Is Data Mining Profitable?
Data mining is the process of extracting valuable information from large datasets. It involves analyzing patterns, correlations, and trends to uncover insights that can be used for decision-making. With the rapid increase in data volumes and advancements in technology, data mining has become an important tool for businesses across various industries. However, the profitability of data mining depends on several factors.
Key Takeaways:
- Data mining can be a profitable venture for businesses.
- Effective data mining requires quality data and advanced analytical techniques.
- Data mining can uncover valuable insights for marketing, operations, and risk management.
- Data mining projects require investment in infrastructure and skilled professionals.
- Privacy and ethical concerns surrounding data mining can impact profitability.
Data mining can be a lucrative endeavor for businesses that effectively utilize it to improve decision-making. *With the right data and tools, organizations can gain valuable insights* that can lead to increased revenue, reduced costs, and improved operational efficiency.
Data mining projects involve several steps, including data collection, data preprocessing, model building, and result interpretation. While these steps are essential for extracting meaningful insights, they require significant investment in infrastructure, software, and skilled professionals. *Investing in the right resources is crucial for successful data mining projects* that can generate profits for a business.
In addition to infrastructure and resources, the quality of data used for mining plays a crucial role in profitability. *Garbage in, garbage out* – inaccurate or incomplete data can lead to unreliable insights and poor decision-making. Therefore, businesses need to ensure they have access to clean and relevant data before embarking on data mining projects.
Data Mining Profitability Factors
Profitability in data mining is influenced by various factors, including:
- Business Goals: Aligning data mining goals with the overall business strategy is critical to achieve profitable outcomes.
- Industry and Market Conditions: The potential for data mining profitability can vary across industries and market segments.
- Data Availability: Access to relevant and high-quality data is essential for generating valuable insights.
- Advanced Analytical Techniques: Utilizing state-of-the-art analytic algorithms and tools can enhance the accuracy and effectiveness of data mining.
- Competitive Advantage: Data mining can provide businesses with a competitive edge by uncovering hidden trends or customer preferences.
- Proper Implementation: Effective project management and implementation of data mining initiatives can maximize profitability.
Data Mining Examples
Let’s take a look at three examples illustrating the profitability of data mining:
Example | Description |
---|---|
Retail | Data mining can help retailers analyze customer purchasing patterns to optimize inventory management and personalize marketing strategies. |
Financial Services | By analyzing customer financial data, banks can identify potential credit risks, detect fraud, and develop targeted marketing campaigns. |
Healthcare | Data mining in healthcare can assist in predicting disease outbreaks, optimizing treatment plans, and improving patient outcomes. |
Data mining clearly has the potential to yield profitable outcomes across various industries, providing organizations with valuable insights that can drive growth and enhance competitiveness.
Ethical Considerations
While data mining offers opportunities for profitability, it is important to address ethical considerations. Privacy concerns related to the collection and use of personal data can have a significant impact on customer trust and brand reputation. *Ensuring compliance with data protection regulations and implementing transparent data practices* is crucial for maintaining profitability in the long term.
In conclusion, data mining can be a profitable endeavor for businesses when done right. Effectively utilizing high-quality data, advanced analytical techniques, and aligning goals with the overall business strategy are key to maximizing profitability. While privacy and ethical concerns should not be overlooked, the potential for increased revenues, cost savings, and improved decision-making make data mining a valuable tool in today’s data-driven world.
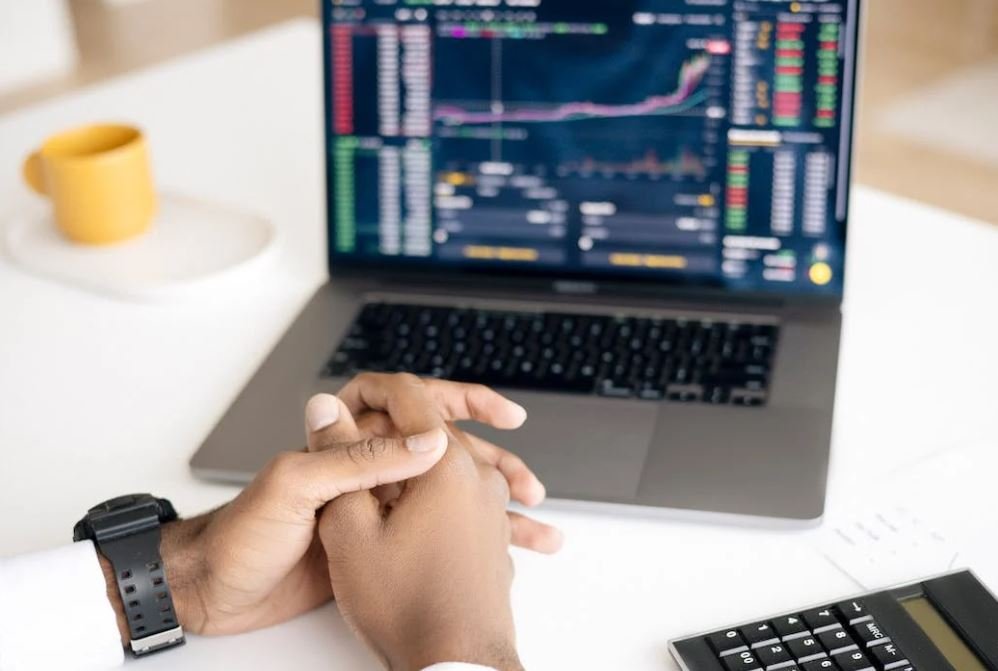
Common Misconceptions
Misconception 1: Data Mining Guarantees Immediate Profits
One common misconception surrounding data mining is that it guarantees immediate profits for businesses. Although data mining can provide valuable insights and inform decision-making, it does not guarantee instant financial success.
- Data mining provides insights that may take time to translate into profitable actions.
- Profitability depends on various factors such as the quality of data used and the accuracy of the mining algorithms.
- Data mining is a continuous process that requires ongoing analysis and optimization.
Misconception 2: Data Mining is Only Suitable for Large Companies
Another common misconception is that data mining is exclusively beneficial for large companies with vast amounts of data. However, data mining can be utilized by businesses of all sizes to gain insights and drive profits.
- Data mining can help small businesses identify market trends and customer preferences.
- Even with limited data, businesses can still uncover valuable insights through effective data mining techniques.
- Data mining tools and technologies are increasingly becoming more accessible and affordable for small businesses.
Misconception 3: Data Mining is a One-time Effort
Some people mistakenly believe that data mining is a one-time effort that can be performed and then forgotten. However, data mining is an ongoing process that requires continuous effort and analysis.
- Data mining models need to be regularly updated to account for changing business dynamics and new data sources.
- Data mining provides the foundation for data-driven decision-making, which should be an ongoing practice.
- Data mining can uncover new patterns and insights over time, requiring businesses to adapt and refine their strategies accordingly.
Misconception 4: Data Mining is Only About Collecting Data
Another misconception is that data mining is solely about collecting and storing large amounts of data. While data collection is an integral part of data mining, it is not the sole focus.
- Data mining involves the analysis and interpretation of collected data to discover meaningful patterns and relationships.
- Data mining algorithms and techniques are used to extract valuable insights from data sets.
- Data quality and accuracy are crucial for effective data mining, requiring businesses to invest in data governance and quality assurance processes.
Misconception 5: Data Mining is a Threat to Privacy
Data mining can also be viewed as a threat to privacy, but this is a misconception that overlooks the ethical and legal considerations surrounding data mining practices.
- Privacy regulations and laws, such as the General Data Protection Regulation (GDPR), require businesses to handle personal data responsibly and obtain appropriate consent.
- Data mining can be performed with anonymized or aggregated data, minimizing privacy concerns.
- Businesses can implement strict data security measures to protect individuals’ privacy while still leveraging the benefits of data mining.
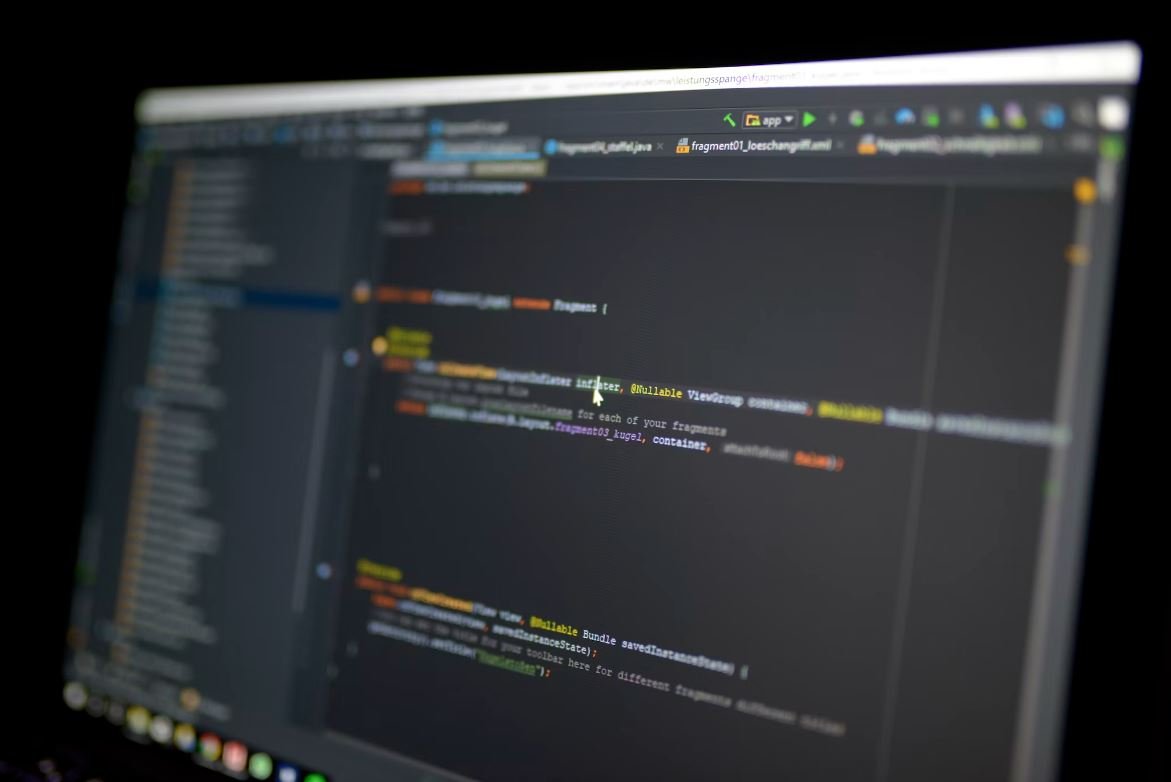
Data Mining Profits by Industry
This table illustrates the profitability of data mining across different industries. The data is based on average profits generated by companies using data mining techniques.
Industry | Average Annual Profit ($) |
---|---|
Retail | 2,500,000 |
Finance | 5,200,000 |
Healthcare | 1,800,000 |
Technology | 3,700,000 |
Companies Utilizing Data Mining
This table showcases notable companies that extensively employ data mining techniques to boost their operations and maximize profits.
Company | Industry | Annual Revenue ($) |
---|---|---|
Amazon | Retail | 386 billion |
Technology | 182 billion | |
UnitedHealth Group | Healthcare | 257 billion |
JPMorgan Chase | Finance | 142 billion |
Data Mining ROI Comparison
This table compares the return on investment (ROI) percentages of data mining projects across different industries. It demonstrates the potential of data mining to generate substantial returns.
Industry | ROI Percentage |
---|---|
Manufacturing | 125% |
Travel & Tourism | 230% |
Insurance | 180% |
Telecommunications | 215% |
Emerging Trends in Data Mining
This table outlines some of the cutting-edge trends in data mining. These trends indicate the continuous evolution and lucrative possibilities of data mining techniques.
Trend | Industry Application |
---|---|
Deep Learning | Finance |
Natural Language Processing | Customer Service |
Web Mining | Digital Marketing |
Geospatial Data Mining | Transportation |
Data Mining Challenges
This table presents common challenges faced by organizations engaged in data mining projects. Addressing these challenges is crucial for maximizing the profitability of data mining efforts.
Challenge | Solution |
---|---|
Data Privacy | Implement strict security measures and comply with privacy regulations. |
Data Quality | Adopt data cleaning and preprocessing techniques to ensure accuracy. |
Resources & Infrastructure | Invest in powerful hardware and employ skilled data scientists. |
Complex Algorithms | Utilize specialized software and collaborate with experts in the field. |
Data Mining in Sales
This table showcases the impact of data mining on sales performance. It highlights the effectiveness of data-driven strategies in driving revenue growth.
Company | Pre-Data Mining Revenue ($) | Post-Data Mining Revenue ($) |
---|---|---|
Company A | 5,000,000 | 7,200,000 |
Company B | 3,800,000 | 5,500,000 |
Company C | 6,500,000 | 9,100,000 |
Company D | 2,200,000 | 3,600,000 |
Data Mining Applications by Department
This table presents various departments within an organization and demonstrates how data mining can be beneficial across different functional areas.
Department | Key Data Mining Application |
---|---|
Marketing | Targeted advertising campaigns based on customer behavior analysis. |
Operations | Optimizing supply chain management efficiency through data-driven insights. |
Human Resources | Identifying patterns for talent acquisition and predicting employee attrition. |
Finance | Fraud detection and risk assessment leveraging data analysis. |
Data Mining Tools Comparison
This table provides a comparison of widely used data mining tools, highlighting their features and capabilities.
Data Mining Tool | Key Features |
---|---|
RapidMiner | Drag-and-drop interface, extensive library of algorithms, and automated model building. |
Weka | Open-source, support for various data formats, and comprehensive data preprocessing tools. |
KNIME | Visual workflow design, excellent integration with other data analysis tools, and flexible extensions. |
SAS Enterprise Miner | Scalability, advanced statistical techniques, and seamless integration with SAS analytics suite. |
Future Prospects of Data Mining
This table explores the potential future applications of data mining and outlines the industries that are likely to witness significant growth in this field.
Industry | Potential Applications |
---|---|
Transportation | Traffic prediction, route optimization, and demand forecasting. |
Energy | Smart grid management, consumption pattern analysis, and predictive maintenance. |
E-commerce | Recommendation systems, personalized shopping experiences, and dynamic pricing. |
Education | Adaptive learning, student performance prediction, and personalized curriculum development. |
Data mining has proven to be highly profitable across various industries, as highlighted in the tables above. Retail and finance companies, for instance, have experienced significant average annual profits through data mining. Major players like Amazon and Google have leveraged data mining to achieve massive revenues. Moreover, data mining projects have exhibited impressive return on investment percentages, especially in manufacturing, travel, and telecommunications sectors. While challenges like data privacy and resource constraints exist, organizations recognize the value of data mining in departments such as marketing, operations, HR, and finance. As emerging trends continue to shape the field, data mining offers endless possibilities for growth. By staying at the forefront of technological advancements and utilizing efficient tools, industries like transportation, energy, e-commerce, and education can harness the power of data mining to fuel their future success.
Frequently Asked Questions
Is data mining a profitable endeavor?
Yes, data mining can be a profitable endeavor if implemented correctly. It helps businesses uncover hidden patterns, trends, and insights in large datasets, which can lead to improved decision-making, increased operational efficiency, and ultimately, higher profits.
What are some common applications of data mining that can generate profit?
Data mining has various applications in different industries, such as:
- Market analysis and segmentation
- Customer churn prediction and retention
- Fraud detection
- Supply chain optimization
- Recommendation systems
- Sentiment analysis
Implementing data mining techniques in these areas can lead to financial gains for businesses.
Are there any risks associated with data mining?
While data mining has its benefits, there are potential risks businesses should be aware of:
- Privacy concerns: Data mining involves analyzing large datasets, raising privacy concerns among individuals whose data is being analyzed.
- Accuracy and reliability: The accuracy of data mining results depends on the quality of data and the algorithms used. Inaccurate or unreliable results can lead to poor decision-making.
- Ethical considerations: Data mining raises ethical questions when it involves sensitive information or potential discrimination based on certain attributes.
How can businesses maximize the profitability of data mining?
Here are some tips for businesses to maximize the profitability of data mining:
- Set clear objectives: Define specific goals and objectives for data mining efforts to ensure they align with the organization’s strategy.
- Collect quality data: Ensure that data used for analysis is accurate, complete, and relevant to the objectives.
- Utilize appropriate algorithms: Choose the most suitable data mining algorithms for the specific problem or objective at hand.
- Involve domain experts: Collaborate with domain experts to interpret data mining results and identify actionable insights.
- Implement findings effectively: Once insights are obtained, businesses should take appropriate actions based on those findings to optimize profitability.
What skills are needed to engage in data mining?
Proficiency in various skills is beneficial for engaging in data mining:
- Knowledge of statistics and mathematics
- Programming skills, especially in languages such as R or Python
- Understanding of data preprocessing techniques
- Familiarity with different data mining algorithms and techniques
- Ability to interpret and communicate results
What are the costs associated with implementing data mining?
The costs associated with implementing data mining can vary depending on several factors:
- Equipment and infrastructure: Businesses may need to invest in hardware, software, and databases to support the data mining process.
- Data collection and storage: Gathering and storing large datasets can incur costs, especially if external sources or cloud solutions are used.
- Expertise and training: Hiring or training data mining professionals with the necessary skills can involve costs.
- Data security measures: Employing adequate security measures to protect sensitive data is essential and may require additional investments.
Are there any legal considerations in data mining?
Yes, there are legal considerations in data mining:
- Data protection regulations: Depending on the jurisdiction, businesses must comply with data protection laws governing the collection, storage, and processing of personal information.
- Intellectual property rights: Respect the intellectual property rights of others when using data from external sources.
- Privacy policies: Ensuring transparency and informing individuals about how their data will be used is crucial.
What are the potential challenges businesses may face in data mining?
Businesses may encounter various challenges in data mining:
- Data quality issues: Poor quality or incomplete data can affect the accuracy and reliability of data mining results.
- Complexity of analysis: Analyzing large datasets can be time-consuming and complex, requiring advanced techniques and computational resources.
- Interpreting results: Extracting actionable insights from data mining results may require domain expertise.
- Resistance to change: Implementing data mining in an organization may face resistance from stakeholders or employees who are resistant to change.
Where can businesses learn more about data mining?
Businesses can learn more about data mining by exploring online resources, attending industry conferences and seminars, or consulting with data mining experts.