Machine Learning and Data Science
In today’s data-driven world, machine learning and data science have become indispensable tools for businesses and organizations. By extracting valuable insights from vast amounts of data, these technologies can help companies make more informed decisions and gain a competitive edge. In this article, we will explore the key concepts of machine learning and data science, their applications, and their impact on various industries.
Key Takeaways:
- Machine learning and data science are crucial for businesses in leveraging data to gain insights and make informed decisions.
- These technologies have diverse applications across multiple industries, including finance, healthcare, and marketing.
- Data science involves the use of statistical techniques and algorithms to extract meaningful insights from data.
- Machine learning is a subset of artificial intelligence (AI) that focuses on creating algorithms that can learn from and make predictions or decisions based on data.
Understanding Machine Learning
**Machine learning** is a field of study that enables computers to learn and make predictions or decisions without being explicitly programmed. It focuses on developing algorithms that can automatically learn from patterns in data and improve their performance over time. This technology is driven by vast amounts of data, powerful computational resources, and sophisticated algorithms.
**One interesting aspect** of machine learning is its ability to handle complex and large-scale data sets. Traditional statistical methods struggle with analyzing such data, but machine learning algorithms can efficiently process and model this information to extract meaningful insights.
There are various **types of machine learning**, including supervised learning, unsupervised learning, and reinforcement learning. Supervised learning involves training a model using labeled data to make predictions or classify new instances. Unsupervised learning, on the other hand, deals with unlabeled data and aims to discover hidden patterns or structures in the dataset. Reinforcement learning is a technique where an agent learns to take actions in an environment to maximize its rewards.
Data Science and its Applications
**Data science** combines various aspects of mathematics, statistics, and computer science to extract insights and knowledge from structured and unstructured data. It involves the use of different tools and techniques to collect, clean, analyze, and interpret data. The goal is to uncover patterns and gain actionable insights that can drive decision-making processes.
Data science has a wide range of **applications** across industries. In finance, it can be used to detect fraudulent transactions, predict stock market trends, or assess credit risk. In healthcare, data science can help researchers identify potential drug candidates, develop personalized medicine options, or analyze patient health records. In marketing, it can be used to segment customers, predict demand, or optimize advertising campaigns.
An **interesting aspect** of data science is its increasing adoption in the field of sports. Teams and coaches utilize data science techniques to gain insights into players’ performance, make informed decisions during games, and develop winning strategies.
The Impact on Industries
Machine learning and data science have **revolutionized various industries**. By leveraging these technologies, organizations can optimize their operations, improve customer experience, and drive innovation. Let’s take a look at some examples of the impact:
- In finance, machine learning algorithms can analyze vast amounts of financial data to detect fraud, predict market trends, and make investment recommendations.
- In healthcare, data science can help with disease diagnosis and treatment planning, as well as analyze patient data to identify potential health risks.
- In marketing, machine learning enables personalized recommendations, targeted advertisements, and effective customer segmentation.
- In transportation, machine learning algorithms can optimize route planning, predict maintenance needs, and improve traffic management.
- In manufacturing, data science can increase efficiency, minimize defects, and optimize supply chain management.
Tables with Interesting Insights
Industry | Application |
---|---|
Finance | Market prediction |
Healthcare | Disease diagnosis |
Marketing | Customer segmentation |
An interesting piece of information from the table above is that **machine learning is used extensively in marketing to segment customers**, helping businesses target their audience more effectively.
Machine Learning Technique | Application |
---|---|
Supervised Learning | Image recognition |
Unsupervised Learning | Market basket analysis |
Reinforcement Learning | Autonomous driving |
From the table above, we can see that **supervised learning is commonly used in image recognition tasks** to classify and identify objects in images.
Data Science Technique | Application |
---|---|
Regression Analysis | Stock market prediction |
Natural Language Processing | Text sentiment analysis |
Cluster Analysis | Customer segmentation |
As shown in the table above, **data science techniques such as regression analysis are frequently used to predict stock market trends** and assist in investment decision-making.
The combination of machine learning and data science has opened up immense possibilities for businesses across industries. By harnessing the power of these technologies, organizations can gain a competitive advantage, drive innovation, and make data-driven decisions. As we continue to generate vast amounts of data, the role of machine learning and data science will only become more prevalent in shaping our future.
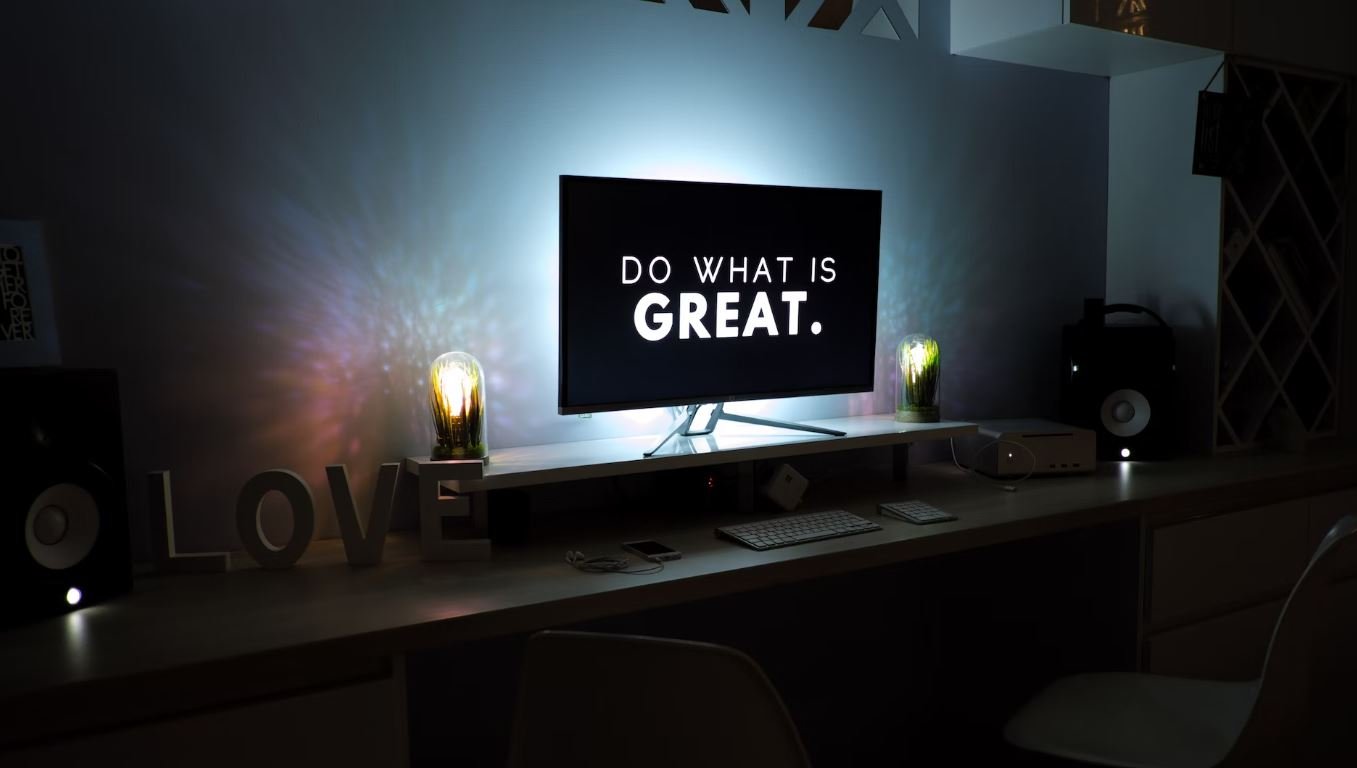
Common Misconceptions
Machine Learning
Machine Learning is often misunderstood by people who do not have a technical background. Here are some common misconceptions:
- Machine Learning is a magical solution that can solve all problems.
- Machine Learning requires a large amount of data to be effective.
- Machine Learning can replace human intelligence entirely.
Data Science
Data Science is a rapidly growing field that is sometimes misunderstood. Here are a few misconceptions:
- Data Science is all about coding and programming.
- Data Science is only applicable to businesses and industries.
- Data Science is only concerned with analyzing numerical data.
Machine Learning vs Data Science
There is often confusion between Machine Learning and Data Science, leading to misconceptions:
- Machine Learning is synonymous with Data Science.
- Data Science is only a small part of Machine Learning.
- Machine Learning and Data Science are two separate disciplines with distinct goals.
Real-world Applications
People often underestimate the wide range of applications for both Machine Learning and Data Science. Here are some common misconceptions:
- Machine Learning and Data Science are only used by high-tech industries.
- Machine Learning and Data Science have limited real-world applications.
- Machine Learning and Data Science are only useful for predicting outcomes.
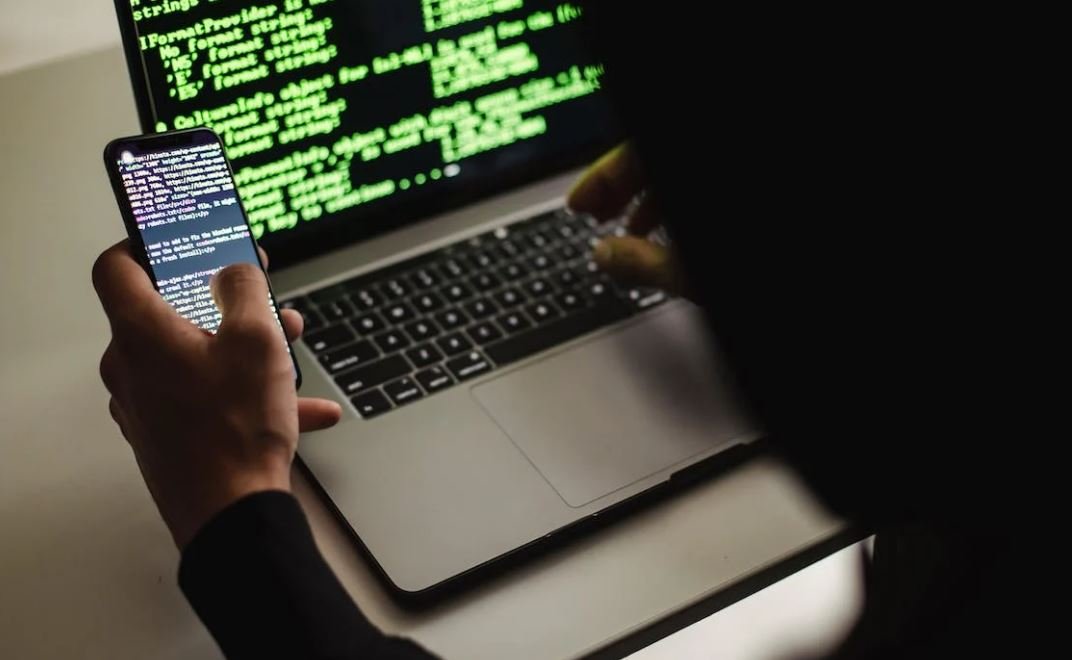
Machine Learning and Data Science
Machine learning and data science have revolutionized various industries by enabling organizations to extract valuable insights and make data-driven decisions. This article explores 10 fascinating aspects of machine learning and data science that have reshaped our world.
1. Growth of Machine Learning
Machine learning has experienced exponential growth in recent years. In 2020, the global machine learning market was valued at $8.43 billion, and it is projected to reach $117.19 billion by 2027. This immense growth is fueled by the increasing adoption of machine learning solutions across different sectors.
2. Employment Opportunities
The rise of machine learning and data science has created abundant job opportunities. It is estimated that there will be over 11.5 million job openings in machine learning and data science by 2026. These roles span various domains, including healthcare, finance, marketing, and cybersecurity.
3. Big Data Revolution
The big data revolution has played a crucial role in advancing machine learning and data science. As of 2020, the digital universe is estimated to consist of 44 zettabytes of data, and this volume is expected to reach 175 zettabytes by 2025. Machine learning algorithms thrive on large datasets, allowing organizations to derive valuable insights.
4. Applications in Healthcare
Machine learning and data science have significantly impacted the healthcare industry. For instance, predictive analytics can assist in early diagnosis, personalized treatment plans, and disease prevention. Moreover, machine learning algorithms are employed in drug discovery, genomics research, and medical image analysis.
5. Fraud Detection
Financial institutions have found great value in machine learning for fraud detection. Machine learning models can analyze vast amounts of data to identify anomalous patterns and flag potential fraudulent activities. This technology has saved companies billions of dollars by preventing financial losses.
6. Natural Language Processing
Natural language processing (NLP) enables machines to understand and interact with human language. This technology powers virtual assistants, chatbots, and language translation services. NLP algorithms can analyze vast amounts of text data to derive sentiment, intent, and extract key information.
7. Autonomous Vehicles
Machine learning is driving the development of autonomous vehicles. Advanced algorithms enable vehicles to perceive and navigate the world, making decisions in real-time. With machine learning, autonomous vehicles can enhance road safety, improve traffic flow, and reduce environmental impacts.
8. Financial Market Predictions
Data science techniques, such as time series analysis and predictive modeling, have improved financial market predictions. Machine learning algorithms can analyze historical data, market trends, and news sentiment to forecast stock prices and identify investment opportunities.
9. Personalized Recommendations
Machine learning has transformed the way businesses provide personalized recommendations to users. By analyzing user preferences and behavior, recommendation systems can suggest articles, products, movies, and music tailored to individual interests. This leads to improved user satisfaction and increased conversions.
10. Environmental Impact
Machine learning and data science contribute to sustainability efforts by optimizing energy consumption, reducing waste, and addressing environmental challenges. These technologies can optimize water usage, improve air quality monitoring, and enhance renewable energy production.
Machine learning and data science have revolutionized industries, fueled job growth, and empowered organizations to make data-driven decisions. With endless possibilities and future advancements, machine learning will continue to shape our world, improving efficiency, accuracy, and our overall quality of life.
Frequently Asked Questions
What is Machine Learning?
What is Machine Learning?
What is Data Science?
What is Data Science?
How does Machine Learning relate to Data Science?
How does Machine Learning relate to Data Science?
What are the key steps involved in a typical Machine Learning project?
What are the key steps involved in a typical Machine Learning project?
What are some common Machine Learning algorithms?
What are some common Machine Learning algorithms?
What is the role of data in Machine Learning?
What is the role of data in Machine Learning?
What is the importance of feature selection in Machine Learning?
What is the importance of feature selection in Machine Learning?
What is the difference between supervised and unsupervised learning?
What is the difference between supervised and unsupervised learning?
What are some common challenges in implementing Machine Learning projects?
What are some common challenges in implementing Machine Learning projects?
What are the ethical considerations in Machine Learning and Data Science?
What are the ethical considerations in Machine Learning and Data Science?