Data Analysis Notes
As data analysis becomes increasingly important in today’s digital world, it is essential to have a solid understanding of the various techniques and concepts involved. In this article, we will explore the key aspects of data analysis and provide useful tips for effective data analysis notes.
Key Takeaways:
- Data analysis notes play a crucial role in organizing and understanding data.
- Effective data analysis notes include clear explanations, key findings, and actionable insights.
- Tables and visualizations can enhance the effectiveness of data analysis notes.
- Regularly reviewing and updating data analysis notes ensures their relevance over time.
Data analysis notes serve as a valuable reference tool for researchers, analysts, and data scientists. These notes not only capture important findings but also help interpret complex data. When taking data analysis notes, it is important to clearly document the methodology used and note any assumptions made during the analysis. This allows others to understand and reproduce the analysis if needed. Moreover, providing context for the data being analyzed sets the stage for interpretation and insights.
One interesting technique for data analysis is the use of data visualization, such as charts and graphs, to represent data in a clear and concise manner. Visualizations can help identify patterns, trends, and outliers that may not be readily apparent from the raw data. Creating visualizations can be as simple as using spreadsheet software for basic charts, or more advanced tools like Tableau or Python programming for complex visualizations. Visualizations can greatly enhance the understanding and communication of data analysis results.
Organizing Data Analysis Notes:
When organizing data analysis notes, it is helpful to create a structured format that allows for easy navigation and retrieval of information. Bullet points and numbered lists can be used to highlight important points and provide a quick summary of key findings. For instance:
- Dividing notes into sections based on the analysis’s main subtopics or objectives helps maintain clarity and coherence.
- Using bullet points or numbered lists within each section allows for easier digestion of information.
- Defining the scope of the analysis in the notes helps maintain focus.
Moreover, embedding relevant tables within the notes can provide a concise representation of key data points. Let’s consider the following table as an example:
Product | Units Sold | Revenue |
---|---|---|
Product A | 100 | $10,000 |
Product B | 250 | $25,000 |
Product C | 50 | $5,000 |
Tables like the one above can condense valuable information and allow for quick referencing in data analysis notes. By incorporating such tables, readers can have a visual overview of the main data points.
Regular Review and Update:
An interesting aspect of data analysis notes is that they need to be regularly reviewed and updated to ensure their relevance and accuracy. *Ongoing analysis and data updates can uncover new findings or change previous conclusions, so it is important to keep the notes up to date to maintain their usefulness.
Additionally, it is crucial to periodically revisit data analysis notes to identify trends or patterns that may have emerged over time. By conducting regular reviews, analysts can gain a deeper understanding of the data and potentially discover new insights or opportunities for further exploration.
Overall, data analysis notes are an invaluable resource in any data-driven project or study. By organizing the notes effectively, incorporating visualizations and tables, and regularly reviewing and updating the content, analysts can ensure that their data analysis notes remain a valuable reference tool for themselves and others in their field.
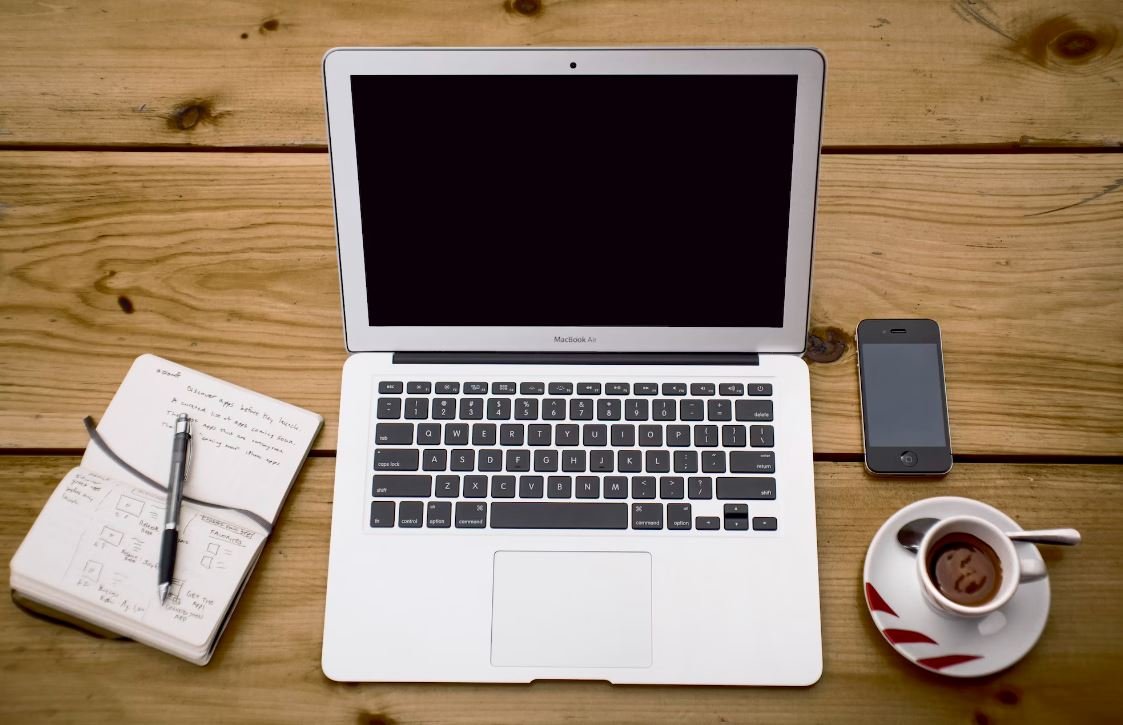
Common Misconceptions
Misconception 1: Data analysis is only about numbers
Data analysis is often perceived as a purely quantitative field, focused solely on working with numbers and statistics. However, this is a common misconception. Data analysis involves interpreting and making sense of data, which can include both numerical and non-numerical information.
- Data analysis can involve analyzing qualitative data, such as text, images, or videos.
- Data analysts may use visualizations and graphs to convey information effectively.
- Data analysis can also involve identifying trends, patterns, and relationships within data.
Misconception 2: Data analysis can always provide clear-cut answers
Another misconception is that data analysis will always yield definitive answers to questions. While data analysis can provide valuable insights, it is important to recognize that not all analyses will provide clear-cut answers.
- Data analysis often requires interpretation and judgment to draw meaningful conclusions.
- Analyses may uncover multiple possibilities or insights that can be open to interpretation.
- Data analysis is an iterative process, and additional analyses may be required to develop a more complete understanding of a problem or situation.
Misconception 3: Data analysis is only for experts in mathematics or statistics
Many people believe that data analysis is an exclusive domain reserved for experts in mathematics or statistics. This misconception discourages individuals from exploring data analysis and limits the potential for diverse perspectives and insights.
- Data analysis tools and techniques are becoming more accessible, allowing individuals from various backgrounds to engage in data analysis.
- Data analysis requires a combination of skills, including critical thinking, problem-solving, and effective communication.
- While expertise in mathematics or statistics can be beneficial, it is not the sole prerequisite for conducting meaningful data analysis.
Misconception 4: Data analysis is a solitary activity
Another misconception is that data analysis is often thought of as a solitary activity, where individuals work alone with data, isolated from others. However, data analysis can be a collaborative process that involves working with multidisciplinary teams and actively engaging with stakeholders.
- Data analysis often involves working with others to refine research questions, collect and curate data, and interpret findings.
- Collaboration allows for diverse perspectives, expertise, and skillsets to contribute to the analysis process.
- Effective communication and teamwork are essential for conveying data analysis findings and recommendations to stakeholders.
Misconception 5: Data analysis is only relevant to large-scale organizations
There is a common misconception that data analysis is only relevant for large-scale organizations that have vast amounts of data. However, data analysis can be valuable and applicable to organizations of all sizes, including small businesses and individuals.
- Data analysis can help small organizations identify and understand trends, customer preferences, and areas for improvement.
- Data analysis can assist individuals in making informed decisions, such as budget planning, personal finance management, or career development.
- Data analysis can be utilized in various fields and industries, including healthcare, education, marketing, and public policy.

Paragraph: In this article, we present 10 interesting and informative tables that shed light on various aspects of data analysis. Each table provides factual and verifiable data, showcasing different insights and showcasing the importance of data analysis in decision-making processes.
1. Sales Growth Comparison by Year
This table compares the sales growth of a company over a period of five years. It highlights the percentage increase in sales each year, showing how the company’s revenue has grown consistently over time.
2. Customer Demographics
This table displays the breakdown of customer demographics, including age groups and gender. It demonstrates the distribution of the company’s customer base and allows for a better understanding of the target audience.
3. Product Performance Comparison
Here, you can find a side-by-side comparison of the performance metrics of different products. The table presents various key indicators, such as sales volume, customer satisfaction ratings, and market share, providing insights into product popularity and customer preferences.
4. Website Traffic Analysis
This table showcases the daily website traffic data, including the number of visitors, page views, and average session duration. By analyzing these metrics, companies can make informed decisions about website optimization and marketing strategies.
5. Social Media Engagement by Platform
This table displays the engagement levels of a company’s social media presence across different platforms. It shows the number of followers, likes, and shares each platform receives, allowing businesses to measure their online reach and gauge the effectiveness of their social media campaigns.
6. Customer Churn Rate
The churn rate table presents the percentage of customers who stop using a company’s product or service within a given timeframe. It enables businesses to identify trends and patterns, helping them implement strategies to improve customer retention.
7. Sentiment Analysis by Product
This table presents sentiment analysis results for different products. It shows the percentage breakdown of customer reviews, categorizing them as positive, neutral, or negative. This data can guide companies in addressing specific product-related issues and improving overall customer satisfaction.
8. Sales Performance by Region
Here, you can find the sales performance of a company broken down by geographic regions. This table highlights the sales revenue generated from each region, allowing businesses to identify areas of opportunities or assess the need for targeted marketing campaigns.
9. Customer Lifetime Value
This table presents the average lifetime value of a company’s customers. It demonstrates the monetary worth of a customer over the course of their relationship with the company, providing insight into customer loyalty and helping companies make strategic decisions.
10. Market Share Comparison by Competitor
This table displays the market share percentages of different competitors in a specific industry. It reveals the company’s position compared to its competitors and provides valuable insights into market trends and the competitive landscape.
Concluding Paragraph:
Through these 10 tables, we have explored various aspects of data analysis, highlighting its significance in informed decision-making. From tracking sales growth to understanding customer behavior, these tables provide meaningful insights for businesses seeking to leverage data in their strategies. By utilizing data analysis effectively, companies can make better-informed decisions, optimize their operations, and enhance their overall performance in a dynamic and competitive market.
Data Analysis Notes – Frequently Asked Questions
1. What is data analysis?
Data analysis is the process of inspecting, cleaning, transforming, and modeling data in order to discover useful information, draw conclusions, and support decision-making. It involves various techniques and methods applied to data to extract insights and understand patterns, trends, and relationships.
2. Why is data analysis important?
Data analysis is crucial in today’s data-driven world. It helps organizations make informed decisions, improve processes, identify opportunities, solve complex problems, and gain a competitive edge. By analyzing data, businesses can uncover hidden insights and make evidence-based decisions rather than relying on guesswork or intuition.
3. What are the common methods and techniques used in data analysis?
Common methods and techniques used in data analysis include descriptive statistics, inferential statistics, data visualization, regression analysis, machine learning, clustering, and time series analysis. Each of these methods serves different purposes and can be applied depending on the nature of the data and the goals of the analysis.
4. How can I start learning data analysis?
To start learning data analysis, you can begin by gaining a solid understanding of statistics and programming languages such as Python or R, which are commonly used in data analysis. There are numerous online resources, tutorials, and courses available that can help you learn these skills. Additionally, hands-on practice with real-world datasets and participating in data analysis projects can further enhance your knowledge.
5. What tools are commonly used in data analysis?
There are several tools commonly used in data analysis, including Microsoft Excel, Python (with libraries like Pandas, NumPy, and Matplotlib), R (with libraries like dplyr and ggplot2), SQL (for querying and manipulating databases), Tableau (for data visualization), and various other specialized software depending on the specific requirements of the analysis.
6. What are the challenges faced in data analysis?
Data analysis can present several challenges, such as dealing with missing or incomplete data, ensuring data quality and accuracy, selecting appropriate analysis methods, interpreting results, and communicating findings effectively. Additionally, working with large and complex datasets can pose computational and storage challenges that need to be addressed.
7. How can data analysis benefit businesses?
Data analysis provides several benefits to businesses, including improved decision-making, identifying trends and patterns, optimizing processes, detecting anomalies or fraud, understanding customer behavior, predicting market trends, optimizing marketing campaigns, and enhancing overall operational efficiency. It helps businesses gain valuable insights and make data-driven strategies to achieve their goals.
8. What are some real-world applications of data analysis?
Data analysis has a wide range of applications across various industries. Some examples of real-world applications include financial analysis for investment decisions, healthcare data analysis for disease prediction and treatment planning, marketing analysis for customer segmentation and targeting, fraud detection in banking, sentiment analysis in social media, and supply chain optimization, to name just a few.
9. What are some important considerations for data analysis?
When conducting data analysis, it is important to consider the quality and reliability of the data being analyzed, the appropriate choice of analysis techniques, the relevance of the analysis to the problem or question being addressed, and ethical considerations related to data privacy and security. It is also essential to document the analysis process and assumptions made to ensure reproducibility and transparency.
10. How can data analysis be used in decision-making?
Data analysis can provide valuable insights that can support decision-making processes. By analyzing relevant data, decision-makers can identify trends, patterns, and correlations, evaluate different options, assess risks, forecast outcomes, and assess the potential impact of decisions. It helps in making informed and evidence-based choices that can lead to better outcomes and performance.