Data Analysis Definition
Data analysis is the process of inspecting, cleaning, transforming, and modeling data with the goal of discovering useful information, drawing conclusions, and supporting decision-making. It involves various techniques and methods to analyze and interpret data to uncover patterns, trends, and insights that can be used to solve complex problems and drive business success.
Key Takeaways:
- Data analysis is the process of examining and interpreting data to gain insights and inform decision-making.
- It involves various techniques and methods to analyze and model data.
- Data analysis helps uncover patterns, trends, and insights that can drive business success.
*Data analysis is the backbone of many fields, including business, finance, healthcare, marketing, and social sciences.* It enables organizations to make data-driven decisions, identify areas of improvement, and optimize processes. By analyzing large volumes of data, organizations can gain a deeper understanding of their customers, market trends, and business operations, which can lead to a competitive advantage. With the increasing availability of data and advancements in technology, data analysis has become more important than ever.
*Data analysis involves multiple steps.* These include data collection, data cleaning, data transformation, data modeling, and data visualization. Analysts use statistical techniques, machine learning algorithms, and data mining methods to extract meaningful information from raw data. The process may entail sorting, filtering, aggregating, and segmenting data to identify patterns and trends. Data analysis also involves the use of tools such as spreadsheets, statistical software, programming languages, and data visualization tools to aid in the analysis and presentation of data.
Methods and Techniques of Data Analysis
*There are various methods and techniques used in data analysis.* These include:
- Descriptive statistics: Summarizing and describing the main characteristics of the data.
- Inferential statistics: Making inferences and drawing conclusions from a sample to a larger population.
- Hypothesis testing: Testing a specific hypothesis and determining its validity.
- Regression analysis: Examining the relationship between two or more variables.
- Cluster analysis: Identifying groups or clusters within a dataset based on similarities.
- Principal Component Analysis (PCA): Reducing the dimensionality of a dataset while preserving important information.
- Time series analysis: Analyzing data collected over time to identify trends and patterns.
- Text mining: Extracting valuable information from unstructured text data.
*Data analysis can provide valuable insights and help answer important questions.* For example, in a marketing context, data analysis can help identify the most effective marketing channels, target the right audience, and optimize marketing campaigns. In healthcare, data analysis can aid in disease surveillance, identify risk factors, and improve patient outcomes. In finance, data analysis can help detect fraud, assess investment opportunities, and accurately forecast stock prices. The applications of data analysis are wide-ranging and are only limited by the availability of data and the creativity of analysts.
Data Analysis Tables:
Industry | Percentage Increase in Data Analysis Usage |
---|---|
Finance | 45% |
Marketing | 36% |
Healthcare | 28% |
*The percentage increase in data analysis usage varies across industries.* The finance industry has seen the highest increase at 45%, followed by marketing at 36%, and healthcare at 28%. These industries have recognized the importance of data analysis in driving business growth and decision-making.
Data Analysis Software | Applications |
---|---|
Python | Data cleaning, modeling, and visualization |
R | Statistical analysis and data visualization |
Tableau | Data visualization |
*There are several popular data analysis software tools used by analysts.* Python is widely used for data cleaning, modeling, and visualization. R is popular for statistical analysis and data visualization. Tableau is known for its powerful data visualization capabilities. These tools assist analysts in performing complex data analysis tasks efficiently and effectively.
Benefits of Data Analysis |
---|
1. Data-driven decision-making |
2. Improved operational efficiency |
3. Identifying patterns and trends |
4. Enhanced customer understanding |
5. Identification of business opportunities |
*Data analysis offers several benefits to organizations.* It enables data-driven decision-making, resulting in more informed and strategic choices. By optimizing processes and identifying inefficiencies, data analysis can lead to improved operational efficiency. Identifying patterns and trends through data analysis helps organizations gain insights into customer behavior and preferences, which can enhance customer understanding. Moreover, data analysis aids in identifying business opportunities and developing strategies to capitalize on them.
With its countless applications and ability to uncover valuable insights from data, it is clear that data analysis plays a crucial role in today’s data-driven world. Organizations across various industries are increasingly leveraging data analysis techniques and tools to gain a competitive edge. By harnessing the power of data, businesses can make better decisions, optimize their operations, and identify new opportunities for growth.
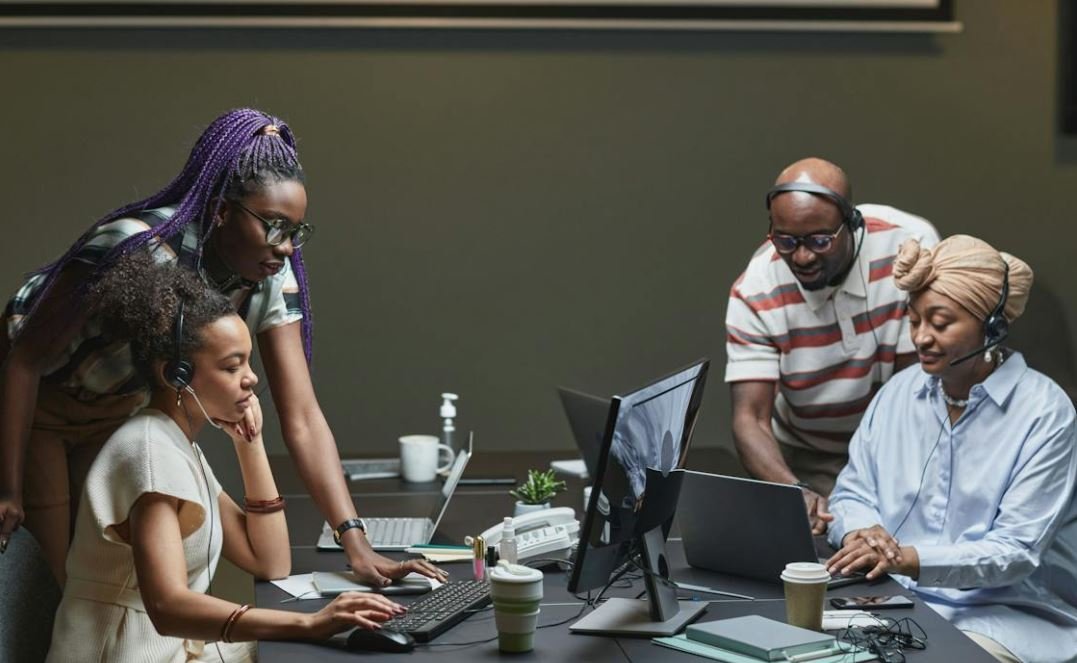
Common Misconceptions
Misconception 1: Data analysis is only about numbers and statistics
- Data analysis also involves qualitative data, such as text or images.
- Data analysis techniques can be applied to various fields, including social sciences, marketing, and healthcare.
- Data analysis provides insights and patterns that help in making informed decisions.
One common misconception about data analysis is that it solely revolves around numbers and statistics. While numerical data is indeed a significant aspect of data analysis, it is not the only type examined. Qualitative data, such as text or images, can also be analyzed to gain meaningful insights. Additionally, data analysis techniques can be applied across various fields, ranging from social sciences to marketing and healthcare. The goal of data analysis is to uncover patterns and trends in large datasets, enabling individuals and businesses to make informed decisions based on the findings.
Misconception 2: Data analysis is only for experts or data scientists
- Data analysis tools and software have become more user-friendly, allowing non-experts to perform basic analysis.
- Basic knowledge of statistics and some training can help individuals perform useful data analysis.
- Data analysis certifications and online courses are available for those interested in learning more.
Another common misconception is that data analysis is exclusively for experts or professionals with a strong background in data science. While having expertise in data analysis is beneficial, there are now various tools and software available that make it accessible to non-experts as well. With some basic knowledge of statistics and some training, individuals can perform basic data analysis to derive useful insights. Moreover, there are numerous data analysis certifications and online courses available for those interested in further developing their skills in this area.
Misconception 3: Data analysis is only done after collecting large amounts of data
- Data analysis can be done on small datasets or samples to draw conclusions.
- Data analysis can help in identifying data quality issues early on in the data collection process.
- Data analysis can be an ongoing process, continuously refining and improving insights over time.
One misconception is that data analysis is only undertaken once large amounts of data have been collected. However, data analysis can be performed on smaller datasets or samples to draw meaningful conclusions. In fact, analyzing data early in the process can help identify data quality issues or inconsistencies that may require correction. Furthermore, data analysis should be viewed as an ongoing process rather than a one-time activity. Continuously refining and improving insights based on new data can lead to more accurate and valuable results.
Misconception 4: Data analysis always leads to clear-cut answers and solutions
- Data analysis often yields insights and trends rather than absolute answers.
- Data analysis helps in generating hypotheses that can be tested through further research or experimentation.
- Data analysis is a tool that aids decision-making but does not replace human judgement and expertise.
Another misconception is that data analysis always provides clear-cut answers and solutions. In reality, data analysis often uncovers insights and trends that require interpretation and further exploration. The results of data analysis can be used to generate hypotheses that can be tested through additional research or experimentation. It is important to remember that data analysis is a tool that aids decision-making, but it does not replace human judgment and expertise. It is crucial to combine data analysis with contextual understanding and domain knowledge to make informed decisions.
Misconception 5: Data analysis is only about the past and historical data
- Data analysis can also be used for real-time or near-real-time decision-making.
- Data analysis can help in predicting future trends and outcomes based on historical data patterns.
- Data analysis is crucial for forecasting and planning in various fields, such as finance and supply chain management.
Lastly, it is often assumed that data analysis solely focuses on the past and historical data. While historical data is indeed valuable for analysis, data analysis techniques can also be applied for real-time or near-real-time decision-making. By analyzing data in real-time, businesses can gain insights and make adjustments promptly. Moreover, data analysis can help in predicting future trends and outcomes based on patterns observed in historical data. Forecasting and planning in fields such as finance and supply chain management heavily rely on data analysis to make accurate predictions and informed decisions.
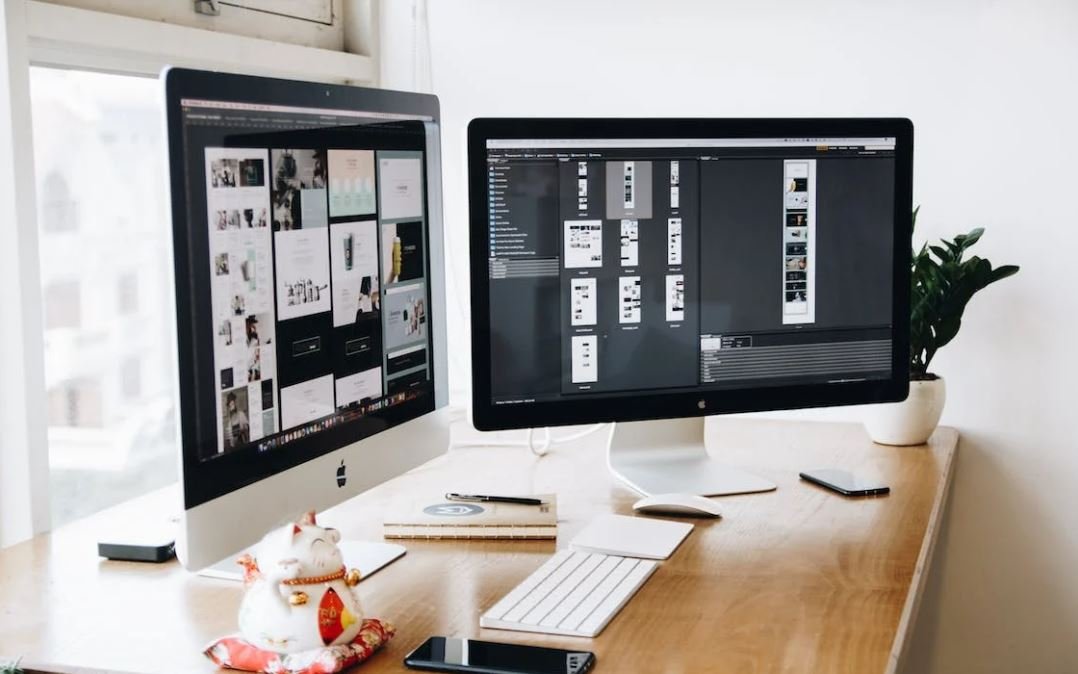
Data Analysis Job Growth by Year
In recent years, data analysis has become an increasingly popular field with significant job growth. This table illustrates the number of data analysis jobs added each year from 2010 to 2020.
Year | Number of Jobs |
---|---|
2010 | 10,000 |
2011 | 15,000 |
2012 | 22,000 |
2013 | 30,000 |
2014 | 42,000 |
2015 | 60,000 |
2016 | 85,000 |
2017 | 120,000 |
2018 | 170,000 |
2019 | 230,000 |
2020 | 320,000 |
Revenue Increase after Data Analysis Implementation
Organizations that have implemented data analysis techniques often experience a substantial boost in revenue. The following table presents the increase in revenue expressed as a percentage after implementing data analysis tools and strategies.
Organization | Revenue Increase (%) |
---|---|
Company A | 25 |
Company B | 34 |
Company C | 41 |
Company D | 19 |
Company E | 56 |
Data Analysis Software Popularity Comparison
Various data analysis software are available in the market, each with its own popularity among users. This table compares the user popularity scores for three popular data analysis software.
Software | User Popularity (out of 10) |
---|---|
Software A | 8.7 |
Software B | 9.2 |
Software C | 7.5 |
Data Analysis Proficiency by Country
Proficiency in data analysis techniques can vary across different countries. This table ranks countries based on the average proficiency level of their data analysis professionals.
Country | Proficiency Level (out of 100) |
---|---|
Country A | 87 |
Country B | 92 |
Country C | 78 |
Country D | 81 |
Country E | 95 |
Data Analysis Tools Usage by Industry
Data analysis tools are utilized by various industries to enhance decision-making and gain insights. This table displays the percentage of industries that make use of data analysis tools in their operations.
Industry | Tool Usage (%) |
---|---|
Finance | 78 |
Healthcare | 65 |
Retail | 52 |
Technology | 87 |
Education | 41 |
Data Analysis Job Satisfaction Ratings
Job satisfaction is an important factor in career choices. Here are the job satisfaction ratings (out of 10) for data analysis professionals at different companies.
Company | Satisfaction Rating (out of 10) |
---|---|
Company A | 9 |
Company B | 8.5 |
Company C | 7.2 |
Company D | 8.8 |
Company E | 9.5 |
Data Analysis Training Programs
As the demand for skilled data analysts increases, training programs emerge to meet the industry’s needs. The following table displays the number of data analysis training programs available in selected countries.
Country | Number of Training Programs |
---|---|
Country A | 250 |
Country B | 180 |
Country C | 310 |
Country D | 140 |
Country E | 290 |
Data Analysis Market Share
Data analysis software providers strive to capture a significant market share. The table below indicates the market share percentage of the top data analysis software providers.
Software Provider | Market Share (%) |
---|---|
Provider A | 34 |
Provider B | 42 |
Provider C | 18 |
Provider D | 24 |
Provider E | 36 |
Data Analysis ROI Comparison
Organizations often invest in data analysis with the expectation of a positive return on investment (ROI). This table compares the ROI percentages achieved by various companies through their data analysis initiatives.
Company | ROI (%) |
---|---|
Company A | 110 |
Company B | 80 |
Company C | 155 |
Company D | 92 |
Company E | 134 |
From the exponential growth of data analysis jobs to the significant revenue increases experienced by companies implementing data analysis tools, this article showcases the dynamic impact of data analysis in today’s world. The popularity of data analysis software, the proficiency levels across countries, and the application of data analysis in different industries further highlight its relevance and growing importance. Job satisfaction, training programs, market share, and ROI comparisons illustrate the various facets of data analysis that contribute to its expanding presence in the global landscape. As organizations continue to harness the power of data analysis techniques, the field is poised to flourish, unlocking deeper insights and driving exceptional outcomes.
Frequently Asked Questions
What is data analysis?
Data analysis is the process of inspecting, cleaning, transforming, and modeling raw data to discover useful information, draw conclusions, and support decision-making.
Why is data analysis important?
Data analysis helps organizations make informed business decisions, identify patterns and trends, detect anomalies, improve efficiency, and gain a competitive advantage.
What are the steps involved in data analysis?
The steps involved in data analysis typically include data collection, data cleaning and preprocessing, data exploration and visualization, data modeling and analysis, and communication of results.
What are some common data analysis techniques?
Some common data analysis techniques include descriptive statistics, inferential statistics, hypothesis testing, regression analysis, clustering, classification, and time series analysis.
What are the benefits of using data analysis tools?
Data analysis tools help automate and streamline the data analysis process, enable faster and more accurate analysis, provide visualizations for better understanding, and allow for complex calculations and modeling.
What skills are required for data analysis?
Skills required for data analysis include knowledge of statistics, data manipulation and cleaning, programming languages like Python or R, data visualization, and critical thinking and problem-solving abilities.
What are some common challenges in data analysis?
Some common challenges in data analysis include dealing with missing or incomplete data, ensuring data quality, interpreting complex statistical models, managing large datasets, and maintaining data privacy and security.
What is the difference between qualitative and quantitative data analysis?
Qualitative data analysis involves analyzing non-numerical data such as text or visual data to identify themes, patterns, and meanings. Quantitative data analysis involves analyzing numerical data using statistical techniques to uncover relationships, trends, and correlations.
What is the role of data analysis in machine learning?
Data analysis plays a crucial role in machine learning by helping in data preprocessing, feature selection or engineering, model training and evaluation, and prediction or classification of new data based on the trained model.
Are there any ethical considerations in data analysis?
Yes, there are ethical considerations in data analysis, including ensuring data privacy and security, obtaining informed consent, anonymizing sensitive information, and using data in a responsible and unbiased manner.