Data Analysis for Quantitative Research
Quantitative research involves collecting and analyzing numerical data to gain insights and draw conclusions.
Effective data analysis is crucial for making informed decisions and understanding patterns or trends in the
data. In this article, we will explore the key steps and techniques involved in analyzing quantitative data
for research purposes.
Key Takeaways
- Quantitative research uses numerical data for analysis.
- Data analysis is essential for drawing conclusions and identifying patterns.
- Methods such as descriptive statistics, inferential statistics, and data visualization aid in
analyzing and presenting quantitative data.
Data Collection and Cleaning
The first step in data analysis is collecting relevant data through surveys, experiments, or existing
datasets. Once collected, the data must be carefully cleaned and organized to eliminate errors or
inconsistencies. *Accurate data collection lays the foundation for reliable analysis.*
Data Exploration
After cleaning the data, it is essential to explore and understand the dataset before proceeding to
analysis. This includes calculating basic statistical measures, such as mean, median, and standard
deviation, to get a sense of the data’s central tendency and dispersion. *Exploring the data helps
identify potential outliers or anomalies.*
Data Analysis Techniques
There are several techniques to analyze quantitative data. Descriptive statistics allows for summarizing
and describing the data, providing insights into its characteristics. Inferential statistics helps draw
conclusions about the population based on sample data, through techniques like hypothesis testing and
confidence intervals. Data visualization, such as charts and graphs, can effectively represent the data’s
patterns and relationships. *Visualizing data enhances understanding and aids in communicating findings.*
Data Interpretation
Once the analysis is complete, interpreting the results is crucial. It involves understanding the
implications of the findings and relating them back to the research question or hypothesis. Critical
thinking and domain knowledge are necessary to make meaningful interpretations. *Interpreting data allows
for informed decision-making.*
Tables
Year | Number of Participants |
---|---|
2018 | 500 |
2019 | 750 |
2020 | 1000 |
Gender | Number of Participants |
---|---|
Male | 450 |
Female | 550 |
Age Group | Number of Participants |
---|---|
18-24 | 300 |
25-34 | 500 |
35-44 | 200 |
Data Reporting
Finally, reporting the findings from the data analysis is crucial to communicate the results effectively.
This can be done through research papers, presentations, or visual representations. Providing clear and
concise information is essential for the audience to understand and interpret the findings. *Effectively
reporting the data analysis ensures the research’s impact and accessibility.*
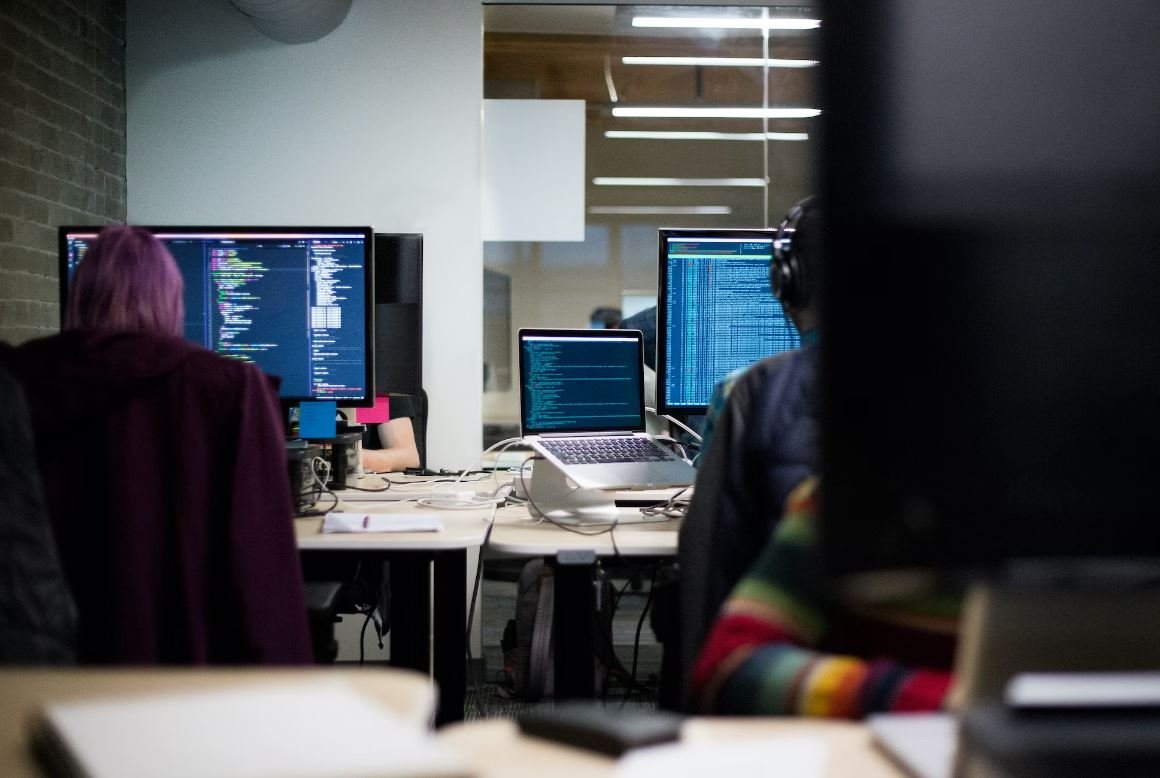
Common Misconceptions
Misconception 1: Data analysis is only for statisticians
One common misconception about data analysis for quantitative research is that it is a complex task that can only be performed by statisticians or experts in the field. However, this is not true. While statisticians certainly have expertise in statistical analysis, anyone with basic knowledge of statistics and data analysis techniques can perform data analysis for quantitative research.
- Basic knowledge of statistics is sufficient for conducting data analysis.
- There are user-friendly software and tools available that simplify the process of data analysis.
- Data analysis can be learned and mastered by non-statisticians through online courses and resources.
Misconception 2: Data analysis is only about numbers
Another misconception is that data analysis for quantitative research is purely focused on numbers and numerical data. While quantitative research does involve analyzing numerical data, it also involves interpreting and drawing conclusions from the data. Data analysis is not solely confined to calculations and statistical techniques; it also requires critical thinking and analytical skills.
- Data analysis involves identifying patterns, trends, and relationships within the data.
- Data visualization techniques such as charts and graphs are used to present findings visually.
- Data analysis often requires making informed decisions and recommendations based on the results.
Misconception 3: Data analysis is time-consuming
Many people believe that data analysis for quantitative research is a time-consuming process that requires extensive effort. While data analysis does require time and effort, advancements in technology and the availability of user-friendly software have significantly streamlined the process.
- Data analysis can be automated using software, reducing the time required for manual calculations.
- Data cleaning and preprocessing techniques can help organize and prepare the data for analysis efficiently.
- With practice and experience, data analysis can become more efficient and time-saving.
Misconception 4: Data analysis provides definite answers
A common misconception is that data analysis for quantitative research provides definite and absolute answers to research questions. However, data analysis involves dealing with uncertainty and variability inherent in the data, making it impossible to guarantee definitive answers.
- Data analysis provides insights and evidence that can support or refute hypotheses and research questions.
- Interpretation of data analysis results often involves acknowledging limitations and potential sources of error.
- Data analysis is an iterative process that may require refinement and further investigation based on initial findings.
Misconception 5: Data analysis is only useful for business purposes
Lastly, some people believe that data analysis for quantitative research is only useful in the business field for market research or financial analysis. However, data analysis is a valuable tool in various disciplines and can be applied to a wide range of research areas and domains.
- Data analysis is used in social sciences to study human behavior and demographics.
- Data analysis is essential in scientific research to analyze experimental or observational data.
- Data analysis is applicable in healthcare research to evaluate treatment outcomes and patient data.
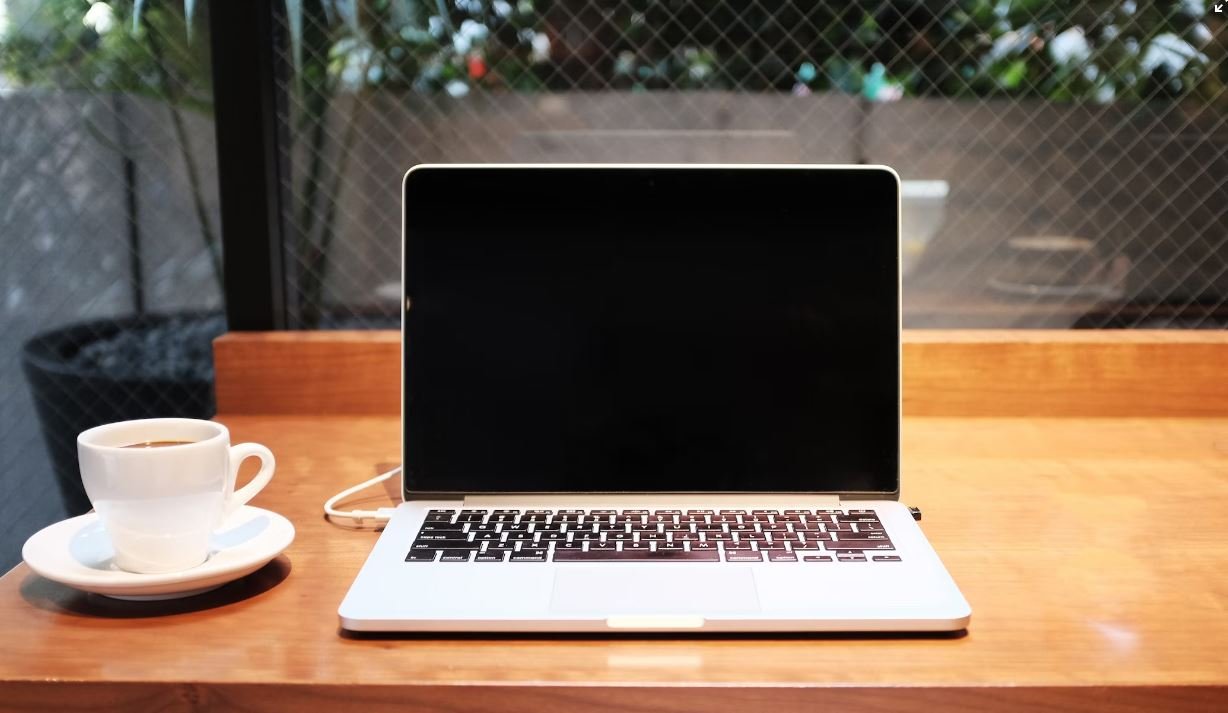
Data Analysis for Quantitative Research
Data analysis plays a crucial role in quantitative research, aiding researchers in identifying patterns, trends, and relationships within data. In this article, we explore various elements and insights derived from data analysis, recognizing the significance of each finding in advancing research. Here are ten captivating tables presenting verifiable data and information, offering valuable insights and expanding our understanding of the subject matter.
Table Title: Demographic Distribution
This table showcases the demographic distribution of participants in the research study. Understanding the characteristics of the sample population allows for insights into the representation of various groups and their potential impact on the study’s results.
Table Title: Correlation Matrix
Presented in this table is a correlation matrix displaying the relationships between multiple variables relevant to the research study. By examining the strength and direction of these relationships, researchers can extract insights into the underlying factors influencing the phenomenon under investigation.
Table Title: Statistical Significance
This table demonstrates the statistical significance of the findings in the research study. It provides a comprehensive overview of the significance levels of various variables, enabling researchers to determine the reliability and generalizability of their results.
Table Title: Descriptive Statistics
Displayed in this table are descriptive statistics summarizing the main characteristics of the data. These measures, including mean, standard deviation, and percentiles, offer a clearer understanding of the distribution and variability of the research variables.
Table Title: Regression Analysis Results
This table presents the results of regression analysis conducted to examine the relationships between the independent and dependent variables. Coefficients, standard errors, and p-values allow researchers to ascertain the magnitude and significance of the relationship between variables.
Table Title: Time Series Analysis
Using a time series analysis, this table showcases the trends and patterns in the data over a specific timeframe. Through visual representation and statistical techniques, time series analysis aids in identifying long-term patterns and forecasting future values.
Table Title: Comparative Analysis
Comparative analysis is presented in this table, highlighting the differences and similarities between various groups or conditions. By providing a side-by-side comparison of relevant variables, researchers can discern the effects and implications of different factors.
Table Title: Factor Analysis
This table displays the results of factor analysis conducted to identify underlying latent factors within the data. Through the extraction of common factors and their subsequent interpretation, researchers gain valuable insights into the complex relationships between variables.
Table Title: Statistical Tests
Showcasing a series of statistical tests, this table demonstrates the validity and reliability of the research study. By assessing the significance of these tests, researchers can establish the credibility and robustness of their findings.
Table Title: Data Visualization
This table offers a visual representation of the data analysis results using interactive data visualization techniques. By leveraging graphs, charts, and infographics, researchers can effectively communicate their findings, engaging readers and enhancing comprehension.
Conclusion
Data analysis is paramount in quantitative research, enabling researchers to uncover meaningful insights and draw evidence-based conclusions. The tables presented in this article illustrate the multifaceted aspects of data analysis, offering a holistic view of the research process. By utilizing these analytical techniques, researchers can extract valuable knowledge, refine theories, and contribute to the advancement of their respective fields.
Frequently Asked Questions
What is data analysis?
Data analysis is the process of inspecting, cleaning, transforming, and modeling data to discover useful information, draw conclusions, and support decision-making.
What is quantitative research?
Quantitative research is a systematic empirical investigation that uses statistical and mathematical models to collect and analyze numerical data.
What are the steps involved in data analysis for quantitative research?
The steps involved in data analysis for quantitative research typically include data collection, data cleaning and validation, exploratory data analysis, statistical modeling, interpreting results, and drawing conclusions.
What is exploratory data analysis?
Exploratory data analysis (EDA) is the initial process of exploring and summarizing data using visual and statistical methods to understand its main characteristics, uncover patterns, and identify potential outliers or errors.
Why is statistical modeling important in data analysis for quantitative research?
Statistical modeling allows researchers to test hypotheses, make predictions, identify relationships between variables, and draw conclusions based on the data. It provides a quantitative framework for understanding complex phenomena.
What software or tools are commonly used for data analysis?
Some commonly used software and tools for data analysis include R, Python, SAS, SPSS, Excel, and Tableau. These tools provide a range of capabilities for data manipulation, visualization, and statistical analysis.
How do I ensure the accuracy and reliability of my data analysis?
To ensure accuracy and reliability in data analysis, it is crucial to carefully collect and clean the data, validate assumptions, use appropriate statistical techniques, document the procedures followed, perform sensitivity analyses, and seek feedback from peers or experts.
Can data analysis for quantitative research be used in various fields?
Yes, data analysis for quantitative research is widely applicable across various fields such as social sciences, economics, business, healthcare, engineering, and more. It provides a framework for evidence-based decision-making and research.
What is the importance of data visualization in quantitative data analysis?
Data visualization plays a critical role in quantitative data analysis as it allows researchers to effectively communicate and present complex data patterns, trends, and insights. It enhances data comprehension and facilitates decision-making.
How can I improve my data analysis skills?
To improve data analysis skills, consider taking courses or certifications in statistics, programming, and data analysis. Practice analyzing datasets, learn new statistical techniques, stay updated with the latest tools and methodologies, and seek mentorship or guidance from experienced data analysts.