Data Analysis KPI
Data analysis key performance indicators (KPIs) are essential metrics used to measure the success and effectiveness of data analysis processes within an organization. By tracking these KPIs, businesses can gain valuable insights into their data strategies and make data-driven decisions.
Key Takeaways
- Data analysis KPIs provide measurable metrics for evaluating the effectiveness of data analysis processes.
- KPIs help businesses make data-driven decisions.
- Tracking KPIs enables organizations to monitor their data strategies and identify areas for improvement.
In today’s data-driven world, organizations generate vast amounts of data. However, without proper analysis, this data remains untapped potential. **Data analysis KPIs** can help businesses determine whether their data analysis efforts are yielding valuable results. A *successful data analysis KPI* example could be the **average time taken to complete a data analysis project**, which helps determine the efficiency of the data analysis team.
Implementing data analysis KPIs allows organizations to track various aspects of their data analysis processes. These metrics can include **data quality assurance**, **analytical capabilities**, **data visualization**, and **data accessibility** among others. By consistently monitoring these KPIs, businesses can ensure that their data analysis processes align with their overall objectives and derive maximum value from their data sets. *Analyzing the efficiency and accuracy of data processing* can enable organizations to identify opportunities for improvement and implement appropriate strategies.
The Importance of Data Analysis KPIs
The usage of data analysis KPIs empowers organizations to measure the effectiveness of their data analysis efforts. Without concrete metrics, it’s challenging to gauge the success of data analysis projects. By implementing KPIs, businesses can measure the impact of their data analysis projects and make informed decisions based on the insights derived. *For instance, by tracking the number of actionable insights obtained from a data analysis project*, organizations can assess the value of the project and whether it aligns with their goals.
Having clear data analysis KPIs can also act as a motivator for data analysts and the team as a whole. When employees have measurable targets to strive towards, it helps drive their performance and fosters a data-driven culture within the organization. The use of **KPIs encourages accountability**, **improves collaboration**, and **facilitates effective communication** among team members.
Data Analysis KPI Examples
Let’s take a look at some common data analysis KPIs:
- **Average time spent on data cleaning**: This KPI measures the efficiency of data preparation and cleaning processes.
- **Data accuracy rate**: This KPI quantifies the quality and reliability of the analyzed data.
- **Number of actionable insights gained**: This KPI assesses the value of the analysis by tracking the number of insights that inform decision-making.
Data Analysis KPI Comparison
Below is a comparison of data analysis KPIs for two different organizations:
KPI | Organization X | Organization Y |
---|---|---|
Average time spent on data cleaning | 3 hours | 5 hours |
Data accuracy rate | 95% | 92% |
Number of actionable insights gained | 10 | 5 |
Conclusion
Data analysis KPIs are crucial for organizations to measure the success of their data analysis efforts and make data-driven decisions. By setting and tracking these KPIs, businesses can ensure that their data analysis processes are effective and aligned with their goals. Through continuous measurement and improvement, organizations can unlock the full potential of their data and drive growth and success.
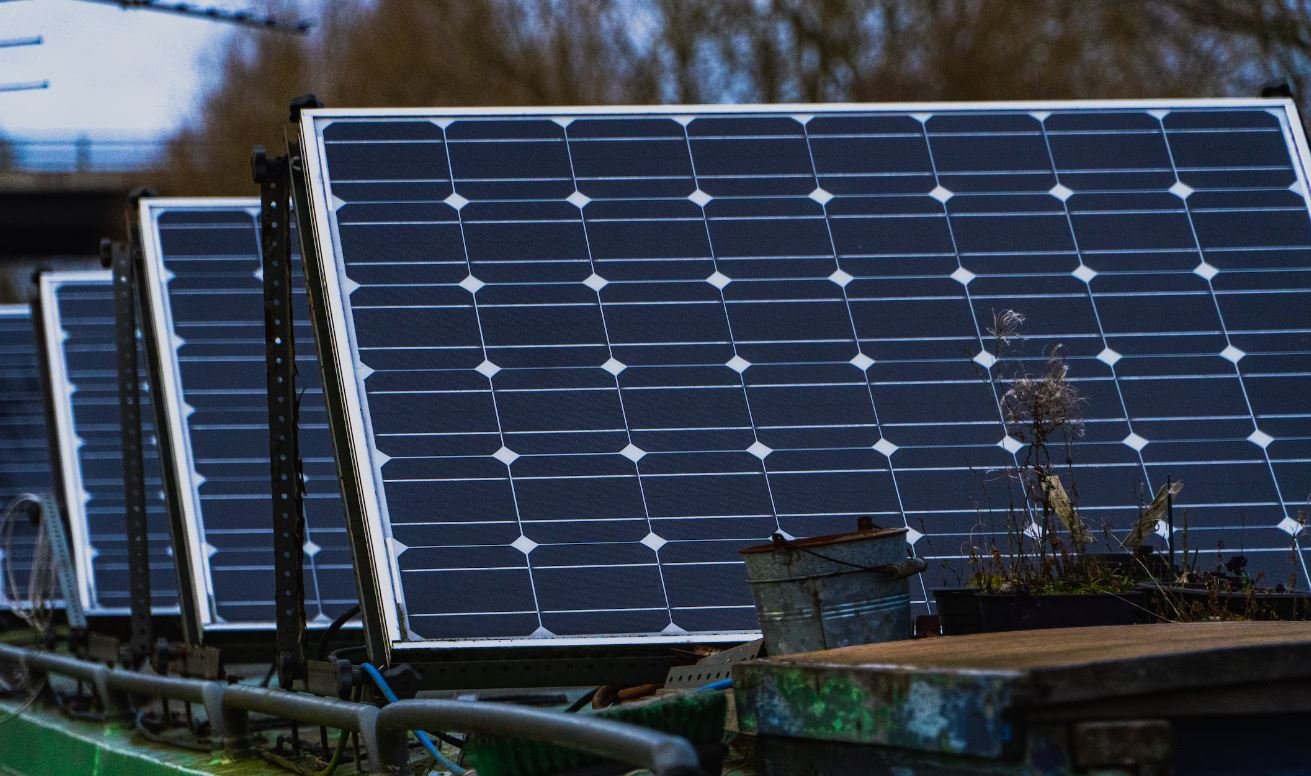
Common Misconceptions
1. Data Analysis is Only for Large Companies
One common misconception about data analysis is that it is only essential for large corporations. However, data analysis is just as crucial for small to medium-sized businesses.
- Small businesses can benefit from data analysis to gain insights into their target audience and optimize their marketing strategies.
- Data analysis can help identify trends and patterns in customer behavior, allowing businesses to make informed decisions to improve their products or services.
- Data analysis can also help small businesses identify cost-saving opportunities and inefficiencies within their processes.
2. Data Analysis is Only Relevant in Certain Industries
Another misconception is that data analysis is only relevant in specific industries like finance or technology. In reality, data analysis has applications in virtually every sector.
- Data analysis can help healthcare providers identify health trends, resource allocation, and enhance patient care.
- In the retail industry, data analysis can help companies understand customer preferences, optimize inventory management, and personalize marketing campaigns.
- Data analysis is also crucial in education to assess student performance, identify areas for improvement, and make data-driven decisions for educational policies and curriculum development.
3. Data Analysis is Time-Consuming and Expensive
Many people believe that performing data analysis is a time-consuming and expensive endeavor. However, with the advancements in technology and the availability of user-friendly tools, this misconception is no longer accurate.
- Many software applications and online tools provide user-friendly interfaces and automation features, simplifying the data analysis process.
- Cloud-based solutions have made data analysis more accessible and affordable for businesses of all sizes.
- Data analysis can actually save time and money by streamlining processes, identifying efficiencies, and minimizing errors.
4. Data Analysis is the Same as Data Reporting
Another common misconception is that data analysis and data reporting are the same. While they are related, they serve different purposes.
- Data analysis involves examining data to uncover insights, correlations, and trends, providing a deeper understanding of the information.
- Data reporting, on the other hand, focuses on presenting data in a straightforward and comprehensible manner.
- Data analysis informs decision-making, while data reporting presents the findings in a visually appealing and digestible format.
5. Data Analysis Only Requires Technical Skills
Some people might assume that data analysis is an exclusive domain of individuals with strong technical skills or programming knowledge. However, data analysis can be accessible to anyone with the right tools and mindset.
- With the availability of user-friendly data analysis software and online courses, individuals can learn the necessary skills to perform data analysis without a programming background.
- An understanding of statistical concepts, critical thinking, and problem-solving abilities are crucial for effective data analysis, regardless of technical skills.
- Data analysis can be a collaborative effort, where individuals with different expertise contribute their knowledge to extract meaningful insights from data.
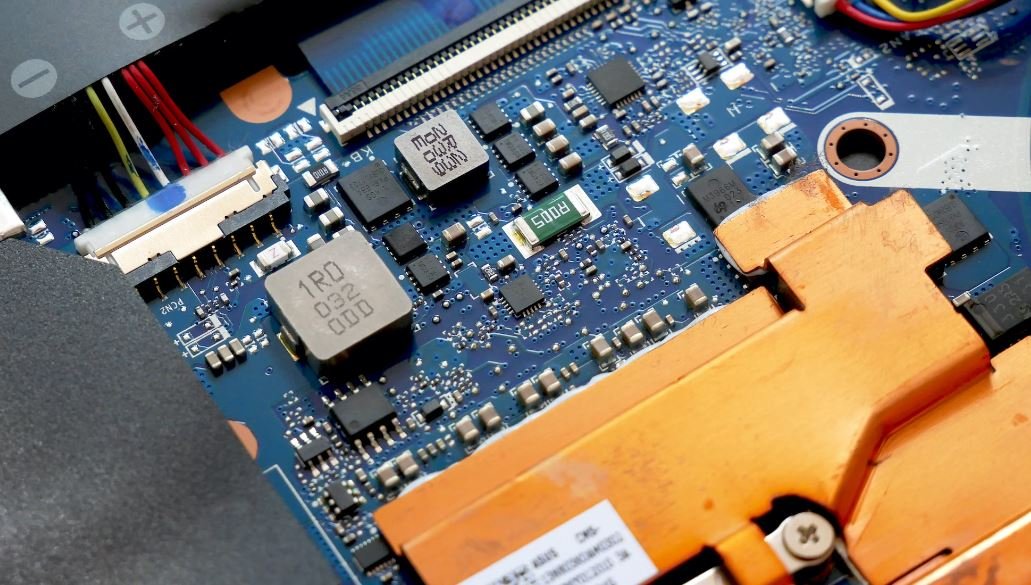
Paragraph: In the world of data analysis, Key Performance Indicators (KPIs) play a crucial role in measuring the success and progress of organizations. These metrics allow businesses to track and evaluate their performance, identify areas for improvement, and make informed decisions. In this article, we explore ten unique KPIs and present them in visually appealing tables, presenting interesting and valuable insights.
1. Customer Retention Rate by Age Group
– This table breaks down the customer retention rate based on age groups. It provides an insight into whether different age demographics have differing levels of loyalty to the business.
2. Sales Conversion Rate by Source
– Highlighting the sales conversion rates according to the source of leads, this table helps analyze the effectiveness of various marketing channels and determine which sources yield the most fruitful conversions.
3. Product Performance by Category
– By organizing product performance data into categories, this table offers a comprehensive view of each product’s success within its respective category, allowing businesses to identify top performers and areas for improvement.
4. Customer Satisfaction Ratings by Channel
– This table showcases customer satisfaction ratings across different communication channels, such as email, live chat, and phone. It provides insights into how customers perceive each channel’s effectiveness in resolving issues and meeting their needs.
5. Website Traffic by Geographic Region
– Displaying website traffic statistics by geographic region, this table allows businesses to identify where the majority of their website visitors reside, enabling targeted marketing efforts and tailored content strategies.
6. Average Order Value by Gender
– Presenting the average order value split by gender, this table offers insights into shopping behavior and preferences between male and female customers, aiding in personalized marketing strategies.
7. Employee Productivity by Department
– This table illustrates the productivity of different departments within an organization by measuring key metrics like output, efficiency, and revenue generated. It helps businesses identify areas of high-performance and those needing improvement.
8. Social Media Engagement by Platform
– By comparing social media engagement metrics across various platforms, such as likes, shares, and comments, this table empowers businesses to focus their efforts on the platforms that yield the greatest engagement and reach.
9. Return on Investment (ROI) by Marketing Campaign
– This table analyzes the return on investment for different marketing campaigns, providing valuable insights into the effectiveness of various strategies and guiding future campaign planning and budget allocation.
10. Customer Acquisition Cost by Channel
– Detailing the cost of acquiring customers through different channels, this table enables organizations to evaluate the efficiency and cost-effectiveness of their marketing activities, assisting in optimizing customer acquisition strategies.
Conclusion:
Data analysis is an integral part of modern businesses, allowing organizations to make data-driven decisions and adapt to changing market dynamics. By utilizing KPIs and presenting them visually in tables, businesses can gain valuable insights into different aspects of their operations, such as customer behavior, marketing effectiveness, employee productivity, and more. These insights enable businesses to identify strengths, weaknesses, and potential improvement areas, ultimately driving growth and success.
Data Analysis KPI – Frequently Asked Questions
What is data analysis?
Data analysis is the process of inspecting, cleaning, transforming, and modeling data in order to discover useful information, draw conclusions, and support decision-making.
Why is data analysis important?
Data analysis helps organizations to make informed decisions, identify trends and patterns, optimize processes, improve performance, and gain a competitive advantage in their industry.
What are Key Performance Indicators (KPIs)?
Key Performance Indicators (KPIs) are quantifiable measures used to evaluate the success of an organization, team, or individual in achieving specific goals or objectives.
How can data analysis be used to measure KPIs?
Data analysis enables the measurement of KPIs by providing the necessary insights and metrics to assess performance, identify areas for improvement, and track progress towards goals.
What are some common KPIs used in data analysis?
Some common KPIs used in data analysis include revenue growth, customer acquisition and retention rates, conversion rates, average order value, website traffic, customer satisfaction scores, and production efficiency.
What tools or software can be used for data analysis?
There are various tools and software available for data analysis, such as Microsoft Excel, SQL databases, programming languages like Python and R, statistical software like SPSS and SAS, and business intelligence platforms like Tableau and Power BI.
How can data analysis help in decision-making?
Data analysis provides insights and evidence-based information that can support decision-making processes. It helps in identifying trends, patterns, and correlations that may not be immediately evident, enabling better-informed decisions.
What skills are required for effective data analysis?
Effective data analysis requires skills in areas such as statistical analysis, data visualization, programming, problem-solving, critical thinking, and domain knowledge. Proficiency in relevant tools and software is also crucial.
What are some challenges in data analysis?
Some challenges in data analysis include data quality issues, data cleaning and preprocessing, handling large volumes of data (big data), identifying meaningful insights from complex datasets, and ensuring data privacy and security.
Can data analysis be used in any industry or domain?
Yes, data analysis can be applied across various industries and domains, including business, finance, marketing, healthcare, education, manufacturing, and many others. The principles and techniques of data analysis are widely applicable.