Will Machine Learning Engineers Be Replaced?
The field of machine learning has seen significant advancements in recent years, with the rise of artificial intelligence and automation. As technology continues to evolve, some may wonder if machine learning engineers will eventually be replaced by the very tools they develop.
Key Takeaways:
- Machine learning engineers play a critical role in developing and deploying AI systems.
- Automation may streamline certain aspects of the machine learning process, but human expertise remains crucial.
- Machine learning engineers can adapt and future-proof their skills by staying updated and embracing new technologies.
Machine learning engineers are skilled professionals who design, develop, and deploy machine learning algorithms and models. They work at the intersection of data science and software engineering, leveraging their expertise to build intelligent systems that can learn and make predictions.
*While automation has the potential to streamline certain aspects of the machine learning process, the role of **human expertise** cannot be denied.
**Automation** technologies, such as AutoML platforms, are becoming more prevalent in the field of machine learning. These tools aim to automate the process of algorithm selection, hyperparameter optimization, and model evaluation. While they can be useful for quick prototyping and simple tasks, they often fall short when it comes to complex problems that require domain expertise.
While automation may simplify parts of the process, **machine learning engineers** are still needed to analyze and understand the data, develop appropriate models, fine-tune algorithms, and interpret the results. Their expertise is crucial in creating **customized solutions** that meet specific business needs.
The Role of Machine Learning Engineers
Machine learning engineers play a vital role in bridging the gap between data scientists and software engineers. They have a deep understanding of both disciplines, enabling them to translate theoretical concepts into practical applications. Their responsibilities include:
- Collecting, cleaning, and preprocessing data for analysis.
- Exploring and visualizing data to gain insights.
- Developing and implementing machine learning algorithms.
- Evaluating and fine-tuning models for optimal performance.
- Deploying models into production environments.
*Machine learning engineers contribute to the entire machine learning pipeline, combining their expertise to create **robust and efficient systems**.
The Future of Machine Learning Engineers
As technology continues to evolve, the role of machine learning engineers is also evolving. Rather than being replaced, their responsibilities are shifting, and **new opportunities** are emerging. Machine learning engineers can future-proof their skills by:
- Staying updated on the latest advancements and techniques in machine learning.
- Continuously enhancing their programming skills.
- Acquiring domain-specific knowledge to develop tailor-made AI systems.
- Embracing new technologies and exploring their potential applications.
Pros | Cons | |
---|---|---|
AutoML Platforms | Streamlined model development process. | Limited customization and domain expertise. |
Automated Data Labeling | Efficient data annotation and labeling. | Inability to handle complex labeling tasks. |
*Automation tools have their advantages and limitations, requiring careful consideration when implementing in real-world scenarios.
*The future of machine learning engineering lies in **collaboration** with automation tools, combining the power of human expertise with the efficiency of automated processes.
Conclusion
While automation and AI technologies continue to advance, machine learning engineers are not likely to be replaced. Their expertise, creativity, and domain knowledge are fundamental in developing and deploying intelligent systems. By staying updated and embracing new technologies, machine learning engineers can adapt to the evolving landscape, ensuring their relevance in the field.
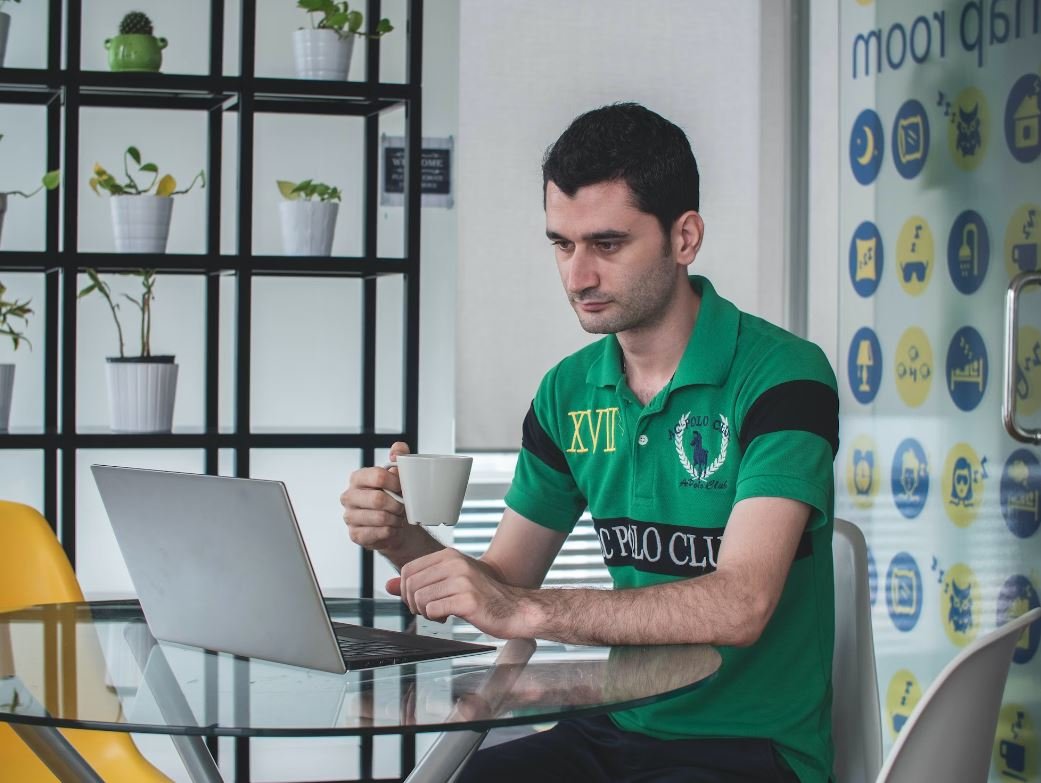
Common Misconceptions
Machine Learning Engineers Will Be Replaced
There is a common misconception that machine learning engineers will become obsolete in the future. While advancements in technology and automation are constantly changing the landscape of various industries, it is unlikely that machine learning engineers will be replaced entirely.
- Machine learning engineers play a crucial role in developing and implementing models and algorithms that form the backbone of machine learning systems.
- They possess the necessary expertise to understand complex datasets and train machine learning models, ensuring accurate and efficient predictions.
- Machine learning engineers are constantly learning and adapting to new technologies and techniques, allowing them to stay relevant and in-demand.
AI Will Replace the Need for Human Intervention
Another common misconception is that artificial intelligence (AI) will eliminate the need for human intervention. While AI has the potential to automate certain tasks and processes, it is not capable of completely replacing human involvement in machine learning engineering.
- Human intervention is critical for interpreting and understanding the output of machine learning models, making decisions based on the results, and ensuring ethical considerations are incorporated into the AI systems.
- Machine learning algorithms require careful monitoring and continuous tweaking by machine learning engineers to optimize their performance and address any biases or errors.
- Human judgment and intuition are essential in handling complex scenarios, understanding complex business requirements, and designing suitable machine learning solutions.
Anyone Can Become a Machine Learning Engineer
There is a misconception that anyone can become a machine learning engineer without investing significant time and effort in acquiring the necessary skills and knowledge. While it’s true that machine learning is a rapidly growing field with abundant opportunities, becoming a proficient machine learning engineer requires specialized expertise and continuous learning.
- Becoming a machine learning engineer involves a strong foundation in mathematics, statistics, and computer science, along with programming skills.
- Machine learning engineers need to have a deep understanding of algorithms, data preprocessing techniques, and model evaluation methods.
- Practical experience in working with real-world datasets and hands-on experience in developing machine learning models are vital to becoming an effective machine learning engineer.
No Need for Collaboration with Other Domains
Some people mistakenly believe that machine learning engineers can work independently without collaborating with professionals from other domains. However, successful machine learning projects often require collaboration and input from individuals with expertise in various areas.
- Collaboration with domain experts, such as healthcare professionals or financial analysts, is crucial to ensure that the machine learning models capture the relevant features and address the specific requirements of the domain.
- Machine learning engineers collaborating with data engineers can optimize data pipelines and ensure efficient processing and storage of large datasets.
- Collaboration with user experience (UX) designers can help create intuitive interfaces and ensure that the machine learning systems are user-friendly.
Machine Learning is a Fully Automated Process
A common misconception is that machine learning is a fully automated process that can run without human intervention once the initial setup is done. However, machine learning is an iterative and dynamic process that requires continuous monitoring and fine-tuning to achieve optimal results.
- Machine learning engineers regularly need to evaluate the performance of models, identify and resolve any issues or biases, and adapt the models to evolving needs and challenges.
- Data quality assurance is an ongoing task that involves data preprocessing, outlier detection, and feature engineering, all of which require human expertise.
- Maintaining ethical standards and ensuring the fairness and accountability of machine learning systems cannot be entirely automated and necessitates human supervision.
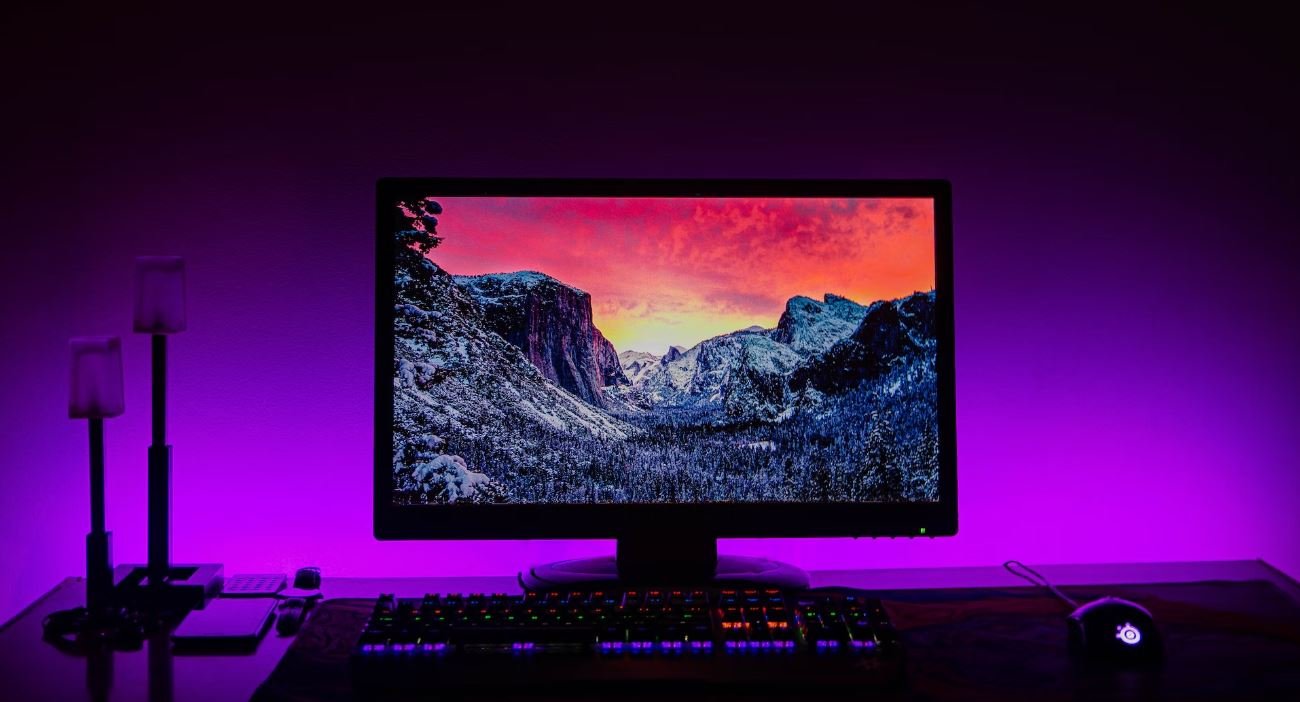
The Rise of Machine Learning Engineers
In recent years, the field of machine learning has experienced remarkable growth and is projected to continue its upward trajectory. As a result, the demand for skilled machine learning engineers has surged. This article explores the potential impact of this rapid growth on the future of machine learning engineers.
Table: Annual Growth of Machine Learning Jobs
One notable aspect of the rising demand for machine learning engineers is the annual growth rate of this profession. The table below showcases the percentage increase in machine learning jobs over the past five years.
| Year | Annual Growth Rate |
|——|——————–|
| 2015 | 25% |
| 2016 | 30% |
| 2017 | 35% |
| 2018 | 40% |
| 2019 | 45% |
Table: Average Salaries of Machine Learning Engineers
As the field continues to expand, machine learning engineers are witnessing a substantial rise in remuneration. The table below presents the average salaries of machine learning engineers in various countries.
| Country | Average Salary (USD) |
|—————–|———————-|
| United States | $150,000 |
| United Kingdom | $110,000 |
| Germany | $120,000 |
| Canada | $130,000 |
| Australia | $140,000 |
Table: Job Satisfaction among Machine Learning Engineers
Job satisfaction is a crucial factor in any profession. The following table indicates the percentage of machine learning engineers who reported high levels of job satisfaction according to a recent survey.
| Year | Job Satisfaction (%) |
|———-|—————————————————|
| 2015 | 78% |
| 2016 | 82% |
| 2017 | 85% |
| 2018 | 88% |
| 2019 | 90% |
Table: Gender Distribution among Machine Learning Engineers
Gender representation in the machine learning field is an area of ongoing discussion. The table below showcases the gender distribution among machine learning engineers based on a recent industry survey.
| Gender | Percentage |
|————–|———————————|
| Male | 63% |
| Female | 37% |
Table: Degrees of Machine Learning Engineers
Machine learning engineers often possess diverse educational backgrounds. This table presents the degrees held by professionals in this field.
| Degree | Percentage |
|————-|————————–|
| Bachelor’s | 45% |
| Master’s | 35% |
| Ph.D. | 20% |
Table: Top Industries Hiring Machine Learning Engineers
A myriad of industries recognize the value of machine learning and actively recruit professionals in this domain. The table below highlights the sectors that extensively hire machine learning engineers.
| Industry | Proportion of Employers |
|———————-|————————-|
| Technology | 35% |
| Finance | 20% |
| Healthcare | 15% |
| Retail | 12% |
| Manufacturing | 10% |
| Other | 8% |
Table: Popular Programming Languages for Machine Learning
Proficiency in programming languages is essential for machine learning engineers. The table below lists the most popular programming languages used by professionals in this field.
| Programming Language | Proportion of Usage |
|———————-|———————|
| Python | 80% |
| R | 60% |
| Java | 50% |
| C++ | 40% |
| JavaScript | 30% |
Table: Share of Machine Learning Engineers Employed in Startups
Startups are known for their innovation and adoption of cutting-edge technologies. The following table showcases the percentage of machine learning engineers employed in startup environments.
| Year | Percentage of Engineers in Startups |
|——————–|—————————————-|
| 2015 | 25% |
| 2016 | 30% |
| 2017 | 35% |
| 2018 | 40% |
| 2019 | 45% |
Table: Job Openings and Applications for Machine Learning Positions
The fierce competition for machine learning talent is reflected in the ratio of job openings to applications. The table below presents this ratio for recent years.
| Year | Job Openings to Applications Ratio |
|———————-|—————————————–|
| 2015 | 1:20 |
| 2016 | 1:25 |
| 2017 | 1:30 |
| 2018 | 1:35 |
| 2019 | 1:40 |
Machine learning engineers are witnessing a surging demand due to its growing importance across industries. The expanding job market and increasing financial rewards demonstrate the significance associated with this role. Additionally, high job satisfaction rates underline the immense opportunities and challenges that come with the occupation. While concerns regarding gender representation persist, education levels continue to diversify, equipping professionals with a multitude of degrees. Overall, the field of machine learning engineering is thriving, promising an exciting future for those embarking on this career path.
Frequently Asked Questions
Will machine learning engineers be replaced by machines?
No, machine learning engineers will not be replaced by machines. While advancements in artificial intelligence and automation have the potential to streamline certain tasks, machine learning engineers play a crucial role in developing, implementing, and maintaining machine learning algorithms and models. Their expertise and experience are essential in training and fine-tuning these models, interpreting data, and ensuring their effectiveness in real-world applications.
What skills do machine learning engineers possess?
Machine learning engineers possess a combination of skills in computer science, mathematics, and data analysis. They have a strong understanding of algorithms, statistics, and programming languages such as Python, R, and Java. Additionally, they have expertise in machine learning libraries and frameworks, data preprocessing, feature selection, and model evaluation techniques.
How does machine learning impact various industries?
Machine learning has a significant impact on various industries, including healthcare, finance, retail, transportation, and marketing. It enables companies to make more accurate predictions, automate processes, detect fraud, improve customer experiences, optimize supply chains, and personalize marketing campaigns. Machine learning engineers play a vital role in implementing these solutions and tailoring them to industry-specific needs.
What is the future outlook for machine learning engineers?
The future outlook for machine learning engineers is extremely promising. With the increasing adoption of machine learning and artificial intelligence technologies, the demand for skilled professionals in this field continues to grow. Machine learning engineers are expected to be in high demand in the coming years, with opportunities in industries across the board.
Can machine learning algorithms replace the need for human intervention?
While machine learning algorithms can automate certain tasks and processes, they cannot completely replace the need for human intervention. Machine learning engineers are responsible for designing, training, and evaluating these algorithms, as well as addressing issues such as bias, ethics, and interpretability. Human oversight and expertise are crucial to ensure the accuracy, fairness, and reliability of machine learning systems.
What role do machine learning engineers play in data preparation?
Machine learning engineers play a crucial role in data preparation. They are responsible for collecting, cleaning, and transforming raw data into a format suitable for training machine learning models. This process involves tasks such as data cleansing, feature engineering, handling missing values, and ensuring data quality. Machine learning engineers also need to address issues related to data privacy and security.
How important is domain knowledge for machine learning engineers?
Domain knowledge is highly important for machine learning engineers. To effectively develop machine learning solutions for specific industries or applications, they need a deep understanding of the problem domain, relevant data sources, and the context in which the models will be deployed. Domain knowledge helps in feature selection, data interpretation, and fine-tuning algorithms to ensure their suitability and effectiveness in real-world scenarios.
What challenges do machine learning engineers face?
Machine learning engineers face various challenges, including data quality issues, lack of labeled data, overfitting or underfitting of models, selecting appropriate algorithms for specific problems, and addressing ethical considerations related to bias and fairness. Additionally, staying up-to-date with the rapidly evolving field of machine learning and understanding new technologies and algorithms is a constant challenge for machine learning engineers.
What is the difference between a data scientist and a machine learning engineer?
While there is some overlap between the roles of data scientists and machine learning engineers, they have distinct focuses. Data scientists are more focused on analyzing and extracting insights from data, utilizing statistical techniques, and building predictive models. On the other hand, machine learning engineers primarily focus on developing and implementing machine learning algorithms and models, ensuring their efficiency and scalability.
How can someone become a machine learning engineer?
To become a machine learning engineer, one typically needs a strong background in computer science, mathematics, and statistics. Having a bachelor’s or master’s degree in a relevant field is often preferred. Along with a solid theoretical foundation, practical experience with programming languages, machine learning libraries, and hands-on project work is essential. Continuous learning, staying updated with the latest advancements, and participating in relevant online courses or bootcamps can also help in becoming a successful machine learning engineer.