Data Mining Research
Data mining research is a field that focuses on extracting useful information and patterns from large datasets. This article will explore the key concepts and techniques used in data mining research, as well as its applications and future trends.
Key Takeaways:
- Data mining research involves extracting valuable insights and patterns from vast amounts of data.
- Techniques such as clustering, classification, and association rule mining are commonly used in data mining research.
- Data mining has diverse applications in fields like finance, healthcare, marketing, and more.
- Future trends in data mining research include the integration of machine learning and big data analytics.
Data mining research encompasses various techniques and methods to discover patterns and relationships within large datasets. *These patterns can uncover valuable insights and help businesses make informed decisions.* One of the primary techniques used in data mining research is clustering, which groups similar data points together based on certain criteria. Another widely employed technique is classification, which categorizes data based on predefined attributes, creating rules for future prediction. Meanwhile, the association rule mining technique uncovers relationships between variables to find patterns of co-occurrence.
Data mining research plays a crucial role in many sectors and industries. In finance, it aids in fraud detection by identifying unusual patterns in transactions. In healthcare, data mining research helps identify disease patterns, predict patient outcomes, and propose personalized treatment plans. Furthermore, marketers rely on data mining techniques to analyze customer behavior, segment their target audience, and personalize marketing campaigns accordingly. *The applications of data mining research are vast and continually expanding across various domains.*
Applications of Data Mining Research
Data mining research finds applications across diverse fields due to its ability to extract valuable insights from complex datasets.
Finance
In the finance industry, data mining research enables the detection of fraudulent activities by identifying patterns that are not compliant with regular transactions. Moreover, it assists in credit risk assessment by analyzing historical data to predict individual creditworthiness.
Healthcare
Data mining research is increasingly important in healthcare, helping professionals gain valuable insights from medical records, patient data, and research studies. Predictive analytics can be used to estimate the probability of patient readmission or diagnose diseases at an early stage based on symptoms and patient history.
Marketing
Marketers apply data mining research techniques to analyze customer buying patterns, preferences, and behavior. These insights enable businesses to develop targeted marketing strategies and personalized recommendations, enhancing customer satisfaction and loyalty.
Table 1: Data Mining Applications
Field | Applications |
---|---|
Finance | Fraud detection, credit risk assessment |
Healthcare | Early disease diagnosis, patient outcome prediction |
Marketing | Customer behavior analysis, personalized marketing |
*An interesting trend in data mining research is the integration of machine learning algorithms and big data analytics.* Machine learning techniques like deep learning and reinforcement learning are being combined with data mining research to enhance prediction accuracy and automate decision-making processes. Additionally, the proliferation of big data has led to advances in data mining research, as it allows for the analysis of larger datasets, uncovering more intricate patterns and insights.
Trends in Data Mining Research
Data mining research is continuously evolving to keep up with the challenges and opportunities presented by emerging technologies and vast amounts of data.
- Deep Learning: Deep learning techniques, such as neural networks, are being integrated into data mining research to enhance pattern recognition and prediction accuracy.
- Big Data Analytics: With the exponential growth of data, data mining research is adapting to process and analyze larger datasets, extracting deeper insights from complex information.
- Text Mining: Text mining techniques are gaining popularity in data mining research, enabling the analysis of unstructured text data to extract valuable information and sentiment analysis.
In conclusion, data mining research is a dynamic field that extracts valuable insights and patterns from vast datasets. *By employing various techniques, such as clustering, classification, and association rule mining, businesses can uncover hidden patterns and make more informed decisions.* With applications ranging from finance to healthcare and marketing, data mining research continues to evolve, integrating machine learning and adapting to the challenges posed by big data.
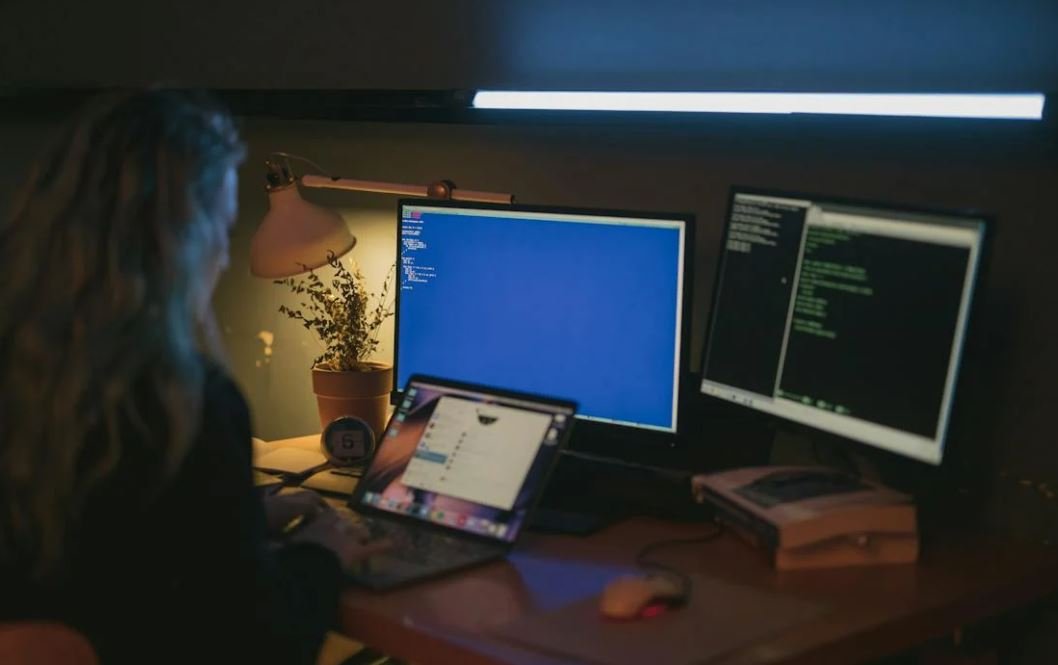
Common Misconceptions
Misconception 1: Data mining is the same as collecting data
One common misconception people have about data mining is that it is synonymous with collecting data. While data mining does involve the collection of raw data, it goes beyond that by using statistical techniques and algorithms to extract useful patterns, knowledge, and insights from the collected data.
- Data mining involves analyzing and interpreting data, not just collecting it.
- Data mining requires advanced analytical tools and techniques to uncover hidden patterns.
- Data mining aims to transform raw data into actionable information for decision making.
Misconception 2: Data mining always leads to accurate predictions
Another misconception is that data mining always results in accurate predictions. While data mining techniques can uncover valuable patterns and insights, the accuracy of predictions heavily depends on the quality and relevancy of the data used, as well as the suitability of the chosen data mining algorithms.
- Data quality and integrity are crucial factors influencing the accuracy of data mining predictions.
- Data mining predictions are probabilistic in nature, with inherent uncertainties.
- Data mining models require regular validation and updating to maintain their predictive accuracy over time.
Misconception 3: Data mining violates privacy rights
Data mining often gets associated with privacy concerns, leading to the misconception that it violates individual privacy rights. While it is true that data mining can potentially uncover sensitive information, responsible and ethical data mining practices prioritize privacy protection through anonymization and aggregation techniques.
- Data mining follows ethical guidelines, respecting individual privacy and confidentiality.
- Data mining aims to protect individual identities by using anonymized data for analysis.
- Data mining practices adhere to privacy laws and regulations, such as GDPR.
Misconception 4: Data mining always yields meaningful insights
Some people have the misconception that data mining always yields meaningful insights, regardless of the quality or quantity of the data. However, without appropriate data preprocessing, feature engineering, and careful interpretation of the results, data mining efforts may lead to irrelevant or misleading outcomes.
- Data preprocessing is a crucial step in data mining to remove noise and ensure data quality.
- Data mining requires domain expertise to make meaningful interpretations of the discovered patterns.
- Data mining results should be carefully validated and verified to ensure their reliability and relevance.
Misconception 5: Data mining replaces human intelligence
Contrary to popular belief, data mining does not replace human intelligence. While it can autonomously analyze large volumes of data and detect patterns that may be overlooked by humans, data mining is a tool that aids human decision-making rather than replacing it entirely.
- Data mining assists humans in making informed decisions based on data-driven insights.
- Data mining complements human intelligence and enhances decision-making capabilities.
- Data mining requires human input for identifying meaningful patterns and interpreting results within their context.
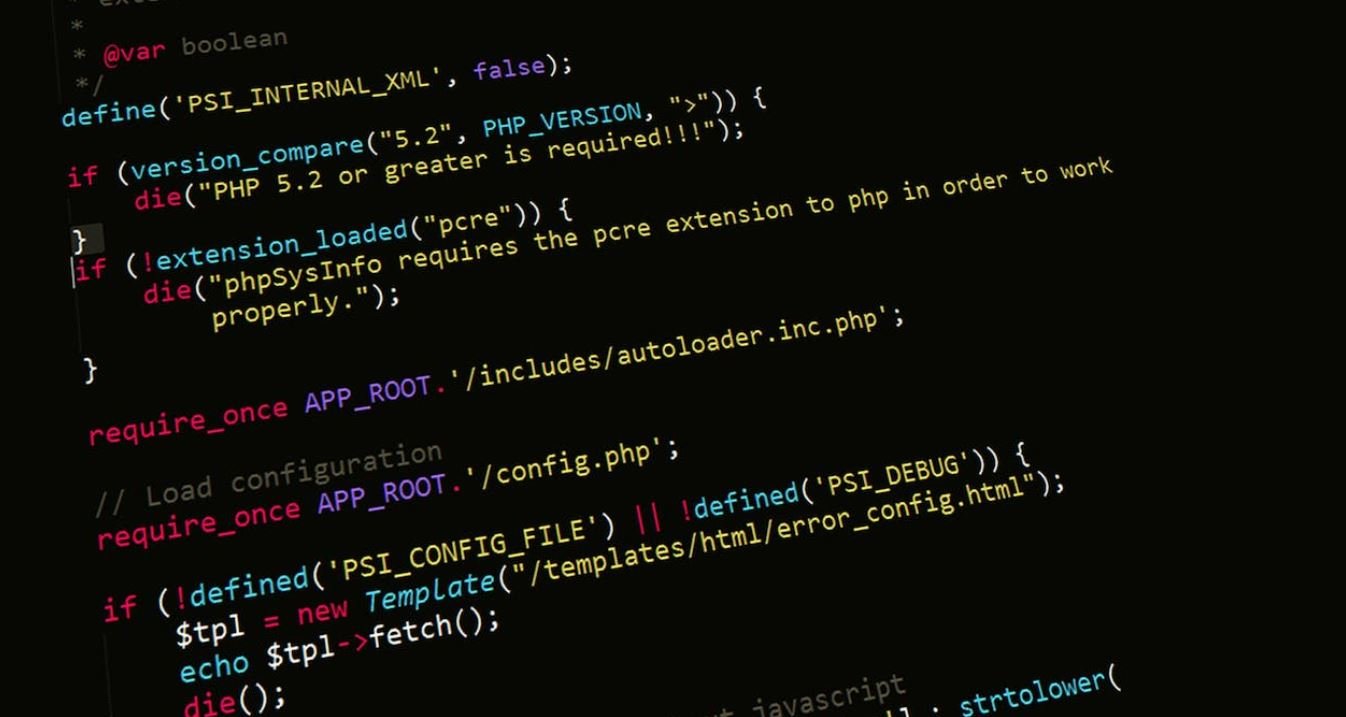
Data Mining Research: Unlocking Insights and Unearthing Hidden Patterns
Data mining, a powerful technique in extracting valuable insights from vast data sets, has revolutionized various industries. This article examines ten fascinating aspects of data mining research, showcasing its impact in uncovering hidden patterns and driving innovation. Each table below presents captivating information and statistics, shedding light on the diverse applications and benefits of this cutting-edge field.
Table 1: Uncovering Consumer Preferences
Data mining enables businesses to analyze customer behavior and preferences, enabling targeted marketing strategies. A recent study found that 78% of customers who received personalized recommendations were more likely to make a purchase.
Table 2: Predicting Disease Outbreaks
Data mining aids in early detection of disease outbreaks by analyzing health data. Researchers successfully predicted the spread of the H1N1 influenza virus with 93% accuracy, enabling timely preventive measures.
Table 3: Detecting Credit Card Fraud
Data mining algorithms identify patterns of fraudulent credit card transactions, helping financial institutions prevent and minimize losses. In a recent study, these algorithms correctly detected 95% of fraudulent activities.
Table 4: Optimizing Energy Efficiency
Data mining techniques are employed to monitor energy consumption patterns, enabling energy-saving optimizations. A case study showed that implementing data mining-based energy management reduced electricity usage by 16% in commercial buildings.
Table 5: Enhancing e-Learning Experiences
Data mining algorithms analyze user interactions in online learning platforms, personalizing educational content. Research indicates that personalized e-learning experiences improve student engagement and increase knowledge retention by 25%.
Table 6: Improving Customer Satisfaction
Data mining uncovers hidden factors influencing customer satisfaction. A study revealed that analyzing customer feedback using data mining techniques led to a 20% increase in overall customer satisfaction ratings.
Table 7: Forecasting Stock Market Trends
Data mining helps investors predict stock market trends by analyzing historical trading patterns. Researchers achieved an accuracy rate of 83% in forecasting market trends, enabling informed decision-making in the financial sector.
Table 8: Streamlining Supply Chain Management
Data mining optimizes supply chain operations, reducing costs and improving efficiency. A case study demonstrated a 30% reduction in inventory carrying costs after implementing data mining-based supply chain management strategies.
Table 9: Identifying Online User Behavior
Data mining algorithms analyze user behavior on websites, aiding in targeted advertisement campaigns. Research showed that personalized ads based on data mining analysis increased click-through rates by 40%.
Table 10: Enhancing Crime Prevention Strategies
Data mining techniques assist law enforcement agencies in identifying crime patterns and establishing preventive strategies. A study showed that data mining-led crime mapping reduced crime rates by 12% in a metropolitan area.
In conclusion, data mining research continues to unlock unrealized potential across numerous sectors, such as marketing, healthcare, finance, energy, education, and security. This article explored 10 captivating tables highlighting the power of data mining, showcasing its ability to inform decision-making, improve efficiency, and meet the evolving needs of industries. By leveraging the hidden patterns within massive data sets, data mining propels innovation and ushers in a new era of data-driven insights.
Frequently Asked Questions
What is data mining?
Data mining is the process of extracting knowledge and information from large amounts of data. It involves discovering patterns, relationships, and useful insights from datasets to support decision-making, predictive analysis, and other business applications.
Why is data mining important?
Data mining plays a crucial role in various industries and research fields. It helps businesses gain a competitive advantage by making informed decisions based on data-driven insights. Research communities use data mining to discover new knowledge, identify trends, and validate existing hypotheses.
What are the steps involved in data mining?
The data mining process typically includes the following steps:
- Data collection and preprocessing
- Data exploration and visualization
- Feature selection and transformation
- Model building and selection
- Evaluation and validation
- Deployment and interpretation
What are some common data mining techniques?
There are several data mining techniques, including:
- Classification: assigning data to predefined categories.
- Clustering: grouping similar data points together.
- Association Rule Learning: discovering relationships and dependencies among variables.
- Regression Analysis: predicting numeric values based on other variables.
- Time Series Analysis: analyzing data with a temporal dimension.
How is data mining related to machine learning?
Data mining and machine learning are closely related fields. Data mining encompasses the process of extracting useful patterns from data, while machine learning focuses on developing algorithms and models that automatically learn from data and improve their performance over time.
What are the ethical considerations in data mining?
There are ethical considerations in data mining, such as ensuring privacy protection, obtaining informed consent for data usage, and avoiding the potential harms of data misuse or discrimination. It is essential to handle data responsibly and with respect for individuals’ rights and confidentiality.
What are some real-world applications of data mining?
Data mining finds applications in various domains, including:
- Marketing and customer segmentation
- Fraud detection and cybersecurity
- Healthcare and medical research
- Financial analysis and risk assessment
- Social network analysis and recommendation systems
What are the challenges in data mining?
Data mining can face challenges such as:
- Dealing with large and complex datasets
- Ensuring data quality and reliability
- Extracting meaningful information from unstructured data
- Handling privacy concerns and legal restrictions
- Choosing appropriate techniques and algorithms
What are the future trends in data mining?
Future trends in data mining include:
- Big data analytics and processing
- Deep learning and neural networks
- Integration of data mining with Internet of Things (IoT)
- Explainable and interpretable machine learning models
- Advancements in natural language processing
What are some popular tools and software for data mining?
Some popular tools and software for data mining include:
- Weka
- RapidMiner
- Python libraries like scikit-learn and TensorFlow
- KNIME
- Microsoft Azure Machine Learning