Data Analysis Terms
Data analysis is an integral part of any business strategy. Whether you are analyzing customer behavior, market trends, or financial performance, understanding the key terms and concepts related to data analysis is crucial for making informed decisions. This article explores some commonly used data analysis terms and their significance in the business world.
Key Takeaways
- Understanding data analysis terms is essential for making informed business decisions.
- These terms relate to various aspects of data processing and analysis.
- Being familiar with these terms enhances communication and collaboration within interdisciplinary teams.
- Data analysis terms help uncover patterns, trends, and insights from raw data.
1. Big Data
Big data refers to extremely large and complex datasets that cannot be easily processed using traditional methods. It involves the analysis of high volumes, velocity, and variety of data to extract meaningful insights. *The exponential growth of digital information has contributed to the rise of big data analytics.*
2. Data Mining
Data mining is the process of discovering patterns, relationships, or anomalies within a dataset. It involves using various statistical techniques and machine learning algorithms to extract valuable information from raw data. *Data mining helps businesses uncover hidden patterns and predict future trends.*
3. Descriptive Analysis
Descriptive analysis focuses on summarizing and interpreting historical data to gain insights into past events or trends. It involves organizing and presenting data in a meaningful way, such as through charts, graphs, or tables. *Descriptive analysis helps businesses understand past performance and identify areas for improvement.*
4. Predictive Analytics
Predictive analytics involves using historical data and statistical models to make informed predictions about future outcomes or events. It uses techniques such as regression analysis, time series forecasting, and machine learning algorithms to generate forecasts and identify potential opportunities or risks. *Predictive analytics empowers businesses to anticipate customer behavior and make proactive decisions.*
5. Correlation
Correlation measures the relationship between two or more variables. It indicates how changes in one variable are associated with changes in another variable. Correlation can be positive, indicating a direct relationship, or negative, indicating an inverse relationship. *Correlation helps businesses understand the interdependencies between different factors and evaluate cause-and-effect relationships.*
6. Statistical Significance
Statistical significance determines whether the results observed in a sample are representative of the entire population or occurred by chance. It involves conducting hypothesis tests and calculating p-values to assess the reliability and validity of research findings. *Understanding statistical significance helps businesses make confident decisions based on reliable data analysis.*
Data Analysis Tables
Term | Definition |
---|---|
Data Visualization | The representation of data in visual formats, such as charts, graphs, or maps, to facilitate understanding and interpretation. |
Hypothesis Testing | A statistical method for testing the validity of a claim or hypothesis about a population based on sample data. |
Method | Description |
---|---|
Regression Analysis | A statistical technique used to model the relationship between a dependent variable and one or more independent variables. |
Cluster Analysis | A technique for grouping similar objects or individuals based on their characteristics or attributes. |
Model | Application |
---|---|
Decision Tree | In business, decision trees are used to analyze potential outcomes and make optimal decisions based on different scenarios. |
Time Series | In finance, time series models are employed to predict stock prices or market trends based on historical data. |
7. Data Visualization
Data visualization is the representation of data in visual formats, such as charts, graphs, or maps, to facilitate understanding and interpretation. It helps in identifying patterns, trends, and outliers in the data. *Effective data visualization enables businesses to communicate complex information more clearly and engage stakeholders.*
8. Hypothesis Testing
Hypothesis testing is a statistical method for testing the validity of a claim or hypothesis about a population based on sample data. It involves defining a null hypothesis and an alternative hypothesis, collecting data, and calculating p-values to determine the significance of the results. *Hypothesis testing allows businesses to make evidence-based decisions and draw reliable conclusions.*
9. Regression Analysis
Regression analysis is a statistical technique used to model the relationship between a dependent variable and one or more independent variables. It helps businesses understand the impact of different factors on the outcome of interest and make predictions based on the observed patterns. *Regression analysis enables businesses to identify key drivers and optimize decision-making processes.*
10. Cluster Analysis
Cluster analysis is a technique for grouping similar objects or individuals based on their characteristics or attributes. It helps identify inherent patterns or segments within the data and categorize them into meaningful clusters. *Cluster analysis assists businesses in segmentation, targeting, and personalization strategies.*
11. Decision Tree
Decision trees are a graphical representation of decision-making models. They map out potential outcomes and the associated probabilities or consequences at each decision point. Decision trees are widely used in business to analyze scenarios, identify optimal paths, and quantify risks. *Decision trees simplify complex decision-making processes and support intelligent choices.*
12. Time Series Analysis
Time series analysis involves analyzing data collected over time to identify patterns and predict future values. It is commonly used in finance, economics, and forecasting industries to predict trends, estimate future values, and assess variability. *Time series analysis equips businesses with insights to make informed predictions and optimize resource allocation.*
As businesses continue to evolve, data analysis becomes increasingly important in gaining a competitive edge. By understanding these key terms and applying the appropriate techniques, businesses can extract valuable insights from data and make informed decisions.

Common Misconceptions
Misconception 1: Data analysis is only useful for large companies
– Small businesses can also benefit from data analysis by gaining insights into customer behavior and preferences.
– Data analysis can help small businesses make informed decisions about product offerings and marketing strategies.
– Implementing data analysis tools can help small businesses stay competitive in their respective markets.
Misconception 2: Data analysis is all about numbers and statistics
– Data analysis also involves data visualization techniques, such as creating charts and graphs, to effectively present the findings.
– Data analysis is not solely focused on complicated mathematical equations, but also includes interpreting patterns and trends in the data.
– Data analysis requires critical thinking and problem-solving skills to draw meaningful insights from the data.
Misconception 3: Data analysis is only for professionals with a background in statistics
– While a background in statistics can be helpful, anyone with basic analytical skills can learn and apply data analysis techniques.
– There are readily available tools and software that simplify the data analysis process, making it accessible to non-experts.
– Learning data analysis can be beneficial for individuals in various fields, such as marketing, finance, and healthcare.
Misconception 4: Data analysis always leads to accurate predictions
– Data analysis provides insights based on historical data, but it doesn’t guarantee accurate predictions for the future.
– External factors and unforeseen events can influence the accuracy of predictions derived from data analysis.
– Data analysis should be used as a tool to inform decision-making rather than relying solely on the outcomes as definitive predictions.
Misconception 5: Data analysis is time-consuming and expensive
– Advanced data analysis techniques can be time-consuming, but simpler analyses can be done quickly with available tools.
– Many free or low-cost data analysis tools are available for individuals and businesses to use without the need for extensive financial investment.
– The benefits gained from data analysis, such as improved decision-making and increased efficiency, can outweigh the initial time and cost investment.
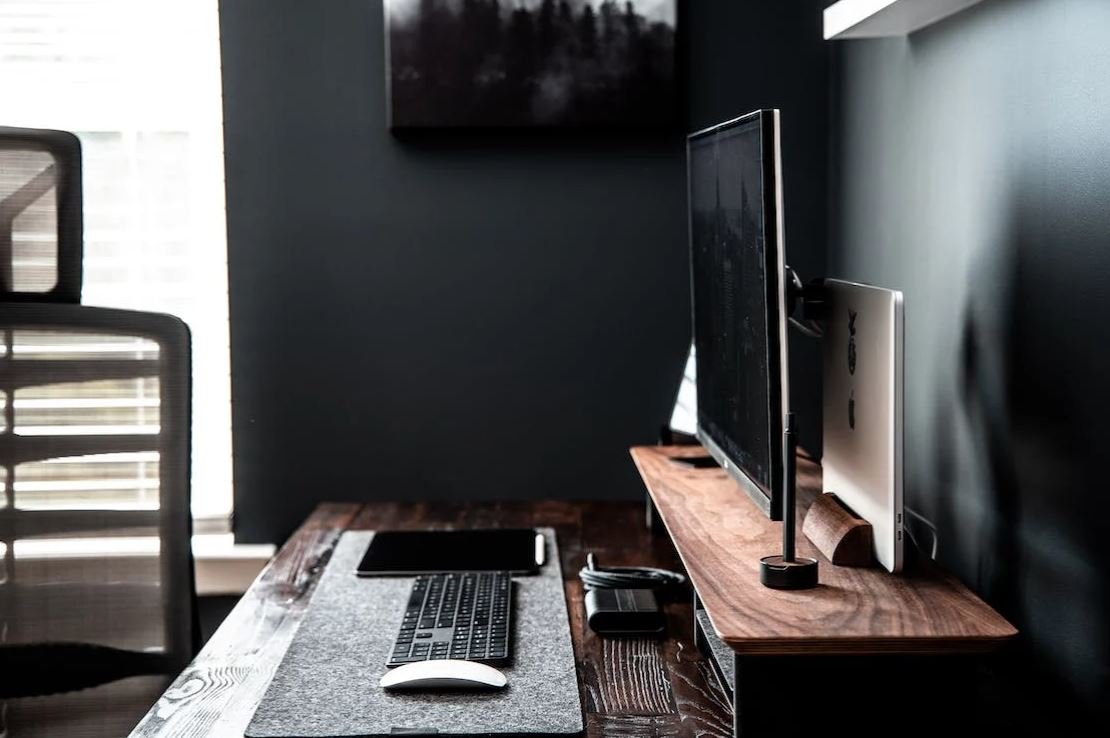
Data Analysis Terms
Data analysis is the process of inspecting, cleaning, transforming, and modeling data to discover useful information, draw conclusions, and support decision-making. Here are ten interesting tables that illustrate various points, data, and elements related to data analysis terms.
Data Types
This table showcases different types of data commonly encountered in data analysis:
| Data Type | Description |
|————|——————————|
| Numeric | Numbers, such as age or cost |
| Categorical| Categories, like colors or genres|
| Text | Alphanumeric characters, like text messages |
| Boolean | Binary values, true or false |
| Date/Time | Dates and times |
| Ordinal | Ranked values, like survey responses |
Distribution Types
Understanding the distribution of data is crucial in data analysis. This table presents various distribution types:
| Distribution Type | Description |
|——————-|———————————————————|
| Normal | Bell-shaped and symmetrical distribution |
| Skewed | Asymmetric distribution, either positively or negatively skewed |
| Bimodal | Two distinct peaks or modes |
| Uniform | Equal probability across the range of values |
| Exponential | Rapid drop-off after an initial peak |
| Log-normal | Skewed distribution of the logarithm of the variable |
Sampling Methods
Sampling techniques are employed when analyzing a subset of a larger population. The following table demonstrates different sampling methods:
| Sampling Method | Description |
|——————–|———————————————————-|
| Simple Random | Each member of the population has an equal chance of being selected |
| Stratified | The population is divided into strata, and a proportional sample is taken from each stratum |
| Cluster | The population is divided into clusters, and a sample of clusters is selected |
| Systematic | Elements are selected at regular intervals from an ordered list |
| Convenience | Choosing subjects based on their easy availability |
| Snowball | Participants refer others who fit the criteria |
Hypothesis Testing Steps
Hypothesis testing aids in making data-driven decisions. The table below outlines the steps involved in hypothesis testing:
| Step | Description |
|——————————————–|——————————————————————|
| Define the null hypothesis | Stating the default position or no effect |
| Set the significance level (alpha) | Deciding on the threshold for accepting or rejecting the hypothesis |
| Collect and analyze the data | Gathering data and performing statistical analysis |
| Calculate the test statistic | The statistic used to evaluate how closely the data adheres to the null hypothesis |
| Compare the test statistic with critical values | Determining if the test statistic falls within the critical region |
| Make a decision and interpret the results | Accepting or rejecting the null hypothesis based on the test statistic |
Data Visualization Types
Visualizing data is essential for effective communication. In this table, we explore different data visualization types:
| Visualization Type | Description |
|———————|————————————————————|
| Bar Chart | Comparative display using horizontal or vertical bars |
| Pie Chart | Circular representation divided into wedges |
| Line Chart | Displaying trends over time using connected data points |
| Scatter Plot | Mapping data points on a two-dimensional plane |
| Heatmap | Color-coded matrix displaying data density patterns |
| Histogram | Visualizing the distribution of numerical data |
Statistical Measures
To gain insights from data, we often compute statistical measures. This table highlights some important statistical measures:
| Statistic | Description |
|——————|————————————————————|
| Mean | Average value of a set of numbers |
| Median | Middle value in a set of numbers |
| Mode | Most frequently occurring value in a dataset |
| Standard Deviation | Measure of dispersion indicating the spread of data values |
| Correlation | Measure of the linear relationship between two variables |
| Regression | Examining the relationship between dependent and independent variables |
Data Cleaning Techniques
Data cleaning is essential for ensuring accuracy and reliability. The subsequent table presents different data cleaning techniques:
| Technique | Description |
|——————————–|—————————————————————————-|
| Removing duplicates | Eliminating identical records or entries in a dataset |
| Handling missing values | Dealing with null or incomplete data by imputation or deletion |
| Standardizing data | Scaling data to a common range or unit for consistency during analysis |
| Handling outliers | Treating extreme values that deviate significantly from the rest of the data |
| Correcting inconsistent data | Resolving discrepancies or errors in the data that impede analysis |
| Encoding categorical variables | Converting categorical data into numerical form for analysis |
Machine Learning Algorithms
Machine learning algorithms enable automated pattern recognition. This table presents various algorithms used in data analysis:
| Algorithm | Description |
|—————————-|—————————————————|
| Linear Regression | Predicting continuous output based on input features |
| Decision Trees | Hierarchical structure to make decisions |
| Random Forests | Ensemble of decision trees for improved accuracy |
| Support Vector Machines | Classifying data into distinct categories |
| K-Nearest Neighbors | Classifying data based on similarity to neighbors |
| Naive Bayes | Probabilistic classifier based on Bayes’ theorem |
Data Analysis Tools
To facilitate data analysis, various tools and software are available. The following table highlights some popular data analysis tools:
| Tool | Description |
|———————|———————————————————-|
| R | Open-source language and environment for statistical computing and graphics |
| Python | Versatile programming language with rich data analysis libraries and packages |
| SQL | Standard language for storing, manipulating, and retrieving structured data |
| Tableau | Data visualization software facilitating interactive dashboards and reports |
| Excel | Widely used spreadsheet application for data manipulation and analysis |
| SAS | Software suite for advanced analytics and business intelligence |
Data analysis encompasses a vast array of terms, concepts, and techniques. Understanding these aspects is crucial for effectively deriving insights from data. Through this article, we explored various data analysis terms, including data types, hypothesis testing steps, visualization types, statistical measures, cleaning techniques, machine learning algorithms, and tools. Armed with this knowledge, analysts can navigate the landscape of data analysis and make informed decisions based on reliable and meaningful information.
Data Analysis Terms
Frequently Asked Questions
What is data analysis?
What are some common data analysis terms?
What is the difference between qualitative and quantitative data analysis?
What is exploratory data analysis?
What is data visualization?
What is a statistical model?
What is data mining?
What is statistical significance?
What is machine learning?
What is hypothesis testing?