ML in an Ounce
Machine learning (ML) is a fascinating field of study that has gained immense popularity in recent years. It involves the use of algorithms and statistical models to enable computer systems to learn from data and improve their performance on specific tasks without being explicitly programmed. ML has a wide range of applications across various industries, including healthcare, finance, marketing, and more. In this article, we will delve into the world of ML, exploring its key concepts, benefits, and challenges.
Key Takeaways:
- Machine learning involves algorithms and statistical models to enable computer systems to learn from data and improve performance.
- ML has applications in industries such as healthcare, finance, marketing, and more.
- Understanding ML concepts, benefits, and challenges is crucial for successful implementation.
Understanding ML Concepts
1. Machine learning algorithms can be categorized into three main types: Supervised, Unsupervised, and Reinforcement Learning. Each type serves a specific purpose in analyzing and making predictions from data.
2. Supervised learning algorithms learn patterns and relationships from a labeled dataset, where the correct answers are known. They can be used for tasks such as classification and regression.
Unsupervised learning, on the other hand, allows machines to learn from an unlabeled dataset, finding hidden patterns and structures within the data.
3. Reinforcement learning involves an agent learning to take actions in an environment to maximize a reward signal. This type of learning is often used in gaming AI and autonomous systems.
The Benefits of ML
- ML enables automation of complex tasks, saving time and increasing efficiency.
- ML algorithms can process and analyze large amounts of data in a short period, extracting valuable insights.
- By leveraging ML, businesses can make data-driven decisions, leading to improved accuracy and better outcomes.
- ML allows for the creation of personalized experiences for users, enhancing customer satisfaction.
The Challenges of ML
1. ML models require high-quality, diverse, and relevant data for training. Insufficient or biased training data can lead to inaccurate results and biased predictions.
2. Overfitting is another challenge, where a model becomes too specialized in the training data and fails to generalize well to new data.
To mitigate these challenges, data preprocessing, feature engineering, and model evaluation techniques are used.
Interesting Info and Data Points
Industry | Benefits of ML |
---|---|
Healthcare |
|
Finance |
|
Type of ML | Example |
---|---|
Supervised Learning | Image classification (identifying objects in images) |
Unsupervised Learning | Market segmentation (grouping customers based on behavior) |
Reinforcement Learning | Autonomous driving (learning to navigate the roads) |
Key Challenges |
---|
|
Implementing ML Successfully
- Identify the problem and define clear objectives. Understanding the business context is important for effective ML implementation.
- Collect, preprocess, and analyze relevant data. Data quality and relevance play a crucial role in the success of ML models.
- Choose the most suitable ML algorithm(s) based on the problem and available data. Experimentation with multiple algorithms may be required for optimal results.
- Evaluate and fine-tune the ML model(s) using appropriate metrics and testing procedures.
- Once the model is ready, deploy it into a production environment and monitor its performance regularly.
Exploring the World of ML
Machine learning opens up a realm of possibilities for businesses and industries, enabling them to harness the power of data for improved decision-making, efficiency, and customer satisfaction. By understanding the concepts, benefits, and challenges associated with ML, organizations can embark on successful implementations, reaping the rewards of this rapidly evolving field.
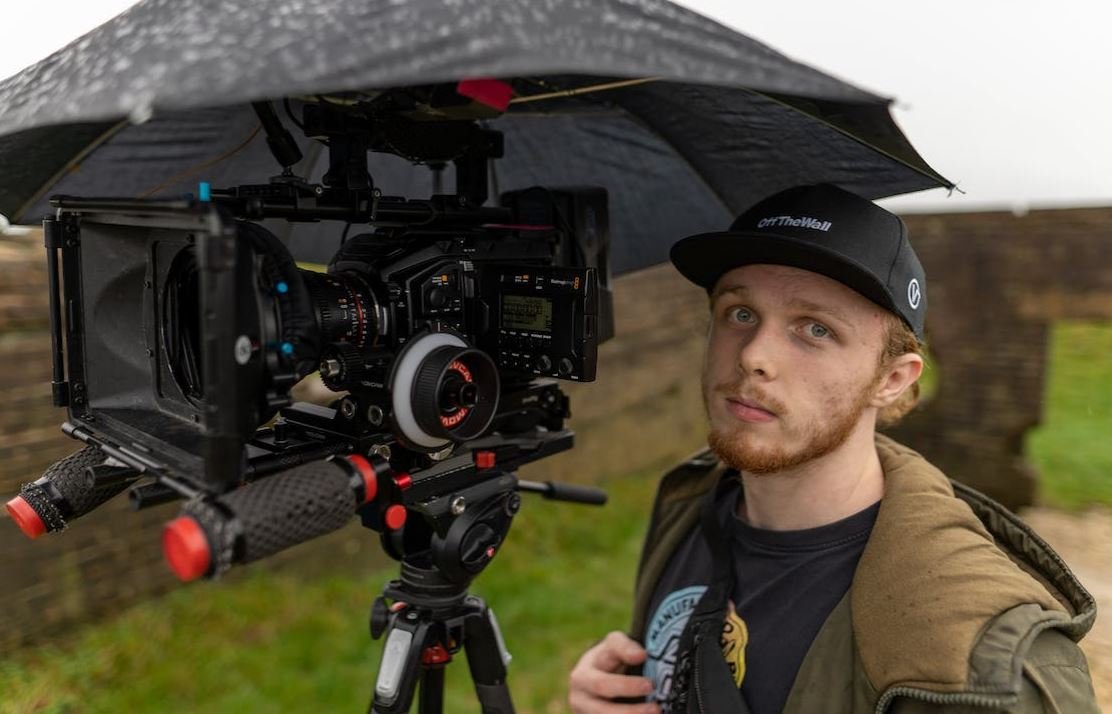
Common Misconceptions
Machine Learning is only for experts
One common misconception about Machine Learning (ML) is that it is a field exclusively for experts or data scientists. However, this is not true as there are now several user-friendly tools and platforms available that allow even non-experts to apply ML algorithms and models.
- ML platforms like Microsoft Azure and Google Cloud AutoML provide user-friendly interfaces.
- Online tutorials and courses make it easier for individuals to learn and apply ML techniques.
- Several open-source libraries, such as scikit-learn and TensorFlow, provide accessible tools for ML.
ML algorithms always provide correct answers
Another misconception is that ML algorithms always produce accurate and correct answers. However, ML algorithms are dependent on the quality and nature of the input data, and their performance can vary.
- Data quality and bias can affect the accuracy of ML algorithms.
- The performance of ML algorithms heavily relies on the appropriate selection and preprocessing of data.
- ML models need continuous monitoring and updating to adapt to changes in the environment.
ML will replace human jobs entirely
Many people believe that ML will eventually replace human jobs entirely. While ML has the potential to automate certain tasks, it is unlikely to completely replace human involvement in many fields.
- ML can automate repetitive and mundane tasks, allowing humans to focus on more complex decision-making.
- Human intervention is often required to interpret and validate ML results.
- ML systems still need supervision and guidance from humans to ensure ethical and responsible decision-making.
ML can solve any problem without limitations
Some people have the misconception that ML algorithms can solve any problem and provide accurate solutions in all scenarios. However, ML has its limitations and is not a one-size-fits-all solution.
- Availability and quality of data can restrict the effectiveness of ML algorithms in certain domains.
- Complex problems may require a combination of multiple ML techniques and human expertise.
- ML algorithms may struggle with interpreting causality and making judgment calls in complex scenarios.
ML is only applicable to large-scale organizations
Lastly, some people assume that ML is only applicable to large-scale organizations with abundant resources. However, ML techniques can be beneficial for businesses of all sizes, even small startups or individual entrepreneurs.
- Cloud-based ML platforms have made it more affordable and accessible for small businesses to utilize ML techniques.
- ML can help small businesses gain insights from their data, optimize processes, and improve decision-making.
- Open-source ML libraries allow developers to leverage existing resources without significant investments.

Ml in an Ounce: The Power of Conversion
When it comes to measuring fluid, different countries and industries use different units of measurement. In the culinary world, ounces are commonly used to measure liquids; however, in scientific and medical contexts, milliliters are the preferred unit. This article explores the fascinating world of conversion between milliliters and ounces, highlighting the importance of accuracy and precision in various domains.
Ancient Egyptian Remedies: Volume in Ounces
Long before the standardized metric system, Ancient Egyptians used their own system of measurement. In their medical practices, they measured fluids in ounces, which were approximately equivalent to 29.57 milliliters. This table showcases the volume of various ancient Egyptian remedies in ounces:
Remedy | Volume (ounces) |
---|---|
Honey | 2 |
Olive Oil | 3 |
Milk | 6 |
Wine | 12 |
The Medicine Dropper: Precision in Milliliters
In modern medicine, precision and accuracy are crucial, and milliliters provide a more precise way of measuring fluids. Consider the milliliter equivalents for various common medicine dropper markings:
Drop Marking | Volume (milliliters) |
---|---|
0.1 | 0.0033814 |
0.2 | 0.0067628 |
0.3 | 0.0101442 |
0.4 | 0.0135256 |
Beauty Conversions: Milliliters in Makeup
When it comes to cosmetics, both ounces and milliliters are commonly used for measuring the contents of beauty products. To help convert between the two, here are some milliliter equivalents for popular beauty product sizes:
Product Size | Volume (milliliters) |
---|---|
Lipstick | 3.5 |
Mascara | 10 |
Foundation | 30 |
Body Lotion | 200 |
The Liquid Gold: Milliliters of Precious Metals
When it comes to valuable metals, such as gold and silver, their worth is often determined by weight. In the jewelry industry, grams and ounces are frequently used, while milliliters come into play when measuring certain liquid forms of precious metals. Here are some approximate milliliter volumes for common liquid specimens:
Precious Metal | Volume (milliliters) |
---|---|
Gold | 34.79 |
Silver | 39.28 |
Platinum | 36.96 |
Rhodium | 87.13 |
The Gastronomic World: Fluid Artistry
Food and drink enthusiasts often engage in culinary experiments, where precision measurements are pivotal for achieving the desired outcomes. Let’s look at some conversions between milliliters and ounces for commonly used culinary ingredients:
Ingredient | Volume (milliliters) |
---|---|
Water | 30 |
Milk | 28.41 |
Olive Oil | 29.57 |
Cooking Wine | 29.57 |
From Petrol to Milliliters: Efficiency Matters
The automobile industry and fuel providers often express fuel efficiency in terms of miles per gallon (MPG) or liters per 100 kilometers (L/100km). Nevertheless, the liquid fuel itself is commonly measured in gallons and liters. Consider the milliliter equivalents for gallons of various fuels:
Fuel Type | Volume (milliliters) |
---|---|
Petrol | 3785.41 |
Diesel | 4164.87 |
Ethanol | 3785.41 |
Propane | 3785.41 |
The Potion Brewing: Volume of Magic
In the realm of fantasy and magical tales, potions and elixirs play significant roles. To add an air of authenticity to your fictional adventures, let’s explore the milliliter measurements for some commonly brewed potions:
Potion Type | Volume (milliliters) |
---|---|
Healing Potion | 100 |
Invisibility Elixir | 50 |
Strength Tonic | 75 |
Love Potion | 10 |
Ml in Your Morning Cup: The Caffeine Kick
Coffee lovers can attest to the importance of getting the right amount of caffeine, particularly in the morning. Let’s delve into the milliliter measurements for various standard coffee sizes:
Coffee Size | Volume (milliliters) |
---|---|
Espresso Shot | 30 |
Small Coffee | 237 |
Medium Coffee | 355 |
Large Coffee | 473 |
A World of Conversion: Bridging Cultures
The globalized world connects people from diverse cultures, resulting in the need for effective communication and conversion between units of measurement. Accurate conversions between milliliters and ounces facilitate interactions in numerous domains, enabling seamless collaboration and understanding among individuals and industries worldwide.
Conclusion
The conversion between milliliters and ounces holds immense significance across various fields, ranging from medicine and culinary arts to ancient practices and fantasy realms. Precise measurement, be it in milliliters or ounces, ensures accuracy and consistency in the outcomes of our endeavors. Regardless of the subject matter, understanding the power of conversion unifies our world, enabling efficient communication and collaboration in an interconnected global society.
Frequently Asked Questions
What is ML in an Ounce?
ML in an Ounce is a machine learning platform that aims to simplify and streamline the process of implementing ML algorithms and models. It provides a user-friendly interface, comprehensive documentation, and various tools to facilitate ML development.
How can ML in an Ounce benefit me?
By using ML in an Ounce, you can leverage the power of machine learning to tackle complex tasks and automate decision-making processes. The platform offers pre-trained models, data preprocessing capabilities, and statistical analysis tools, making it easier for you to build, train, and deploy ML models without extensive programming knowledge or experience.
What types of machine learning algorithms are supported by ML in an Ounce?
ML in an Ounce supports a wide range of machine learning algorithms, including but not limited to regression, classification, clustering, deep learning, and reinforcement learning. It also incorporates popular libraries such as TensorFlow and Scikit-learn to provide a comprehensive set of tools for ML development.
Can I use my own dataset with ML in an Ounce?
Absolutely! ML in an Ounce allows you to upload and use your own datasets for training and testing ML models. The platform also offers data preprocessing features to handle common data cleaning and transformation tasks, ensuring that your dataset is prepared for analysis and model development.
Is ML in an Ounce suitable for beginners?
Yes, ML in an Ounce is designed to be beginner-friendly. It provides an intuitive user interface and offers step-by-step guidance throughout the ML development process. Even if you have little to no prior experience in machine learning, you can still use ML in an Ounce to harness the power of ML algorithms and models.
Does ML in an Ounce require coding skills?
No, ML in an Ounce is designed to minimize the need for extensive coding skills. While having some programming knowledge can be beneficial, it is not a requirement to use the platform. ML in an Ounce offers a visual interface and drag-and-drop functionality, allowing users to build and customize ML models without writing complex code.
Can I deploy ML models developed in ML in an Ounce?
Absolutely! ML in an Ounce offers deployment options for ML models. Once you have developed and trained your model in the platform, you can export it in various formats, such as TensorFlow SavedModel or ONNX, for integration with other applications or hosting on a cloud-based platform.
Is my data secure when using ML in an Ounce?
ML in an Ounce takes data security and privacy seriously. The platform implements industry-standard security measures to protect user data and prevent unauthorized access. Additionally, ML in an Ounce gives users full control over their data, allowing them to choose whether to store it locally or in secured cloud storage.
Can I collaborate with others on ML projects using ML in an Ounce?
Yes, ML in an Ounce supports collaboration features that enable users to work together on ML projects. You can invite team members, share datasets and models, and collaborate in real-time. This makes it easier for teams to collaborate, share knowledge, and collectively develop ML solutions.
Are there any tutorials or resources available for ML in an Ounce?
Absolutely! ML in an Ounce provides comprehensive documentation, tutorials, and resources to assist users in getting started with the platform and exploring its features. You can find step-by-step guides, video tutorials, and example projects that showcase the capabilities of ML in an Ounce.