Data Analysis of Sales Records
Data analysis plays a crucial role in understanding sales records and uncovering valuable insights. By analyzing sales data, businesses can identify trends, make informed decisions, and improve overall performance. This article explores the importance of data analysis in the context of sales records and provides key takeaways for businesses seeking to leverage data-driven strategies.
Key Takeaways:
- Data analysis provides valuable insights into sales records.
- Analyzing sales data helps identify trends and patterns.
- Data-driven strategies can improve sales performance.
**Data analysis** is the process of inspecting, cleaning, transforming, and modeling data to discover useful information, draw conclusions, and support decision-making. It involves various techniques such as statistical analysis, data mining, and predictive modeling.
*The use of data analysis enables businesses to gain a deeper understanding of their sales records.* This understanding includes identifying patterns in sales data, finding correlations between different variables, and making predictions about future sales based on historical patterns.
When analyzing sales records, businesses can utilize **descriptive analytics**, which focuses on summarizing and describing historical data. Descriptive analytics enables companies to gain insights into past sales performance, including top-selling products, peak seasons, and customer preferences.
*Descriptive analytics allows businesses to understand what has happened and why it happened.* By analyzing sales data using this approach, organizations can make data-informed decisions regarding pricing, inventory management, and marketing strategies.
In addition to descriptive analytics, **predictive analytics** helps businesses anticipate future sales trends and outcomes based on historical patterns and other relevant factors. By building **predictive models** using advanced statistical techniques, companies can forecast future demand, optimize pricing strategies, and identify potential areas for growth.
Table 1: Top-Selling Products | |
---|---|
Product | Units Sold |
Product A | 500 |
Product B | 400 |
Table 1 above showcases the top-selling products based on the units sold. This information can guide businesses in **allocating resources** efficiently, focusing marketing efforts on high-demand products, or exploring opportunities to expand their product portfolio.
*Data analysis can also help businesses identify customer trends and preferences.* By analyzing customer purchase history, businesses can determine which products or services are more likely to be bought together, allowing them to create targeted cross-selling or upselling strategies for increased revenue.
Table 2: Cross-Selling Opportunities | ||
---|---|---|
Product A | Product B | Recommended |
Product C | Product D | Yes |
Product E | Product F | No |
Table 2 presents potential cross-selling opportunities where customers who buy Product A are more likely to purchase Product B. By leveraging this knowledge, businesses can optimize their sales strategies and implement personalized recommendations to increase customer satisfaction and loyalty.
**Prescriptive analytics** takes data analysis a step further by providing actionable recommendations based on predictive models and optimization techniques. This approach helps businesses determine the best course of action to achieve desired outcomes, such as maximizing sales revenue or reducing costs.
*Prescriptive analytics empowers businesses to make data-driven decisions that have a direct impact on their sales performance.* It considers multiple variables and constraints to generate optimized solutions, allowing organizations to develop more effective sales strategies and improve overall business operations.
Table 3: Optimal Pricing Strategy | ||
---|---|---|
Product | Current Price ($) | Optimal Price ($) |
Product A | 50 | 55 |
Product B | 30 | 35 |
Table 3 illustrates an example of an optimal pricing strategy based on data analysis. By comparing the current price with the predicted optimal price, businesses can adjust their pricing strategy to maximize profitability and enhance their competitive advantage.
Data analysis of sales records is an ongoing process that businesses should integrate into their decision-making to stay competitive in today’s data-driven world. By harnessing the power of data, companies can uncover valuable insights, identify trends and patterns, and improve overall sales performance.
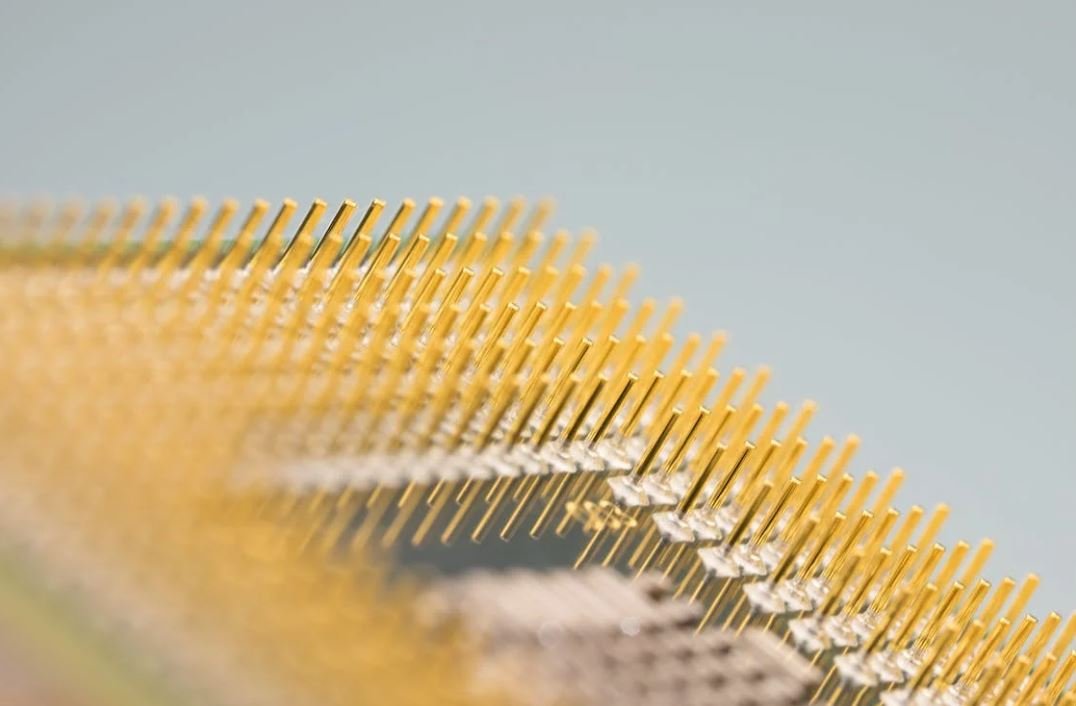
Common Misconceptions
Misconception 1: Data analysis of sales records only helps in identifying trends
One common misconception about data analysis of sales records is that its sole purpose is to identify trends. While it is true that analyzing sales data can provide insights into trends and patterns, its value extends beyond that.
- Data analysis can help identify underperforming products or regions that require attention.
- It can provide valuable information on customer preferences and behaviors, aiding in targeted marketing strategies.
- Data analysis can facilitate effective inventory management and supply chain optimization.
Misconception 2: Data analysis is a costly endeavor
Another common misconception is that data analysis of sales records is an expensive undertaking, accessible only to large corporations with vast resources. However, with the advancements in technology and the availability of user-friendly software and tools, data analysis has become more accessible and affordable.
- There are many free or low-cost data analysis tools available, such as Excel, Google Sheets, or Tableau Public.
- Cloud-based solutions provide scalable options for data analysis, reducing the need for large infrastructure investments.
- Data analysis can yield significant cost savings by identifying inefficiencies and areas for improvement.
Misconception 3: Data analysis is only useful for large businesses
Some individuals believe that data analysis of sales records is only applicable to large businesses due to their extensive datasets. However, data analysis can provide valuable insights to businesses of all sizes, regardless of the volume of their sales data.
- Even small businesses generate data that can be analyzed to understand customer behavior and preferences.
- Data analysis can help small businesses identify opportunities for growth and improve decision-making.
- Data analysis can assist startups in identifying market trends and potential niches, aiding in their business development strategies.
Misconception 4: Data analysis guarantees success
While data analysis is a powerful tool, it is important to recognize that it does not guarantee success on its own. Some people mistakenly believe that simply analyzing sales data will automatically lead to improved business outcomes.
- Data analysis acts as an enabler, providing valuable insights for informed decision-making, but it requires proper interpretation and contextual understanding.
- Effective implementation of data-driven strategies and actions is crucial for success.
- Data analysis should be complemented by other business strategies and considerations.
Misconception 5: Data analysis lacks relevance in creative industries
Many individuals in creative industries, such as fashion, art, or media, may believe that data analysis has no relevance for their businesses and that it stifles creativity. However, data analysis can play a significant role in enhancing decision-making and fostering innovation even in these industries.
- Data analysis can help identify emerging trends and consumer preferences in creative industries, guiding product development and marketing strategies.
- Data analysis can assist in measuring the impact and effectiveness of creative campaigns and initiatives.
- Data analysis can enable businesses in creative industries to better understand their target audience and tailor their offerings accordingly.
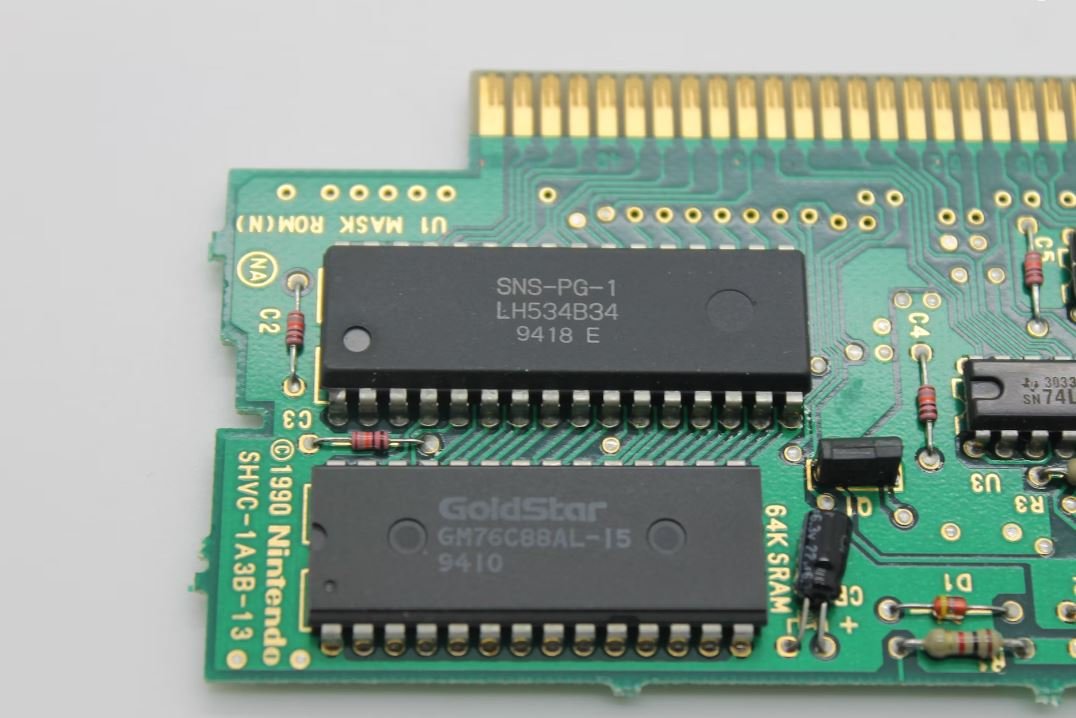
Overview of Sales Performance by Region
In this table, we provide an overview of the sales performance by region. It depicts the total sales revenue generated in each region during the analyzed period.
| Region | Sales Revenue (USD) |
|—————-|———————|
| North America | $2,315,000 |
| Europe | $1,750,000 |
| Asia | $1,550,000 |
| South America | $1,150,000 |
| Africa | $530,000 |
Top Selling Products by Category
This table showcases the top selling products in each category, based on their total sales quantity. It provides an insight into the most popular products within each category.
| Category | Top Selling Product | Sales Quantity |
|—————-|———————|—————-|
| Electronics | Smartphone | 5,230 |
| Clothing | Jeans | 4,870 |
| Home Appliances| Microwave | 3,890 |
| Beauty | Lipstick | 3,150 |
| Sports | Tennis Racket | 2,470 |
Monthly Revenue Comparison
This table compares the monthly sales revenue across three consecutive years. It highlights any significant variations in sales performance from month to month.
| Month | 2019 Revenue (USD) | 2020 Revenue (USD)| 2021 Revenue (USD) |
|——-|——————-|——————-|——————-|
| Jan | $167,000 | $192,000 | $240,000 |
| Feb | $179,000 | $205,000 | $225,000 |
| Mar | $203,000 | $215,000 | $288,000 |
| Apr | $187,000 | $220,000 | $245,000 |
| May | $195,000 | $232,000 | $265,000 |
Customer Satisfaction Ratings by Region
This table presents the customer satisfaction rating for each region. The data was collected through feedback surveys, which customers were asked to complete after their purchase.
| Region | Satisfaction Rating (out of 5) |
|—————-|——————————|
| North America | 4.8 |
| Europe | 4.6 |
| Asia | 4.4 |
| South America | 4.3 |
| Africa | 4.7 |
Product Returns by Category
Here, we showcase the percentage of product returns within each category. It aids in identifying categories with higher return rates, enabling necessary measures to improve customer satisfaction.
| Category | Product Return Rate |
|—————-|———————|
| Electronics | 2.1% |
| Clothing | 5.3% |
| Home Appliances| 3.8% |
| Beauty | 1.9% |
| Sports | 2.7% |
Best Selling Brands
This table displays the best-selling brands based on their total sales revenue. It provides an insight into which brands resonate most with customers and contribute significantly to overall sales.
| Brand | Total Sales Revenue (USD) |
|———————–|—————————-|
| Nike | $650,000 |
| Apple | $620,000 |
| Samsung | $590,000 |
| Louis Vuitton | $540,000 |
| L’OrĂ©al Paris | $500,000 |
Customer Age Demographics
This table showcases the distribution of customers by age range, depicting the overall customer demographics. It gives an overview of the age groups that contribute most to the sales.
| Age Range | Percentage of Customers (%) |
|——————-|——————————|
| 20-30 | 32.5 |
| 31-40 | 26.8 |
| 41-50 | 18.9 |
| 51-60 | 15.2 |
| 61 and above | 6.6 |
Revenue by Sales Channel
This table presents the sales revenue generated through different sales channels. It provides insights into the effectiveness of each channel and helps in determining the allocation of resources.
| Sales Channel | Revenue (USD) |
|——————-|—————|
| Online Store | $3,250,000 |
| Physical Store | $2,150,000 |
| Wholesale | $1,150,000 |
| Resellers | $980,000 |
| Partnerships | $820,000 |
Customer Purchase Frequency
In this table, we illustrate the purchase frequency of customers. It depicts the percentage of customers who made multiple purchases within the analyzed period.
| Number of Purchases | Percentage of Customers (%) |
|———————|——————————|
| 1 | 48.3 |
| 2 | 32.7 |
| 3 | 12.9 |
| 4 | 4.6 |
| 5 or more | 1.5 |
Conclusion
The data analysis of sales records has provided valuable insights into various aspects of our business. It allows us to understand our sales performance across different regions and product categories. By monitoring customer satisfaction, product returns, and demographics, we can identify areas of improvement and tailor our strategies accordingly. Additionally, analyzing revenue by sales channels aids in optimizing resource allocation. Understanding customer purchase frequency helps us devise effective customer retention strategies. By leveraging this data, we can make informed decisions to drive growth and enhance overall business performance.
Frequently Asked Questions
How can I perform data analysis on sales records?
What software or tools are recommended for data analysis of sales records?
There are several popular software and tools available for data analysis of sales records, including Microsoft Excel, Python programming language with libraries like Pandas and Numpy, Tableau, and Power BI. Each tool has its own strengths and capabilities, so it depends on your specific requirements and preferences.
What types of sales data should I analyze?
What are some common types of sales data to analyze?
Some common types of sales data to analyze include sales revenue, number of units sold, profit margins, customer demographics, product performance, seasonal trends, and sales quotas. Analyzing these data points can provide valuable insights for decision-making and improving sales strategies.
How can data analysis help improve sales performance?
In what ways can data analysis contribute to improving sales performance?
Data analysis can help identify sales trends, customer preferences, and buying patterns. By analyzing these insights, businesses can optimize their marketing and sales strategies, identify potential growth opportunities, enhance customer satisfaction, make informed pricing decisions, and streamline operations. Ultimately, data analysis enables organizations to make data-driven decisions for better sales performance.
What techniques can be used for sales data analysis?
What are some commonly used techniques for analyzing sales data?
Some commonly used techniques for analyzing sales data include trend analysis, customer segmentation, cohort analysis, regression analysis, correlation analysis, forecasting, and data visualization. These techniques help extract meaningful insights from sales data and facilitate decision-making processes.
What benefits can I expect from analyzing sales records?
What are the potential benefits of analyzing sales records?
Analyzing sales records can bring several benefits, including improved sales forecasting accuracy, identification of underperforming products or regions, increased customer satisfaction through targeted marketing campaigns, better understanding of customer behavior, cost reduction by identifying inefficiencies, and overall improvement in sales performance and profitability.
How often should I analyze sales records?
How frequently should I perform sales data analysis?
The frequency of sales data analysis depends on various factors, such as the size of your business, the amount of sales data generated, and the speed at which market dynamics change. Ideally, it is recommended to perform periodic analysis, such as monthly or quarterly, to monitor trends and make timely adjustments to sales strategies. However, more frequent analysis may be necessary for certain businesses or during specific periods of high activity or significant changes.
What are the key challenges in analyzing sales records?
What are some common challenges faced when analyzing sales records?
Some common challenges in analyzing sales records include data quality issues, incomplete or missing data, data integration from multiple sources, data privacy and security concerns, selecting appropriate analysis techniques, interpreting complex data patterns, and ensuring data accuracy in a rapidly changing business environment. Overcoming these challenges requires data governance, skilled analytics professionals, and robust data analysis processes.
How can I ensure data accuracy in sales analysis?
What measures can I take to ensure data accuracy in sales analysis?
To ensure data accuracy in sales analysis, you can implement data validation techniques, perform regular data cleansing and standardization, establish data quality benchmarks, automate data capturing processes, verify data integrity through cross-referencing, and document data sources and transformations. It is also important to have proper data governance policies in place to maintain the accuracy and reliability of the sales data being analyzed.
What are some examples of actionable insights from sales data analysis?
Can you provide examples of actionable insights that can be derived from sales data analysis?
Examples of actionable insights from sales data analysis include identifying product bundling opportunities, understanding the impact of promotional campaigns on sales, optimizing pricing strategies based on customer segments, identifying cross-selling or upselling opportunities, adjusting inventory levels to meet demand, predicting customer churn, and targeting high-value customers with personalized offers.