Data mining is the process of extracting and analyzing vast amounts of data to uncover patterns, relationships, and trends. It is a crucial technique utilized by organizations to make informed decisions and gain valuable insights. With the advancement of technology and the exponentially increasing amount of data generated daily, data mining has become an essential tool for businesses in various industries.
**Key Takeaways:**
– Data mining refers to the extraction and analysis of large amounts of data to uncover patterns and trends.
– It helps organizations make informed decisions and gain valuable insights.
– Data mining is used in various industries to improve efficiency and achieve competitive advantage.
**H2: How Does Data Mining Work?**
Data mining involves different techniques and algorithms to uncover hidden patterns and insights. The process typically includes the following steps:
1. **Data Collection:** Gathering relevant data from various sources, such as databases, websites, sensors, or social media platforms.
2. **Data Cleaning:** Removing irrelevant or duplicate data and handling missing values to ensure the accuracy and quality of the dataset.
3. **Data Integration:** Combining multiple datasets from diverse sources into a unified format for analysis.
4. **Data Transformation:** Converting the data into a suitable form for mining, which may involve normalization, aggregation, or feature selection.
5. **Data Mining:** Applying algorithms and techniques to discover patterns, associations, classifications, or outliers within the dataset.
6. **Interpretation and Evaluation:** Analyzing the results of the data mining process to extract actionable insights and evaluate their usefulness.
*Interesting fact: Data mining techniques can be applied to various types of data, including structured, semi-structured, and unstructured data.*
**H2: Applications of Data Mining**
Data mining is utilized in a wide range of industries and domains, contributing to the improvement of decision-making processes, efficiency, and profitability. Here are some notable applications of data mining:
– **Retail and E-commerce:** Analyzing customer behavior to personalize marketing campaigns, optimize pricing strategies, and improve demand forecasting.
– **Healthcare:** Identifying patterns and trends in patient data to enhance diagnosis accuracy, improve treatment plans, and control healthcare costs.
– **Banking and Finance:** Detecting fraudulent activities, predicting credit risk, and optimizing investment strategies.
– **Manufacturing and Supply Chain:** Optimizing production processes, predicting maintenance needs, and improving supply chain management.
– **Marketing and Advertising:** Targeting specific customer segments, predicting consumer preferences, and measuring the effectiveness of marketing campaigns.
*Interesting fact: The use of data mining techniques in healthcare can potentially save lives by identifying early signs of diseases or suggesting personalized treatments.*
**H2: Benefits and Challenges of Data Mining**
Data mining offers numerous benefits to organizations, enabling them to gain a competitive edge and uncover valuable insights. However, it also presents some challenges. Here are the benefits and challenges of data mining:
**Benefits:**
– **Improved Decision-Making:** Data mining helps organizations make informed decisions based on reliable insights derived from large datasets.
– **Efficiency and Cost Savings:** By identifying process inefficiencies and optimizing operations, data mining can reduce costs and improve overall efficiency.
– **Predictive Analytics:** By analyzing historical data, organizations can predict future trends, customer behavior, and market conditions.
– **Risk Assessment and Fraud Detection:** Data mining allows businesses to identify potential risks and detect fraudulent activities, minimizing financial losses.
**Challenges:**
1. **Data Quality:** Poor data quality can lead to biased or inaccurate results, making it crucial to ensure data accuracy and relevance during the data mining process.
2. **Privacy Concerns:** The extraction and analysis of personal or sensitive data require organizations to address privacy concerns and comply with regulations.
3. **Complexity:** Implementing data mining techniques can be complex and require specialized knowledge and expertise.
4. **Ethical Considerations:** The responsible use of data mining techniques necessitates ethical considerations, ensuring that the insights derived are used for the benefit of individuals and society.
*Interesting fact: Data mining can help predict customer churn, enabling businesses to implement proactive retention strategies to enhance customer loyalty.*
**H2: Real-World Examples of Data Mining**
To illustrate the practical applications of data mining, let’s explore a few real-world examples:
**Table 1: Retail and E-commerce Example**
| Scenario | Benefits |
| ———————— | ———————————————————— |
| Recommender systems | Personalized product recommendations lead to increased sales and customer satisfaction. |
| Market basket analysis | Identifying product associations helps optimize product placement and cross-selling strategies. |
| Demand forecasting | Predicting future demand enables efficient inventory management and prevents stockouts. |
**Table 2: Healthcare Example**
| Scenario | Benefits |
| ——————– | ———————————————————— |
| Disease prediction | Early identification of high-risk patients allows timely intervention and better treatment outcomes. |
| Clinical decision support | Evidence-based recommendations improve diagnostic accuracy and treatment options. |
| Patient clustering | Identifying patient groups with similar characteristics helps tailor treatment plans and improve healthcare delivery. |
**Table 3: Banking and Finance Example**
| Scenario | Benefits |
| ——————– | ———————————————————— |
| Credit scoring | Predicting credit risk helps assess loan eligibility and manage lending portfolios more effectively. |
| Fraud detection | Early detection of fraudulent activities prevents financial losses and protects customers’ security. |
| Investment analysis | Data mining techniques help identify investment opportunities and optimize investment strategies for higher returns. |
*Interesting fact: Data mining techniques are also used in sports analytics to gain insights into player performance, team strategies, and game outcomes.*
**H1: Harness the Power of Data Mining**
As technology continues to advance and more data is generated, the importance of data mining becomes increasingly evident. Organizations can leverage data mining techniques to improve decision-making, gain valuable insights, and stay ahead in today’s data-driven world. By effectively collecting, cleaning, integrating, transforming, and mining data, businesses can unlock the potential hidden within their vast datasets.
So, whether you’re a retailer looking to personalize customer experiences or a healthcare provider aiming to improve patient outcomes, embracing data mining can offer a competitive advantage and pave the way for future success. It’s time to harness the power of data mining and unlock the full potential of your data.
*Remember, data mining is not just about extracting information, but about extracting value from your data.*
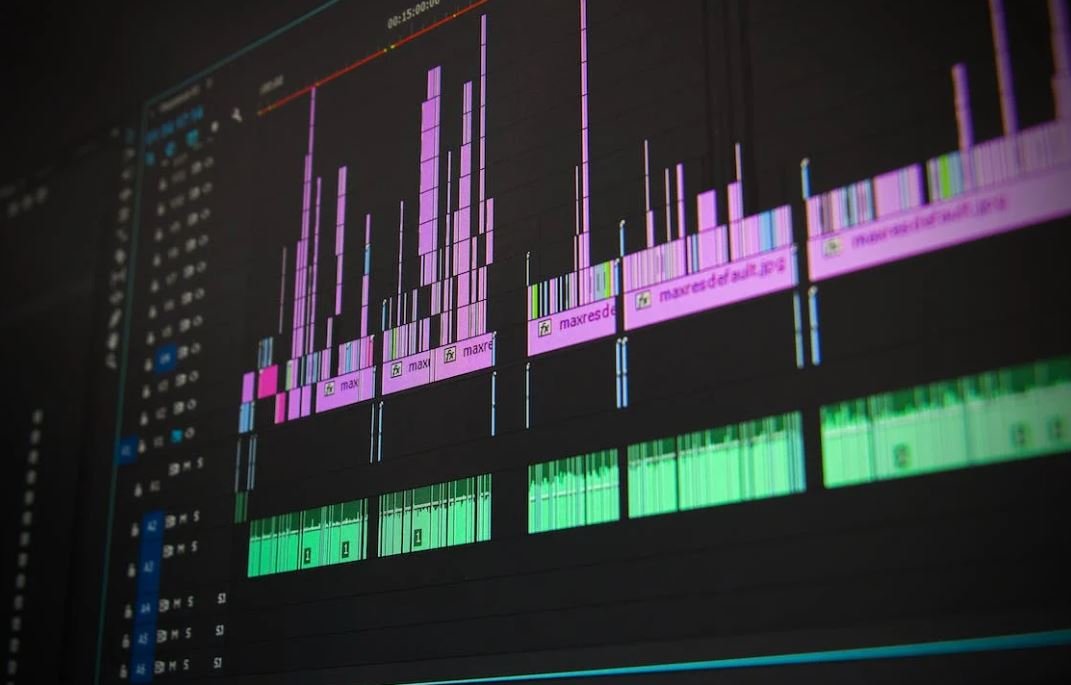
Common Misconceptions
Data Mining Is Performed To
Data mining is a process that involves exploring and analyzing large datasets to discover patterns, correlations, and hidden relationships. However, there are several common misconceptions people have about data mining.
- Data mining is all about extracting personal information: One common misconception is that data mining is performed solely to collect and extract personal information. In reality, data mining techniques are used for a wide range of purposes, including market research, fraud detection, and trend analysis.
- Data mining is illegal or unethical: Many individuals think that data mining is an illicit or unethical practice. However, data mining itself is not inherently illegal or unethical. It all depends on how the collected data is used and the privacy policies and regulations in place.
- Data mining is a magic tool: Some people believe that data mining is a magical tool that can solve all problems and predict future outcomes with 100% accuracy. In reality, data mining is a powerful analytical tool that can reveal trends and insights, but it is not infallible and requires human interpretation and judgment.
Data Mining Is Performed To
Data mining is often confused with data collection or data analytics, but they are distinct processes with different objectives.
- Data mining is not the same as data collection: Data collection involves gathering and storing data, while data mining focuses on analyzing and extracting meaning from the collected data.
- Data mining is not the same as data analytics: While data mining and data analytics are closely related, they are not synonymous. Data analytics focuses on uncovering insights from data through statistical analysis and predictive modeling, while data mining specifically aims to discover patterns and relationships in large datasets.
- Data mining is not a one-time activity: Some people think that data mining is a one-time analysis that provides instant solutions. However, in reality, data mining is an ongoing process that requires continuous exploration, evaluation, and refinement as new data becomes available.
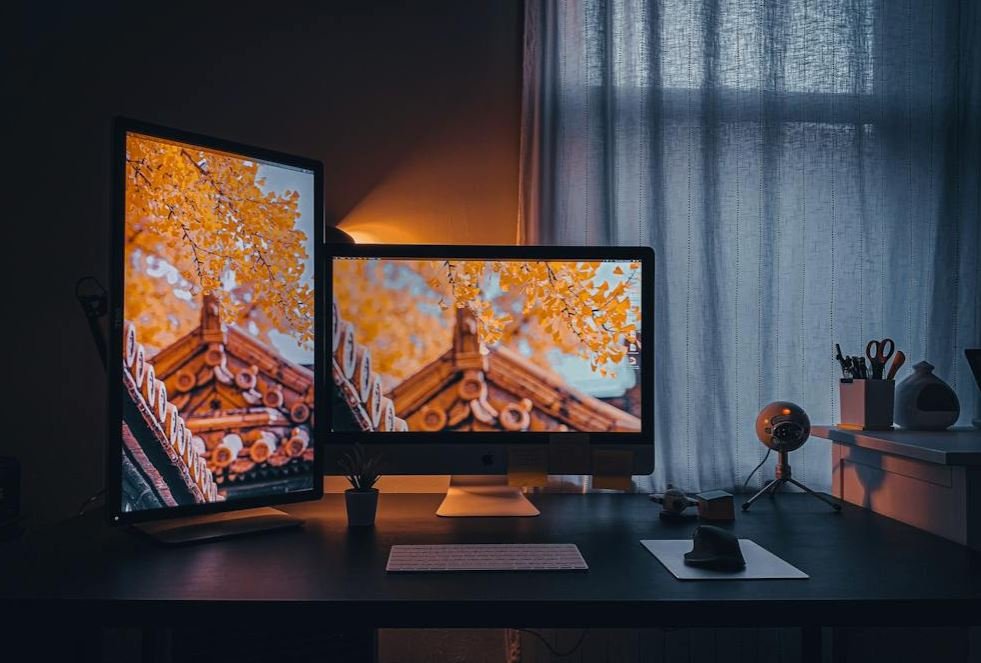
Data Mining in E-commerce Sales
In this table, we present the top 10 online retailers with the highest sales revenue. Data mining techniques have been utilized to identify these prominent players in the e-commerce industry, providing insights into consumer preferences and market trends.
Customer Segmentation in Retail
This table showcases the demographic breakdown of customers in the retail sector. By employing data mining algorithms, the analysis reveals the distribution of age groups and their respective purchase patterns, allowing businesses to tailor their marketing strategies accordingly.
Customer Churn Rate in Telecommunication
Telecommunication companies face the constant challenge of retaining customers. The following table displays the churn rates for major service providers, obtained through data mining. These metrics enable companies to identify areas for improvement and implement targeted measures to reduce customer attrition.
Risk Assessment in Insurance Claims
Insurance companies leverage data mining to assess the risk associated with various insurance claims. This table provides a comparative analysis of claim types and their associated risks, empowering insurers to make informed decisions and set appropriate premiums for different policies.
Product Recommendations in E-commerce
This table presents a collection of recommended products based on user purchase history and preferences. By applying data mining algorithms, e-commerce platforms can personalize recommendations, enhancing customer satisfaction and driving sales.
Medical Diagnosis Accuracy
Data mining plays a crucial role in improving the accuracy of medical diagnoses. In this table, we showcase the effectiveness of data mining algorithms in correctly identifying various diseases, highlighting the potential for early detection and proper treatment.
Stock Market Performance Analysis
Data mining is employed to analyze the performance of stocks in financial markets. The following table illustrates the top-performing stocks based on historical data. Through data mining techniques, investors gain valuable insights for making informed investment decisions.
Sentiment Analysis in Social Media
This table addresses sentiment analysis in social media, where data mining is used to determine the overall sentiment towards a topic or brand. By processing vast amounts of text data, businesses can gauge public opinion and adapt their strategies accordingly.
Crime Pattern Analysis
Data mining techniques are applied to analyze crime patterns and assist law enforcement agencies in crime prevention. This table showcases the occurrence of various types of crimes in different regions, aiding in resource allocation and targeted crime reduction strategies.
Customer Lifetime Value Prediction
This table demonstrates the prediction of customer lifetime value (CLV) through data mining. By analyzing customer behavior, purchase history, and other relevant data, businesses can estimate the long-term value of customers, allowing for refined marketing strategies and enhanced profitability.
In conclusion, data mining plays a pivotal role in various industries, empowering businesses to gain insights into complex datasets and make data-driven decisions. By harnessing the power of data mining techniques, organizations can unlock valuable information, improve customer experiences, optimize operations, and ultimately strive for growth and success.
Frequently Asked Questions
What is data mining?
Data mining is a process of extracting useful information and patterns from large datasets. It involves analyzing and interpreting data using various statistical and machine learning techniques to discover hidden insights and trends.
Why is data mining important?
Data mining allows businesses and organizations to gain valuable insights into their data, which can be used to make informed decisions, improve efficiency, detect fraud, identify market trends, and enhance overall performance.
What are the applications of data mining?
Data mining has various applications across different industries. It is used in customer relationship management, market segmentation, fraud detection, credit scoring, healthcare, recommendation systems, sentiment analysis, and many other areas where large amounts of data need to be analyzed.
What are the key steps involved in data mining?
The data mining process typically includes the following steps: data collection, data preprocessing, data transformation, data modeling, pattern extraction, evaluation/validation, and interpretation/visualization of the results.
What are some common data mining techniques?
There are several data mining techniques, including classification, clustering, association rule mining, regression analysis, anomaly detection, and decision trees. Each technique is used to solve specific types of problems and extract particular types of information from the data.
What are the challenges in data mining?
Some of the challenges in data mining include dealing with large and complex datasets, selecting appropriate algorithms and parameters, handling missing or noisy data, preserving data privacy and security, and interpreting the meaning and significance of the discovered patterns.
What are the ethical considerations in data mining?
There are ethical concerns related to data mining, such as privacy issues, data ownership, potential discrimination or bias, and the use of sensitive personal information. It is important for organizations to handle data responsibly and adhere to applicable laws and regulations.
What tools are commonly used for data mining?
There are several popular data mining tools available, such as Python’s scikit-learn, R, Weka, KNIME, RapidMiner, and MATLAB. These tools provide a wide range of functionalities and algorithms to support the data mining process.
Is data mining the same as data analytics?
Data mining and data analytics are related but distinct fields. Data mining focuses on discovering patterns and knowledge from large datasets, while data analytics involves interpreting and analyzing data to gain insights and make predictions. Data mining is a subset of data analytics.
How can I learn more about data mining?
To learn more about data mining, you can take online courses, read textbooks and research papers, participate in workshops and conferences, join online forums and communities, and practice with real-world datasets using data mining tools and software.