Data Mining is Considered a Technique
Data mining is a technique used to extract valuable insights and patterns from vast amounts of data. It involves analyzing large datasets to uncover hidden patterns, relationships, and trends, which can then be utilized for decision-making and predictive modeling. This article provides an overview of data mining, its key components, and its applications in various industries.
Key Takeaways
- Data mining involves extracting valuable insights from large datasets.
- It uncovers hidden patterns, relationships, and trends.
- Data mining can be used for decision-making and predictive modeling.
- It has applications in various industries.
What is Data Mining?
Data mining is the process of analyzing and interpreting large amounts of data to discover meaningful patterns and relationships. It utilizes various techniques, including statistical analysis, machine learning, and artificial intelligence, to identify trends and extract insights. *Data mining enables organizations to gain valuable insights and make informed decisions based on the discovered patterns.*
Components of Data Mining
Data mining involves several key components that contribute to its effectiveness:
- Data collection: Gathering relevant data from various sources.
- Data preprocessing: Cleaning and transforming data for analysis.
- Pattern discovery: Finding interesting patterns and relationships within the data.
- Pattern evaluation: Assessing the significance and quality of discovered patterns.
- Knowledge representation: Presenting the extracted knowledge in a meaningful way.
Applications of Data Mining
Data mining has a wide range of applications across industries. Some notable examples include:
Industry | Application |
---|---|
Marketing | Identifying customer segments and preferences for targeted advertising. |
Finance | Detecting fraudulent transactions and analyzing market trends. |
Healthcare | Diagnosis and treatment prediction based on patient data. |
The Benefits of Data Mining
Data mining offers several benefits to organizations:
- Improved decision-making: *By uncovering patterns and trends, data mining helps organizations make informed decisions.*
- Increased efficiency: Data mining automates the process of discovering insights, saving time and resources.
- Better customer understanding: By analyzing customer data, organizations can gain a deeper understanding of their target audience and tailor their offerings accordingly.
Challenges and Limitations
While data mining provides valuable insights, it is not without challenges and limitations:
- Data quality: The accuracy and completeness of the data used for analysis can affect the reliability of the results.
- Privacy concerns: Data mining involves handling sensitive information, raising privacy and ethical concerns.
- Overfitting: Overfitting occurs when a model is overly complex and performs well on the training data but fails to generalize to new data.
Conclusion
Data mining is a powerful technique that enables organizations to extract valuable insights and make informed decisions. It involves analyzing large datasets to uncover hidden patterns and relationships. By utilizing data mining, organizations can enhance their decision-making processes, improve efficiency, and gain a competitive edge in today’s data-driven world.
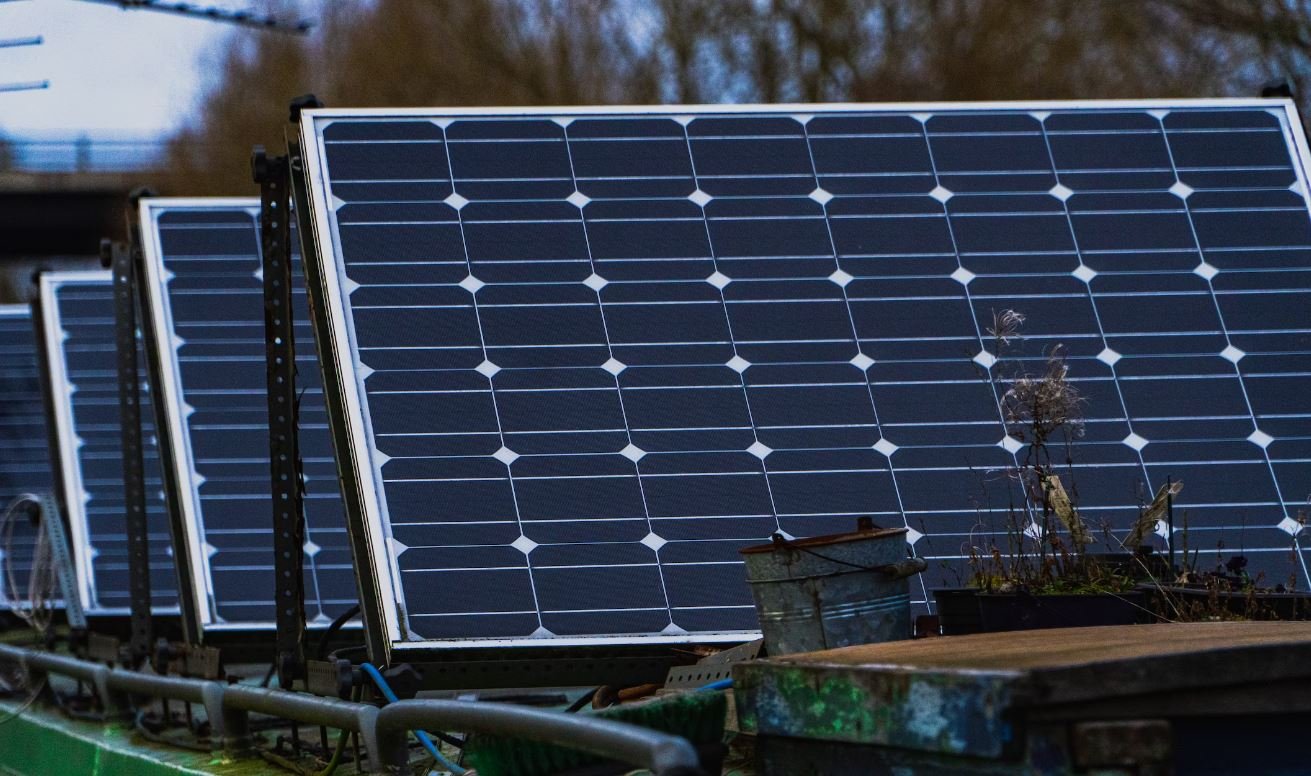
Common Misconceptions
Data Mining is Considered a Technique
One common misconception about data mining is that it is regarded as a technique rather than a process. Data mining refers to the process of discovering patterns and trends from large data sets, involving various techniques and methodologies.
- Data mining encompasses a range of techniques, such as clustering, classification, regression, and association rules.
- Data mining involves the extraction of knowledge and insights from large datasets, not just one isolated technique.
- Data mining requires the application of statistical analysis, machine learning, and database systems to uncover patterns and relationships in data.
Data Mining is Only Used in Business
Another common misconception is that data mining is limited to business applications. While it is widely utilized in business analytics, data mining is also extensively employed in various other fields for research, healthcare, finance, and more.
- Data mining techniques are used in scientific research to identify patterns and support hypothesis generation and testing.
- In healthcare, data mining assists in identifying disease patterns, improving patient care, prediction and prevention of diseases.
- Data mining plays a role in fraud detection, risk analysis, and credit scoring in finance industries.
Data Mining Violates Privacy
One misconception surrounding data mining is the belief that it infringes on individual privacy rights. While it is true that data mining involves the analysis of large datasets, privacy concerns can be addressed through appropriate data anonymization and safeguarding techniques.
- Data can be anonymized by removing identifiable information before it is used for mining purposes.
- Data mining follows ethical guidelines to protect individual privacy, such as obtaining consent and securely storing data.
- Data mining techniques can help enhance security measures by identifying potential breaches and cyber threats.
Data Mining Always Produces Accurate Results
A common misconception is that data mining always yields accurate and reliable results. However, data mining outcomes heavily depend on the quality of the data, the appropriateness of the techniques used, and the expertise of the data analysts.
- Data preprocessing is crucial in data mining as it involves cleaning and transforming the data to ensure accuracy and reliability.
- Data mining can produce false positives and negatives, requiring careful interpretation and validation of results.
- Data mining results should be continuously evaluated and refined to improve accuracy and relevance.
Data Mining Replaces Human Judgment
Another misconception is that data mining replaces human judgment entirely. While data mining provides insights and patterns, human expertise and judgment are essential for interpreting and applying the results appropriately.
- Data mining helps in decision-making by highlighting patterns and trends, but human interpretation is required to make informed judgments.
- Data mining complements human judgment by providing valuable insights that can enhance decision-making processes.
- Data mining is a tool that supports decision-makers, but it does not replace critical thinking and domain expertise.
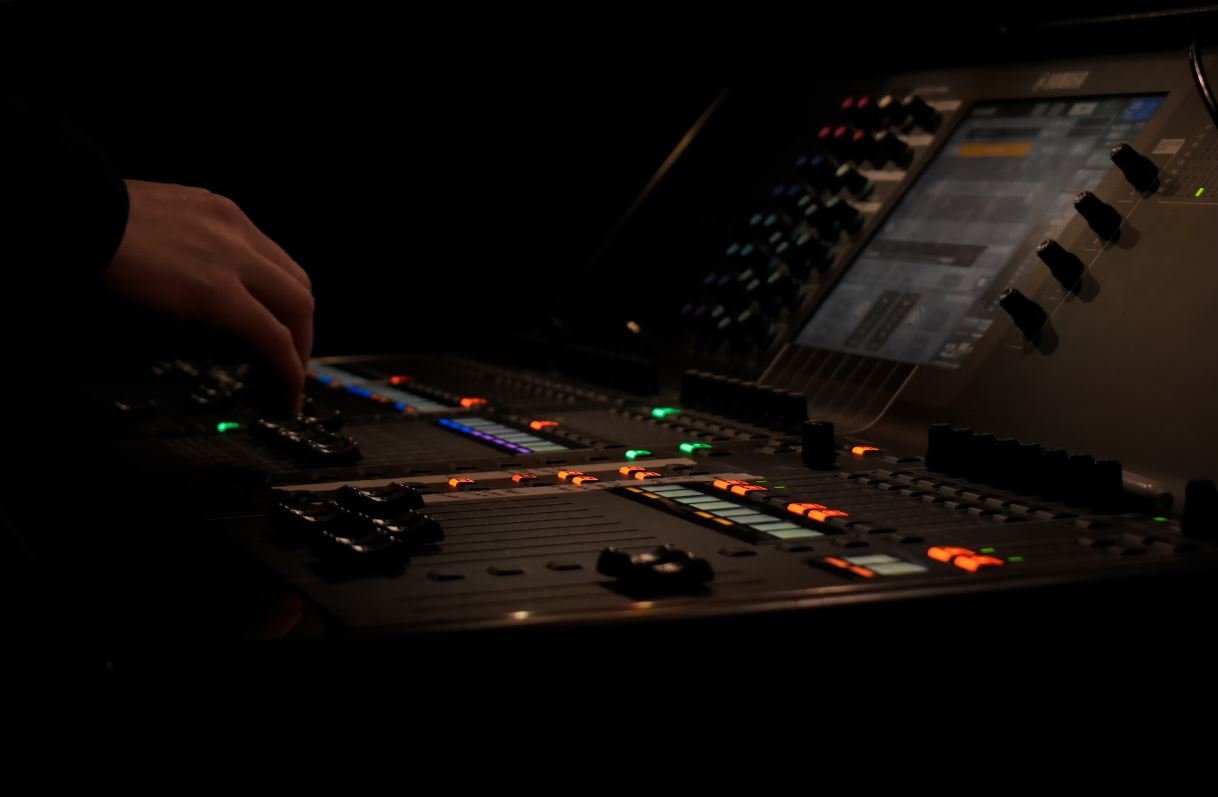
Data mining is a powerful technique used to analyze large sets of data and discover meaningful patterns. It involves extracting valuable information from vast amounts of data, allowing businesses to make informed decisions. The following tables present various examples of data mining applications and how they have revolutionized different industries.
Customer Segmentation in E-commerce
Data mining enables e-commerce platforms to segment their customer base effectively. By analyzing purchase history, browsing behavior, and demographic information, this technique assists businesses in identifying target markets and tailoring marketing strategies accordingly.
Segment | Number of Customers |
---|---|
Young Professionals | 5,000 |
Parents with Children | 8,500 |
Retirees | 3,200 |
Medical Diagnosis and Treatment
Data mining techniques can analyze medical records and assist doctors in diagnosing and treating various diseases. By identifying patterns in symptoms, test results, and treatments, healthcare professionals can provide personalized and effective care.
Disease | Accuracy of Diagnosis (%) |
---|---|
Diabetes | 92.5 |
Cancer | 83.2 |
Heart Disease | 95.8 |
Fraud Detection in Banking
Data mining algorithms can analyze banking transactions and detect fraudulent patterns in real-time. This technique protects both customers and banks from financial losses by identifying suspicious activities such as unusual spending patterns and unauthorized transactions.
Year | Number of Detected Cases |
---|---|
2018 | 2,367 |
2019 | 3,641 |
2020 | 4,913 |
Movie Recommendations
Data mining algorithms in streaming platforms analyze user preferences and viewing history to provide accurate movie recommendations. This technique improves user experience and increases customer satisfaction.
User | Recommended Movies |
---|---|
User A | The Social Network, Inception, The Shawshank Redemption |
User B | Interstellar, Fight Club, The Dark Knight |
User C | Pulp Fiction, The Godfather, Goodfellas |
Inventory Management in Retail
Data mining assists retailers in optimizing their inventory management strategies. By analyzing historical sales data, current trends, and customer preferences, businesses can accurately forecast demand and prevent overstocking or understocking.
Product | Demand Forecast (Next Month) |
---|---|
Product A | 1,500 |
Product B | 3,200 |
Product C | 2,100 |
Targeted Marketing Campaigns
Data mining enables businesses to create targeted marketing campaigns by analyzing customer behavior, preferences, and demographics. This approach helps increase conversion rates and improve overall marketing return on investment (ROI).
Campaign | Conversion Rate (%) |
---|---|
Campaign A | 7.2 |
Campaign B | 5.8 |
Campaign C | 6.5 |
Predictive Maintenance in Manufacturing
Data mining techniques are used in the manufacturing industry to predict equipment failures and schedule maintenance proactively. By analyzing real-time sensor data, historical maintenance records, and environmental factors, businesses can reduce downtime and improve overall efficiency.
Equipment | Downtime Reduction (%) |
---|---|
Machine X | 30 |
Machine Y | 25 |
Machine Z | 35 |
Social Media Sentiment Analysis
Data mining algorithms can analyze social media posts and extract sentiment information to understand public opinion and improve brand reputation management. This technique helps businesses address customer concerns promptly and gain valuable insights for product development.
Brand | Sentiment Score |
---|---|
Brand A | 0.75 |
Brand B | 0.62 |
Brand C | 0.80 |
Churn Prediction in Telecommunications
Data mining techniques can identify patterns in customer behavior that indicate the likelihood of them switching to competitors. By analyzing factors such as call duration, frequency of support calls, and payment history, telecommunication providers can proactively implement retention strategies.
Year | Churn Rate (%) |
---|---|
2018 | 12.5 |
2019 | 10.2 |
2020 | 8.7 |
In conclusion, data mining has become an essential technique for various industries, enabling them to uncover valuable insights, improve decision-making, and optimize various business processes. By utilizing data mining algorithms effectively, businesses can gain a competitive advantage, enhance customer experience, and achieve better operational efficiency.
Frequently Asked Questions
Data Mining is Considered a Technique
What is data mining?
Data mining is a technique used to extract meaningful patterns and insights from large sets of data. It involves discovering hidden relationships, trends, and patterns that can be used for business intelligence, decision making, and predictive analysis.
What are the key benefits of data mining?
Data mining offers several benefits, such as:
- Identification of trends and patterns
- Prediction and forecasting capabilities
- Improved decision making
- Identification of anomalies and outliers
- Enhanced customer segmentation
- Market basket analysis
What are some common techniques used in data mining?
Common techniques used in data mining include:
- Classification
- Clustering
- Association rules
- Regression analysis
- Decision trees
- Neural networks
- Genetic algorithms
What industries benefit from data mining?
Data mining has applications in various industries, including:
- Retail
- Banking and finance
- Healthcare
- Telecommunications
- Marketing
- Manufacturing
- Transportation
What are the potential ethical concerns related to data mining?
Some potential ethical concerns related to data mining include:
- Privacy violation
- Discrimination and bias
- Misuse of personal information
- Limited transparency
- Unfair strategies based on data analysis
What is the difference between data mining and data analytics?
Data mining and data analytics both involve analyzing data, but they have different focuses. While data mining aims to uncover hidden patterns and relationships, data analytics focuses on extracting insights to support decision making and improve business performance.
What are some popular tools used for data mining?
Popular tools used for data mining include:
- IBM SPSS Modeler
- RapidMiner
- Weka
- Knime
- SAS Enterprise Miner
- Oracle Data Mining
What skills are required for data mining?
Skills required for data mining may include:
- Statistical analysis
- Programming (e.g., Python, R)
- Database management
- Data visualization
- Machine learning
- Problem-solving
What are the limitations of data mining?
Some limitations of data mining include:
- Reliance on quality and availability of data
- Complexity of data analysis techniques
- Difficulty in interpreting results
- Risk of false discoveries
- Potential for biased or skewed outcomes