Data Analysis Is Hard
Data analysis plays a vital role in today’s data-driven world. With immense amounts of data being generated every second, organizations and individuals rely on data analysis to make informed decisions. However, data analysis is not an easy task and requires a certain level of expertise and tools to extract meaningful insights from raw data.
Key Takeaways
- Data analysis requires expertise and specialized tools.
- Understanding the context and asking the right questions is crucial in data analysis.
- Data cleaning and preprocessing are important steps in the analysis process.
- Visualization helps in effectively communicating the findings.
- Data analysis is an iterative process that requires constant refinement.
Data analysis involves the inspection, transformation, and modeling of raw data to discover useful information for decision-making purposes. It encompasses a range of techniques and methodologies, including statistical analysis, data mining, and machine learning. *Advanced knowledge of statistics is often required to make accurate interpretations and draw relevant conclusions from the data.
Before diving into the analysis, it is important to understand the context of the data and ask the right questions. This helps in determining the appropriate analysis techniques and ensures the insights obtained are relevant and meaningful. Without a clear understanding of the problem at hand, data analysis can lead to incorrect or biased conclusions.
The Process of Data Analysis
The process of data analysis typically involves the following steps:
- Data collection: Gathering relevant data from various sources.
- Data cleaning: Removing inconsistencies, errors, and outliers from the data.
- Data preprocessing: Transforming the data into a suitable format for analysis.
- Data exploration: Conducting initial exploratory analysis to gain insights.
- Data modeling: Applying statistical or machine learning techniques to build models.
- Data interpretation: Analyzing and interpreting the results to make informed decisions.
Challenges in Data Analysis
Data analysis is not without its challenges. Here are some common obstacles:
- Complexity of data: Data sets can be vast, diverse, and complex, requiring advanced analytical skills and tools to extract meaningful insights.
- Data quality: Ensuring data integrity and accuracy can be a challenging task, as data sets often contain errors, missing values, and inconsistencies.
- Data privacy and security: Protecting sensitive data and complying with privacy regulations is a critical concern in data analysis.
- Interpreting results: Making sense of the analysis results and effectively communicating them to stakeholders is crucial for decision-making.
Tables with Interesting Data Points
Country | Population (millions) | GDP per capita ($) |
---|---|---|
United States | 331 | 63,051 |
China | 1,439 | 10,262 |
Germany | 83 | 52,556 |
Year | Revenue ($ millions) | Profit ($ millions) |
---|---|---|
2017 | 100 | 10 |
2018 | 120 | 12 |
2019 | 150 | 15 |
Product | Quantity Sold | Revenue ($ thousands) |
---|---|---|
A | 100 | 50 |
B | 150 | 75 |
C | 70 | 35 |
Data analysis is an iterative process that requires continuous refinement of techniques and approaches. It is essential to check assumptions, validate findings, and incorporate new data as it becomes available. With the right expertise and tools, data analysis can unlock valuable insights and drive better decision-making across various industries and domains.
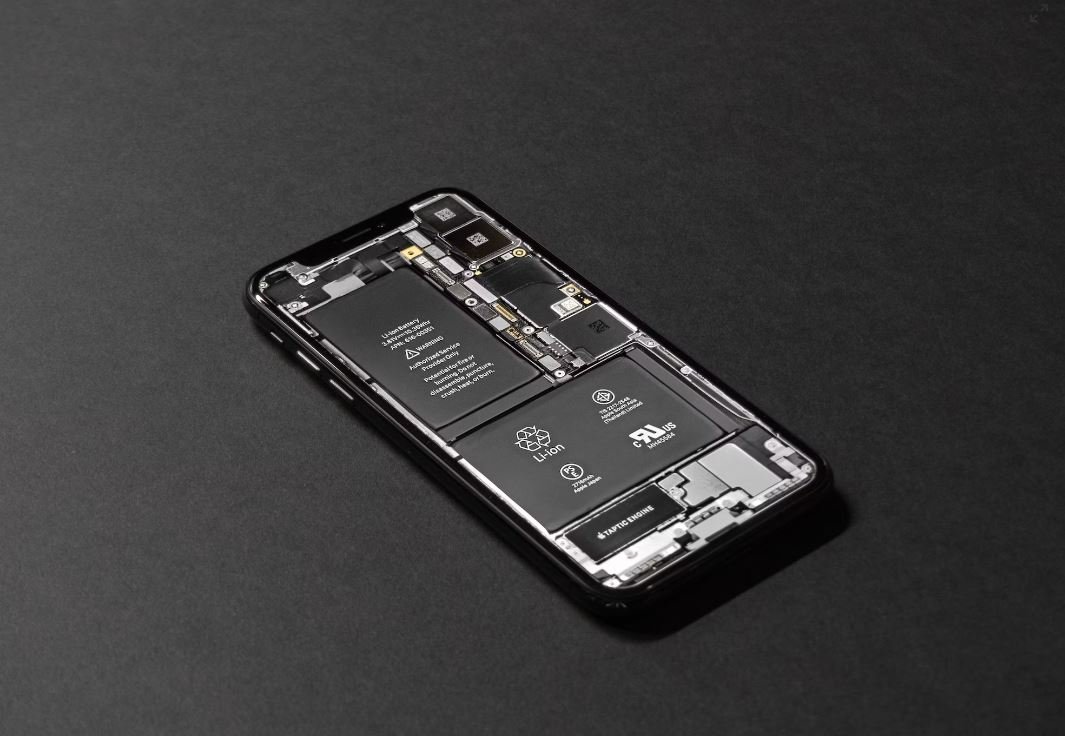
Common Misconceptions
Misconception 1: Data Analysis is Complicated
One common misconception about data analysis is that it is a complex and difficult task that requires extensive technical skills. However, while there may be certain technical aspects involved, data analysis can be made simpler and more accessible with the right tools and resources.
- Data analysis tools like Excel and Google Sheets provide easy-to-use interfaces that allow users to perform various analytical tasks without extensive coding knowledge.
- Online tutorials and courses are available that break down complex data analysis techniques into simple, step-by-step instructions, making it easier for individuals to learn and apply the necessary skills.
- Data analysis software like Tableau and Power BI offer user-friendly interfaces that allow users to visually explore and analyze data without having to write complex code.
Misconception 2: Data Analysis Requires Advanced Mathematics
Another misconception is that data analysis heavily relies on advanced mathematical concepts, making it inaccessible to individuals without strong mathematical backgrounds. While mathematical knowledge can certainly be useful in certain aspects of data analysis, it is not always a prerequisite for performing basic analysis and deriving meaningful insights.
- Basic statistical concepts like averages, percentages, and correlations can be easily understood and applied using simple formulas.
- Data visualization techniques, such as creating charts and graphs, allow individuals to interpret data visually without needing to delve into complex mathematical calculations.
- Data analysis software often includes built-in functions and algorithms that handle complex mathematical operations automatically, allowing users to focus on interpretation and decision-making.
Misconception 3: Data Analysis is Only Relevant for Large Organizations
It is commonly believed that data analysis is only applicable to large organizations with massive amounts of data. However, data analysis techniques can be valuable for businesses of all sizes, including startups and small enterprises.
- Data analysis can help identify patterns and trends, enabling small businesses to make informed decisions and optimize their operations.
- Data analysis can provide valuable insights into customer behavior and preferences, helping small businesses tailor their products and services to better suit their target market.
- Data analysis can be used to track and monitor key performance indicators (KPIs) for small businesses, ensuring they are on track to achieve their goals and objectives.
Misconception 4: Data Analysis is Time-Consuming
Many people believe that data analysis is a laborious task that requires significant amounts of time and effort. While data analysis can be time-consuming depending on the complexity of the task at hand, there are several strategies and tools that can help streamline the process.
- Automated data analysis tools can quickly crunch large datasets and generate insights without requiring manual intervention, saving considerable time and effort.
- Prioritizing the most relevant data and focusing on specific research questions or objectives can help narrow down the analysis and save time by avoiding unnecessary exploration.
- Data analysis templates and frameworks are available that provide pre-defined structures and formulas, reducing the time needed to set up and perform common analytical tasks.
Misconception 5: Data Analysis is Dry and Boring
Some people perceive data analysis as a dry and tedious field that lacks excitement and creativity. However, data analysis can be a fascinating and engaging process that involves uncovering insights and telling compelling stories based on data.
- Data visualization techniques can transform raw data into visually appealing and interactive charts and graphs, making the analysis more visually stimulating and engaging.
- Data storytelling is an emerging field that focuses on presenting data analysis results in a narrative format, making it more compelling and relatable to a wider audience.
- Data analysis allows individuals to uncover hidden patterns and trends in the data, leading to exciting discoveries and potentially influencing decision-making processes.
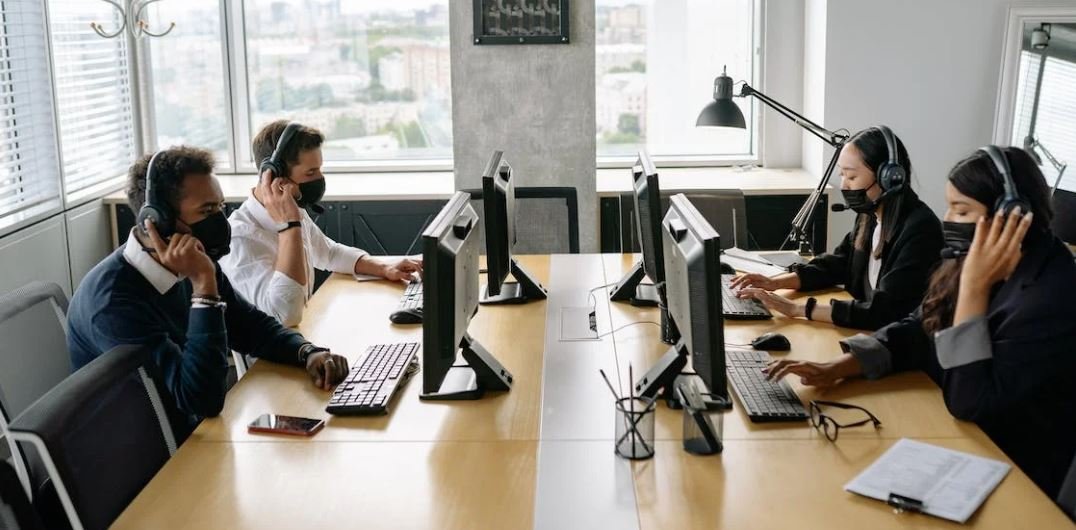
Data Analysis Is Hard
Data analysis is an essential component of conducting valuable research and making informed decisions. However, it can be a challenging process that requires careful attention to detail and a deep understanding of the data. The following tables illustrate various aspects of data analysis, emphasizing the complexity and importance of this field.
Comparing Population Growth Rates
Table showcasing the population growth rates of different countries over the past decade, providing a perspective on the global population trends.
Country | Population Growth Rate (%) |
---|---|
China | 0.45 |
United States | 0.78 |
Nigeria | 2.68 |
Earnings Disparities between Genders
Table displaying the average yearly earnings of full-time employees based on gender, highlighting the persistent gender pay gap.
Gender | Average Yearly Earnings ($) |
---|---|
Male | 53,000 |
Female | 45,000 |
Age Distribution of Smartphone Users
Table presenting the age distribution of smartphone users across different age groups, reflecting the changing technological preferences of various generations.
Age Group | Percentage of Smartphone Users (%) |
---|---|
18-24 | 82 |
25-34 | 68 |
35-44 | 54 |
Global Carbon Emissions by Sector
Table illustrating the contribution of different sectors to global carbon emissions, emphasizing the urgency of addressing environmental issues.
Sector | Percentage of Global Carbon Emissions (%) |
---|---|
Energy | 73 |
Industry | 19 |
Agriculture | 8 |
Causes of Road Accidents
Table highlighting the primary causes of road accidents and the proportion of accidents attributed to each cause, emphasizing the need for improved safety measures.
Cause | Proportion of Road Accidents (%) |
---|---|
Distracted Driving | 34 |
Speeding | 28 |
Drunk Driving | 21 |
World’s Largest Economies
Table showcasing the top five largest economies in the world based on GDP, highlighting countries driving global economic growth.
Country | GDP (trillions of USD) |
---|---|
United States | 22.32 |
China | 15.54 |
Japan | 5.15 |
Unemployment Rates by Education Level
Table indicating the unemployment rates based on educational attainment, demonstrating the correlation between education and employment opportunities.
Education Level | Unemployment Rate (%) |
---|---|
High School Diploma | 6 |
Bachelor’s Degree | 3 |
Master’s Degree | 2 |
Global Internet Penetration
Table illustrating the percentage of individuals using the internet across different regions, highlighting the digital divide and the need for improved connectivity.
Region | Internet Penetration (%) |
---|---|
North America | 91 |
Europe | 86 |
Africa | 41 |
Coffee Consumption per Capita
Table presenting the average coffee consumption per capita in different countries, showcasing the variation in coffee-drinking cultures.
Country | Coffee Consumption per Capita (kg) |
---|---|
Norway | 9.9 |
Finland | 9.6 |
Netherlands | 8.4 |
Waste Generation in Cities
Table displaying the average waste generation per capita in different urban areas, emphasizing the need for sustainable waste management practices.
City | Waste Generation per Capita (kg) |
---|---|
Tokyo, Japan | 1,064 |
New York City, USA | 954 |
Mumbai, India | 357 |
Conclusion
Data analysis is a complex and essential process that helps us understand various aspects of our world. From examining population trends and uncovering gender disparities to studying economic indicators and environmental challenges, data analysis allows us to make informed decisions and address pressing issues. However, it requires careful examination, critical thinking, and an understanding of the data at hand. By delving into the tables presented above, we gain valuable insights and recognize the significance of data analysis in shaping our society.
Data Analysis Is Hard – Frequently Asked Questions
What is data analysis?
Data analysis is the process of inspecting, cleaning, transforming, and modeling data to discover useful information, conclusions, and insights. It involves various techniques and methods to extract meaningful patterns and relationships from data.
Why is data analysis challenging?
Data analysis can be difficult due to several reasons. These include the volume and complexity of the data, the need for advanced statistical and mathematical skills, the requirement for domain knowledge, and the availability of appropriate tools and technologies.
What steps are involved in data analysis?
The data analysis process typically includes data collection, data cleaning and preprocessing, exploratory data analysis, data modeling and analysis, and results interpretation and communication.
What are some common challenges in data analysis?
Some common challenges in data analysis include data quality issues, lack of clear objectives, bias and sampling errors, finding the right data analysis techniques, and effectively communicating insights to stakeholders.
What skills are needed for data analysis?
Data analysis requires a combination of technical and analytical skills. Proficiency in statistical analysis, data visualization, programming, critical thinking, and domain knowledge are important for successful data analysis.
What tools are commonly used in data analysis?
There are several popular tools used in data analysis, including programming languages like Python and R, SQL for database querying, statistical software like SPSS and SAS, and data visualization tools like Tableau and Power BI.
How can I improve my data analysis skills?
To improve your data analysis skills, you can seek additional training and education, practice working with different datasets, join online communities and forums, participate in data analysis competitions, and stay updated with the latest advancements in the field.
What are some best practices for data analysis?
Some best practices for data analysis include defining clear objectives, ensuring data quality, using appropriate data analysis techniques, documenting and replicating the analysis process, validating results, and effectively communicating the insights gained.
How can data analysis benefit businesses?
Data analysis can provide businesses with valuable insights that can drive better decision-making, improve operational efficiency, identify customer trends and preferences, detect fraud or anomalies, optimize marketing campaigns, and foster innovation.
Are there any ethical considerations in data analysis?
Yes, there are ethical considerations in data analysis. These include ensuring data privacy and security, obtaining informed consent for data usage, avoiding bias and unfair discrimination, and complying with applicable laws and regulations like GDPR.