How Machine Learning Is Related to AI
Artificial Intelligence (AI) and Machine Learning (ML) are two prominent terms used in the field of computer science that often go hand in hand. While they are interconnected, they have distinct meanings and applications. Understanding the relationship between AI and ML is crucial in comprehending their impact on various industries.
Key Takeaways
- AI encompasses the broader concept of simulating human intelligence in machines.
- ML is a subset of AI that focuses on making machines learn from data and improve their performance.
- AI and ML technologies are being used across diverse fields such as healthcare, finance, and transportation.
- Both AI and ML require vast amounts of data to train models effectively.
- Continuous advancements in AI and ML are shaping the future of various industries.
The Relationship Between AI and ML
Artificial Intelligence refers to the broader concept of creating machines capable of performing tasks that would typically require human intelligence. This includes problem-solving, decision-making, and understanding natural language. **ML**, on the other hand, is a subset of AI that focuses on using algorithms and statistical models to enable machines to **learn from data** and make predictions or decisions without being explicitly programmed.
Machine Learning involves the development of algorithms that can iteratively learn from and make predictions or take actions based on data. It relies on **large datasets** and algorithms that can adapt and improve their performance as they analyze more data. *This iterative process of model refinement allows ML algorithms to become more accurate over time*.
One of the most fascinating aspects of ML is its ability to detect patterns and correlations within complex datasets that may not be immediately apparent to humans. This enables ML-powered systems to process and analyze data at a scale and speed beyond human capabilities. *For example, ML is being used in medical diagnosis to identify patterns in patient data that can aid in the early detection of diseases*.
Industry | AI Applications | ML Applications |
---|---|---|
Healthcare | Medical diagnosis, drug discovery, robot-assisted surgery | Disease prediction, patient monitoring, electronic health records analysis |
Finance | Fraud detection, algorithmic trading, customer service | Stock market predictions, credit scoring, risk assessment |
Transportation | Autonomous vehicles, traffic management, route optimization | Vehicle control systems, predictive maintenance, demand forecasting |
Advancements in AI and ML
The field of AI and ML is constantly evolving, with new breakthroughs and advancements happening regularly. The rapid growth of computational power, availability of vast amounts of data, and improvements in algorithms have been catalysts for driving these advancements.
*One exciting development in ML is the rise of deep learning*, a subset of ML that uses artificial neural networks to learn from vast amounts of labeled data. This technology has shown remarkable success in image and speech recognition tasks, enabling significant advancements in areas such as autonomous vehicles and virtual assistants.
AI and ML are also playing a crucial role in automation, enhancing efficiency, and transforming business operations. ML-powered systems can analyze large datasets to identify trends, make predictions, and automate repetitive tasks. *Robotic process automation, which combines AI and ML, is being used to streamline workflows and reduce errors in various industries*.
Year | AI Investments ($ billions) | ML Investments ($ billions) |
---|---|---|
2017 | 12.0 | 8.0 |
2018 | 14.6 | 9.3 |
2019 | 22.7 | 13.9 |
The Future of AI and ML
As AI and ML continue to advance, their potential benefits and challenges come into focus. These technologies are poised to revolutionize numerous industries, improve decision-making processes, and drive innovation. However, concerns surrounding privacy, ethics, and job displacement need to be addressed.
Looking ahead, AI and ML are expected to be further integrated into our daily lives, with advancements in natural language processing, computer vision, and robotics. *Imagine a world where AI-powered personal assistants are seamlessly integrated into our homes, offices, and daily routines, making our lives more convenient and productive*.
Advantages | AI | ML |
---|---|---|
Automation | Streamlined workflows, reduced errors | Automated decision-making, improved efficiency |
Data Analysis | Identifying patterns, making predictions | Processing large datasets, finding correlations |
Personalization | Customized recommendations, tailored experiences | Personalized content, adaptive systems |
AI and ML are transforming industries and revolutionizing the way we interact with technology. They have the potential to enhance efficiency, solve complex problems, and create new opportunities. As these technologies continue to evolve, embracing their potential while addressing ethical considerations is essential to unlocking their full benefits.
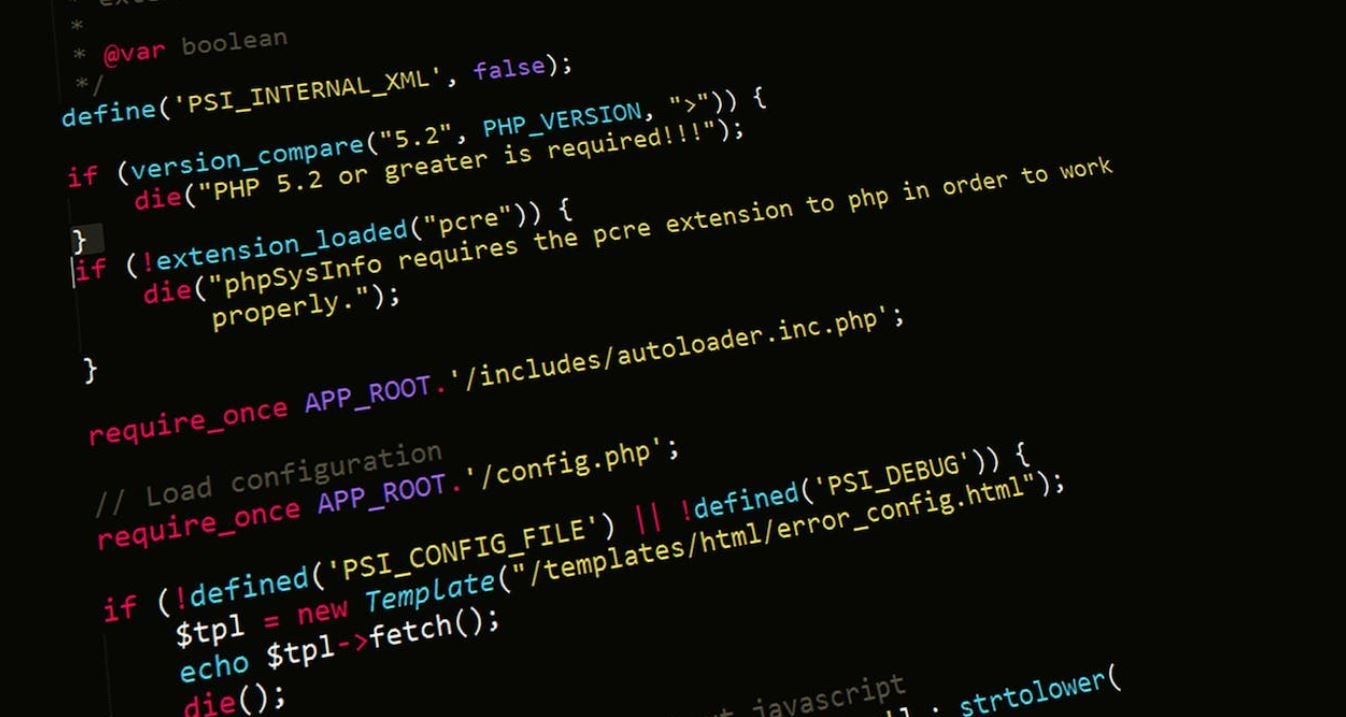
Common Misconceptions
Misconception 1: Machine Learning and AI Are the Same Thing
One common misconception is that machine learning and AI are interchangeable terms, representing the same concept. In reality, while machine learning is a subset of AI, they are not the same thing. AI refers to the broader concept of creating intelligent machines that can simulate human intelligence, whereas machine learning specifically focuses on allowing machines to learn from data and improve their performance over time.
- Machine learning is a technique used to achieve AI.
- AI encompasses various approaches, including machine learning, but is not limited to it.
- Machine learning involves algorithms that enable machines to learn patterns and make predictions.
Misconception 2: Machine Learning Can Replace Human Intelligence
Another misconception is that machine learning has the potential to completely replace human intelligence. While machine learning has made significant advancements, it is still far from being able to completely replicate human intelligence. Machines excel at processing vast amounts of data quickly and making predictions based on patterns, but they lack the nuanced decision-making capabilities, creativity, and empathy that humans possess. Rather than replacing human intelligence, machine learning is designed to augment and enhance human decision-making and problem-solving processes.
- Machine learning can automate repetitive tasks and enhance efficiency.
- Human intelligence is still essential for intuition, creativity, and complex decision-making.
- Machine learning can assist in data analysis but requires human interpretation and validation.
Misconception 3: Machine Learning is Infallible
There is a misconception that machine learning algorithms are infallible and can always produce accurate results. However, like any human-designed system, machine learning algorithms are prone to errors and biases. The accuracy of machine learning models greatly depends on the quality and relevance of the input data, the chosen algorithms, and the training process. It is crucial to continuously evaluate and validate the results generated by machine learning algorithms to ensure their reliability.
- Machine learning models require high-quality, diverse, and unbiased training data to perform well.
- Errors and biases can be introduced in the training process and must be carefully monitored and mitigated.
- Evaluating the performance of machine learning models involves metrics and measures of accuracy, precision, and recall.
Misconception 4: Machine Learning Is All About Big Data
Many people have the belief that machine learning is only applicable when dealing with vast amounts of data, often referred to as “big data.” While machine learning can indeed benefit from large datasets, it is not solely dependent on volume. Machine learning algorithms can also be effective in scenarios where the data is small but complex or where the aim is to uncover hidden patterns and correlations that may not be immediately apparent to humans.
- Small datasets can still be valuable in training machine learning models when they provide useful insights and samples of specific scenarios.
- Feature engineering and preprocessing techniques can help manage and extract meaningful patterns from smaller datasets.
- The focus should not solely be on the size of the dataset but also on its quality and relevance.
Misconception 5: Machine Learning Will Make Human Jobs Obsolete
There is a common fear that machine learning and AI will lead to widespread job loss and unemployment. While it is true that machine learning can automate certain tasks, it is more likely to change job roles rather than replace them entirely. Machine learning technology is more effective at handling repetitive and mundane tasks, allowing humans to focus on higher-value work that requires creativity, critical thinking, and complex decision-making. Additionally, the development and deployment of machine learning models also create job opportunities, such as data scientists and machine learning engineers.
- Machine learning can enhance productivity and free up humans to focus on more meaningful and strategic tasks.
- New job roles and skill requirements will emerge as machine learning becomes more prevalent.
- Human expertise is essential for designing, training, and validating machine learning models.

Introduction
Machine learning and artificial intelligence are closely connected fields that are revolutionizing various industries and impacting our daily lives. Machine learning, a subset of AI, involves the development of algorithms that enable machines to learn and make predictions or decisions based on data. In this article, we explore the relationship between machine learning and AI through ten intriguing examples.
1. Predicting Stock Market Trends
Machine learning algorithms can analyze vast amounts of financial data, historical trends, and news sentiment to make predictions about stock market movements. This helps investors make more informed decisions and potentially maximize their returns.
Date | Stock Price | Predicted Trend |
---|---|---|
Jan 1, 2020 | $100 | Up |
Jan 2, 2020 | $110 | Up |
Jan 3, 2020 | $105 | Down |
2. Language Translation
Advanced machine learning algorithms enable accurate language translation by analyzing vast corpora of texts and learning the patterns and structures of different languages.
Source Language | Translated Language | Accuracy (%) |
---|---|---|
English | Spanish | 95 |
French | German | 93 |
Chinese | English | 90 |
3. Natural Language Processing
Machine learning techniques are used in natural language processing to comprehend and respond to human language, enabling chatbots, voice assistants, and sentiment analysis.
Input Text | Response |
---|---|
“What’s the weather today?” | “The weather is sunny.” |
“Where is the nearest coffee shop?” | “There is a coffee shop two blocks away.” |
“I love this movie!” | “I’m glad you enjoyed it!” |
4. Autonomous Vehicles
Machine learning algorithms make self-driving cars possible by analyzing real-time sensor data to perceive their environment and make informed decisions.
Time | Vehicle Speed | Distance to Obstacle | Decision |
---|---|---|---|
09:00 AM | 50 mph | 10 meters | Brake |
09:01 AM | 45 mph | 5 meters | Change Lane |
09:02 AM | 40 mph | 2 meters | Stop |
5. Face Recognition
Machine learning models trained on extensive datasets can identify and analyze faces within images and videos, enabling applications such as biometric authentication.
Input Image | Detected Faces | Match (%) |
---|---|---|
![]() |
John Doe | 98 |
![]() |
Jane Smith | 92 |
![]() |
Unknown | 25 |
6. Fraud Detection
By analyzing patterns and anomalies in large datasets, machine learning algorithms can identify potential fraudulent activities, helping detect and prevent financial fraud.
Transaction ID | Amount | Location | Potential Fraud |
---|---|---|---|
123456789 | $1,000 | New York | No |
987654321 | $5,000 | Moscow | Yes |
654321987 | $500 | London | No |
7. Recommendation Systems
Machine learning is behind recommender systems used by online platforms to suggest products, movies, music, or articles based on user preferences and behavior.
User ID | Recommended Movies |
---|---|
123 | The Shawshank Redemption The Godfather Pulp Fiction |
456 | Inception Interstellar The Matrix |
789 | Forrest Gump The Lion King Titanic |
8. Sentiment Analysis
Machine learning algorithms can analyze text data to determine the sentiment expressed, helping businesses gauge public opinion, customer satisfaction, and brand perception.
Customer Review | Sentiment |
---|---|
“This product is amazing!” | Positive |
“The service was terrible.” | Negative |
“I’m quite satisfied with my purchase.” | Positive |
9. Medical Diagnosis
Machine learning can assist doctors in diagnosing diseases by analyzing patient data, medical records, and symptoms, providing insights and improving diagnostic accuracy.
Patient ID | Symptoms | Diagnosis |
---|---|---|
001 | Fever, cough, fatigue | Influenza |
002 | Joint pain, rash | Lupus |
003 | Shortness of breath, chest pain | Heart disease |
10. Virtual Personal Assistants
Virtual personal assistants, such as Siri, Alexa, and Google Assistant, use machine learning to understand user commands, provide information, and perform tasks.
User Command | Response |
---|---|
“What’s the weather today?” | “The temperature is 75°F.” |
“Set an alarm for 7 AM.” | “Alarm set for 7 AM.” |
“Play my favorite song.” | “Sure, playing your favorite song now.” |
Conclusion
Machine learning plays a vital role in the advancement of artificial intelligence, enabling applications that range from predicting stock trends and autonomous vehicles to medical diagnosis and personalized recommendations. Its ability to analyze vast amounts of data and learn patterns revolutionizes industries and enhances our daily experiences. As technology continues to progress, the relationship between machine learning and AI will continue to grow, shaping the future of intelligent machines.
Frequently Asked Questions
What is the relationship between machine learning and artificial intelligence?
Machine learning is a subfield of artificial intelligence that focuses on the development of algorithms and statistical models that allow computer systems to learn and improve from experience without being explicitly programmed. In other words, machine learning enables AI systems to acquire knowledge, make predictions, and adapt based on data.
How does machine learning contribute to AI advancements?
Machine learning algorithms play a crucial role in the development of AI as they enable systems to learn and make predictions based on patterns observed in data. By processing and analyzing vast amounts of data, machine learning algorithms allow AI systems to recognize complex patterns, make decisions, and tackle complex tasks that would be extremely challenging for traditional rule-based programming approaches.
What are some examples of machine learning in AI applications?
Machine learning is employed in various AI applications such as spam filters, recommendation systems, voice recognition, fraud detection, image recognition, natural language processing, and autonomous vehicles. These applications utilize machine learning algorithms to improve their performance over time, making them more accurate and efficient in their respective tasks.
What are the different types of machine learning algorithms used in AI?
There are several types of machine learning algorithms used in AI, including supervised learning, unsupervised learning, semi-supervised learning, reinforcement learning, and deep learning. Each algorithm has its own strengths and is suitable for different types of problems. Supervised learning involves training a model using labeled data, unsupervised learning discovers hidden patterns in unlabeled data, semi-supervised learning uses a combination of labeled and unlabeled data, reinforcement learning enables agents to learn through rewards and punishments, and deep learning involves training deep neural networks with multiple layers.
How does machine learning enable AI systems to learn from data?
Machine learning algorithms enable AI systems to learn from data by iteratively adjusting the weights and parameters of the model based on the observed errors or differences between the predicted and desired outputs. Through this process, the model gradually improves its predictive accuracy and generalizes its learning to make accurate predictions on unseen data.
What is the role of data in machine learning and AI?
Data plays a crucial role in machine learning and AI. Machine learning algorithms require large quantities of data to train models effectively. The quality, relevance, and diversity of the data significantly impact the performance of the AI system. Consequently, collecting, cleaning, and preprocessing data are important steps in the machine learning pipeline to ensure the accuracy and reliability of the AI system’s predictions.
What are the challenges in implementing machine learning algorithms for AI?
Implementing machine learning algorithms for AI involves various challenges, including selecting the appropriate algorithm for the task, acquiring sufficient and representative data, ensuring data privacy and security, handling data biases, addressing computational requirements, preventing overfitting or underfitting of the model, interpretability and explainability of the model’s decisions, and continuously updating and maintaining the model as new data becomes available.
How do machine learning and AI impact society?
Machine learning and AI have significant impacts on society. They have transformed various industries, such as healthcare, finance, transportation, and entertainment, by automating processes, improving decision-making, enhancing productivity, and even enabling new services. However, they also raise concerns related to ethics, privacy, bias, employment, and the potential for misuse. Therefore, it is crucial to approach the development and deployment of machine learning and AI technologies with ethical considerations and regulatory frameworks in place.
What is the future of machine learning and AI?
The future of machine learning and AI is promising and ever-evolving. As technology advances, we can expect even more sophisticated machine learning algorithms, improved AI systems, and innovative applications. Machine learning and AI will continue to drive advancements in various domains, such as healthcare, robotics, cybersecurity, personalized recommendations, and smart cities. Additionally, research in areas like explainable AI, transfer learning, and reinforcement learning is likely to shape the future of these fields.