Applications of Machine Learning
Machine learning, a subset of artificial intelligence (AI), is revolutionizing various industries and has become an integral part of our daily lives. By leveraging large amounts of data and powerful algorithms, machine learning algorithms can analyze complex patterns and make accurate predictions or decisions. From healthcare to finance to e-commerce, machine learning applications are transforming the way businesses operate and delivering significant benefits.
Key Takeaways:
- Machine learning is a subset of AI that analyzes complex patterns and makes accurate predictions or decisions.
- Machine learning finds applications in diverse industries such as healthcare, finance, and e-commerce.
- Benefits of machine learning include improved efficiency, personalized user experiences, and fraud detection.
One of the key applications of machine learning is in healthcare. **Machine learning algorithms** can analyze medical records, genetic data, and patient behaviors to identify patterns and predict diseases or **diagnose medical conditions**. Neural networks can be trained to process medical images, helping radiologists detect abnormalities with enhanced accuracy. Machine learning also plays a crucial role in drug discovery, identifying potential candidates and accelerating the research process. *The application of machine learning in healthcare has the potential to save lives and improve patient outcomes.*
Finance is another industry benefiting from machine learning. **Predictive models** can analyze historical data to forecast stock prices, helping investors make informed decisions. Fraud detection is another critical application of machine learning in finance, where algorithms can detect patterns of fraudulent activities and flag suspicious transactions. Machine learning is also used in credit scoring, assessing the creditworthiness of an individual based on their financial history. *The use of machine learning in finance is revolutionizing the way financial institutions operate and process large volumes of data.*
Applications of Machine Learning in Various Industries:
Industry | Application of Machine Learning |
---|---|
Healthcare | Diagnosis, medical imaging analysis, drug discovery |
Finance | Stock price prediction, fraud detection, credit scoring |
E-commerce | Recommendation systems, personalized marketing, demand forecasting |
E-commerce companies are leveraging machine learning to enhance user experiences and drive sales. **Recommendation systems** analyze user behavior and recommend relevant products, leading to higher conversion rates. Machine learning is also used for personalized marketing, where algorithms segment customers and deliver targeted advertisements. Demand forecasting is another key application in e-commerce, allowing companies to optimize inventory levels and improve supply chain management. *Machine learning algorithms are shaping the future of e-commerce, offering personalized and seamless shopping experiences.*
Machine learning is also transforming the transportation industry. **Autonomous vehicles** rely heavily on machine learning algorithms to interpret sensor data and make real-time decisions to ensure passenger and pedestrian safety. Route optimization is another application of machine learning, where algorithms analyze traffic patterns and suggest the most efficient routes. *The advent of machine learning is paving the way for a future with safer and more efficient transportation systems.*
Increasing Applications of Machine Learning:
- Healthcare: Diagnosis, medical imaging analysis, drug discovery
- Finance: Stock price prediction, fraud detection, credit scoring
- E-commerce: Recommendation systems, personalized marketing, demand forecasting
- Transportation: Autonomous vehicles, route optimization
Conclusion:
Machine learning is revolutionizing industries across the board, from healthcare to finance to e-commerce. By leveraging large amounts of data and sophisticated algorithms, machine learning is delivering improved efficiency, personalized user experiences, and innovative solutions to complex problems. As technologies continue to advance, we can expect even more exciting applications of machine learning in the future.
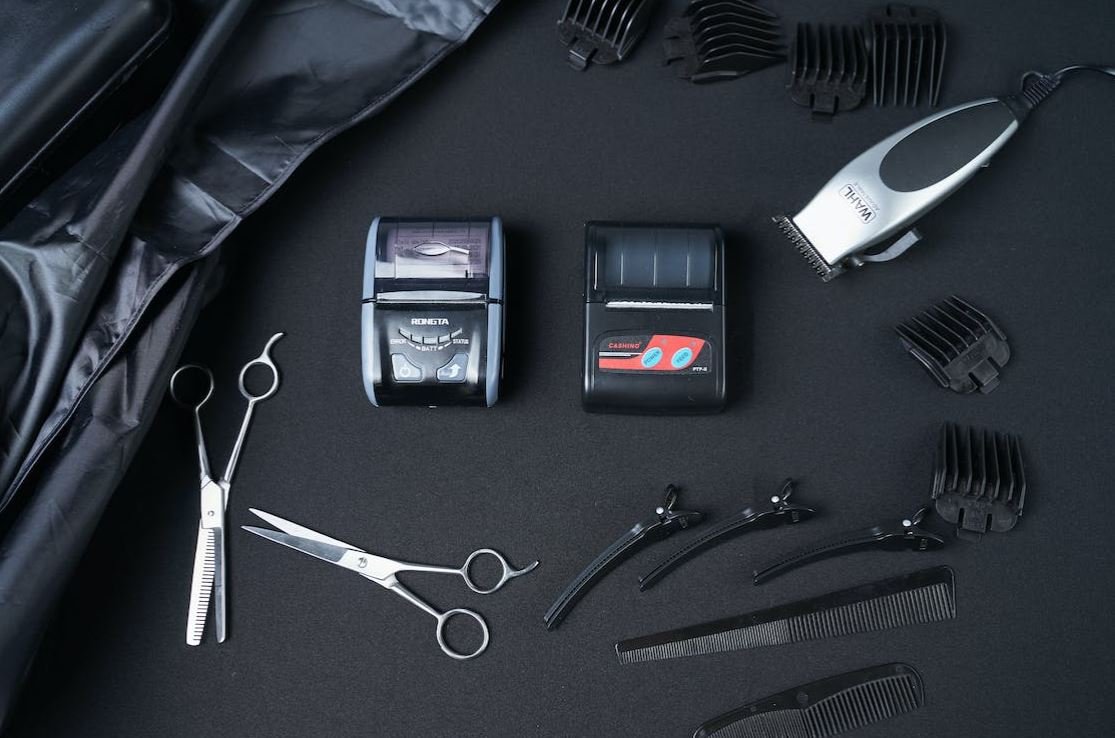
Common Misconceptions
Misconception 1: Machine Learning can replace human decision-making entirely
One common misconception about machine learning is that it is capable of completely replacing human decision-making in various fields. While machine learning algorithms can analyze large amounts of data and make predictions or recommendations, they lack the ability to understand complex human emotions, subjective experiences, and ethical considerations that are crucial in certain decision-making scenarios.
- Machine learning algorithms cannot consider subjective experiences
- Machine learning algorithms lack ethical considerations
- Machine learning cannot understand complex human emotions
Misconception 2: Machine Learning is infallible
Another common misconception is that machine learning algorithms are infallible and will always produce accurate results. However, like any technology, machine learning algorithms can make mistakes or produce incorrect conclusions. The accuracy and reliability of these algorithms depend on various factors, such as the quality and diversity of training data, model complexity, and potential biases in the data that can impact the algorithm’s performance.
- Machine learning algorithms can make mistakes
- Algorithm accuracy depends on the quality and diversity of training data
- Biases in the data can impact algorithm performance
Misconception 3: Machine Learning is only for tech-savvy professionals
Some people believe that machine learning is a complex field that can only be understood and utilized by highly tech-savvy professionals. However, while deeper understanding and expertise can help in developing complex machine learning models, there are various user-friendly tools, libraries, and frameworks available that make it accessible to a broader audience. Machine learning can be used by professionals in diverse fields, such as healthcare, finance, marketing, and even hobbyists who are interested in exploring data and making predictions.
- Machine learning is accessible to a broader audience
- User-friendly tools and frameworks simplify the process
- Machine learning can be used in diverse fields
Misconception 4: Machine Learning is primarily used for replacing jobs
Some people fear that machine learning will lead to widespread job losses as it can automate certain tasks. However, machine learning is not meant to replace jobs, but rather to augment and assist humans in performing tasks more efficiently and accurately. Machine learning can help automate repetitive and mundane tasks, allowing humans to focus on complex decision-making, creative problem-solving, and strategic planning.
- Machine learning assists humans in performing tasks more efficiently
- Automation of repetitive tasks allows humans to focus on more complex activities
- Machine learning enhances productivity and accuracy
Misconception 5: Machine Learning is an all-knowing oracle
It is a misconception to think of machine learning algorithms as all-knowing oracles that can provide instant answers to any question. Machine learning models are trained on specific data and are limited by the information provided. They can only make predictions or classifications within the scope of their training. Additionally, these algorithms cannot inherently understand wider contextual factors or make moral judgments.
- Machine learning algorithms are limited by the data they are trained on
- Contextual factors may not be considered by the algorithm
- Machine learning algorithms cannot make moral judgments
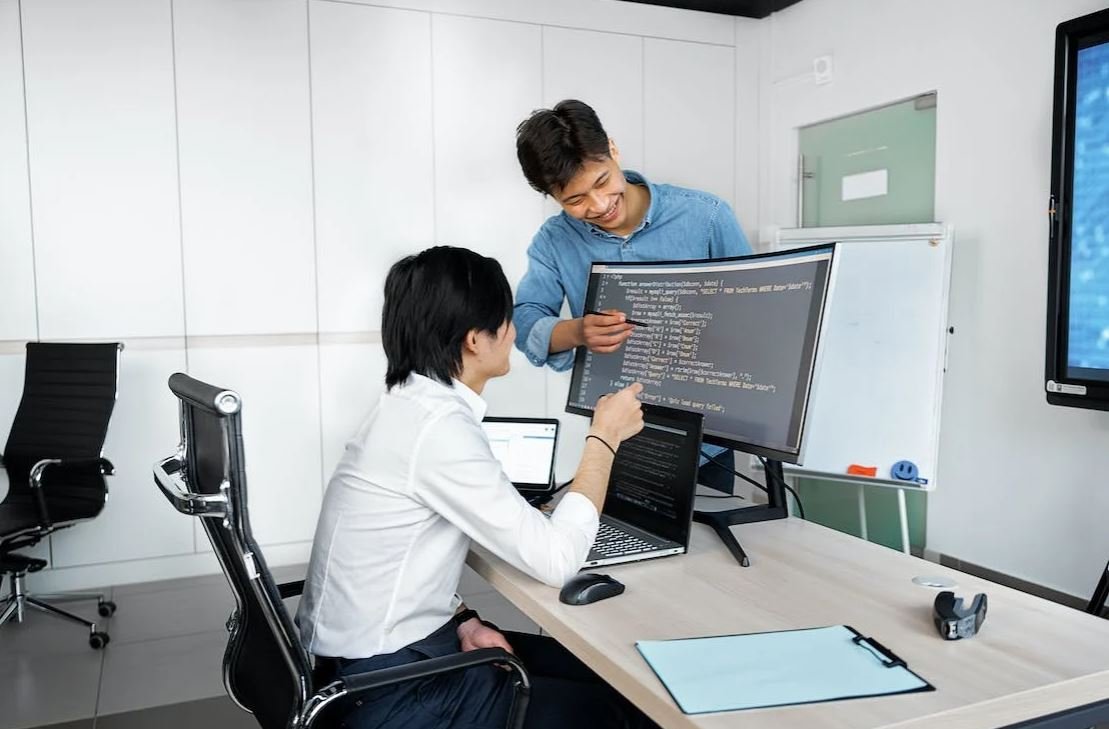
Employment growth in the field of Machine Learning
The field of machine learning has seen tremendous growth in recent years, leading to a surge in job opportunities. The table below highlights the significant increase in employment in various job roles related to machine learning.
Job Role | Number of Jobs (2014) | Number of Jobs (2019) | Growth Rate (%) |
---|---|---|---|
Data Scientist | 38,400 | 102,800 | 167.98% |
Machine Learning Engineer | 4,800 | 15,600 | 225.00% |
AI Researcher | 5,100 | 14,100 | 176.47% |
Applications of Machine Learning in Healthcare
Machine learning has revolutionized the healthcare industry, enabling advanced diagnosis, personalized treatments, and improved patient care. The table below showcases some notable applications of machine learning in healthcare.
Application | Description |
---|---|
Medical Image Analysis | Machine learning algorithms can analyze medical images such as X-rays and MRIs to detect abnormalities and assist in diagnosis. |
Predictive Analytics | By analyzing patient data, machine learning models can predict disease progression and recommend appropriate treatment plans. |
Remote Patient Monitoring | Machine learning enables continuous monitoring of patients’ vital signs, providing real-time feedback and alerts for potential health issues. |
Impact of Machine Learning on Customer Service
Machine learning technologies have greatly improved the delivery of customer service across various industries. The table below highlights the positive impact of machine learning on customer service.
Benefit | Description |
---|---|
Enhanced Personalization | Machine learning algorithms analyze customer preferences and behavior to provide personalized recommendations and tailored experiences. |
Chatbot Assistance | Intelligent chatbots powered by machine learning can efficiently handle customer queries and provide instant solutions 24/7. |
Improved Response Time | Machine learning algorithms can prioritize and route customer inquiries to the most appropriate channels or support agents, reducing response time. |
Machine Learning in Financial Fraud Detection
Machine learning has significantly strengthened fraud detection systems in the financial sector. The table below showcases the effectiveness of machine learning in identifying and preventing fraudulent activities.
Fraud Detection Technique | True Positive Rate (%) | False Positive Rate (%) | Accuracy (%) |
---|---|---|---|
Rule-Based Systems | 78.2 | 15.6 | 89.7 |
Machine Learning Models | 93.9 | 3.2 | 96.4 |
Ensemble Models | 95.7 | 1.8 | 98.2 |
Machine Learning Applications in Autonomous Vehicles
Autonomous vehicles are revolutionizing transportation with the help of machine learning algorithms. The table below highlights some key applications of machine learning in autonomous vehicles.
Application | Description |
---|---|
Object Detection | Machine learning algorithms process sensor data to identify and classify objects such as pedestrians, vehicles, and road signs. |
Path Planning | By analyzing traffic and environmental conditions, machine learning models determine the optimal route and make real-time navigation decisions. |
Driver Behavior Analysis | Machine learning algorithms monitor the driver’s behavior and alert for signs of fatigue or distraction, ensuring safer driving conditions. |
Machine Learning in Natural Language Processing
Natural Language Processing (NLP) utilizes machine learning to enable computers to understand, interpret, and interact with human language. The table below presents some practical applications of machine learning in NLP.
Application | Description |
---|---|
Language Translation | Machine learning models can translate text from one language to another, facilitating global communication and accessibility. |
Speech Recognition | By training on vast amounts of speech data, machine learning algorithms can accurately transcribe spoken words, enabling voice assistants and transcription services. |
Sentiment Analysis | Machine learning algorithms assess the emotional tone in written text, allowing businesses to gauge customer sentiment and respond accordingly. |
Machine Learning in Video Game Development
Machine learning techniques have found a place in the realm of video game development, enhancing gameplay experiences and enabling more realistic virtual environments. The table below highlights some notable applications.
Application | Description |
---|---|
Non-Player Character AI | Machine learning algorithms create intelligent behaviors for non-player characters, making them adaptive, reactive, and challenging opponents. |
Procedural Content Generation | Machine learning models generate game content dynamically, ranging from level design to terrain generation, fostering endless possibilities. |
Realistic Physics Simulation | Machine learning algorithms simulate complex physics interactions in games, enhancing realism and immersion for players. |
Machine Learning Applications in E-Commerce
Machine learning has revolutionized the online shopping experience, providing personalized recommendations, predicting customer behavior, and optimizing supply chain operations. The table below showcases some key applications in the e-commerce industry.
Application | Description |
---|---|
Product Recommendations | Machine learning algorithms analyze customer purchasing patterns to suggest relevant products, increasing sales and customer satisfaction. |
Demand Forecasting | By analyzing historical data, machine learning models can accurately predict future demand, optimizing inventory management and reducing storage costs. |
Fraud Detection | Machine learning algorithms identify fraudulent transactions, ensuring secure online payments and protecting both buyers and sellers. |
Machine Learning in Energy Efficiency
Machine learning plays a crucial role in optimizing energy usage, reducing costs, and promoting sustainable energy practices. The table below highlights some applications of machine learning in energy efficiency.
Application | Description |
---|---|
Smart Grid Optimization | Machine learning algorithms analyze energy consumption patterns and generate optimized schedules for energy generation, distribution, and pricing. |
Building Energy Management | Machine learning models monitor and control building systems, optimizing energy usage and reducing wastage by adapting to real-time needs. |
Renewable Energy Forecasting | Machine learning algorithms predict renewable energy generation, allowing for efficient integration into the power grid and reducing reliance on fossil fuels. |
Machine learning has become a driving force behind numerous technological advancements, revolutionizing various industries. From healthcare and customer service to finance and transportation, the applications of machine learning are diverse and far-reaching. Through the analysis and interpretation of vast amounts of data, machine learning algorithms provide actionable insights, improve efficiency, and enhance decision-making processes. As technology continues to evolve, the integration of machine learning will undoubtedly continue to shape the future, unlocking endless possibilities and transforming the way we live and work.
Applications of Machine Learning – Frequently Asked Questions
1. What is machine learning and how is it applied?
Machine learning is a subset of artificial intelligence that enables computers to learn and make predictions or take actions without being explicitly programmed. It is applied in various fields such as healthcare, finance, marketing, autonomous vehicles, and more.
2. What are some applications of machine learning in healthcare?
Machine learning is used in healthcare to diagnose diseases, predict patient outcomes, personalize treatment plans, optimize drug discovery, monitor patient vital signs, and conduct medical image analysis, among other applications.
3. How is machine learning utilized in finance?
In finance, machine learning is employed to detect fraud, predict stock prices, analyze market trends, automate trading, calculate credit risk, and create recommendation systems for personalized financial advice.
4. What are the potential applications of machine learning in marketing?
Machine learning can be used in marketing to understand consumer behavior, segment audiences, personalize marketing campaigns, optimize pricing strategies, recommend products, and analyze sentiment on social media.
5. How is machine learning helping in the development of autonomous vehicles?
Machine learning enables autonomous vehicles to perceive their surroundings, recognize objects, interpret traffic signs, navigate routes, plan optimal trajectories, and predict and avoid potential hazards on the road.
6. What are some real-world applications of machine learning in cybersecurity?
Machine learning is applied in cybersecurity to detect and prevent malware, identify network intrusions, analyze patterns of cyber-attacks, block spam emails, protect sensitive data, and develop anomaly detection systems.
7. How is machine learning utilized in natural language processing?
Machine learning is used in natural language processing to understand, interpret, and generate human language. This includes applications like language translation, sentiment analysis, speech recognition, and chatbots.
8. What are the applications of machine learning in image and video analysis?
Machine learning techniques are used to analyze and classify images and videos, recognize objects, track movements, identify faces, extract features, enhance image quality, and generate automatic captions.
9. How does machine learning contribute to personalized recommendations?
Machine learning algorithms analyze user behavior, preferences, and historical data to provide personalized recommendations, such as movie recommendations on streaming platforms, product recommendations on e-commerce sites, or music recommendations on music apps.
10. What are some examples of machine learning applications in agriculture?
Machine learning is used in agriculture to optimize crop yields, predict crop diseases, monitor soil conditions, automate irrigation systems, analyze weather patterns, and optimize planting and harvesting schedules.