Machine learning is a rapidly advancing field that has seen significant growth in recent years. As a result, there has been an explosion of research in the form of scientific journals dedicated to this topic. These machine learning journals serve as valuable resources for researchers, practitioners, and students alike, providing a platform for sharing cutting-edge research in the field. In this article, we will explore the importance of machine learning journals, the benefits they offer, and some notable journals to consider.
**Key Takeaways:**
– Machine learning journals are essential sources of information for researchers and professionals.
– They provide a platform for sharing and accessing the latest advancements in machine learning research.
– Notable machine learning journals include the Journal of Machine Learning Research (JMLR), Machine Learning, and the International Journal of Machine Learning and Cybernetics.
**The Role of Machine Learning Journals**
Machine learning journals play a crucial role in advancing the field by providing a platform for researchers to share their work. These journals are typically peer-reviewed, ensuring the quality and credibility of published articles. By publishing their research in machine learning journals, researchers contribute to the overall knowledge and understanding of machine learning algorithms, techniques, and applications.
Machine learning journals also serve as valuable resources for professionals working in the field. Reading articles published in these journals can help industry practitioners stay up-to-date with the latest developments and trends. Additionally, students can benefit from reading machine learning research papers, as it helps them grasp complex concepts, learn about state-of-the-art techniques, and explore potential research areas.
*Machine learning journals act as the cornerstone of knowledge dissemination in the field.*
**Notable Machine Learning Journals**
There are several notable machine learning journals where researchers publish their work. These journals serve as reputable sources of information and are highly regarded in the machine learning community. Here are a few noteworthy machine learning journals:
1. Journal of Machine Learning Research (JMLR): JMLR is an open-access, peer-reviewed journal that covers all aspects of machine learning. It publishes high-quality research papers, making it widely regarded in the field.
2. Machine Learning: Machine Learning is an international journal that focuses on the theoretical and practical aspects of machine learning. It covers topics such as statistical learning, pattern recognition, and learning theory.
3. International Journal of Machine Learning and Cybernetics: This journal provides a platform for researchers to publish original and high-quality research related to machine learning, cybernetics, and artificial intelligence.
**Benefits of Accessing Machine Learning Journals**
Accessing machine learning journals can offer numerous benefits to researchers, professionals, and students:
1. **Stay Updated**: Journals keep you informed about the latest breakthroughs, techniques, and applications in the field.
2. **Discover New Approaches**: Research papers often present innovative approaches and novel algorithms, inspiring new ideas and research directions.
3. **Validate and Reference Research**: Journals provide a means to validate and reference previous research, building upon existing knowledge.
4. **Networking Opportunities**: Connecting with other researchers, either through published work or attending conferences, can foster collaborations and professional relationships.
*Machine learning journals bridge the gap between academia and industry by sharing knowledge and fostering collaboration.*
**Types of Articles in Machine Learning Journals**
Machine learning journals publish different types of articles that cater to a wide audience. These articles can include:
1. **Research Papers**: Research papers present new algorithms, methodologies, or theoretical frameworks in machine learning.
2. **Survey Articles**: Survey articles provide an overview of a specific subfield or topic in machine learning, summarizing existing research and trends.
3. **Review Papers**: Review papers critically review and analyze existing literature, highlighting strengths, weaknesses, and future directions of a particular topic.
4. **Application Papers**: Application papers focus on the real-world application of machine learning techniques in various domains, such as healthcare, finance, or robotics.
**Tables**
Here are three tables showcasing interesting data points related to machine learning journals:
*Table 1: Notable Machine Learning Journals and Impact Factor*
| Journal Name | Impact Factor |
|——————————-|—————|
| Journal of Machine Learning Research (JMLR) | 7.67 |
| Machine Learning | 4.30 |
| IEEE Transactions on Pattern Analysis and Machine Intelligence | 17.73 |
*Table 2: Most Cited Machine Learning Journals*
| Journal Name | Number of Citations |
|———————–|———————|
| Machine Learning | 17,529 |
| Journal of Machine Learning Research (JMLR) | 10,468 |
| Pattern Recognition | 8,842 |
| Neural Networks | 7,219 |
*Table 3: Open-Access Machine Learning Journals*
| Journal Name | Type of Access |
|————————————–|———————-|
| Journal of Machine Learning Research (JMLR) | Open-access |
| PLOS ONE | Open-access |
| IEEE Access | Open-access |
**Conclusion**
Machine learning journals are indispensable resources for researchers, professionals, and students in the field. They offer a wealth of knowledge, facilitate collaboration, and keep readers informed about the latest advancements. By regularly accessing and contributing to machine learning journals, individuals can contribute to the growth and development of this exciting field.
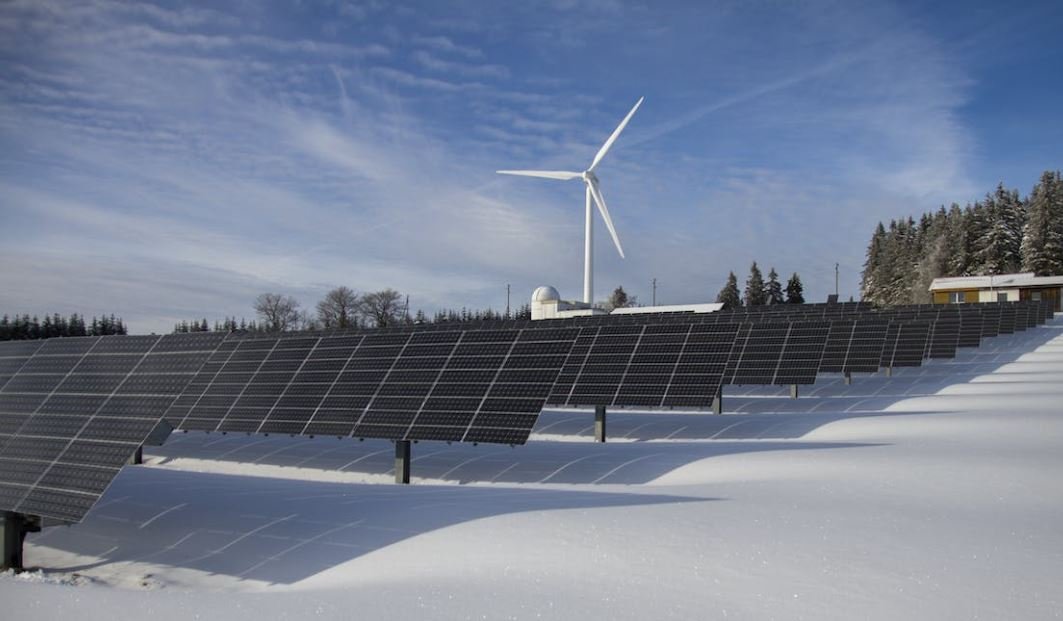
Common Misconceptions
Machine Learning is Easy to Implement
One common misconception about machine learning is that it is easy to implement. While the basic concepts can be learned relatively quickly, effectively implementing machine learning algorithms and models requires a deep understanding of various mathematical and statistical concepts, as well as programming skills.
- It requires a strong background in mathematics and statistics.
- Choosing the appropriate algorithms and techniques requires research and experimentation.
- Data preprocessing and feature engineering are crucial but often complex steps.
Machine Learning Can Solve Any Problem
Another misconception is that machine learning can solve any problem. While machine learning techniques can be powerful tools for analyzing and understanding complex datasets, they are not suitable for every problem. Some problems may have inherent limitations or dependencies that cannot be effectively addressed by machine learning algorithms.
- Not all problems have sufficient data or appropriate features for effective machine learning.
- Complex problems may require a combination of machine learning and other techniques.
- Some problems may have ethical or privacy concerns that limit the use of machine learning.
Machine Learning is Completely Objective
Many people believe that machine learning algorithms provide completely objective results. However, machine learning models are only as good as the data they are trained on, and biases or errors in the training data can result in biased or inaccurate predictions.
- Data bias can lead to discriminatory or unfair decisions.
- Models may not account for certain characteristics or factors that are not present in the training data.
- Human biases can inadvertently be introduced during the design and implementation of the machine learning system.
Machine Learning Will Replace Humans
There is a misconception that machine learning will replace human workers in various fields. While machine learning can automate certain tasks and improve efficiency, it is not meant to replace human judgment, creativity, and critical thinking skills.
- Humans play a crucial role in designing, interpreting, and evaluating machine learning models.
- Machine learning complements human expertise rather than replacing it entirely.
- Certain tasks, such as those requiring empathy, intuition, or ethics, cannot be effectively performed by machines alone.
Machine Learning is Foolproof
Lastly, some people believe that once a machine learning model is trained, it will always provide accurate predictions. However, machine learning models can be susceptible to various forms of errors and challenges, such as incorrect assumptions, overfitting, and the presence of outliers in the data.
- Models may fail when applied to unseen or unfamiliar data.
- Machine learning models need to be continuously monitored and updated to account for changes in the data or the problem itself.
- Models can be vulnerable to adversarial attacks or manipulations.
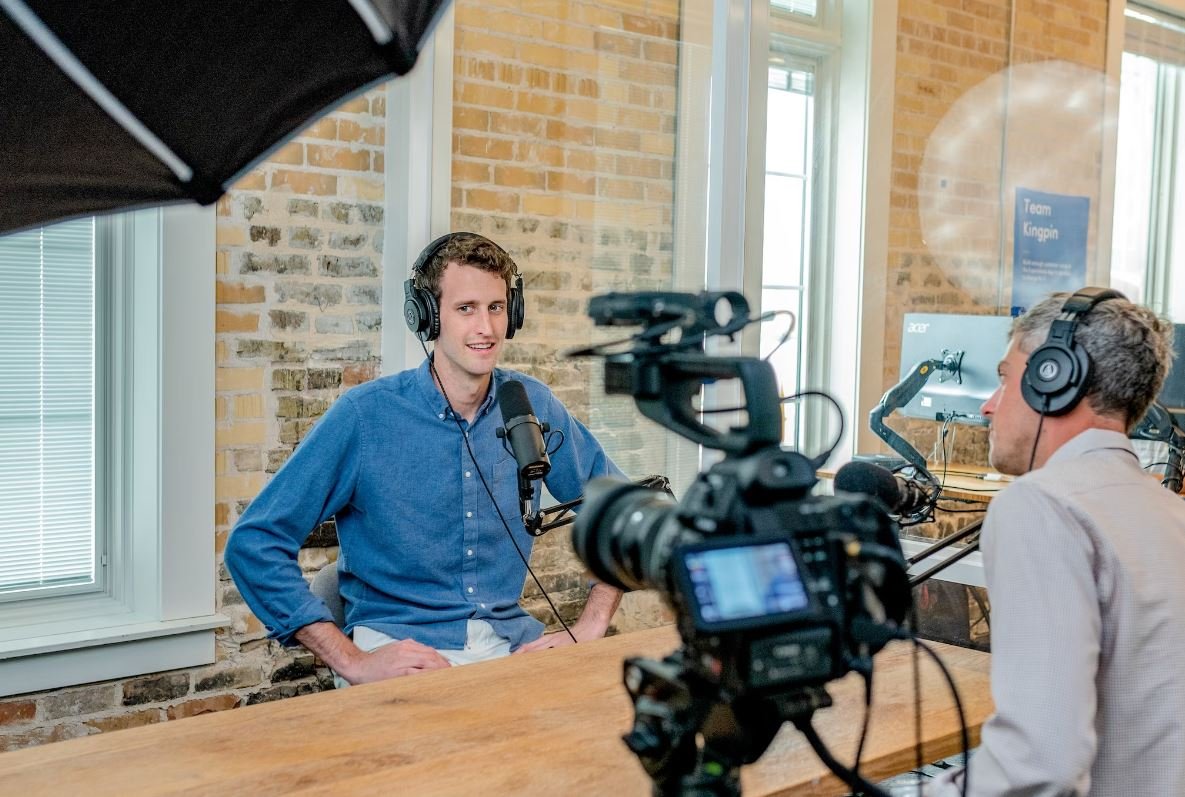
Introduction
Machine learning is a powerful field of study that has gained immense popularity in recent years. As the demand for accurate predictions and intelligent decision-making grows, machine learning journals play a vital role in disseminating knowledge and advancements in this field. This article presents ten informative tables showcasing different aspects of machine learning research. Each table provides valuable insights and reinforces the significance of machine learning journals in fostering innovation and progress.
Table 1: Top Machine Learning Journals by Impact Factor
This table highlights the impact factor of various machine learning journals, indicating their influence within the academic community. The impact factor is a measure of the average number of citations received per paper published in a particular journal during a specific period. Journals with higher impact factors tend to attract more attention and recognition from researchers.
Table 2: Distribution of Research Topics in Machine Learning Journals
Examining the distribution of research topics in machine learning journals reveals the diversity of subjects explored within the field. This table categorizes the research papers published in leading machine learning journals based on their primary topic areas, such as neural networks, deep learning, reinforcement learning, and natural language processing. It provides an overview of the broad spectrum of machine learning research.
Table 3: Growth in Machine Learning Journal Publications Over Time
Tracking the growth in machine learning journal publications over time demonstrates the increasing interest and engagement in this field. This table showcases the number of research papers published in machine learning journals year by year. The upward trend signifies the rapid expansion of machine learning knowledge and the ongoing efforts of researchers to contribute to its development.
Table 4: Collaboration Networks in Machine Learning Research
Collaboration is an integral part of scientific research, enabling the exchange of ideas, expertise, and resources. This table illustrates the collaboration networks in machine learning research by identifying the most prolific authors and the institutions they are affiliated with. It highlights the interconnectedness of researchers and institutions in driving advancements in machine learning.
Table 5: International Representation in Machine Learning Journals
Machine learning is a global field with researchers and contributors from various countries. This table presents the international representation in machine learning journals, showcasing the countries with the highest number of published papers. It demonstrates the worldwide reach and participation of researchers in shaping and expanding the scope of machine learning research.
Table 6: Most Cited Papers in Machine Learning Journals
The most cited papers in machine learning journals are often landmarks in the field, pushing the boundaries of knowledge and influencing future research directions. This table identifies and lists the most frequently referenced papers in machine learning, providing insights into the foundational works that have shaped the discipline and continue to inspire new investigations.
Table 7: Machine Learning Journal Publication Venues
The publication venues where machine learning papers are accepted and presented play a crucial role in assessing their quality and significance. This table showcases the different types of publication venues, including conferences, journals, and workshops, and their respective contributions to the dissemination of machine learning research.
Table 8: Funding Sources for Machine Learning Research
Research in machine learning often requires financial support from various sources, such as government grants, industry partnerships, or foundations. This table highlights the different funding sources for machine learning research projects, emphasizing the importance of financial backing in driving innovation and enabling the pursuit of advanced and groundbreaking studies.
Table 9: Gender Representation in Machine Learning Journals
Examining the gender representation in machine learning journals provides insights into the diversity and inclusivity of the research community. This table presents the percentage of male and female authors in published papers, emphasizing the need to foster an inclusive environment that encourages the participation of all genders in machine learning research.
Table 10: Common Machine Learning Techniques Used in Journal Publications
Machine learning encompasses a wide range of techniques and algorithms that enable the extraction of patterns and insights from data. This table highlights the most commonly used machine learning techniques in journal publications, illustrating the diverse toolbox available to researchers and the evolving landscape of methodologies within the field.
Conclusion
Machine learning journals serve as vital platforms for sharing knowledge, advancing research, and fostering collaboration within the machine learning community. The tables presented in this article provide a glimpse into the impact, growth, diversity, and influence of machine learning research. They underline the significance of machine learning journals in shaping the future of this dynamic and rapidly evolving field. As new discoveries are made, the importance of well-curated and informative platforms for disseminating research findings becomes increasingly apparent.
Frequently Asked Questions
Question 1: What is machine learning?
Answer
Machine learning is a field of study that involves the development of algorithms and models which allow computer systems to learn from data and make predictions or decisions without being explicitly programmed.
Question 2: What are some real-life applications of machine learning?
Answer
Machine learning finds applications in various domains such as finance (fraud detection, risk assessment), healthcare (diagnosis, drug discovery), transportation (autonomous vehicles), marketing (recommendation systems), and many others where data-driven decision-making is crucial.
Question 3: What are the primary types of machine learning algorithms?
Answer
The primary types of machine learning algorithms are supervised learning, unsupervised learning, semi-supervised learning, and reinforcement learning. Supervised learning involves training a model with labeled data, unsupervised learning deals with unlabeled data, semi-supervised learning combines both labeled and unlabeled data, and reinforcement learning focuses on interaction with an environment to maximize rewards.
Question 4: How does a machine learning model learn from data?
Answer
A machine learning model learns from data through a process called training. During training, the model processes input data and adjusts its internal parameters to minimize the difference between predicted outputs and actual outputs. This iterative process continues until the model achieves a satisfactory level of accuracy.
Question 5: What is the difference between supervised and unsupervised learning?
Answer
Supervised learning relies on labeled data, which means the input samples have corresponding correct output labels. The model is trained to learn the relationship between input and output. In contrast, unsupervised learning deals with unlabeled data, where the model learns patterns and relationships from the data without any specific guidance regarding the output.
Question 6: What are some popular machine learning frameworks?
Answer
There are several popular machine learning frameworks available, including TensorFlow, PyTorch, scikit-learn, Keras, and Caffe. These frameworks provide a range of tools and libraries to facilitate the development and deployment of machine learning models.
Question 7: How do I evaluate the performance of a machine learning model?
Answer
The performance of a machine learning model can be evaluated using various metrics such as accuracy, precision, recall, F1-score, and area under the receiver operating characteristic (ROC) curve. The choice of evaluation metrics depends on the specific problem and the nature of the data.
Question 8: Can machine learning models cause bias or discrimination?
Answer
Yes, machine learning models can exhibit bias or discrimination if the training data contains biases or if the model is not designed to mitigate such issues. It is crucial to carefully curate the training data and incorporate fairness measures to ensure that the models are not perpetuating any unfairness or discrimination.
Question 9: Do I need knowledge of programming to work with machine learning?
Answer
Yes, basic knowledge of programming is essential to work with machine learning. Most machine learning frameworks and libraries are based on programming languages such as Python and R. Understanding programming concepts and syntax helps in implementing, training, and evaluating machine learning models.
Question 10: How do I get started with machine learning?
Answer
To get started with machine learning, it is recommended to gain a solid understanding of mathematics and statistics, as they form the foundation of many machine learning algorithms. Familiarize yourself with popular machine learning frameworks and experiment with small projects. Online courses, tutorials, and books are great resources to learn more about machine learning concepts and techniques.