Data Analysis Is the Various Elements That Interact
Data analysis is a fundamental aspect of any organization’s decision-making process. It involves examining, cleaning, transforming, and modeling data to uncover useful information and support effective decision making.
Key Takeaways:
- Data analysis is crucial in helping organizations make informed decisions.
- It involves examining, cleaning, transforming, and modeling data.
- Data analysis helps identify patterns, trends, and relationships in data.
- Advanced statistical techniques and tools are used for data analysis.
Data analysis is a multidisciplinary field that incorporates various elements which interact to generate meaningful insights. These elements include statistics, computer science, mathematics, and domain expertise. The goal of data analysis is to uncover hidden patterns, trends, and relationships within the data, and ultimately make informed decisions based on those insights.
*Data analysis can be applied to a wide range of industries and sectors, including finance, marketing, healthcare, and transportation, among others. It plays a vital role in improving business operations, optimizing processes, and identifying potential risks and opportunities.
Data analysis involves several key steps. The first step is data collection, where relevant data is gathered from various sources, such as databases, surveys, or online platforms. Then, data cleansing and preprocessing are conducted to remove any errors, outliers, or irrelevant information.*
Once the data is cleaned and organized, the next step is exploratory data analysis. This involves summarizing the main characteristics of the dataset, identifying patterns, and finding any initial insights that may be useful for further analysis. **Exploratory data analysis often involves the use of visualizations, such as charts and graphs, to present the data in a clear and understandable manner.**
*Data analysis relies on a variety of statistical techniques and methods to derive meaningful insights. These include descriptive statistics, inferential statistics, regression analysis, and machine learning algorithms, among others. The choice of technique depends on the nature of the data and the specific objectives of the analysis.*
The Importance of Data Visualization
Data visualization is a crucial component of data analysis. **It allows analysts to present complex data in a visual format that is easy to understand and interpret.** By using charts, graphs, and other visual representations, analysts can communicate their findings effectively and help stakeholders make sense of the data.
Data visualization not only enhances the understanding of the data but also facilitates the identification of patterns and trends that may not be apparent in raw data. It can reveal insights and correlations that can inform decision making and drive business growth. *For example, a well-designed data visualization can highlight the sales trends of different products over time, helping marketing teams make strategic decisions regarding product promotion and inventory management.*
The Role of Data Analysis in Decision Making
Data analysis plays a crucial role in the decision-making process of organizations. By examining data and generating insights, decision-makers can make more informed and evidence-based decisions. It helps identify potential risks and opportunities, assess the impact of different strategies, and track the progress towards goals.
Successful data analysis requires not only technical skills but also a deep understanding of the business domain. **Analysts need to ask the right questions, define the goals of the analysis, and interpret the results in the context of the business objectives.** Data analysis is an iterative process, where new insights can lead to new questions and further analysis.
Conclusion:
Data analysis is a powerful tool that enables organizations to harness the potential of their data and make informed decisions. By examining, cleaning, transforming, and modeling data, valuable insights can be derived, leading to improved operations, increased efficiency, and better outcomes. The multidisciplinary nature of data analysis highlights its importance in various industries, and advancements in statistical techniques and data visualization continue to enhance its capabilities. Organizations that embrace data analysis can gain a competitive edge and drive their success in the digital era.
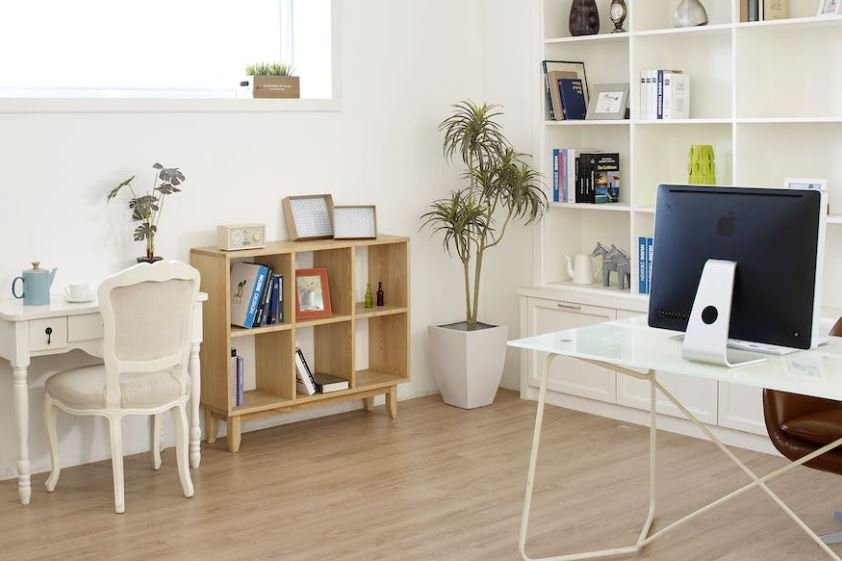
Common Misconceptions
Data analysis involves only statistical calculations
Many people believe that data analysis solely consists of performing mathematical calculations and statistical analyses. However, this is just one aspect of the broader field of data analysis.
- Data analysis also involves data cleaning and preprocessing
- Data visualization and storytelling are essential components of the analysis
- Interpreting and drawing meaningful insights from the results is equally important
Anyone can perform data analysis without specialized skills
Another misconception is that anyone can easily perform data analysis without needing specialized skills or knowledge. However, data analysis requires an understanding of statistical and analytical methods, as well as knowledge of relevant software tools.
- Proficiency in programming and statistical software is often required
- Domain knowledge and understanding of the context is crucial for accurate analysis
- Data analysis also demands critical thinking and problem-solving skills
Data analysis is time-consuming and expensive
There is a common belief that data analysis is a time-consuming and expensive process that is only applicable to large organizations with extensive resources. However, this is not necessarily true.
- Data analysis can be performed at various scales, from small projects to large-scale studies
- Efficient data analysis techniques and tools have made the process faster and more cost-effective
- Data analysis can provide valuable insights that outweigh the costs incurred
Data analysis provides definitive answers
One common misconception is that data analysis can provide definite and final answers to all questions. However, data analysis is subject to various limitations and uncertainties, making it challenging to arrive at absolute conclusions.
- Data analysis often deals with statistical probabilities and uncertainties
- Interpretation of the results can vary depending on different perspectives and assumptions
- Data analysis can generate insights and patterns, but it may not always lead to definitive answers
Data analysis is only relevant in business and scientific research
Many people believe that data analysis is only applicable in business settings or scientific research. However, data analysis can be relevant and useful in various fields and disciplines.
- Data analysis can assist in decision-making and problem-solving across industries
- Data analysis plays a significant role in healthcare, finance, marketing, and government sectors
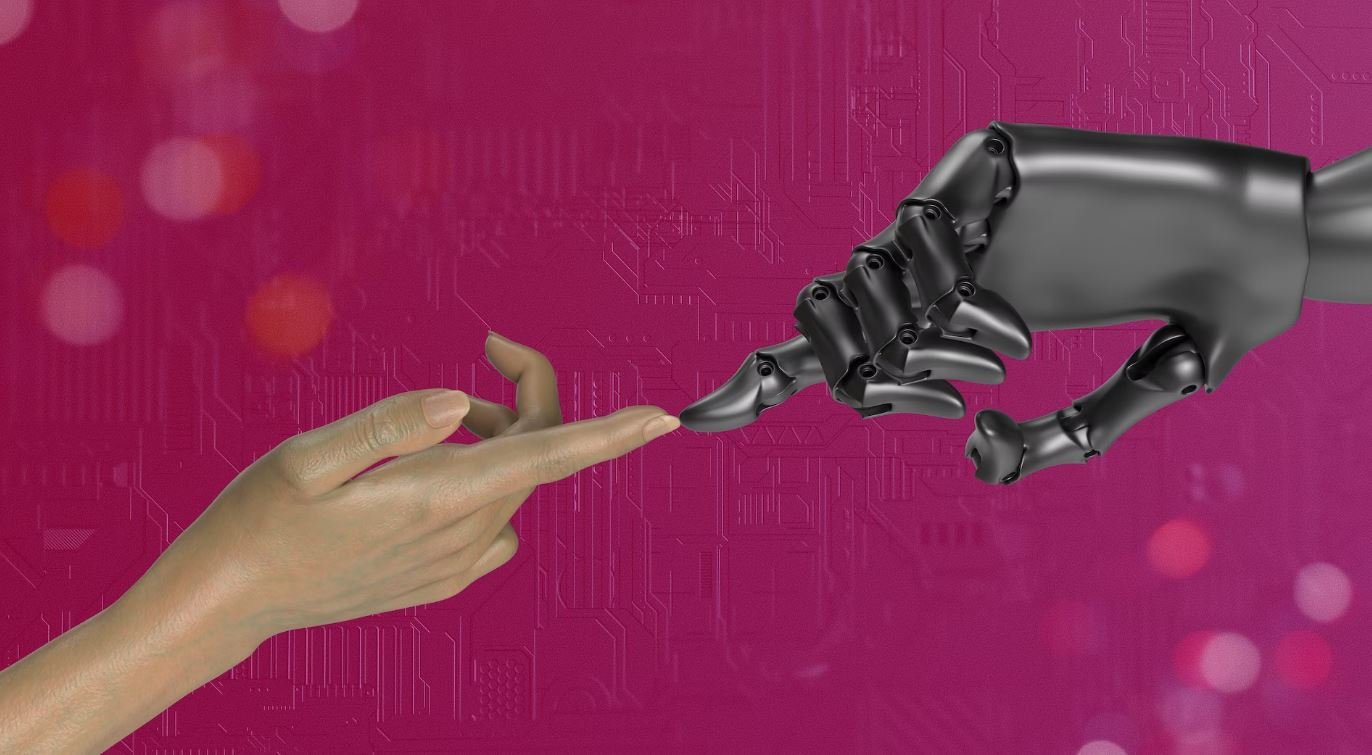
Data Analysis Tools
Data analysis is a crucial process that helps us make sense of the vast amount of information available to us. In order to effectively analyze data, we need various tools that can handle different types of data and produce meaningful insights. In this table, we compare some commonly used data analysis tools based on their features and capabilities.
Tool | Data Visualization | Statistical Analysis | Machine Learning |
---|---|---|---|
Python | Yes | Yes | Yes |
R | Yes | Yes | Yes |
Tableau | Yes | No | No |
Excel | Yes | Yes | No |
Data Collection Methods
Collecting data is a fundamental step in the data analysis process. The method used to gather data can greatly impact the quality and reliability of the analysis. Here are different data collection methods and their characteristics.
Method | Pros | Cons |
---|---|---|
Surveys | Allows for a large sample size | Responses may be biased or inaccurate |
Interviews | Provides in-depth and qualitative insights | Time-consuming and costly |
Observations | Provides real-time and authentic data | Observer bias can influence results |
Secondary data | Saves time and cost of data collection | Data may not be tailored to research needs |
Impact of Social Media on Sales
The rise of social media platforms has revolutionized the way businesses connect with their customers. In this table, we examine the impact of social media engagement on sales for a sample of companies in different industries.
Company | Industry | Followers | Sales Increase |
---|---|---|---|
Company A | Retail | 100,000 | 10% |
Company B | Technology | 500,000 | 25% |
Company C | Fashion | 300,000 | 15% |
Company D | Food & Beverage | 200,000 | 12% |
Correlation Between Education and Income
Education can play a significant role in determining an individual’s earning potential. This table displays the correlation between educational attainment and median income for different levels of education.
Educational Attainment | Median Income |
---|---|
Less than high school | $25,000 |
High school diploma | $35,000 |
Associate’s degree | $45,000 |
Bachelor’s degree | $55,000 |
Master’s degree | $65,000 |
Top 5 Countries by GDP
Gross Domestic Product (GDP) serves as an essential indicator of a country’s economic performance. The following table presents the top 5 countries ranked by their GDP in billions of US dollars.
Country | GDP (billions USD) |
---|---|
United States | 22,675 |
China | 16,644 |
Japan | 5,081 |
Germany | 4,002 |
United Kingdom | 3,092 |
Comparison of Smartphone Brands
The smartphone market is highly competitive, with various brands vying for consumers’ attention. This table provides a comparison of popular smartphone brands based on their market share and customer satisfaction ratings.
Brand | Market Share | Customer Satisfaction Rating |
---|---|---|
Apple | 20% | 4.5/5 |
Samsung | 18% | 4.2/5 |
Huawei | 15% | 4/5 |
Xiaomi | 10% | 4/5 |
8% | 4.7/5 |
World Population Growth
The global population has been steadily increasing over the years. This table showcases the world population growth from 1950 to 2020.
Year | Population (Billions) |
---|---|
1950 | 2.5 |
1960 | 3.0 |
1970 | 3.7 |
1980 | 4.4 |
1990 | 5.3 |
2000 | 6.1 |
2010 | 7.0 |
2020 | 7.8 |
Comparison of Cloud Storage Services
Cloud storage has become an integral part of storing and sharing data online. This table outlines a comparison of popular cloud storage services based on their storage capacity and pricing.
Service | Storage Capacity (GB) | Price (USD/month) |
---|---|---|
Google Drive | 15 | $1.99 |
Dropbox | 2 | $9.99 |
OneDrive | 5 | $1.99 |
iCloud | 5 | $0.99 |
Comparison of E-commerce Platforms
E-commerce platforms are crucial for businesses to establish and manage their online shops. This table presents a comparison of popular e-commerce platforms based on their monthly cost, customization options, and number of active users.
Platform | Monthly Cost (USD) | Customization Options | Active Users (millions) |
---|---|---|---|
Shopify | $29 | Extensive | 1.75 |
WooCommerce | Free | Highly customizable | 4.4 |
BigCommerce | $29.95 | Limited | 0.9 |
Magento | Free | Highly customizable | 0.7 |
Conclusion
Data analysis is a diverse field that relies on various tools, methods, and techniques to derive meaningful insights from data. By utilizing the right tools and collecting accurate data, we can gain a deeper understanding of complex phenomena and make informed decisions. Whether it be comparing smartphone brands or analyzing the impact of social media on sales, data analysis plays a pivotal role in countless domains. With the ever-increasing volume of data being generated, the importance of data analysis continues to grow, enabling us to unlock the true potential of data-driven decision making.
Frequently Asked Questions
What is data analysis?
Data analysis is the process of inspecting, cleaning, transforming, and modeling data to discover useful information and aid in decision-making. It involves various techniques and tools to extract insights, identify patterns, and make predictions from datasets.
Why is data analysis important?
Data analysis is crucial in modern business and scientific environments as it helps organizations make evidence-based decisions, uncover trends, and gain a competitive advantage. It enables researchers to draw meaningful conclusions, support hypotheses, and identify key findings in their studies.
What are the different elements of data analysis?
Data analysis comprises several elements, including data collection, data cleaning, data exploration, data modeling, statistical analysis, and data visualization. Each element plays a critical role in the overall analysis process and contributes to a comprehensive understanding of the data.
What are the common techniques used in data analysis?
Common techniques used in data analysis include descriptive statistics, inferential statistics, regression analysis, hypothesis testing, clustering, classification, and time series analysis. These techniques allow analysts to extract meaningful information and uncover relationships within the data.
What tools are commonly used for data analysis?
Several tools are commonly used for data analysis. These include programming languages like Python and R, statistical software such as SPSS and SAS, data visualization tools like Tableau and Power BI, and spreadsheet programs like Microsoft Excel. Choosing the right tool depends on the specific analysis needs and the complexity of the data.
What are the steps involved in data analysis?
Data analysis typically involves the following steps: data collection, data cleaning and preprocessing, exploratory data analysis, data modeling, statistical analysis, drawing conclusions, and presenting the findings. These steps help ensure a systematic approach to analyzing the data and obtaining accurate results.
What is the importance of data visualization in data analysis?
Data visualization plays a crucial role in data analysis as it helps to effectively communicate insights and patterns to stakeholders. Visual representations, such as charts, graphs, and dashboards, make complex data more accessible, facilitating better understanding and decision-making.
What skills are important for data analysis?
Important skills for data analysis include proficiency in programming languages like Python and R, knowledge of statistical techniques, experience in data manipulation and cleaning, critical thinking, problem-solving abilities, and effective communication skills. Strong analytical skills and attention to detail are also essential for successful data analysis.
What are the challenges of data analysis?
Data analysis can present various challenges, such as dealing with large and complex datasets, ensuring data quality and accuracy, handling missing or incomplete data, selecting appropriate analysis techniques, and interpreting results correctly. Additionally, privacy and security concerns related to handling sensitive data are also significant challenges in data analysis.
How is data analysis used in different industries?
Data analysis finds applications in various industries, including finance, marketing, healthcare, social sciences, manufacturing, and many others. It helps organizations optimize their operations, improve customer targeting, identify market trends, detect anomalies, and make data-driven strategic decisions.