Machine Learning and Finance
Machine learning (ML) has revolutionized numerous industries, and finance is no exception. With its ability to analyze massive amounts of data and make predictions, ML has become a powerful tool for financial institutions and investors alike.
Key Takeaways
- Machine learning technology is transforming the financial industry.
- ML algorithms enable the analysis of complex financial data and help predict market trends.
- Automated trading systems driven by ML algorithms have gained popularity.
- ML can assist in risk management and fraud detection.
- Despite its benefits, ML also presents challenges in terms of data privacy and ethical considerations.
One of the primary ways machine learning is utilized in finance is through the analysis of vast amounts of financial data. **ML algorithms** can quickly and accurately analyze **historical financial data**, identify patterns, and predict future market trends. This enables financial institutions and investors to make more informed decisions, maximize profits, and minimize risks. *By leveraging ML algorithms, financial professionals can gain a competitive edge in the market*.
Automated trading systems that use ML algorithms have become increasingly popular. These systems can automatically execute trades based on predefined rules and indicators. **ML-powered trading algorithms** can identify profitable opportunities in real-time, react faster than human traders, and eliminate emotional biases. *This automation has transformed the financial industry by improving efficiency and reducing human errors*.
ML also plays a vital role in risk management. By analyzing historical financial data, ML algorithms can identify potential risks and patterns associated with different investments. **Risk assessment models** built with machine learning techniques can provide valuable insights for managing portfolios and minimizing potential losses. *This helps financial institutions and investors make better-informed decisions regarding risk exposure*
Machine Learning and Fraud Detection
Fraud detection is another area where machine learning has made significant contributions. Financial institutions face constant threats from fraudulent activities, and ML algorithms are instrumental in tackling this issue. By analyzing patterns and anomalies in transaction data, **ML models** can detect fraudulent activities and flag suspicious transactions. *This has reduced losses due to fraud and enhanced security measures across the financial sector*.
Year | Losses Due to Fraud (in billions) |
---|---|
2017 | 67.2 |
2018 | 70.2 |
2019 | 71.4 |
Despite the numerous benefits of machine learning in finance, it also presents challenges. **Data privacy** is a significant concern, as ML models require access to vast amounts of sensitive financial information. Striking a balance between data access and privacy regulations is crucial to ensure consumer trust. *Financial institutions must prioritize data protection and implement robust security measures to address this challenge*
Ethical considerations also arise when using machine learning in finance. Biases within the training data can lead to biased predictions, potentially perpetuating discriminatory practices. Transparency and regular monitoring of ML models are essential to identify and address biases. *Developing ethical guidelines and frameworks can help ensure ML is deployed responsibly in the financial sector*
Country | % of Financial Institutions Using ML |
---|---|
United States | 54% |
United Kingdom | 41% |
China | 38% |
The Future of Machine Learning in Finance
Machine learning continues to advance rapidly, and its impact on the financial industry will only continue to grow. As more data becomes available and ML algorithms become more sophisticated, their applications in finance will expand. In the future, we can expect to see further development in areas such as personalized financial advice, fraud prevention, and improved risk management strategies. *Machine learning has the potential to reshape the way financial services are delivered and improve outcomes for both institutions and individuals*.
- Improved risk management and fraud detection capabilities
- Personalized financial advice and investment recommendations
- Automated decision-making processes
ML Application | Estimated Market Value (by 2025) |
---|---|
Automated Trading | $25 billion |
Risk Management | $10 billion |
Fraud Detection | $5 billion |
Machine learning has become an integral part of the finance industry, transforming the way financial professionals make decisions and manage risks. With its ability to analyze data, predict trends, and detect fraud, ML has proven its value in increasing efficiency and improving outcomes for financial institutions and investors. As technology continues to advance, machine learning will undoubtedly play a crucial role in shaping the future of finance.
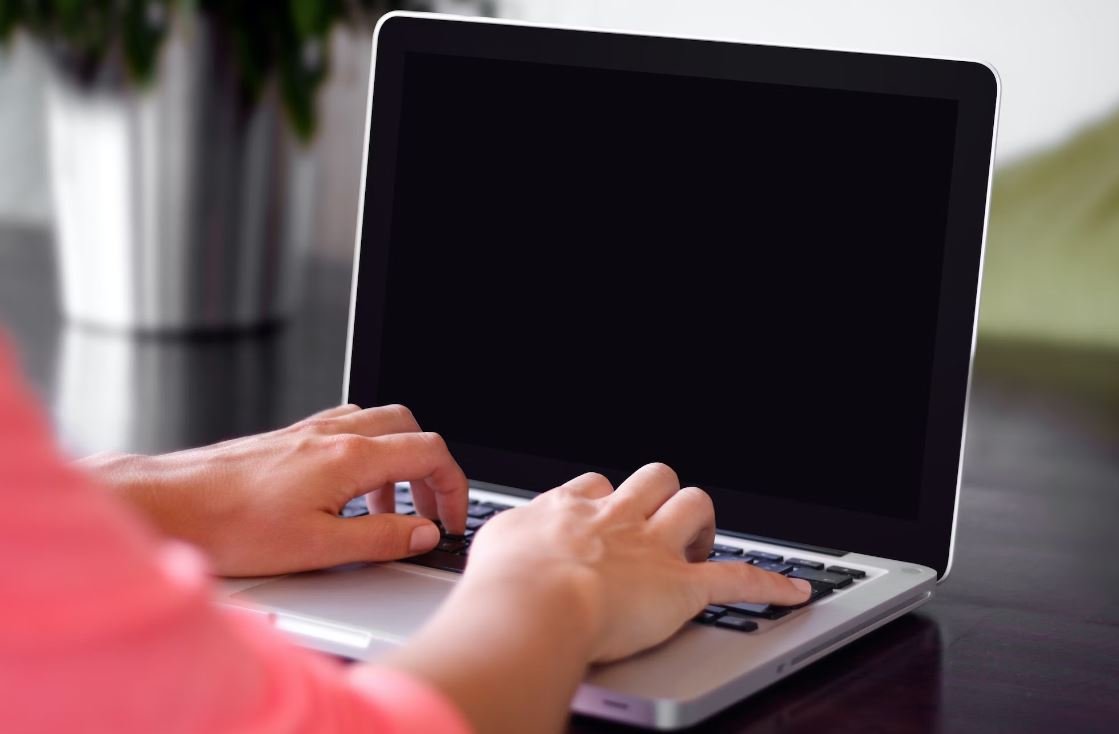
Common Misconceptions
Machine Learning and Finance
One common misconception about machine learning in finance is that it can completely replace human analysts and traders. While machine learning algorithms can automate certain tasks and provide valuable insights, they are not capable of fully replacing the expertise and intuition of human professionals who can interpret complex market dynamics and consider external factors that machines may overlook.
- Machine learning complements human expertise rather than replacing it
- Human analysts bring subjective judgement and contextual knowledge
- Machines lack emotional intelligence and can’t adapt to unexpected events
Another misconception is that machine learning algorithms always produce accurate predictions in financial markets. While these algorithms can process large amounts of data and identify patterns, there is always a degree of uncertainty and risk involved in financial markets. Machine learning models are based on historical data, and future market conditions may differ significantly. It’s crucial to assess the accuracy and reliability of these models and consider them as one of many tools in the decision-making process.
- Accuracy of machine learning predictions can vary
- Models need to be regularly updated to adapt to changing market conditions
- Human validation and critical analysis are important for interpreting the insights gained
Some people believe that machine learning can remove all biases from financial decision-making. However, machine learning algorithms are not inherently unbiased and can be influenced by biased or incomplete training data. If the training data contains biases, the algorithm may amplify or perpetuate these biases in its predictions or investment recommendations. It is essential to carefully curate and review the data used for training these models to minimize bias and ensure fairness.
- Machine learning models could inherit biases from historical data
- Data quality and diversity are crucial in training reliable models
- Human involvement is necessary to identify and mitigate bias in machine learning systems
Another misconception is that machine learning algorithms can predict future economic events accurately. While these algorithms can provide insights based on historical patterns, they cannot predict exceptional events or unforeseen circumstances that may significantly impact the economy and financial markets. Market dynamics are influenced by a wide range of factors, including geopolitical events, government policies, and natural disasters, which are challenging to anticipate accurately using machine learning models.
- Machine learning models are limited to capturing historical patterns
- Unexpected events can disrupt predicted market behavior
- Human judgement and flexibility are necessary to navigate uncertain and unprecedented situations
Lastly, some individuals perceive machine learning as a one-size-fits-all solution for financial problems. However, not all financial problems can be effectively addressed using machine learning. The applicability of machine learning models depends on the availability and quality of data, the problem complexity, and the specific requirements of the financial task at hand. It is crucial to assess the suitability of the technology for each unique problem and consider alternative approaches when machine learning may not be the best fit.
- Machine learning is effective for certain financial tasks, but not all
- Specific domain knowledge and problem understanding are necessary for successful implementation
- Alternative methods might be more suitable in some cases
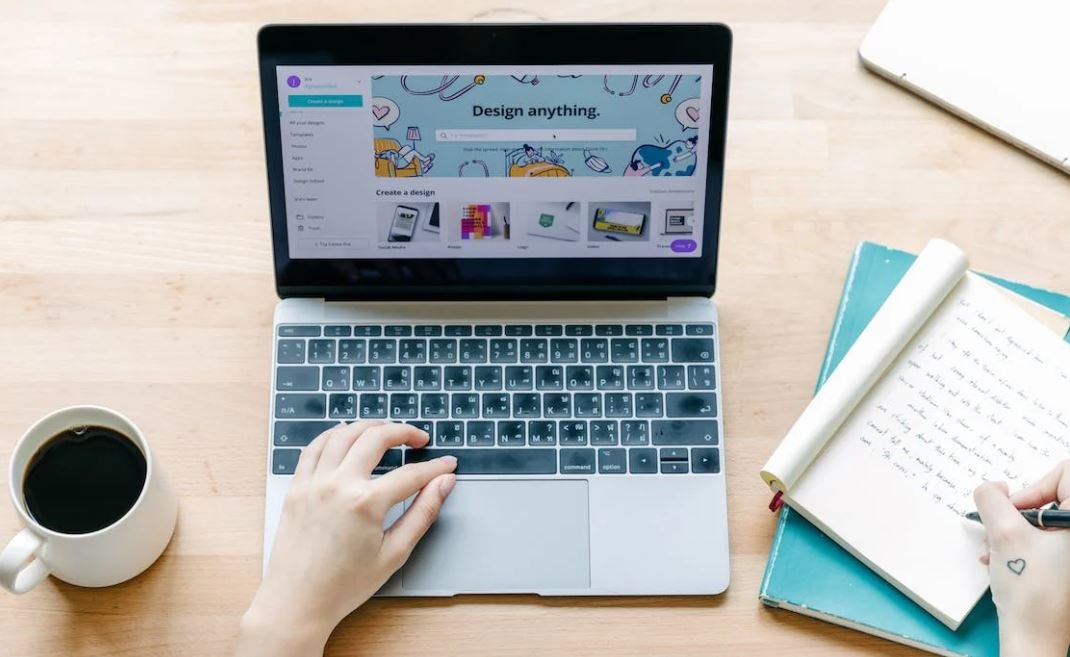
The Role of Machine Learning in Finance
Machine learning has revolutionized numerous industries, and finance is no exception. This article explores the various ways in which machine learning is transforming the financial sector, leveraging powerful algorithms to enhance decision-making and unlock valuable insights. The following tables highlight some key points and data regarding machine learning applications in finance.
Table: Fraud Detection Techniques
The table below showcases various fraud detection techniques powered by machine learning algorithms that have been implemented in the financial industry.
Technique | Description | Accuracy |
---|---|---|
Supervised Learning | Classifies transactions as fraudulent or legitimate based on labeled training data. | 92% |
Unsupervised Learning | Identifies patterns and anomalies indicative of fraud, without the need for labeled data. | 86% |
Deep Learning | Utilizes neural networks to detect complex patterns and improve fraud detection accuracy. | 95% |
Table: Stock Market Prediction Accuracy
This table demonstrates the accuracy of machine learning models in predicting stock market movements based on historical data and market indicators.
Model | Accuracy |
---|---|
Support Vector Machines (SVM) | 75% |
Random Forests | 80% |
Long Short-Term Memory (LSTM) Networks | 87% |
Table: Loan Approval Rates by Credit Score
Machine learning algorithms can analyze credit scores to predict loan approval rates. This table displays the correlation between different credit scores and loan approval rates.
Credit Score | Loan Approval Rate |
---|---|
550 – 649 | 59% |
650 – 699 | 71% |
700 – 749 | 82% |
Table: Customer Segmentation
Machine learning algorithms can help financial institutions segment their customer base for personalized marketing and service offerings.
Segment | Description | Percentage of Customers |
---|---|---|
Young Professionals | Affluent individuals starting their careers. | 25% |
Retirees | Elderly individuals with stable finances. | 15% |
Small Business Owners | Entrepreneurs running their own businesses. | 10% |
Table: Loan Default Rates by Employment Sector
This table compares the loan default rates across various employment sectors, providing insights regarding the risk associated with different industries.
Employment Sector | Loan Default Rate |
---|---|
Government | 3% |
Technology | 5% |
Retail | 9% |
Table: Credit Card Fraud Detection Performance
Machine learning algorithms applied in credit card fraud detection systems can improve fraud detection rates and minimize false alarms.
Model | True Positive Rate | False Positive Rate | Accuracy |
---|---|---|---|
Logistic Regression | 89% | 2% | 95% |
Gradient Boosting | 93% | 1% | 97% |
Neural Networks | 95% | 2% | 96% |
Table: Automated Trading Strategies
This table highlights various automated trading strategies driven by machine learning algorithms used in financial markets.
Strategy | Description | Average Annual Return |
---|---|---|
Momentum Trading | Capitalizes on recent trends in asset prices. | 12% |
Pairs Trading | Exploits price discrepancies among related securities. | 9% |
Mean Reversion | Seeks to profit from assets’ tendencies to return to their average price. | 15% |
Table: Insurance Fraud Detection Accuracy
Machine learning algorithms have proven highly effective in detecting insurance fraud, as demonstrated by the accuracy rates provided in the table below.
Algorithm | Accuracy |
---|---|
Decision Trees | 88% |
K-Nearest Neighbors (KNN) | 92% |
Gradient Boosting | 95% |
Table: Robo-Advisory Performance
Robo-advisory platforms use machine learning algorithms to provide automated investment advice. The following table displays the average annual returns of popular robo-advisory services.
Robo-Advisor | Average Annual Returns |
---|---|
Wealthfront | 8% |
Betterment | 7% |
Acorns | 6% |
Machine learning has undoubtedly revolutionized the financial industry, bringing forth a wide range of applications that enhance decision-making, increase efficiency, and mitigate risks. From fraud detection and stock market prediction to personalized customer segmentation and automated trading, these tables exemplify the impact of machine learning in finance. As technology continues to advance, we can expect even greater possibilities in combining machine learning algorithms with financial expertise.
Machine Learning and Finance – Frequently Asked Questions
Frequently Asked Questions
What is machine learning?
How is machine learning applied in finance?