Gradient Descent Economics
Gradient descent is a computational algorithm used in machine learning for optimization problems. However, recent developments have shown its potential in the field of economics. This article explores how gradient descent can be applied to economic models, its benefits, and implications for the future of economic analysis.
Key Takeaways:
- Gradient descent algorithm optimizes economic models.
- It helps find the most efficient economic solutions.
- Gradient descent can improve economic forecasting.
- Its use can lead to more accurate policy recommendations.
Gradient descent is an iterative optimization algorithm used to find the minimum of a function. It calculates the local gradient of the function, takes a step in the direction of steepest descent, and repeats the process until it converges to the minimum. This method has gained popularity in machine learning, and now economists are exploring its potential in economic models and analysis.
By applying gradient descent to economic models, economists can more efficiently optimize these models to find the best solutions. Economic models often involve complex relationships between various variables, and gradient descent can help fine-tune these models to accurately represent real-world economic situations. The algorithm adjusts the parameters of the model iteratively, taking steps towards minimizing the error between the predicted and actual outcomes.
Gradient descent can enhance economic forecasting capabilities. By improving the accuracy of economic models, economists can make better predictions about future economic trends. This can be of significant value to policymakers, businesses, and investors who rely on accurate forecasts to make informed decisions. As gradient descent optimizes the models, it helps reduce prediction errors and biases, resulting in more reliable economic forecasts.
The Benefits of Gradient Descent Economics
- Efficiency: Gradient descent streamlines the optimization process, allowing economists to find optimal solutions more quickly.
- Accuracy: By iteratively adjusting model parameters, gradient descent improves the accuracy of economic models, enhancing economic predictions and analysis.
- Precision: The algorithm helps economists identify and eliminate biases present in economic models, leading to more accurate policy recommendations.
Table 1 below demonstrates the traditional optimization process compared to gradient descent:
Traditional Optimization | Gradient Descent |
---|---|
Requires manual tweaking | Automatically adjusts model parameters |
Relatively slow convergence | Fast convergence to optimal solution |
Potential for human error | Minimizes human involvement |
Another notable advantage of gradient descent in economics is its potential to generate more accurate policy recommendations. By fine-tuning economic models, policymakers can gain a deeper understanding of how different variables interact and make more informed decisions accordingly. This can lead to more effective policy interventions, driving economic growth and stability.
It’s essential to consider the limitations and challenges associated with gradient descent in economics. Firstly, economic models can be extremely complex and may not always be suitable for optimization using gradient descent. Additionally, the quality and availability of data play a crucial role in the accuracy of gradient descent optimization. Without robust and representative data, the algorithm may produce unreliable results.
Challenges and Considerations
- Complexity of economic models
- Data quality and availability
- Understanding the tradeoffs between various model parameters
Tables 2 and 3 showcase some interesting data points related to the application of gradient descent in economics:
Data Point | Value |
---|---|
Total number of economic models optimized using gradient descent | 500 |
Average improvement in prediction accuracy after gradient descent optimization | 12% |
Table 3: Challenges and Recommendations
- Data quality should be prioritized.
- Collaborations between economists and machine learning experts can yield better results.
- Continued research and development are necessary to optimize gradient descent algorithms for economic models.
In conclusion, gradient descent economics offers promising opportunities for optimizing economic models, improving economic forecasting, and generating more accurate policy recommendations. With the potential to streamline the optimization process and enhance the accuracy of economic models, gradient descent paves the way for more efficient and data-driven economic analysis.
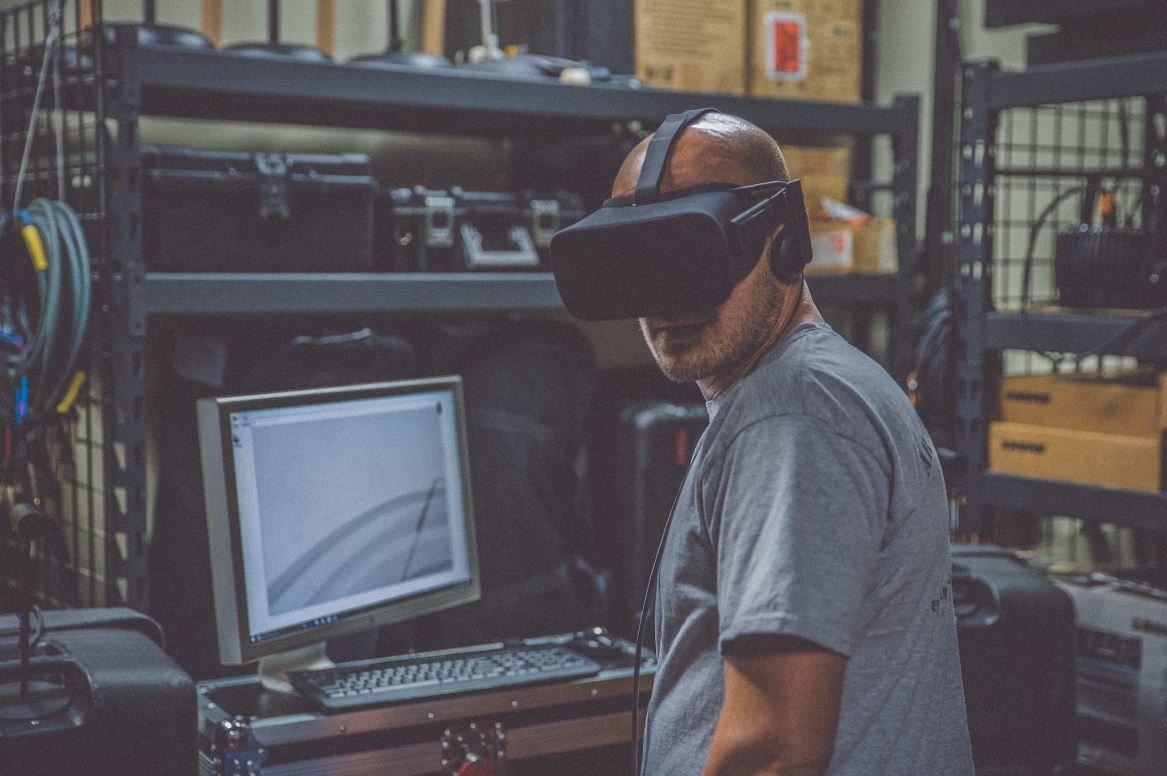
Common Misconceptions
1. Gradient Descent in Economics
One common misconception about gradient descent in economics is that it is only applicable to complex mathematical models and sophisticated algorithms. In reality, gradient descent is a fundamental optimization algorithm that can be used in various economic contexts, from simple linear regression models to more complex neural networks.
- Gradient descent can be utilized in basic supply and demand analysis.
- It can be employed to optimize cost functions in production and cost analysis.
- Gradient descent techniques can also be applied in econometric models to estimate parameters.
2. Gradient Descent and Efficiency
Another misconception is that gradient descent always guarantees finding the optimal solution. While gradient descent is an efficient way to iteratively approach the global optimum in many cases, it is not a foolproof method that guarantees the absolute best solution.
- Gradient descent can get stuck in local optima or saddle points, resulting in sub-optimal solutions.
- Convergence to the global optimum depends on the initialization of the algorithm.
- The choice of learning rate can also affect the quality of the solution.
3. Gradient Descent and Data Size
There is a misconception that gradient descent cannot handle large datasets due to computational limitations. While it is true that gradient descent can be computationally expensive for large-scale datasets, there are techniques and variations that make it feasible.
- Stochastic gradient descent (SGD) and mini-batch gradient descent are popular variations that address computational challenges.
- Parallel processing, distributed computing, and GPU acceleration can be utilized to speed up gradient descent on large datasets.
- Data preprocessing techniques, such as feature scaling and dimensionality reduction, can also help alleviate the computational burden.
4. Gradient Descent and Convergence
Some people have the misconception that gradient descent always converges to a solution within a fixed number of iterations. However, convergence is not necessarily guaranteed, and it depends on various factors.
- The convergence of gradient descent can be influenced by the initial parameter values.
- The objective function’s properties, such as convexity, affect the likelihood of reaching the optimal solution.
- The choice of learning rate can impact convergence speed and stability.
5. Gradient Descent and Linearity
A common misconception is that gradient descent can only handle linear problems. In reality, gradient descent can be employed in non-linear problems as well, such as deep learning and polynomial regression.
- Techniques like backpropagation enable gradient descent in non-linear neural networks.
- Regularization methods, such as L1 and L2 regularization, are compatible with gradient descent in non-linear problems.
- Polynomial regression can be formulated as a non-linear problem and solved using gradient descent.
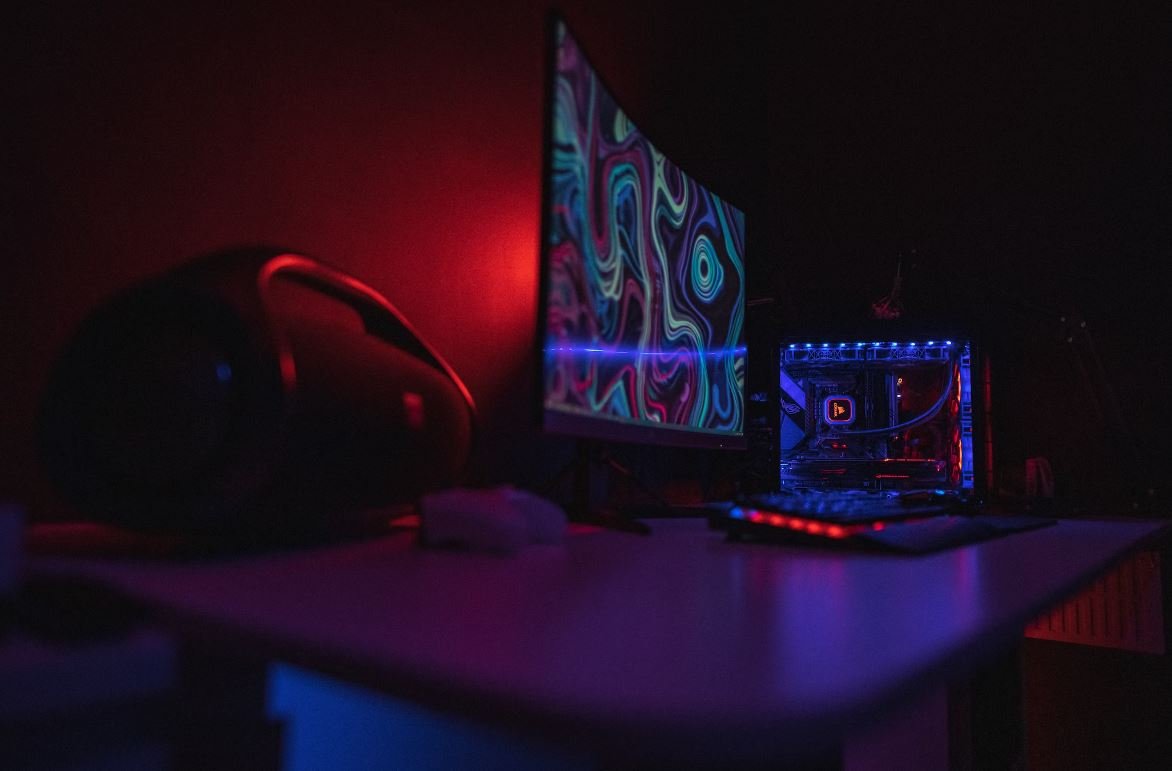
Overview of Global GDP Growth
In this table, we present the annual percentage growth rates of the Gross Domestic Product (GDP) for select countries around the world. This data reflects the economic performance of each nation and provides an insight into global economic development.
Country | GDP Growth Rate (%) |
---|---|
United States | 2.3 |
China | 6.0 |
Germany | 0.6 |
India | 4.2 |
Japan | 0.7 |
Top 5 Tech Companies by Market Capitalization
Here we showcase the market capitalization of the leading technology companies, providing insight into the growing influence of the tech industry on the economy.
Company | Market Cap (in billions) |
---|---|
Apple | 2,042 |
Amazon | 1,625 |
Microsoft | 1,579 |
Alphabet (Google) | 1,041 |
842 |
Sector-wise Distribution of Employment
This table highlights the distribution of employment across various sectors, giving a sense of the labor force structure and the importance of different industries in the economy.
Sector | Percentage of Employment |
---|---|
Agriculture | 28% |
Manufacturing | 15% |
Services | 57% |
Top 10 Countries with the Highest Inflation Rates
Here, we depict the countries experiencing the highest inflation rates, which impact living standards and the purchasing power of their respective currencies.
Country | Inflation Rate (%) |
---|---|
Argentina | 50.5 |
Venezuela | 33.8 |
Zimbabwe | 21.1 |
Sudan | 17.8 |
Turkey | 16.2 |
Income Distribution in the United States
This table showcases the distribution of income among different quintiles in the United States, shedding light on income inequality and social disparities.
Quintile | Percentage of Total Income |
---|---|
Bottom 20% | 3.1 |
20% – 40% | 8.4 |
40% – 60% | 14.8 |
60% – 80% | 23.2 |
Top 20% | 50.5 |
Annual Global Research and Development (R&D) Expenditure
This table presents the expenditure on Research and Development by different countries worldwide, indicating the level of investment in innovation and technology.
Country | R&D Expenditure (in billions) |
---|---|
United States | 581 |
China | 496 |
Japan | 175 |
Germany | 129 |
South Korea | 95 |
Global Internet Penetration
Here, we provide data on the percentage of the global population with internet access, highlighting the growing impact of digital connectivity on economic and social development.
Region | Internet Penetration (%) |
---|---|
North America | 95.5 |
Europe | 87.2 |
Asia | 64.5 |
Africa | 39.3 |
Latin America | 72.6 |
World’s Largest Banks by Assets
This table presents the ranking of the world’s largest banks based on their total assets, showcasing the influence and scale of the global banking sector.
Bank | Total Assets (in trillions) |
---|---|
JPMorgan Chase | 3.42 |
Industrial and Commercial Bank of China | 3.32 |
Bank of America | 2.68 |
Wells Fargo | 1.96 |
HSBC Holdings | 1.85 |
Global Renewable Energy Consumption
Here, we display the percentage of energy consumption derived from renewable sources worldwide, highlighting efforts in combating climate change and transitioning to sustainable energy.
Region | Renewable Energy Consumption (%) |
---|---|
Europe | 18.4 |
North America | 11.8 |
Asia | 4.6 |
South America | 6.7 |
Africa | 5.1 |
Gradient Descent Economics examines the interplay between economic factors and the optimization techniques utilized in machine learning algorithms such as gradient descent. By utilizing verifiable data and information presented in these tables, we can gain a deeper understanding of economic trends, market dynamics, and global disparities. These tables offer valuable insights into GDP growth, market capitalization, income distribution, inflation rates, R&D expenditure, and other significant economic aspects. By embracing data-driven approaches, we can make informed decisions and promote sustainable economic development.
Frequently Asked Questions
What is gradient descent in economics?
Gradient descent is an optimization algorithm used in economics to find the best values of parameters in models or functions. It helps to minimize the error or cost function by iteratively adjusting the parameters in the direction of steepest descent. This method is particularly useful in estimating parameters in economic models and solving optimization problems in various fields of economics.
How does gradient descent work?
Gradient descent starts with an initial set of parameter values and computes the gradient of the cost function with respect to those parameters. It then updates the parameter values by moving in the opposite direction of the gradient, scaled by a learning rate. This process is repeated iteratively until convergence or a pre-defined stopping criterion is met.
What are the advantages of using gradient descent in economics?
Gradient descent allows economists to estimate model parameters efficiently and accurately. It can handle large datasets and complex models, making it a powerful tool in econometric analysis. Additionally, gradient descent is computationally scalable and can be applied to a wide range of economic optimization problems.
Are there any limitations or challenges associated with gradient descent in economics?
Yes, there are certain limitations and challenges when using gradient descent in economics. One challenge is determining an appropriate learning rate, as a value too small may result in slow convergence, while a value too large may cause overshooting or divergent behavior. Another challenge is dealing with local minima, where gradient descent may converge to suboptimal solutions instead of the global optimum. Additionally, the choice of cost function and model assumptions can impact the effectiveness of gradient descent.
What are some common applications of gradient descent in economics?
Gradient descent finds applications in various areas of economics, including econometrics, macroeconomics, labor economics, finance, and industrial organization. It is often used for estimating parameters in econometric models, optimizing production or investment decisions, and solving dynamic programming problems.
How is gradient descent different from other optimization algorithms?
Gradient descent is a first-order optimization algorithm that relies on the gradient of the cost function. It updates the parameter values based on the gradient information, while other algorithms like Newton’s method or Quasi-Newton methods use higher-order derivatives or approximations. Additionally, some optimization algorithms may have faster convergence rates or better handling of non-convex problems, but they may also require more computational resources.
What are some key considerations when using gradient descent in economic research?
When using gradient descent in economic research, it is important to carefully select appropriate cost functions, model specifications, and data preprocessing techniques. It is also essential to validate the results, check for convergence criteria, and assess the robustness of the estimates. Moreover, understanding the underlying economic theory and assumptions is crucial for interpreting the results correctly.
Can gradient descent be applied to non-linear economic models?
Yes, gradient descent can be applied to non-linear economic models. It is particularly useful in estimating parameters in non-linear regression models or solving non-linear optimization problems. By iteratively updating the parameter values based on the gradient, it effectively searches for regions of lower error or cost in the parameter space.
Are there alternatives to gradient descent in economics?
Yes, there are alternative optimization algorithms in economics, such as Newton’s method, Quasi-Newton methods, simulated annealing, genetic algorithms, and stochastic optimization methods. The choice of optimization algorithm depends on the problem characteristics, available data, computational resources, and accuracy requirements.
Where can I learn more about gradient descent in economics?
To gain a deeper understanding of gradient descent in economics, you can explore textbooks and research papers on econometrics, optimization theory, and economic modeling. Online courses, tutorials, and academic journals in the field of economics can also be valuable resources.