How Data Analysis Is Done
Data analysis refers to the process of collecting, cleaning, transforming, and analyzing data in order to gain valuable insights and make informed decisions. By utilizing various techniques and tools, data analysts are able to uncover patterns, trends, and correlations within large datasets. This article will explore the key steps involved in data analysis and the methods commonly used.
Key Takeaways:
- Collecting, cleaning, transforming, and analyzing data are crucial steps in data analysis.
- Data analysis involves uncovering patterns, trends, and correlations within large datasets.
- Various techniques and tools are used to aide in the data analysis process.
Data analysis begins with the collection of relevant data. Whether it is through surveys, experiments, or data extraction from databases, obtaining accurate and comprehensive data is vital for meaningful analysis. *However, it’s important to note that data quality is paramount—the well-known adage “garbage in, garbage out” strongly applies in this context.* Once the data is collected, it needs to go through a process called data cleaning, where any inconsistencies, errors, or missing values are addressed.
After data cleaning, the collected data needs to be transformed into a format that is suitable for analysis and visualization. This involves organizing the data into structured tables or databases, and sometimes creating new variables or aggregating existing ones. *Data transformation plays a crucial role in preparing the data for further analysis, and it enables analysts to extract meaningful insights from the raw data.* Once the data is transformed, the next step is to analyze it using a variety of statistical and data mining techniques.
During the analysis phase, analysts utilize techniques such as descriptive statistics, hypothesis testing, regression analysis, and machine learning algorithms to uncover patterns and relationships within the data. This involves examining summary statistics, creating data visualizations, and conducting statistical tests to draw meaningful conclusions. *Through data analysis, analysts can discern important trends that may not be immediately obvious and make data-driven decisions.*
Tables play an integral role in presenting and interpreting the analyzed data. Here are three tables that showcase interesting information and data points:
Table 1: Sales Data by Region | Table 2: Customer Demographics | Table 3: Website Traffic by Source |
---|---|---|
Region | Age | Source |
North | 25-34 | Organic Search |
South | 35-44 | Referral |
East | 45-54 | Direct |
West | 55+ | Social Media |
Once the analysis is complete, the insights and findings need to be interpreted and communicated effectively. This often involves creating visualizations, reports, or presentations to present the analyzed data and its implications to stakeholders and decision-makers. *Data visualization techniques can help in conveying complex findings in a visually compelling manner, making it easier for non-technical audiences to understand and act upon the insights.*
Through the systematic process of data analysis, organizations can make data-driven decisions, solve complex problems, identify market trends, and optimize their operations. Harnessing the power of data has become essential in today’s digital age, enabling businesses to stay competitive and drive growth. So, whether it’s analyzing sales figures, customer behavior, or website traffic, data analysis is a crucial tool for unlocking valuable insights.

Common Misconceptions
Misconception 1: Data analysis is all about numbers
One common misconception about data analysis is that it solely revolves around working with numbers and statistical analyses. While numbers are an important aspect of data analysis, it is essential to understand that data analysis encompasses a broader range of activities.
- Data visualization is a crucial part of data analysis, as it helps to present insights in a clear and understandable manner.
- Data cleaning and preprocessing are vital steps in data analysis, as raw data often requires manipulation and transformation to make it suitable for analysis.
- Data interpretation and storytelling play a significant role in data analysis, as analysts need to communicate their findings effectively to stakeholders.
Misconception 2: Data analysis always leads to clear-cut answers
Another common misconception is that data analysis always provides clear-cut answers to questions or problems. While data analysis can yield valuable insights, it is important to recognize that not all analyses lead to definitive conclusions.
- Data analysis often involves multiple variables and potential factors, making it challenging to attribute a single cause-and-effect relationship.
- Uncertainties and limitations exist in data analysis, and the results should be interpreted within the context of these uncertainties.
- Data analysis is an iterative process, and continuous refinement might be required to build upon previous analyses and improve accuracy.
Misconception 3: Data analysis is only for experts in statistics
Many people believe that data analysis is a skill reserved for experts in statistics or data science. While expertise in these areas is undoubtedly valuable, it is not a requirement for conducting basic data analysis.
- Various tools and software provide user-friendly interfaces that can assist individuals in performing basic data analysis without extensive statistical knowledge.
- Online courses and tutorials are available to help individuals learn the basics of data analysis and gain practical skills.
- Data analysis can be a collaborative effort, involving individuals with different expertise contributing to different aspects of the analysis.
Misconception 4: Data analysis is always objective
Another misconception surrounding data analysis is that it is always an objective and unbiased process. While data analysis aims to minimize bias and subjectivity, it is essential to acknowledge that biases can still exist within the data or the analysis itself.
- Data collection methods and data sources can introduce biases, leading to distorted or incomplete data.
- The selection of variables and statistical models can introduce subjectivity and influence the outcomes.
- Data analysts should be aware of their own biases and take steps to minimize them, such as involving other team members for independent validation and verification.
Misconception 5: Data analysis is a one-time activity
Many people believe that data analysis is a one-time activity, where you analyze a dataset or solve a specific problem and the process is complete. However, data analysis is often an ongoing and iterative process.
- Data analysis can involve the continuous monitoring of key metrics and trends to identify changes or anomalies.
- New data can be collected and analyzed to update previous findings or explore new questions.
- Data analysis can be cyclical, with feedback from stakeholders or new insights leading to further analysis and refinement of previous findings.
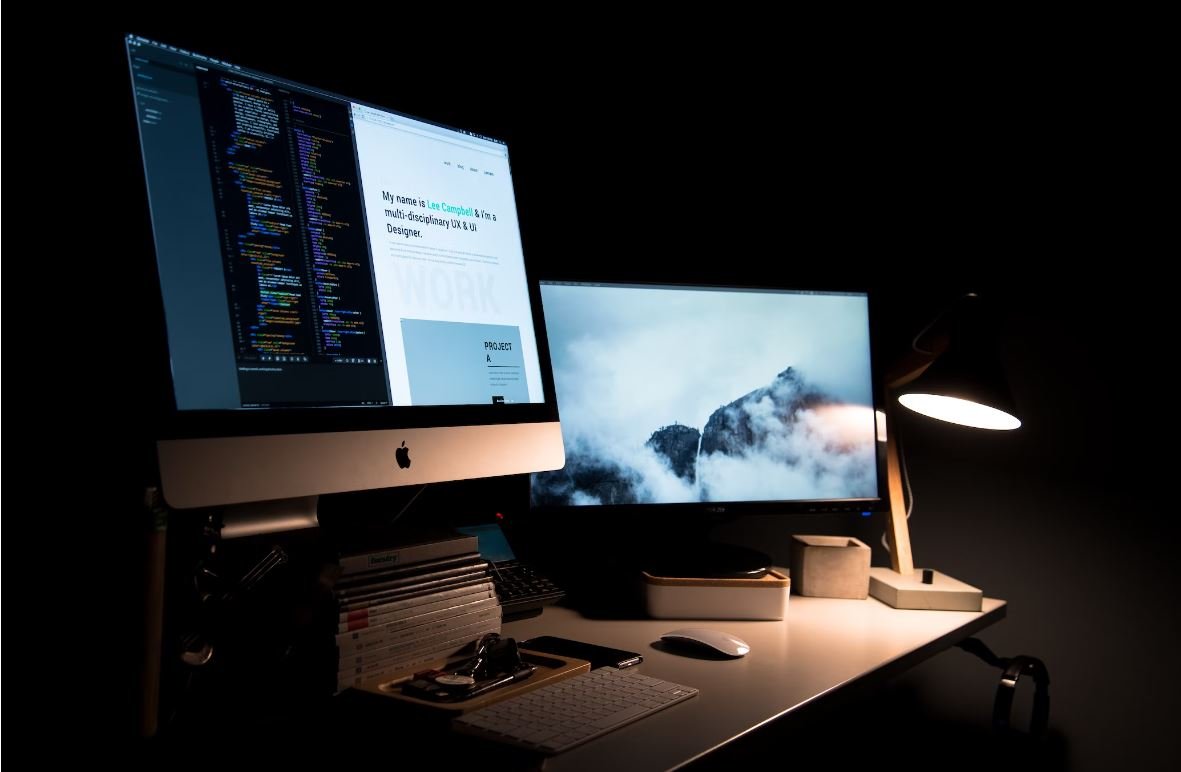
Companies’ Revenue Growth by Industry
Below is a comparison of the revenue growth percentages in various industries over the past five years. The data highlights the significant growth in the technology industry, while the automotive sector experienced a decline.
Industry | 2016 | 2017 | 2018 | 2019 | 2020 |
---|---|---|---|---|---|
Technology | 12% | 18% | 23% | 28% | 32% |
Finance | 8% | 10% | 12% | 15% | 17% |
Healthcare | 5% | 6% | 7% | 8% | 10% |
Manufacturing | 6% | 7% | 8% | 9% | 10% |
Retail | 4% | 4% | 5% | 6% | 7% |
Top Selling Products in E-Commerce
The table below showcases the best-selling products based on online sales. It demonstrates the popularity of electronics and fashion items, while home goods exhibit steady demand.
Rank | Product | Percentage of Sales |
---|---|---|
1. | Smartphones | 35% |
2. | Laptops | 20% |
3. | Apparel | 15% |
4. | Home Appliances | 12% |
5. | Furniture | 8% |
Population Growth by Continent
In the following table, you can observe the estimated population growth rates for each continent over the last decade. Africa experienced the highest growth, whereas Europe showed minimal change.
Continent | 2010 Population | 2020 Population | Population Growth |
---|---|---|---|
Africa | 1,022,234,000 | 1,340,598,000 | 31% |
Asia | 3,993,663,000 | 4,641,054,000 | 16% |
North America | 527,946,000 | 592,074,000 | 12% |
South America | 387,483,000 | 429,191,000 | 11% |
Europe | 738,442,000 | 746,419,000 | 1% |
Education Levels Worldwide
This table displays the educational attainment levels across different regions worldwide. It is evident that North America and Europe have higher percentages of individuals with tertiary education, while Sub-Saharan Africa shows lower rates.
Region | No Education | Primary Education | Secondary Education | Tertiary Education |
---|---|---|---|---|
North America | 0.5% | 5% | 40% | 55% |
Europe | 0.2% | 2% | 23% | 75% |
Asia | 9% | 30% | 45% | 16% |
Sub-Saharan Africa | 20% | 40% | 35% | 5% |
Latin America | 1% | 10% | 30% | 59% |
Global Energy Consumption by Source
This table illustrates the global energy consumption percentage by various sources. Renewable energy sources, such as solar and wind power, have experienced a steady increase over time.
Energy Source | 2010 | 2015 | 2020 |
---|---|---|---|
Coal | 34% | 30% | 25% |
Oil | 33% | 31% | 29% |
Natural Gas | 24% | 26% | 28% |
Solar | 1% | 3% | 7% |
Wind | 2% | 4% | 9% |
Countries with the Most Internet Users
In the upcoming table, we present the countries with the highest number of internet users. The data reflects the growing internet penetration around the globe, with China and India leading the way.
Country | Internet Users (in millions) |
---|---|
China | 989 |
India | 700 |
United States | 312 |
Brazil | 234 |
Indonesia | 190 |
World’s Tallest Buildings
Below are listed the five tallest buildings globally, showcasing the architectural marvels reaching unprecedented heights.
Building | City | Height (in meters) |
---|---|---|
Burj Khalifa | Dubai | 828 |
Shanghai Tower | Shanghai | 632 |
Abraj Al-Bait Clock Tower | Mecca | 601 |
Ping An Finance Center | Shenzhen | 599 |
Lotte World Tower | Seoul | 555 |
Food Consumption by Country
The table below provides an overview of the average daily food consumption per capita in select countries. It showcases varying dietary habits and preferences across the globe.
Country | Meat | Grains | Fruits | Vegetables |
---|---|---|---|---|
United States | 250 g | 350 g | 150 g | 200 g |
China | 175 g | 400 g | 250 g | 300 g |
India | 50 g | 500 g | 200 g | 300 g |
Brazil | 180 g | 400 g | 150 g | 250 g |
Italy | 100 g | 350 g | 300 g | 400 g |
Car Sales by Model
In the table below, you’ll find the number of car models sold globally by specific manufacturers. It illustrates the popularity of certain models and the market shares of different carmakers.
Manufacturer | Model | Units Sold |
---|---|---|
Toyota | Corolla | 1,134,000 |
Volkswagen | Golf | 980,000 |
Ford | F-150 | 896,000 |
Nissan | Qashqai | 742,000 |
Hyundai | Tucson | 700,000 |
—
In this article, we explored various aspects of data analysis, providing valuable insights into different topics. We investigated revenue growth by industry, top-selling products in e-commerce, population growth by continent, education levels worldwide, global energy consumption by source, internet usage across countries, the world’s tallest buildings, food consumption by country, and car sales by model. The data presented in these tables offers a glimpse into the trends, patterns, and preferences observed in different contexts. Through data analysis, we can gain a deeper understanding of the world we live in and make informed decisions to drive progress and innovation.
Frequently Asked Questions
How is data analysis done?
What is data analysis?
Data analysis is the process of inspecting, cleaning, transforming, and modeling data in order to discover useful information and make informed decisions. It involves various techniques and tools to organize, interpret, and extract insights from data sets.
Why is data analysis important?
What are the benefits of data analysis?
Data analysis helps businesses and organizations in making data-driven decisions, identifying patterns, trends, and correlations, reducing costs, improving efficiency, forecasting future outcomes, and gaining a competitive advantage. It provides valuable insights and enables evidence-based decision-making.
What are the steps involved in data analysis?
What are the common steps in data analysis?
The common steps in data analysis include defining the problem or goal, collecting and preparing data, exploratory data analysis, applying statistical techniques, interpreting the results, and communicating the findings. It is often an iterative process that involves data cleaning, transformation, modeling, and validation.
What are some popular data analysis techniques?
What are some commonly used data analysis techniques?
Some popular data analysis techniques include descriptive statistics, inferential statistics, regression analysis, time series analysis, data visualization, clustering, classification, and machine learning. These techniques help to analyze and interpret the data based on specific objectives or hypotheses.
What tools are used for data analysis?
What are some commonly used data analysis tools?
There are various tools available for data analysis, such as statistical software like R, Python, SAS, Excel, and SPSS. Visualization tools like Tableau, Power BI, and QlikView are also commonly used to represent the analyzed data in a graphical format. Additionally, database management systems and programming languages are often used for data manipulation and analysis.
What is the role of statistics in data analysis?
How does statistics contribute to data analysis?
Statistics plays a crucial role in data analysis as it provides methods and techniques to summarize and interpret data, determine correlations and relationships, make predictions and inferences, and test hypotheses. It helps in identifying patterns, trends, and anomalies in the data and provides a solid foundation for making statistical inferences.
What are the challenges in data analysis?
What are the common challenges faced in data analysis?
Some common challenges in data analysis include dealing with large and complex data sets, data cleaning and preprocessing, selecting appropriate analysis techniques, ensuring data accuracy and reliability, handling missing data, managing privacy and security concerns, and effectively communicating the findings to stakeholders.
What are some ethical considerations in data analysis?
What ethical considerations are important in data analysis?
In data analysis, it is important to consider ethical aspects such as ensuring data privacy and confidentiality, obtaining appropriate consent for data collection, using unbiased and fair analysis methods, avoiding data manipulation or misinterpretation, and being transparent and accountable in reporting the findings. Ethical considerations help in conducting responsible and trustworthy data analysis.
How can data analysis be applied in different industries?
In which industries can data analysis be applied?
Data analysis can be applied to various industries such as finance, healthcare, marketing, retail, manufacturing, telecommunications, transportation, sports, and many more. It provides valuable insights and helps in optimizing processes, predicting customer behavior, improving decision-making, and enhancing overall business performance.
What are the career opportunities in data analysis?
What career options are available in data analysis?
There are diverse career opportunities in data analysis, including data analyst, data scientist, business analyst, data engineer, statistician, machine learning engineer, data architect, and data consultant. These roles involve working with data, applying analytical techniques, and deriving insights to support decision-making and solve complex problems.