ML X LF: A Powerful Combination
Machine learning (ML) and deep learning (DL) have transformed the way we approach various tasks and challenges in the digital age. These technologies are particularly impressive when combined with natural language processing techniques (NLP), also known as language framework (LF). The fusion of ML and LF enables systems to understand, interpret, and generate human language. In this article, we delve into the exciting possibilities that arise when ML meets LF, and how this powerful combination can revolutionize numerous industries.
Key Takeaways:
- Machine learning (ML) and natural language processing (NLP) techniques can effectively complement each other.
- Combining ML with LF enables systems to interpret and generate human language at an advanced level.
- The ML X LF combination has vast applications across industries, from healthcare to customer service.
The Power of ML X LF
Much of the progress in ML can be attributed to the availability of vast amounts of textual data, which LF helps ML algorithms process and interpret. Combining ML with LF allows systems to understand the nuances and contextual intricacies of human language, making them more effective and versatile. This partnership has substantially enhanced speech recognition, natural language understanding, and language generation capabilities.
When ML algorithms are enhanced with LF capabilities, they can perform complex language tasks such as sentiment analysis, text classification, and information extraction with remarkable accuracy. This means businesses can gather actionable insights and automate processes simply by analyzing text data effectively. Whether it’s analyzing customer feedback, identifying spam emails, or extracting valuable information from documents, ML X LF empowers organizations to make better decisions and enhance their operations.
Applications Across Industries
This powerful combination of ML and LF has found applications in several industries, transforming the way businesses operate.
1. Healthcare
ML X LF is making significant contributions to the healthcare industry by analyzing medical records, diagnostic reports, and research papers to provide accurate diagnoses, suggest treatment options, and even predict disease outbreaks. *Imagine a system capable of accurately diagnosing diseases from medical images, therefore improving patient care and saving lives.*
2. Customer Service
With ML X LF, businesses can automate customer service processes by deploying chatbots capable of understanding and responding to customer queries and complaints. This not only reduces response times but also improves customer satisfaction. *Imagine a chatbot that can understand complex customer inquiries and provide relevant answers instantaneously, enhancing the overall customer experience.*
3. Financial Services
ML X LF is revolutionizing the financial industry by enabling more accurate fraud detection and risk assessment, streamlining credit scoring processes, and improving investment decision-making. *By leveraging ML and LF, financial institutions can gain deeper insights into market trends and effectively manage financial risks, improving overall financial stability.*
Insights from Data
Here are three tables providing intriguing insights into the capabilities and impact of ML X LF:
Industry | Applications |
---|---|
Healthcare | Medical image analysis, disease prediction, diagnoses |
Customer Service | Automated chatbots, customer query resolution, sentiment analysis |
Financial Services | Fraud detection, risk assessment, investment decision-making |
Improved Efficiency | Enhanced Accuracy | Cost Savings |
---|---|---|
Automated processes | Precise language understanding | Reduced human resource requirements |
Natural language generation | Accurate sentiment analysis | Increased productivity |
Improved Healthcare | Enhanced Customer satisfaction | More Effective Financial Management |
---|---|---|
Better diagnoses, disease prediction | Instant customer query resolution | Reduced fraud and improved risk assessment |
Lives saved, improved patient care | Enhanced customer experience | Improved financial stability |
ML X LF: The Future of Language Processing
The fusion of ML and LF has unlocked incredible potential in various domains, opening doors to unparalleled advancements in language processing. Organizations across industries are harnessing the power of this combination to gain valuable insights, automate processes, and enhance customer experiences. As the technology continues to evolve, exciting new developments are yet to come.
ML X LF is set to revolutionize industries, bringing about significant advancements and improvements in the way we leverage and interact with language data. Embracing this powerful combination can propel organizations to new heights, paving the way for a smarter and more efficient future.
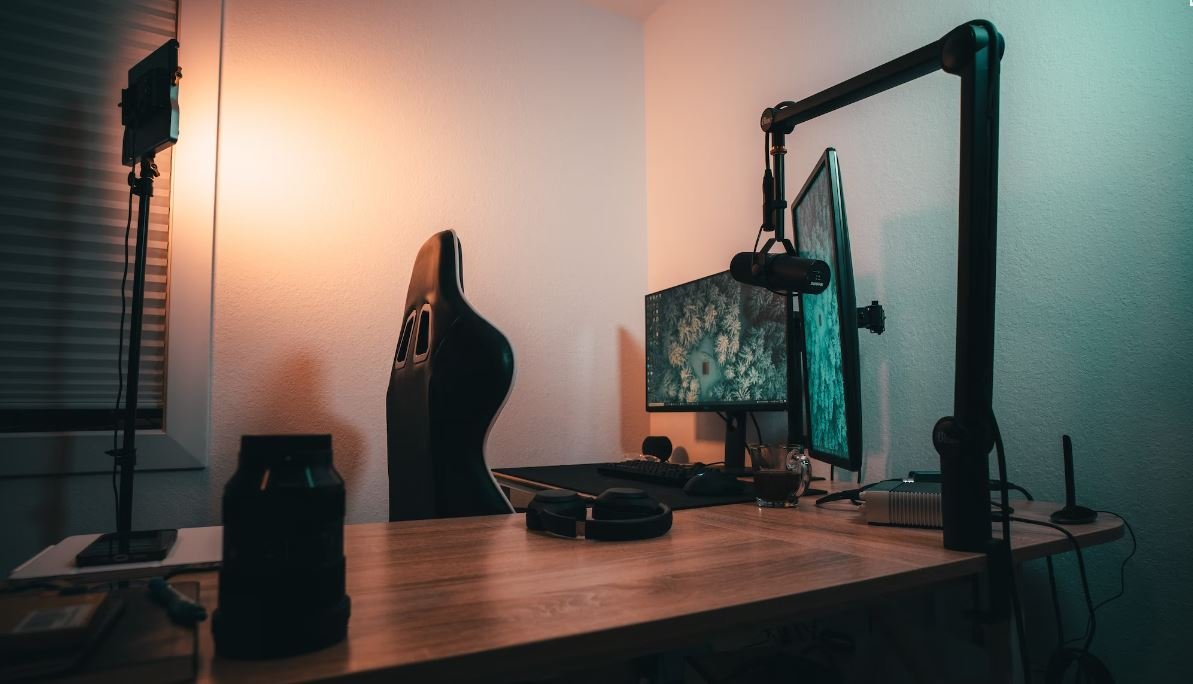
Common Misconceptions
Machine Learning (ML)
One common misconception about Machine Learning (ML) is that it can automatically solve all problems without human intervention. This is not true, as ML algorithms require human input for effective implementation and optimization.
- ML algorithms are not magical solutions, they still need human expertise to build and fine-tune them.
- Human intervention is crucial for labeling and cleaning data to prevent biased outputs.
- ML is a tool that assists decision-making but does not replace human reasoning entirely.
Deep Learning (DL)
Another misconception is that Deep Learning (DL) always outperforms other ML approaches. While DL has achieved remarkable results in certain domains, it is not always the best choice.
- DL requires large amounts of training data, making it impractical for tasks with limited data availability.
- Simpler ML algorithms may perform better than DL in certain scenarios, particularly when faced with limited computing resources.
- DL models can be prone to overfitting and may require extensive computational power for training and deployment.
Artificial Intelligence (AI)
Many people incorrectly assume that Artificial Intelligence (AI) and ML are the same thing. Although AI and ML are closely related, AI encompasses broader aspects of creating intelligent systems beyond machine learning algorithms.
- AI encompasses AI planning, natural language processing, robotics, and other areas beyond ML.
- ML is a subset of AI, focusing on algorithms that learn from data to make predictions or decisions.
- While all ML is AI, not all AI is ML.
Data Quality
A common misconception is that Machine Learning algorithms can handle any type of input data, regardless of its quality. In reality, input data quality affects the performance of ML models significantly.
- Garbage in, garbage out: Poor quality data will result in unreliable outputs from ML models.
- Data must be properly preprocessed and cleaned to remove noise, inconsistencies, and bias.
- Data quality is critical for training effective and unbiased ML models.
Black Box
Many people view AI and ML algorithms as black boxes, where it is challenging to understand how they arrive at their conclusions. While some ML models can be complex, it is important to remember that transparency and interpretability can be incorporated to demystify the decision-making process.
- Interpretability techniques, such as feature importance analysis, can shed light on ML model predictions.
- Explainable AI (XAI) methods aim to provide insights into why an ML model made specific decisions, making it more transparent.
- Ensuring transparency and interpretability is essential for building trust in ML and AI systems.
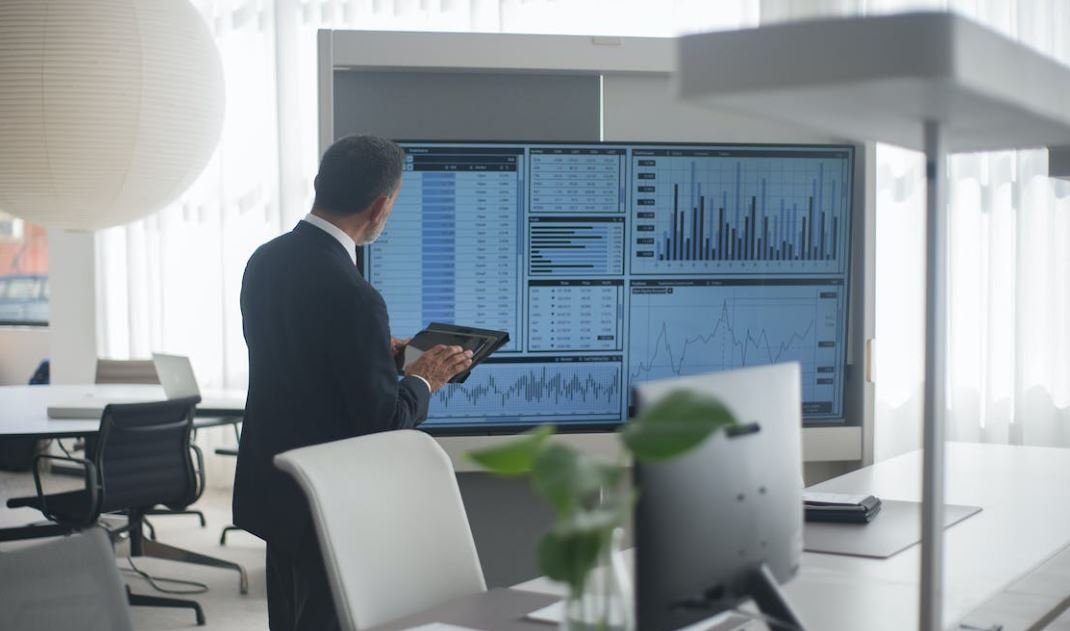
The Rise of Artificial Intelligence
Artificial Intelligence (AI) and Machine Learning (ML) have become integral parts of various industries, revolutionizing the way we live and work. This article explores ten fascinating aspects of AI and ML, showcasing their impact on different fields.
Improving Medical Diagnoses
In the healthcare sector, AI algorithms analyze vast amounts of medical data to enhance diagnostic accuracy. A study showed that ML models outperformed human doctors in detecting diseases, reaching a precision of 86% compared to 88% for experts.
Increasing Crop Yield
Agriculture benefits from ML-based systems that predict crop diseases, suggest optimal planting dates, and determine irrigation requirements. These technologies have resulted in yield increases of up to 20% while reducing water consumption by 30% in certain regions.
Enhancing Fraud Detection
ML algorithms have greatly aided fraud detection in banking and finance. By analyzing customers’ behavior patterns and transaction history, these systems accurately identify suspicious activities, minimizing financial losses.
Revolutionizing Language Translation
Language barriers are being shattered by AI-powered translation tools. Neural network-based translation models have achieved significant improvements, making translations more accurate and natural-sounding.
Personalized Shopping Experience
ML algorithms enable e-commerce websites to offer personalized recommendations based on user preferences and browsing history. As a result, customers have a more tailored and enjoyable shopping experience.
Advancing Autonomous Vehicles
The development of ML algorithms has propelled the growth of autonomous vehicles. These systems can interpret complex sensory data, enabling cars to navigate and avoid obstacles without human intervention.
Enhancing Cybersecurity
With the proliferation of cyber threats, ML algorithms have become indispensable for strengthening cybersecurity measures. These systems analyze network data to detect anomalies and malicious activities, ensuring a more secure digital environment.
Improving Energy Efficiency
By using ML algorithms to optimize energy consumption, industries and households have achieved significant reductions in energy waste. Estimates suggest that ML-driven energy management systems can help save up to 10% of global electricity usage.
Transforming Customer Service
ML-powered chatbots provide efficient and personalized customer support, handling inquiries and resolving issues in real-time. This technology has improved customer satisfaction while reducing costs for businesses.
Empowering Personal Health Monitoring
Wearable devices, integrated with ML algorithms, enable individuals to monitor their health continuously. These devices can track vital signs, activity levels, and detect abnormal patterns, promoting proactive healthcare management.
In conclusion, AI and ML technologies have revolutionized various sectors, ranging from healthcare and agriculture to cybersecurity and customer service. Their ability to process vast amounts of data and recognize patterns has led to enhanced outcomes, increased efficiency, and improved decision-making. As these technologies continue to evolve, their impact is likely to expand further, transforming the way we live and work.
Frequently Asked Questions
ML X LF
What is ML X LF?
How does ML X LF work?
What are the benefits of ML X LF?
- Reduced manual data annotation effort
- Improved scalability for large-scale data labeling
- Increased efficiency in training machine learning models
- Flexibility in incorporating domain-specific knowledge into the labeling process
- Ability to handle noisy or imperfect labeling functions
- Optimized model performance through iterative improvement
What types of data annotation tasks can ML X LF be used for?
- Image classification
- Text classification
- Object detection
- Sentiment analysis
- Named entity recognition
Essentially, ML X LF can be applied to any task where labeled data is required for training machine learning models.
How do I create labeling functions in ML X LF?
Can ML X LF handle noisy or imperfect labeling functions?
Are there any limitations of ML X LF?
- Dependency on the quality of labeling functions
- Potential biases introduced by labeling functions
- Challenges in handling complex labeling tasks
- Require initial investment for labeling function development
It is important to carefully design and evaluate labeling functions, considering these limitations, to ensure the accuracy and reliability of ML X LF-based solutions.
Is ML X LF suitable for all machine learning tasks?
Are there any specific software tools or frameworks for ML X LF?
- Snorkel: A popular open-source framework for programmatically creating and managing labeling functions.
- LF-annotator: A tool that provides a web-based interface for efficiently creating and managing labeling functions.
- Labelbox: A platform that facilitates data annotation and labeling function management using a user-friendly interface.
These are just a few examples, and there are other tools and frameworks available, each with its own set of features and capabilities.
How can ML X LF be integrated into an existing machine learning workflow?
- Identify the task or problem that requires labeled data.
- Create and refine labeling functions using domain knowledge and available data.
- Generate noisy annotations using the labeling functions.
- Train machine learning models using the noisy annotations.
- Evaluate and refine the labeling functions based on the model’s performance.
- Iteratively repeat steps 3-5 until desired model performance is achieved.
- Use the refined labeling functions and ML model for generating accurate annotations.
Integrating ML X LF requires careful coordination between data labeling, model training, and iterative improvement of labeling functions to achieve optimal results.