Data Mining Business Intelligence
Data mining is a crucial component of business intelligence. It involves extracting useful information from large datasets, providing valuable insights that can drive decision-making and improve business performance. By analyzing data patterns, relationships, and trends, organizations can gain a competitive edge and make more informed decisions.
Key Takeaways:
- Data mining is a key aspect of business intelligence.
- It involves extracting valuable insights from large datasets.
- By analyzing data patterns, organizations can make more informed decisions.
Data mining employs various techniques to uncover hidden patterns and relationships within datasets. These techniques include classification, regression, clustering, and association rules. With classification, data is categorized into predefined classes based on certain attributes. Regression analysis helps predict numerical outcomes based on historical data, while clustering groups similar data points together. Lastly, association rule mining discovers relationships among variables in the dataset.
Data mining plays an integral role in diverse industries. In finance, it helps detect fraudulent activities and predict stock market trends. Retailers use it to analyze customer behavior, enhance marketing strategies, and improve customer loyalty. Additionally, healthcare organizations employ it for disease prediction and early diagnosis. *With the exponential growth of data, data mining is becoming increasingly essential in these industries and beyond.*
Industry | Application |
---|---|
Finance | Fraud detection and stock market predictions |
Retail | Customer behavior analysis, marketing strategies, and loyalty programs |
Healthcare | Disease prediction and early diagnosis |
Data mining processes typically involve several steps, including data collection, data preparation, modeling, evaluation, and deployment. First, data is collected from various sources such as databases, websites, and social media platforms. Next, the data is cleaned, transformed, and consolidated to ensure its quality and compatibility. Then, models are built using algorithms that analyze the data and uncover underlying patterns. These models are evaluated to assess their accuracy and effectiveness, after which the insights gained are deployed into practical applications.
Data Mining Process Steps:
- Data collection from multiple sources.
- Data preparation by cleaning, transforming, and consolidating.
- Modeling using algorithms to uncover patterns.
- Evaluation of models for accuracy and effectiveness.
- Deployment of insights gained into practical applications.
Algorithm | Application |
---|---|
Decision Trees | Classification and prediction |
Neural Networks | Pattern recognition and forecasting |
Apriori | Association rule mining |
It is important to consider ethical and legal aspects when performing data mining. Organizations must ensure compliance with data protection laws and acquire informed consent from individuals whose data is being analyzed. Anonymizing data can be another protective measure to safeguard privacy. In addition, organizations should be transparent about their data mining practices and provide clear opt-out options to individuals concerned about the use of their data.
In conclusion, data mining is a vital part of business intelligence that enables organizations to gain valuable insights from vast amounts of data. By employing various techniques and algorithms, businesses can make informed decisions and drive performance in diverse industries. With proper consideration for ethical and legal implications, data mining can unlock opportunities and deliver competitive advantages for businesses in the modern data-driven world.
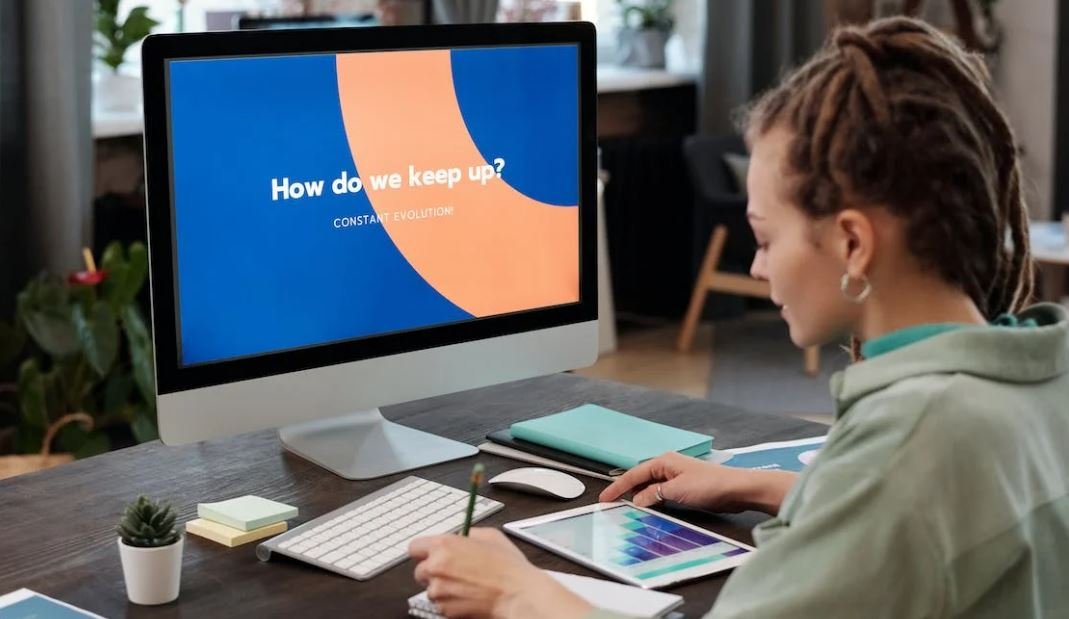
Common Misconceptions
1. Data mining is the same as business intelligence
One common misconception is that data mining and business intelligence are the same thing. While they are related, they are not synonymous. Data mining is the process of extracting patterns and knowledge from large sets of data, whereas business intelligence involves the collection, analysis, and reporting of data to help organizations make informed decisions.
- Data mining involves finding hidden patterns and relationships in data.
- Business intelligence focuses on analyzing and reporting data to gain insights.
- Both data mining and business intelligence play crucial roles in leveraging data for decision-making.
2. Data mining always violates privacy
Another misconception surrounding data mining is that it always involves a violation of privacy. While it is true that data mining can be used for unethical purposes, such as extracting sensitive information without consent, it is not inherently privacy-invasive. Data mining can be conducted ethically and legally by ensuring proper data anonymization, obtaining explicit consent, and adhering to privacy regulations.
- Data mining can be conducted in a privacy-preserving manner.
- Organizations should adopt ethical practices to protect user privacy during data mining activities.
- Proper data anonymization techniques can help prevent privacy violations during data mining.
3. Data mining is a guarantee for success
Some individuals believe that implementing data mining in their business automatically ensures success. However, this is not the case. While data mining can provide valuable insights and help identify opportunities, success still relies on various other factors such as strategy, execution, market dynamics, and customer satisfaction.
- Data mining is a tool that aids decision-making but does not guarantee success on its own.
- Success requires a comprehensive approach that considers multiple aspects beyond just data mining.
- Data mining is most effective when combined with other business strategies and practices.
4. Data mining is expensive and complex
Many people believe that implementing data mining in their business is a costly and complex endeavor. While it is true that data mining can be resource-intensive, advancements in technology have made it more accessible and affordable. There are various tools and platforms available that simplify the data mining process and help organizations leverage their data effectively.
- Data mining tools and platforms are becoming more user-friendly and affordable.
- Data mining can be scaled according to the organization’s size and needs.
- Training and education programs exist to demystify data mining and make it more accessible to businesses.
5. Data mining replaces human decision-making
Although data mining can assist in decision-making processes, it does not replace human judgment and expertise. Data mining algorithms provide insights and recommendations based on patterns and trends in data, but it is up to individuals to interpret and incorporate these insights into their decision-making process.
- Data mining serves as a valuable tool to support decision-making, but humans play a crucial role in analyzing and applying the insights.
- Data mining algorithms require human interpretation and contextual understanding.
- Data mining complements human decision-making but does not replace it.
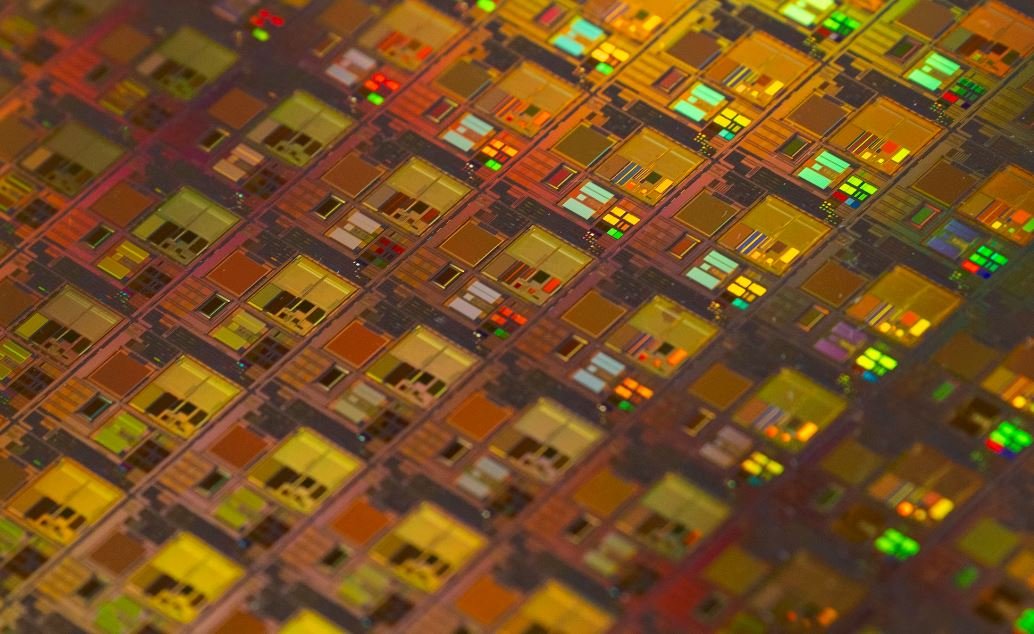
The Growth of Data Mining Business Intelligence
Data mining, a crucial aspect of business intelligence, involves the exploration and analysis of large sets of data to uncover meaningful patterns and insights. The practice has gained considerable prominence in recent years, as organizations strive to leverage their data to drive informed decision-making and gain a competitive edge. In this article, we dive into ten intriguing tables that exemplify the various aspects and benefits of data mining business intelligence.
E-commerce Sales by Country (in Millions)
This table showcases e-commerce sales figures by country, providing a snapshot of the market size and potential opportunities. By analyzing this data, businesses can identify which countries offer the highest revenue potential and tailor their strategies accordingly.
| Country | Sales |
| ————— | ———: |
| United States | $542.6 |
| China | $505.8 |
| Japan | $122.6 |
| United Kingdom | $99.3 |
| Germany | $96.4 |
| France | $94.1 |
| South Korea | $82.6 |
| Canada | $64.4 |
| India | $61.4 |
| Australia | $40.9 |
Customer Churn Rates by Industry (%)
This table reveals the customer churn rates across diverse industries, shedding light on which sectors struggle the most with retaining customers. Understanding the specific industries prone to higher churn rates enables businesses to implement targeted retention strategies and reduce customer attrition.
| Industry | Churn Rate |
| ————————- | :——–: |
| Telecommunications | 23.5 |
| Subscription Services | 18.9 |
| Cable/Satellite TV | 16.7 |
| Internet Service Providers| 15.2 |
| Insurance | 14.6 |
| E-commerce | 12.8 |
| Banking | 11.4 |
| Retail | 10.9 |
| Utilities | 9.6 |
| Hospitality | 7.3 |
Return on Investment (ROI) Across Marketing Channels (%)
This table outlines the average return on investment (ROI) achieved through different marketing channels. Analyzing these figures helps businesses identify the most effective channels for maximizing their marketing budget and driving revenue growth.
| Marketing Channel | ROI |
| —————— | :—: |
| Email Marketing | 42.7 |
| Social Media Ads | 38.2 |
| Search Engine Ads | 34.6 |
| Content Marketing | 30.1 |
| Influencer Marketing | 28.9 |
| TV Commercials | 25.2 |
| Print Advertising | 20.6 |
| Radio Ads | 18.3 |
| Outdoor Billboards | 15.7 |
| Direct Mail | 11.9 |
Average Employee Productivity by Industry (Units)
This table presents the average productivity of employees across different industries, offering insights into industries that typically have high levels of output per employee. Understanding these variations in productivity can help organizations streamline their operations and potentially identify areas for improvement.
| Industry | Productivity |
| ———————- | :———-: |
| Technology | 2500 |
| Manufacturing | 1800 |
| Finance and Insurance | 1500 |
| Professional Services | 1200 |
| Healthcare | 1000 |
| Retail | 800 |
| Construction | 700 |
| Hospitality | 500 |
| Agriculture | 400 |
| Arts and Entertainment | 300 |
Customer Satisfaction Ratings by Product Category (out of 5)
This table highlights customer satisfaction ratings for various product categories, helping businesses identify areas of strength and weakness in their offerings. By assessing these ratings, organizations can make informed decisions on product development and customer experience improvements.
| Product Category | Satisfaction Rating |
| ——————- | :—————–: |
| Electronics | 4.2 |
| Home Appliances | 4.1 |
| Fashion | 3.9 |
| Beauty and Cosmetics | 3.8 |
| Health and Wellness | 3.7 |
| Toys and Games | 3.6 |
| Furniture | 3.5 |
| Automotive | 3.4 |
| Sports and Fitness | 3.3 |
| Books | 3.2 |
Annual Revenue Growth by Industry (%)
This table illustrates the annual revenue growth achieved by different industries, giving businesses insights into the sectors experiencing the highest levels of growth. Understanding these trends can help organizations prioritize resources and make strategic decisions for future business expansion.
| Industry | Growth Rate |
| ———————– | :———: |
| Technology | 10.5 |
| E-commerce | 9.2 |
| Healthcare | 7.8 |
| Renewable Energy | 6.3 |
| Financial Services | 6.1 |
| Food and Beverage | 5.9 |
| Construction | 4.7 |
| Automotive | 4.4 |
| Retail | 3.6 |
| Higher Education | 3.2 |
Customer Demographics and Preferred Payment Methods
This table provides insights into customer demographics and their preferred payment methods, helping businesses tailor their marketing and sales strategies accordingly. By analyzing this data, organizations can align their offerings with customer preferences and enhance the overall customer experience.
| Age Group | Gender | Preferred Payment Method |
| ——— | —— | ———————— |
| 18-24 | Male | Credit Card |
| 18-24 | Female | Digital Wallet |
| 25-34 | Male | Digital Wallet |
| 25-34 | Female | Credit Card |
| 35-44 | Male | Debit Card |
| 35-44 | Female | Debit Card |
| 45-54 | Male | Cash |
| 45-54 | Female | Credit Card |
| 55+ | Male | Cash |
| 55+ | Female | Debit Card |
Customer Lifetime Value (CLTV) by Segment ($)
This table displays the customer lifetime value (CLTV) for different customer segments, allowing organizations to identify their most valuable customer groups. By understanding the CLTV by segment, businesses can prioritize their resources and customize their strategies to maximize profitability.
| Customer Segment | CLTV |
| —————- | —— |
| High-Value | $5,200 |
| Mid-Value | $3,400 |
| Low-Value | $950 |
| New Customers | $280 |
| VIP Customers | $8,700 |
| Occasional Buyers | $710 |
| Loyal Customers | $4,600 |
| Churned Customers| $40 |
| Dormant Customers| $20 |
| Potential Market | $12,000|
Data Security Breaches by Industry (2021)
This table highlights the number of data security breaches reported in different industries in the year 2021. It assists businesses in understanding the potential risks and vulnerabilities in various sectors, enabling them to implement robust security measures and safeguard sensitive information.
| Industry | Number of Breaches |
| ———————-| :—————-: |
| Technology | 156 |
| Finance and Insurance | 90 |
| Healthcare | 72 |
| Retail | 57 |
| E-commerce | 44 |
| Education | 38 |
| Government | 29 |
| Hospitality | 22 |
| Manufacturing | 18 |
| Telecommunications | 12 |
Conclusion:
Data mining and business intelligence have become integral to modern organizations’ success. The ten tables presented in this article provide valuable insights into various aspects of data mining, including e-commerce sales, customer churn rates, marketing ROI, employee productivity, customer satisfaction, revenue growth, customer demographics, CLTV, and data security breaches. By leveraging these tables and analyzing the data, businesses can make informed decisions, optimize their processes, and stay ahead of the competition in today’s data-driven business landscape.
Frequently Asked Questions
What is data mining in the context of business intelligence?
Answer goes here…
What are the benefits of data mining for businesses?
Answer goes here…
What are the common techniques used in data mining?
Answer goes here…
How is data mining different from business intelligence?
Answer goes here…
What are the challenges associated with data mining for business intelligence?
Answer goes here…
What tools are commonly used for data mining in business intelligence?
Answer goes here…
How can data mining be effectively implemented in business intelligence?
Answer goes here…
What are some real-world applications of data mining in business intelligence?
Answer goes here…
What are the ethical considerations when using data mining for business intelligence purposes?
Answer goes here…
What are some potential future advancements in data mining for business intelligence?
Answer goes here…