Data Analysis Applications
Data analysis has become an essential component in various industries, allowing businesses to make data-driven decisions and gain valuable insights. Whether it’s analyzing customer behavior, optimizing marketing strategies, or predicting market trends, data analysis applications play a crucial role in gathering, interpreting, and visualizing data.
Key Takeaways:
- Data analysis applications help businesses make data-driven decisions and gain insights.
- They assist in analyzing customer behavior, optimizing marketing strategies, and predicting market trends.
- Data analysis applications enable data gathering, interpretation, and visualization.
The Power of Data Analysis
Data analysis applications provide businesses with the power to harness the abundance of data available to them, helping them make informed decisions and improve their processes.
*Data analysis can uncover patterns and trends that may not be immediately apparent to humans.
The Applications of Data Analysis
Data analysis finds applications in various industries and areas:
- 1. **Finance**: Data analysis is used to identify investment opportunities, assess risks, and detect fraudulent activities.
- 2. **Healthcare**: It aids in patient diagnosis, disease outbreak monitoring, and optimizing treatment plans.
- 3. **E-commerce**: Data analysis helps businesses understand customer behavior, personalize recommendations, and optimize pricing strategies.
- 4. **Marketing**: It enables businesses to evaluate campaign performance, segment audiences, and identify target markets.
- 5. **Supply Chain**: Data analysis optimizes inventory management, tracks shipments, and eliminates bottlenecks.
Data Analysis Techniques and Tools
There are various techniques and tools used in data analysis:
- 1. **Descriptive Analysis**: Summarizes and describes the main features of a dataset, providing initial insights.
- 2. **Predictive Analysis**: Uses historical data to make predictions and forecasts about future outcomes.
- 3. **Prescriptive Analysis**: Suggests optimal solutions and actions based on data analysis findings.
- 4. **Machine Learning**: Utilizes algorithms to enable systems to learn and improve from data without explicit programming.
Data Analysis in Action
Let’s explore some real-world examples of data analysis applications:
Example | Industry | Outcome |
---|---|---|
1 | Finance | Identifying fraudulent transactions to protect customers. |
2 | Marketing | Segmenting customers for targeted advertising campaigns. |
Example | Industry | Outcome |
---|---|---|
3 | Healthcare | Identifying patterns in patient data to improve diagnosis accuracy. |
4 | E-commerce | Optimizing pricing strategies based on customer purchasing behavior. |
Example | Industry | Outcome |
---|---|---|
5 | Supply Chain | Reducing inventory costs by optimizing reorder points. |
Bringing It All Together
Data analysis applications have transformed various industries, empowering businesses to harness the power of data and make better decisions. By utilizing data analysis techniques and tools, businesses can uncover valuable insights, optimize processes, and stay ahead of the competition.
*The possibilities for data analysis applications are extensive and continually evolving, as businesses recognize the importance of leveraging data in their strategies.
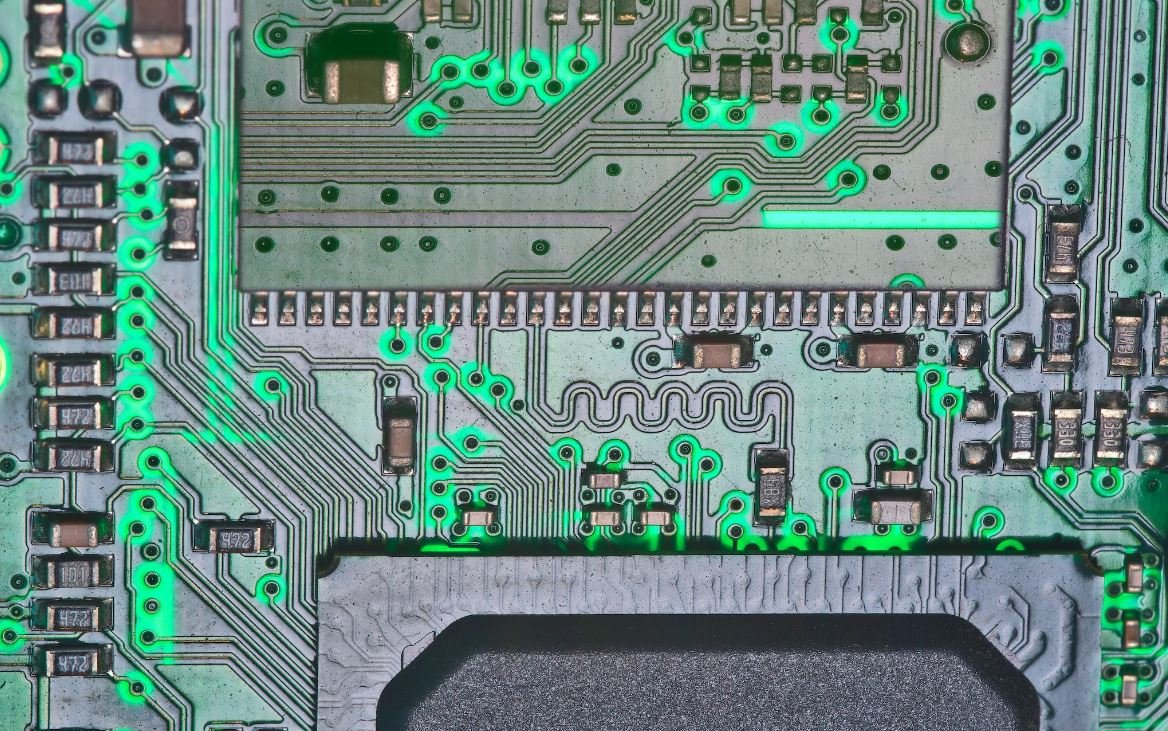
Common Misconceptions
Misconception 1: Data Analysis Applications are only for experts
One common misconception about data analysis applications is that they are too complex and can only be used by experts in the field. However, many data analysis tools and applications have been designed with user-friendly interfaces, making it easier for non-experts to utilize them.
- Data analysis applications often offer intuitive drag-and-drop features that allow users to easily manipulate and analyze data without coding knowledge.
- Online tutorials and support communities are available to help beginners learn how to use data analysis applications effectively.
- Many data analysis applications offer pre-built templates and workflows, allowing users to get started quickly without having to build everything from scratch.
Misconception 2: Data Analysis Applications can only handle small data
Another common misconception is that data analysis applications are limited to processing small amounts of data and cannot handle large datasets. However, many modern data analysis applications are equipped with advanced processing capabilities that can handle massive volumes of data.
- Data storage and processing technologies such as distributed computing and cloud storage enable data analysis applications to scale and handle big data efficiently.
- Data analysis applications often provide features like data sampling and filtering to focus on specific subsets of large datasets, improving performance and analysis speed.
- Furthermore, data analysis applications can leverage parallel processing and distributed computing to perform computations across multiple machines, making it possible to analyze large datasets in a reasonable time frame.
Misconception 3: Data Analysis Applications always provide accurate results
One misconception is that data analysis applications always produce accurate results. While data analysis applications are powerful tools, the accuracy of the results heavily depends on the quality and integrity of the data used for analysis.
- Data analysis applications may not be able to detect errors in the input data, so it is crucial for users to ensure the data they are working with is accurate and reliable.
- Data cleaning and validation processes should be applied before analysis to minimize the risk of errors and biases in the results.
- It is essential to interpret the results of data analysis critically, taking into account potential limitations and assumptions made during the analysis.
Misconception 4: Data Analysis Applications are only used in business settings
Another common misconception is that data analysis applications are only used in business settings. While businesses often utilize data analysis for decision-making and forecasting, data analysis applications are valuable in various fields outside of the business world.
- In academia, data analysis applications are used in scientific research to analyze experimental results, perform statistical analyses, and identify patterns in data.
- In healthcare, data analysis applications are used to analyze patient data, identify trends in public health data, and support medical research.
- In government and public administration, data analysis applications are used to analyze census data, track and analyze crime statistics, and inform policy decisions.
Misconception 5: Data Analysis Applications eliminate the need for human expertise
A common misconception is that data analysis applications eliminate the need for human expertise in data analysis. While data analysis applications can automate certain processes, human expertise and critical thinking are still essential for effective analysis.
- Data analysis applications can provide insights and identify patterns, but it is up to human analysts to interpret and contextualize those findings.
- Human experts are vital for formulating appropriate research questions, selecting relevant data variables, and ensuring the accuracy and validity of the results.
- Data analysis applications can enhance human decision-making, but they should be seen as tools that complement human expertise rather than replace it entirely.
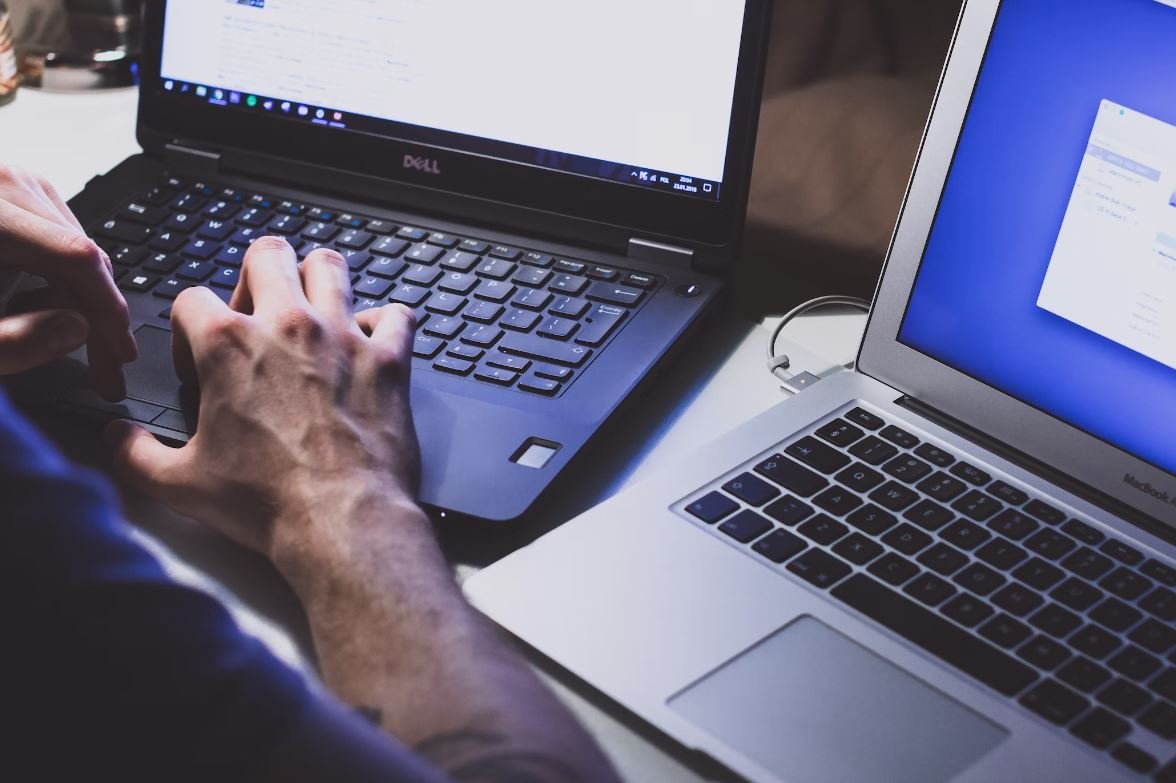
Data Analysis Applications in Various Industries
Data analysis has become an essential tool in today’s data-driven world. In this article, we explore 10 real-life examples of how data analysis is applied across different industries. These tables showcase the impact of data analysis and provide interesting insights into the applications of this powerful technique.
Stock Market Analysis: Top Performers in the S&P 500
This table presents the top 10 performing companies in the S&P 500 for the year 2020. By analyzing stock market data, investors can identify trends and make informed investment decisions.
Company | Industry | Percentage Return |
---|---|---|
Amazon | E-commerce | 73.1% |
Tesla | Automotive | 695.0% |
Zoom | Technology | 395.4% |
Peloton | Fitness | 470.0% |
Netflix | Streaming | 67.1% |
Apple | Technology | 82.0% |
Microsoft | Technology | 38.3% |
Alphabet | Technology | 31.5% |
Technology | 33.1% | |
Adobe | Software | 52.6% |
Customer Satisfaction Scores: Retail Chains
This table showcases the customer satisfaction scores of various retail chains, highlighting the importance of data analysis in understanding consumer preferences and improving the overall shopping experience.
Retail Chain | Customer Satisfaction Score |
---|---|
Costco | 4.8/5 |
Amazon | 4.7/5 |
Walmart | 4.5/5 |
Target | 4.4/5 |
Kroger | 4.3/5 |
Energy Consumption: Residential vs. Commercial Buildings
This table compares the energy consumption patterns of residential and commercial buildings, emphasizing the importance of data analysis in identifying energy-saving opportunities and promoting sustainability.
Building Type | Average Monthly Energy Consumption (kWh) |
---|---|
Residential | 850 |
Commercial | 2,500 |
Mobile App Downloads: Popular Categories
This table illustrates the number of mobile app downloads for different app categories, indicating the significance of data analysis in understanding user preferences and targeting specific markets.
App Category | Number of Downloads (in millions) |
---|---|
Social Media | 2,100 |
Productivity | 1,800 |
Entertainment | 1,500 |
Games | 1,200 |
Health & Fitness | 950 |
Crime Rates: Major U.S. Cities
This table showcases the crime rates in major U.S. cities, highlighting the role of data analysis in identifying crime patterns and assisting law enforcement in proactive decision-making.
City | Crime Rate per 100,000 People |
---|---|
New York City | 580 |
Los Angeles | 450 |
Chicago | 700 |
Houston | 400 |
Miami | 350 |
Sales Performance: Regional Comparison
This table demonstrates the sales performance of different regions, emphasizing the role of data analysis in evaluating market trends, identifying growth opportunities, and optimizing sales strategies.
Region | Total Sales (in millions) |
---|---|
North America | $800 |
Europe | $600 |
Asia | $450 |
South America | $250 |
Africa | $150 |
Customer Churn Rate: Telecommunication Companies
This table displays the customer churn rate for various telecommunication companies, highlighting the value of data analysis in tracking customer behavior, improving customer satisfaction, and reducing customer turnover.
Telecommunication Company | Churn Rate (%) |
---|---|
Verizon | 1.9% |
AT&T | 2.5% |
T-Mobile | 2.8% |
Sprint | 3.1% |
US Cellular | 2.2% |
Website Traffic: Monthly Visitors
This table showcases the monthly visitor count for different websites, underscoring the importance of data analysis in understanding user engagement, optimizing website performance, and driving business growth.
Website | Monthly Visitors (in millions) |
---|---|
6,200 | |
4,500 | |
YouTube | 3,800 |
Amazon | 2,300 |
1,900 |
Population Growth: Global Comparison
This table compares the population growth rates of different countries, highlighting the role of data analysis in predicting demographics, planning resources, and understanding global trends.
Country | Population Growth Rate (%) |
---|---|
India | 1.0% |
China | 0.4% |
Nigeria | 2.6% |
United States | 0.7% |
Brazil | 0.8% |
In this rapidly evolving digital era, data analysis applications have become integral across various industries. Through analyzing real data, businesses can make data-driven decisions, identify market trends, and uncover valuable insights. Whether it is optimizing financial investments, improving customer satisfaction, reducing energy consumption, or understanding population changes, data analysis empowers organizations to stay competitive and adapt to an ever-changing landscape. Embracing data analysis has become crucial for success and growth in the modern world.
Frequently Asked Questions
What is data analysis?
Data analysis is the process of inspecting, cleansing, transforming, and modeling data to discover useful information and make informed decisions. It involves analyzing large datasets to uncover patterns, trends, and correlations that can bring valuable insights to various industries.
How is data analysis used in business?
Data analysis is widely used in business to improve decision-making processes. It helps businesses identify key trends, predict future outcomes, optimize operations, and gain a competitive edge. By analyzing data, businesses can make data-driven decisions that lead to increased efficiency, reduced costs, and better customer satisfaction.
What are some common data analysis applications?
There are several common data analysis applications, including market research, customer segmentation, risk analysis, fraud detection, demand forecasting, and sentiment analysis. These applications can be used across industries such as finance, healthcare, marketing, and e-commerce to drive strategic decisions and improve business outcomes.
What tools are used in data analysis?
Various tools and technologies are used for data analysis. Some popular tools include statistical software like R and Python, data visualization tools like Tableau and Power BI, and database management systems like SQL. In addition, machine learning and artificial intelligence technologies are commonly used for advanced data analysis and predictive modeling.
What skills are needed for data analysis?
Data analysis requires a combination of technical and analytical skills. Proficiency in programming languages like R or Python is necessary to manipulate and analyze data. Statistical knowledge, data visualization skills, problem-solving abilities, and critical thinking are also important for effective data analysis. Good communication skills are essential to present findings and insights to stakeholders.
How does data analysis impact decision-making?
Data analysis plays a significant role in decision-making by providing actionable insights based on data patterns and trends. It reduces reliance on intuition and gut feelings and helps decision-makers make informed and evidence-based choices. Data analysis enhances the decision-making process by minimizing risks, identifying opportunities, and improving overall business performance.
What challenges are involved in data analysis?
Data analysis poses several challenges, including data quality issues, data privacy concerns, and the complexity of handling large datasets. Ensuring data accuracy and completeness, maintaining data security, and handling missing or inconsistent data are some of the common challenges faced during the data analysis process.
How can data analysis be used for predictive analytics?
Data analysis is an integral part of predictive analytics. By analyzing historical data and patterns, predictive models can be built to forecast future outcomes and trends. These models can be used to make predictions and inform decision-making in various fields, such as sales forecasting, demand planning, risk assessment, and customer behavior prediction.
What is the role of data visualization in data analysis?
Data visualization plays a crucial role in data analysis as it helps in presenting complex data in a visually appealing and understandable manner. Visualizations, such as charts, graphs, and dashboards, make it easier to identify patterns, trends, and outliers in data. They enable analysts and stakeholders to gain insights quickly and make data-driven decisions more effectively.
How is data analysis used in healthcare?
Data analysis is extensively used in healthcare for various purposes. It helps in improving efficiency, identifying disease patterns, predicting disease outbreaks, and personalizing patient care. Data analysis in healthcare can be used for clinical research, patient monitoring, disease surveillance, resource optimization, and decision support systems.