Data Analysis Is Defined by the Statistician
Data analysis plays a crucial role in making informed decisions and gaining insights from large sets of data. When it comes to data analysis, it is the statistician who holds the power to make sense of the information at hand. Utilizing specialized statistical techniques, the statistician’s expertise ensures accurate interpretation and meaningful findings.
Key Takeaways
- Data analysis is essential for informed decision-making.
- Statistician’s role is to interpret data and provide meaningful insights.
- Specialized statistical techniques are used to ensure accuracy.
With every dataset, the statistician’s objective is to extract valuable information and uncover patterns and trends that can drive business decisions and improvements.
The Importance of Data Analysis
Data analysis enables organizations to gain insights into various aspects of their operations. By analyzing data, businesses can identify patterns, trends, and correlations that might not be apparent initially. These insights can be used to improve processes, optimize resources, enhance customer experiences, and identify new opportunities for growth. Data analysis empowers decision-makers to make informed choices based on evidence rather than relying on intuition alone.
Effective data analysis serves as a compass, guiding organizations towards data-driven strategies and informed decision-making.
The Role of the Statistician
Statisticians are skilled professionals who possess expertise in statistical methodologies. Their role in data analysis revolves around interpreting and drawing conclusions from data, ensuring the accuracy and reliability of the findings. Statisticians employ various statistical techniques to analyze data, such as hypothesis testing, regression analysis, and data visualization.
A statistician’s statistical prowess is the backbone of insightful conclusions derived from complex datasets.
Statistical Techniques for Accurate Analysis
When it comes to data analysis, statisticians employ a range of specialized techniques to obtain accurate results. These techniques include:
- Hypothesis Testing: This method allows statisticians to determine if there is a significant difference between two or more groups. By formulating null and alternative hypotheses, statisticians can draw conclusions about the population based on sample data.
- Regression Analysis: Regression analysis helps statisticians identify relationships and dependencies between variables. It allows them to predict outcomes based on historical data and quantify the impact of different factors.
- Data Visualization: Graphical representations of data make it easier to understand complex information. Statisticians utilize charts, graphs, and other visualizations to present their findings in a concise and visually appealing manner.
Utilizing specialized statistical techniques ensures a rigorous and reliable analysis of data.
Tables: Insights from Data
Technique | Purpose |
---|---|
Hypothesis Testing | Determine significant differences between groups |
Regression Analysis | Identify relationships and predict outcomes |
Data Visualization | Present findings in a visually appealing manner |
Improved Decision-Making | Optimized Processes |
---|---|
Informed choices based on evidence | Efficient allocation of resources |
Enhanced customer experiences | Identification of growth opportunities |
Role | Responsibilities |
---|---|
Statistician | Interpreting data and drawing conclusions |
Data Analyst | Cleaning and processing data, generating reports |
The Power of Data Analysis
Data analysis, led by skilled statisticians, empowers organizations to harness the power of their data effectively. By interpreting data through statistical methodologies, businesses can uncover valuable insights that drive growth, maximize operational efficiency, and enhance decision-making. The potential of data analysis is boundless, making it an indispensable tool across various industries and sectors.
Embracing the power of data analysis can propel organizations towards success in an increasingly data-driven world.
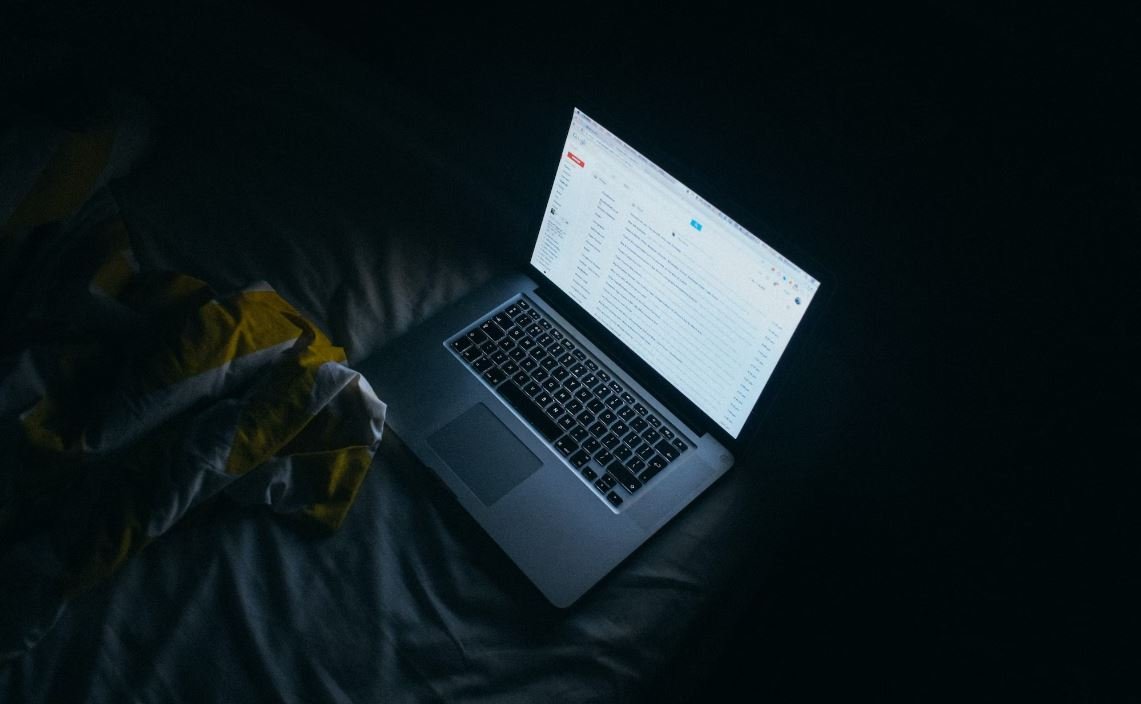
Common Misconceptions
Data Analysis Is Defined by the Statistician Title
One common misconception about data analysis is that it is exclusively defined by the statistician title. While statisticians do play a crucial role in data analysis, it is important to understand that data analysis encompasses a broader set of skills and expertise. Data analysis involves activities such as data cleaning and preprocessing, data visualization, and finding patterns and insights in large datasets. Statisticians certainly possess the statistical knowledge required for advanced data analysis, but other professionals with expertise in fields such as computer science and machine learning also contribute significantly.
- Data analysis involves a range of skills beyond statistics
- Statisticians are not the sole experts in data analysis
- Data analysis benefits from multidisciplinary collaboration
Statisticians Are Always Objective in Data Analysis
Another misconception is that statisticians are always objective when performing data analysis. While objectivity is a fundamental principle in statistical analysis, it is essential to acknowledge that bias and subjectivity can still influence the interpretation and presentation of data. Statisticians, like any other human being, are susceptible to cognitive biases, personal beliefs, and external influences. It is crucial to practice critical thinking and ensure transparency in data analysis, performing peer reviews, and seeking diverse perspectives to minimize potential biases.
- Objectivity is vital in data analysis but not guaranteed
- Subjectivity can affect the interpretation of data
- Critical thinking and diverse perspectives help minimize bias
Data Analysis Produces Only One Correct Conclusion
One of the most common misconceptions about data analysis is that it can produce only one correct conclusion. In reality, data analysis is an iterative process that involves exploring multiple hypotheses, testing different models, and interpreting results within the context of uncertainty. Data can be subject to various interpretations, and the conclusions drawn may depend on the specific methods, assumptions, and limitations of the analysis. It is essential to communicate the uncertainty associated with the results and consider alternate explanations or interpretations when conducting rigorous data analysis.
- Data analysis involves exploring multiple hypotheses
- Interpretations depend on methods, assumptions, and limitations
- Uncertainty and alternate explanations should be considered
Data Analysis Is a Highly Technical Process
Many people believe that data analysis is a highly technical and complex process that requires advanced knowledge of mathematics and programming. While proficiency in these areas is undoubtedly beneficial, data analysis can be approached at different levels of complexity depending on the scale and nature of the problem. Basic data analysis techniques, such as summary statistics and basic visualizations, can be performed on small datasets using software tools with intuitive user interfaces. For more complex analysis, collaboration between domain experts, statisticians, and data scientists can bring together the necessary technical expertise and domain knowledge.
- Data analysis can be performed at different levels of complexity
- Basic analysis techniques are accessible to non-technical users
- Collaboration leverages technical expertise and domain knowledge
Data Analysis Is Time-Consuming and Can Delay Decision Making
There is a misconception that data analysis is always a time-consuming task that can delay decision making. While some analyses might involve rigorous data collection, cleaning, and processing steps, the availability of modern tools and techniques in data analysis has significantly reduced the time required for many routine tasks. Furthermore, exploratory data analysis can provide valuable insights even with limited time and resources, allowing for informed decision making within constrained timelines. It is important to optimize the data analysis process based on the specific goals, urgency, and available resources.
- Data analysis can be performed efficiently with modern tools
- Exploratory analysis provides valuable insights within timelines
- Data analysis process can be optimized based on goals and resources
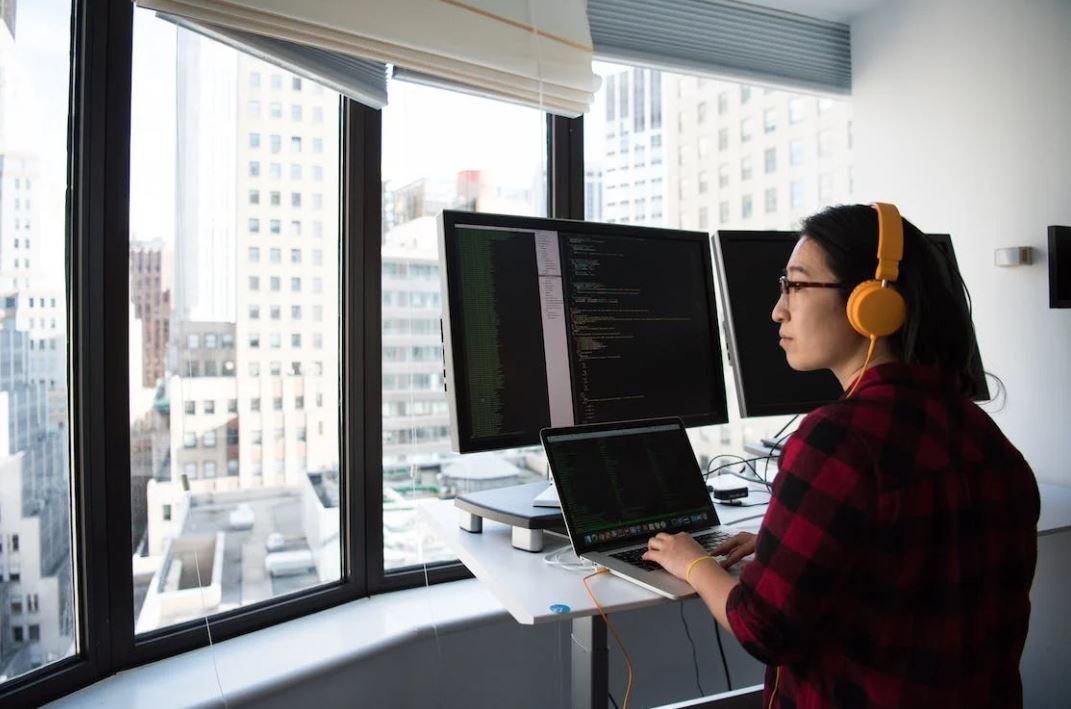
Data Analysis Job Market
According to a recent report by Glassdoor, the job market for data analysts is booming. The demand for skilled professionals in this field has surged in recent years, with companies increasingly relying on data-driven insights to drive their decision-making processes. The table below provides a breakdown of the average salaries of data analysts in selected cities.
City | Average Salary | Annual Growth |
---|---|---|
New York City, NY | $92,750 | 7% |
San Francisco, CA | $99,500 | 10% |
Chicago, IL | $84,200 | 6% |
Seattle, WA | $88,600 | 8% |
Boston, MA | $91,900 | 7% |
Data Analysis Techniques
Data analysis involves using various techniques to extract meaningful insights from datasets. The following table highlights some common techniques used by data analysts along with their brief descriptions.
Technique | Description |
---|---|
Regression Analysis | Statistical technique for determining the relationship between variables. |
Data Mining | Process of discovering patterns and insights in large datasets. |
Time Series Analysis | Examining data points collected over time to identify trends or patterns. |
Cluster Analysis | Grouping data points based on similarities or differences. |
Hypothesis Testing | Statistical analysis to test a hypothesis or claim about a population. |
Data Analytics Software
Data analysts often rely on specialized software to manipulate and analyze data efficiently. The table below presents some popular data analytics software along with their key features.
Software | Key Features |
---|---|
Microsoft Excel | Data manipulation, formula-based analysis, and visualizations. |
Python (Pandas) | Powerful data manipulation, analysis, and visualization libraries. |
Tableau | Interactive data visualization, drag-and-drop functionality. |
RapidMiner | Machine learning algorithms, data preprocessing, and predictive analytics. |
IBM Watson Analytics | Advanced analytics, natural language processing, and cognitive capabilities. |
Big Data Analysis Platforms
With the advent of big data, specialized platforms have emerged to handle the challenges of processing and analyzing large datasets. The table below outlines some notable big data analysis platforms and their primary features.
Platform | Primary Features |
---|---|
Apache Hadoop | Distributed file system, fault tolerance, and parallel processing. |
Apache Spark | Distributed computing, in-memory processing, and real-time data streaming. |
Amazon Redshift | Columnar storage, scalability, and integration with other AWS services. |
Google BigQuery | Serverless, highly scalable, and fast querying of large datasets. |
Microsoft Azure HDInsight | Integration with Microsoft ecosystem, scalable and secure big data processing. |
Data Analysis Software Salaries
The salary range for professionals specializing in data analysis software varies based on experience and expertise. The table below showcases the salary ranges for different positions in this field.
Position | Salary Range |
---|---|
Data Analyst | $55,000 – $120,000 |
Data Scientist | $70,000 – $150,000 |
Data Engineer | $80,000 – $140,000 |
Business Intelligence Analyst | $60,000 – $130,000 |
Machine Learning Engineer | $90,000 – $170,000 |
Data Visualization Tools
Data analysts utilize visualization tools to present their findings in a visually compelling manner. The table below showcases some popular data visualization tools along with their primary features.
Tool | Primary Features |
---|---|
Tableau | Drag-and-drop functionality, interactive dashboards, and storytelling capabilities. |
Power BI | Extensive data connections, interactive visualizations, and collaboration features. |
D3.js | JavaScript library for creating custom interactive visualizations on the web. |
Plotly | Online graphing tool with interactive and collaborative features. |
QlikView | Associative data model, dynamic dashboards, and self-service analytics. |
Data Analysis in Healthcare
Data analysis plays a crucial role in the healthcare industry for improving patient outcomes and optimizing operations. The table below presents some key applications of data analysis in healthcare.
Application | Description |
---|---|
Medical Research | Analyzing clinical trial data to evaluate the effectiveness of treatments. |
Diagnosis and Prognosis | Using predictive models to diagnose diseases and determine patient outcomes. |
Resource Allocation | Optimizing the distribution of healthcare resources based on data-driven insights. |
Public Health Surveillance | Monitoring population health trends to identify disease outbreaks or patterns. |
Electronic Health Records Analysis | Extracting valuable insights from vast amounts of patient health data. |
Data Analysts vs. Data Scientists
Data analysts and data scientists are both professionals working with data, but they have different roles and responsibilities. The table below highlights some of the key distinctions between these two roles.
Data Analysts | Data Scientists |
---|---|
Focus on data cleaning, analysis, and visualization. | Design complex statistical models and predictive algorithms. |
Identify trends and patterns in data to support decision-making. | Create and implement machine learning models for predictive analytics. |
Proficient in statistical analysis and data visualization tools. | Have expertise in programming languages and advanced data modeling techniques. |
Typically work with structured and less complex datasets. | Handle unstructured and complex datasets, often leveraging big data technologies. |
Provide actionable insights based on data analysis. | Research, develop, and deploy data-driven solutions. |
Conclusion
Data analysis has become an indispensable discipline in today’s data-driven world. With the increasing availability of data and the rise of advanced analytics tools, professionals in the field are in high demand. From job market trends to various analysis techniques, software, and specialized platforms, this article has provided a comprehensive overview of data analysis. Whether it’s in industries like healthcare or navigating the distinctions between data analysts and data scientists, the power of data analysis continues to shape our decision-making processes and drive innovation.
Frequently Asked Questions
What is data analysis?
Data analysis refers to the process of inspecting, cleaning, transforming, and modeling data in order to discover useful information, draw conclusions, and support decision-making.
Who is a statistician?
A statistician is a professional who specializes in the collection, analysis, interpretation, presentation, and organization of data. They apply statistical techniques to understand and solve real-world problems.
Why is data analysis important?
Data analysis plays a crucial role in various fields such as business, healthcare, finance, marketing, and research. It helps in identifying patterns, trends, and insights from data, which can be used to make informed decisions and improve processes.
What are the steps involved in data analysis?
Data analysis typically involves several steps including data collection, data cleaning, data transformation, data modeling, statistical analysis, data visualization, and interpretation of results.
What tools can be used for data analysis?
There are several tools available for data analysis, including statistical software like R, Python, SPSS, and SAS. Excel and Tableau are also commonly used for analyzing and visualizing data.
What statistical techniques are used in data analysis?
Various statistical techniques are employed in data analysis, such as descriptive statistics, inferential statistics, regression analysis, hypothesis testing, cluster analysis, factor analysis, and time series analysis.
How can data analysis benefit businesses?
Data analysis enables businesses to gain insights into customer behavior, market trends, and competitor strategies. It helps in optimizing operations, improving decision-making, identifying opportunities for growth, and enhancing overall performance.
What skills are required to become a data analyst?
To become a data analyst, one needs a strong understanding of statistics, mathematics, and programming. Skills in data manipulation, data visualization, problem-solving, and critical thinking are also valuable in this field.
What is the role of data analysis in research?
Data analysis is vital in research as it allows researchers to draw meaningful conclusions, validate hypotheses, and present findings. It helps in ensuring the reliability and validity of research studies.
Is data analysis only applicable to quantitative data?
No, data analysis can be applied to both quantitative and qualitative data. Statistical methods are mainly used for quantitative data analysis, while other techniques such as content analysis and thematic analysis are employed for qualitative data analysis.