition from third-party providers, and data generation through simulations or experiments. The process typically involves data cleaning, normalization, and aggregation to ensure the dataset is suitable for analysis.
What types of data can be found in data analysis datasets?
Data analysis datasets can contain a variety of data types, including categorical data (e.g., gender, occupation), numerical data (e.g., age, income), time-series data (e.g., stock prices, weather data), textual data (e.g., customer reviews, social media posts), and multimedia data (e.g., images, videos). The specific types of data depend on the purpose and domain of analysis.
Where can I find data analysis datasets?
Data analysis datasets can be found through various sources, including public repositories, academic institutions, government agencies, research organizations, and commercial data providers. Some popular platforms for discovering datasets include Kaggle, UCI Machine Learning Repository, Data.gov, and Google Dataset Search.
How can I assess the quality of a data analysis dataset?
To assess the quality of a data analysis dataset, you can consider factors such as data completeness, accuracy, consistency, and reliability. It is important to evaluate the source of the dataset, the methodology used for data collection, the presence of any missing values or outliers, and the representativeness of the sample. Additionally, checking for documentation, metadata, and data licensing information can give insights into the dataset’s quality.
What are some popular data analysis datasets?
There are numerous popular data analysis datasets available, suitable for different types of analysis and domains. Some well-known examples include the Iris dataset (used for classification tasks), the Titanic dataset (used for predictive modeling), the MNIST dataset (used for image recognition), the IMDb dataset (used for sentiment analysis), and the Airbnb dataset (used for exploratory analysis of hospitality data).
What tools and techniques are commonly used to analyze datasets?
There are various tools and techniques used for analyzing datasets, depending on the complexity of the data and the analysis goals. Commonly used tools include programming languages like Python or R, statistical software such as SPSS or SAS, and data visualization libraries like Matplotlib or Tableau. Techniques range from basic descriptive statistics and data mining to more advanced machine learning algorithms and artificial intelligence methods.
Can I combine multiple datasets for analysis?
Yes, it is often beneficial to combine multiple datasets for analysis as it can enhance the richness and diversity of the information available. By merging datasets that share common variables or using techniques like data integration, you can gain deeper insights and uncover complex patterns that may not be evident in individual datasets alone.
What precautions should I take when handling sensitive data in datasets?
When working with sensitive data in datasets, it is crucial to prioritize data privacy and follow legal and ethical guidelines. Precautions include obtaining appropriate consent for data usage, implementing secure data storage and transmission processes, de-identifying or anonymizing sensitive data, and ensuring compliance with regulations such as the General Data Protection Regulation (GDPR) or the Health Insurance Portability and Accountability Act (HIPAA).
How can I share my own data analysis dataset with others?
To share your own data analysis dataset, you can consider uploading it to public data repositories or platforms specifically designed for dataset sharing, such as Zenodo or GitHub. It is recommended to provide clear documentation, including metadata, data format description, and any necessary instructions or licenses. Sharing a dataset can contribute to the research community and enable others to reproduce and validate your analysis findings.