**Intro**
Data analysis is a crucial process in extracting valuable insights from large sets of raw data. It involves organizing, cleansing, modeling, and interpreting data to uncover patterns, trends, and correlations. However, data analysis can be an overwhelming task, especially for those new to the field. This article will explore different synonyms for data analysis, providing readers with alternative terms they can use to expand their understanding and vocabulary in the realm of data analysis.
**Key Takeaways**
– Synonyms for data analysis can offer a broader understanding of the field.
– Understanding various terms can facilitate effective communication and collaboration within data analysis teams.
– Different terms for data analysis exist across different industries and sectors.
– Expanding one’s data analysis vocabulary can lead to new opportunities and perspectives.
– Familiarity with data analysis synonyms enhances proficiency in related disciplines like data science and business intelligence.
**Exploring Synonyms:**
1. **Data Analytics**: Often used interchangeably with data analysis, data analytics focuses on analyzing data to drive insights, learnings, and decision-making.
2. **Data Mining**: This term refers to the process of identifying patterns or relationships in large datasets using techniques like clustering or association rules.
3. **Data Interpretation**: The act of interpreting data to understand its meaning, uncover trends, and draw conclusions for various purposes.
4. **Statistical Analysis**: Applying statistical methods to analyze and interpret data in order to inform quantifiable conclusions.
5. **Business Intelligence**: A broader term referring to the methods and technologies used to collect and analyze data to support business decision-making.
*Interesting Sentence: Despite slight differences in emphasis, all of these terms are integral to the process of data analysis and can be used synonymously in many contexts.*
**Synergies Between Data Analysis and Related Disciplines**
Data analysis shares certain similarities with related fields, complementing and drawing from each other’s methodologies and techniques. Here are some notable synergies:
1. **Data Science**: A multi-disciplinary field that combines statistics, machine learning, and related methods to extract knowledge and insights from data.
2. **Machine Learning**: A subset of artificial intelligence that enables computers to learn from data and improve performance without explicit programming.
3. **Predictive Analytics**: Using historical data and statistical modeling to predict future trends or outcomes.
4. **Data Visualization**: The graphical representation of data to facilitate understanding and communicate insights effectively.
(Table 1: Synergies Between Data Analysis and Related Disciplines)
| Field | Shared Techniques |
|—————-|————————————-|
| Data Science | Statistical analysis, machine learning, data mining |
| Machine Learning | Statistical analysis, data mining |
| Predictive Analytics | Statistical analysis, data mining, machine learning |
| Data Visualization | Data interpretation, graphical representation |
*Interesting Sentence: The overlap between these fields opens up opportunities for professionals to acquire a broader skill set and collaborate across disciplines.*
**Industry-Specific Terms**
Different industries may have their own unique terms for data analysis processes. Here are a few examples:
1. **Marketing Analytics**: The study of marketing data to assess and optimize marketing campaigns, customer behavior, and the performance of marketing initiatives.
2. **Financial Modeling**: Applying statistical analysis to financial data to create mathematical representations of financial systems for decision-making and forecasting.
3. **Operations Research**: The application of advanced analytical and mathematical methods to optimize complex operational processes and decision-making.
(Table 2: Terms for Data Analysis in Different Industries)
| Industry | Data Analysis Synonyms |
|————————–|——————————————|
| Marketing | Marketing analytics, customer analytics, campaign analysis |
| Finance | Financial modeling, financial analysis |
| Operations | Operations research, process optimization, decision analysis |
*Interesting Sentence: By understanding domain-specific terms, data analysts can tailor their approach to fit specific industries and collaborate more effectively with experts in those fields.*
**Enhancing Data Analysis Skills**
Continuous learning and skill enhancement are crucial for staying competitive as a data analyst. Here are some suggested approaches:
1. **Stay up-to-date with industry trends**: Subscribing to industry publications, attending webinars, and networking with peers can keep one informed about the latest advancements and best practices in data analysis.
2. **Master new tools and technologies**: Expanding proficiency in popular data analysis tools like Python, R, SQL, and Tableau can enhance the efficiency and accuracy of data analysis processes.
3. **Apply data analysis to real-world problems**: Undertaking side projects or participating in competitions can provide valuable hands-on experience and help sharpen analytical skills.
*Interesting Sentence: A continually evolving field, data analysis requires professionals to remain curious, proactive, and adaptable in order to stay ahead.*
**Conclusion**
Expanding one’s knowledge and vocabulary in the field of data analysis is essential for effective communication, collaboration, and personal growth. By understanding the various synonyms for data analysis and exploring related disciplines, professionals can strengthen their expertise and uncover new opportunities in this ever-evolving field. So, whether you refer to it as data analytics, data mining, or any of the other terms, the core objective remains the same: to extract meaningful insights from data that drive informed decision-making and add value to organizations.

Common Misconceptions
Misconception 1: Data analysis is only for big companies
One common misconception about data analysis is that it is only applicable to large corporations that have massive amounts of data. However, data analysis can be beneficial for businesses of all sizes.
- Even small businesses can use data analysis to identify customer preferences and make informed decisions.
- Data analysis can help startups optimize their marketing strategies and improve their chances of success.
- Data analysis provides useful insights that can be applied by any business striving for growth.
Misconception 2: Data analysis requires advanced technical skills
Many people believe that data analysis is a complex task that requires advanced technical skills, such as programming and statistical knowledge. While having these skills can certainly be helpful, they are not always necessary.
- There are various user-friendly data analysis tools available that require minimal technical expertise.
- Data analysis can often be conducted using simple spreadsheet software, such as Microsoft Excel.
- With the right training and resources, individuals can develop basic data analysis skills without being experts in programming or statistics.
Misconception 3: Data analysis is time-consuming and expensive
Another misconception about data analysis is that it is a time-consuming and expensive process. While data analysis can certainly require time and resources, it can also be done efficiently and cost-effectively.
- Automated data analysis tools and software can significantly speed up the analysis process.
- Data analysis can help businesses save money by identifying inefficiencies and areas of improvement.
- Investing in data analysis can lead to long-term cost-savings and improved decision-making.
Misconception 4: Data analysis always provides definitive answers
Some individuals mistakenly believe that data analysis always provides definite answers or solutions. While data analysis can provide valuable insights, it is important to remember that it is not a magic bullet.
- Data analysis helps to uncover patterns and trends, but interpretation is still necessary.
- Data analysis can offer multiple insights or possibilities, requiring decision-makers to weigh options.
- Data analysis should be used as a tool for informed decision-making, rather than the sole source of definitive answers.
Misconception 5: Data analysis is only used for historical data
Lastly, it is a common misconception that data analysis is only used for historical data. While historical data is indeed valuable for analysis, data analysis can also be applied to real-time and predictive scenarios.
- Data analysis can help businesses detect early signs of emerging trends or potential issues.
- Real-time data analysis enables quick decision-making and proactive strategies.
- Predictive analysis uses past and current data to forecast future outcomes and aid in strategic planning.
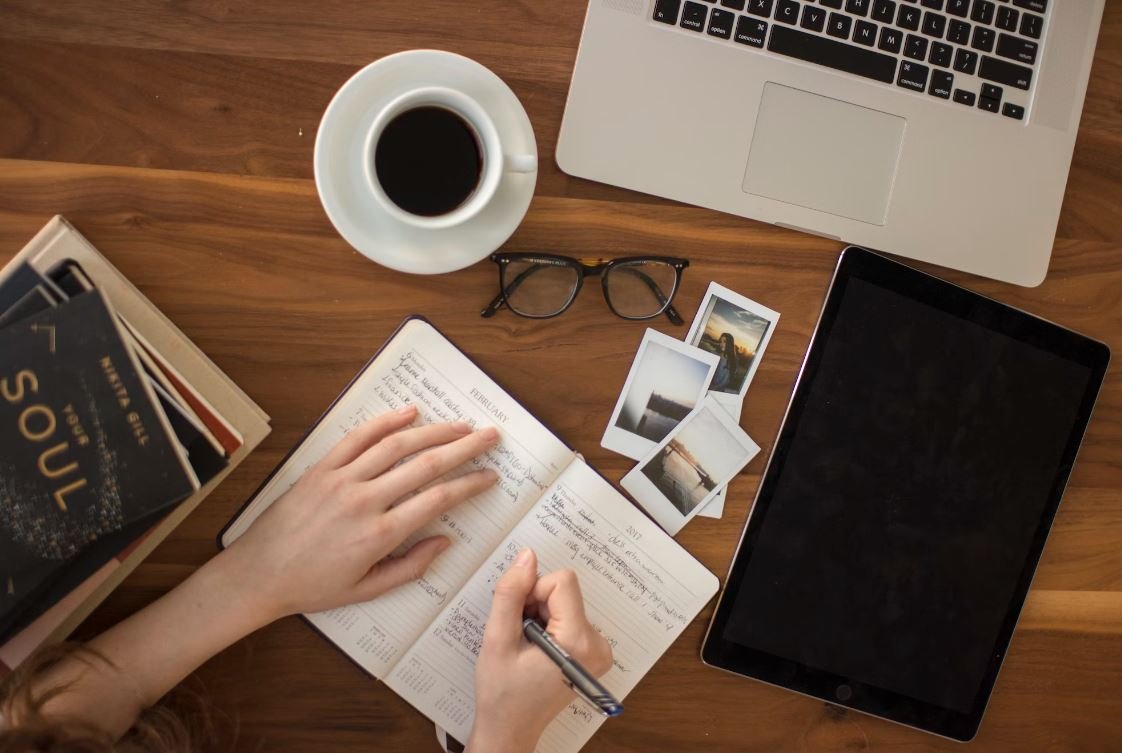
Data Analysis Synonym: Data Synonyms
In this table, we explore a variety of synonyms for the term “data analysis.” Each synonym offers a unique perspective on the process of analyzing data, highlighting the diverse techniques and approaches used in this field.
Synonym | Definition |
---|---|
Data Mining | The practice of examining large databases to generate new information or patterns. |
Data Exploration | The initial phase of analyzing data to discover its main characteristics and patterns. |
Data Interpretation | The process of examining data to extract meaning and draw conclusions. |
Data Analytics | The systematic exploration of data to uncover insights and predict future trends. |
Data Visualization | The representation of data using visual elements such as charts, graphs, or maps. |
Data Analysis Synonym: Statistical Techniques
In this table, we present various statistical techniques commonly employed in data analysis. These techniques help researchers draw meaningful inferences and make informed decisions based on the collected data.
Technique | Description |
---|---|
Regression Analysis | A statistical method used to model relationships between variables and make predictions. |
Hypothesis Testing | A statistical approach to assess the significance of an observed difference or relationship. |
Cluster Analysis | A technique to classify data into groups or clusters based on similarities or differences. |
ANOVA (Analysis of Variance) | A statistical test to compare means of three or more groups to determine if they are significantly different. |
Time Series Analysis | A method for analyzing data points collected over time to identify patterns or trends. |
Data Analysis Synonym: Tools and Software
In this table, we explore different tools and software commonly utilized in data analysis. These tools assist researchers in managing and analyzing large datasets efficiently.
Tool/Software | Description |
---|---|
R | A programming language and software environment for statistical computing and graphics. |
Python | A versatile programming language widely used for data analysis and scientific computing. |
Tableau | A data visualization tool allowing users to explore and present data in interactive dashboards. |
Excel | A spreadsheet software that enables data organization, manipulation, and basic analysis. |
SPSS | A software package used for statistical analysis and data management. |
Data Analysis Synonym: Steps in the Process
In this table, we outline the key steps involved in the data analysis process. Each step contributes to the overall understanding and insights gained from the data.
Step | Description |
---|---|
Data Collection | Gathering relevant data from various sources, such as surveys, experiments, or databases. |
Data Cleaning | Reviewing and correcting the collected data to ensure accuracy, completeness, and consistency. |
Data Transformation | Converting data into a suitable format for analysis, often involving aggregating or reshaping it. |
Data Analysis | Performing statistical or other analytical techniques to uncover patterns and relationships within the data. |
Data Interpretation | Examining the analysis results to draw meaningful conclusions and make informed decisions. |
Data Analysis Synonym: Types of Data
This table highlights various types of data that can be analyzed, providing insights into the diverse nature of information used in data analysis.
Data Type | Description |
---|---|
Numerical Data | Data represented by numbers, allowing for various mathematical operations and analysis. |
Categorical Data | Data that can be grouped into categories or labels, often analyzed using frequency distributions or cross-tabulations. |
Time Series Data | Data collected at regular intervals over time, enabling analysis of trends and patterns. |
Textual Data | Data in the form of text or documents, often analyzed using natural language processing techniques. |
Geospatial Data | Data associated with geographic locations, enabling spatial analysis and visualization. |
Data Analysis Synonym: Ethical Considerations
In this table, we address the ethical considerations associated with data analysis, emphasizing the importance of responsible and ethical practices in this field.
Ethical Consideration | Description |
---|---|
Data Privacy | The protection of personal or sensitive information from unauthorized access or disclosure. |
Informed Consent | The ethical requirement of obtaining voluntary and knowledgeable consent from participants before collecting or analyzing their data. |
Data Anonymization | The process of removing or altering identifying information in data to ensure individual privacy and confidentiality. |
Data Ownership | The consideration of rightful ownership and control over data, ensuring transparency and accountability. |
Data Security | The safeguarding of data against unauthorized access, loss, or alteration. |
Data Analysis Synonym: Popular Data Sources
This table presents a range of popular data sources commonly used for analysis. These sources provide the foundation for conducting various research studies and projects.
Data Source | Description |
---|---|
Census Data | Official population and demographic data collected by governments. |
Surveys | Specially designed questionnaires or interviews used to gather data directly from individuals or groups. |
Sales Data | Data related to the sales of products or services, often utilized in market analysis. |
Social Media Data | Data collected from various social media platforms, enabling analysis of user behavior and sentiment. |
Research Studies | Data generated from academic or scientific studies, contributing to broader research and knowledge. |
Data Analysis Synonym: Key Challenges
In this table, we outline several key challenges that researchers and analysts often face during the data analysis process. Recognizing these challenges is crucial for developing effective strategies and solutions.
Challenge | Description |
---|---|
Data Quality | The reliability, accuracy, and completeness of collected data, which can affect the validity of analysis results. |
Data Bias | The presence of systematic errors or prejudices in data collection, leading to skewed or unfair analysis outcomes. |
Data Volume | The sheer size and complexity of big data, requiring advanced tools and techniques for analysis and interpretation. |
Data Integration | The process of combining and harmonizing data from multiple sources, addressing format, structure, and compatibility issues. |
Data Security | The protection of data throughout its lifecycle, ensuring confidentiality and preventing unauthorized access. |
Data analysis is a multifaceted discipline that encompasses various synonyms, statistical techniques, and tools. This article aimed to provide a comprehensive overview of the different aspects involved in data analysis. From exploring synonyms and types of data to addressing ethical considerations and common challenges, data analysis plays a crucial role in extracting knowledge and insights from vast amounts of information. By navigating these complexities and leveraging the appropriate techniques and tools, analysts can unlock hidden patterns, make informed decisions, and contribute to advancements in diverse fields.
Frequently Asked Questions
What is data analysis?
Data analysis is the process of inspecting, cleaning, transforming, and modeling data to uncover useful information, draw conclusions, and support decision-making. It involves various techniques and methodologies to understand patterns, trends, and correlations within datasets.
What are the different types of data analysis?
There are several types of data analysis techniques, including exploratory data analysis, descriptive statistics, inferential statistics, predictive analytics, and prescriptive analytics. Each of these approaches serves different purposes and leverages various statistical and mathematical methods.
Why is data analysis important?
Data analysis is crucial because it enables organizations and individuals to make informed decisions based on facts and evidence. It helps identify trends, patterns, and relationships within data that can lead to improved efficiency, problem-solving, and strategic planning.
What skills are required for data analysis?
Data analysis requires proficiency in statistical analysis, critical thinking, data manipulation, programming languages like Python or R, data visualization, and domain knowledge. Strong communication skills and the ability to interpret and present findings are also essential.
How does data analysis benefit businesses?
Data analysis provides businesses with valuable insights into customer behavior, market trends, and business performance. It helps optimize operations, identify areas for improvement, target marketing efforts, make data-driven decisions, and gain a competitive edge in the market.
What tools are commonly used for data analysis?
There are various tools available for data analysis, including statistical software like R and Python’s libraries (such as Pandas and NumPy), spreadsheet programs like Microsoft Excel, data visualization tools like Tableau, and programming environments like Jupyter Notebooks.
What is the difference between qualitative and quantitative data analysis?
Qualitative data analysis involves examining non-numerical data, such as text, images, or videos, to identify themes, patterns, and insights. On the other hand, quantitative data analysis focuses on numerical data, applying statistical techniques to analyze and interpret the data.
What is the data analysis process?
The data analysis process typically involves several stages: 1) Formulating a research question or objective, 2) Data collection, 3) Data cleaning and preparation, 4) Exploratory data analysis, 5) Applying statistical techniques, 6) Drawing conclusions and making inferences, and 7) Presenting findings in a clear and understandable manner.
How can data analysis be applied in different industries?
Data analysis can be applied across various industries. For example, in healthcare, it can be used to analyze patient data and identify effective treatments. In finance, it can help with risk assessment and investment strategies. In marketing, it aids in understanding customer behavior and targeting advertising campaigns.
What ethical considerations are important in data analysis?
Ethical considerations in data analysis include ensuring data privacy and security, obtaining proper consent for data use, avoiding bias in data collection and analysis, maintaining transparency in data handling, and using data in ways that align with legal and ethical standards.