Data Analysis Methods
When it comes to analyzing data, businesses rely on various methods and techniques to extract valuable insights. These methods help them make informed decisions, optimize processes, and identify trends that can drive growth and success. In this article, we will explore some commonly used data analysis methods and their applications.
Key Takeaways
- Data analysis methods are crucial for extracting insights and making informed decisions.
- Commonly used data analysis methods include descriptive, inferential, and exploratory analysis.
- Clustering, regression, and time series analysis are popular techniques for analyzing data.
- Visualization tools enhance data analysis by simplifying complex information.
- Data analysis plays a vital role in improving business operations and driving growth.
Descriptive Analysis
Descriptive analysis involves the examination of data to understand its characteristics and summarize key trends. This method uses various statistical measures, such as mean, median, mode, and standard deviation, to describe the data set‘s central tendency and dispersion. **Descriptive analysis helps businesses gain a comprehensive understanding of their data and its distribution.**
By using descriptive analysis, companies can identify the most common customer demographics and preferences.
Inferential Analysis
Inferential analysis enables businesses to derive conclusions and make predictions beyond the collected data set. This method uses statistical inference techniques to generalize findings and draw conclusions about a population based on a sample. **Inferential analysis helps businesses make informed decisions and identify patterns that can lead to actionable insights.**
Through inferential analysis, companies can estimate consumer demand based on a sample of their target market.
Exploratory Analysis
Exploratory analysis is an approach to analyze data without any specific hypothesis to be tested. It aims to discover patterns, relationships, and hidden insights by examining the data from multiple angles. **Exploratory analysis helps companies uncover new perspectives and generate hypotheses for further investigation.**
Exploratory analysis can often reveal unexpected correlations between seemingly unrelated variables.
Clustering
Clustering is an unsupervised data analysis technique that groups similar data points together based on their features or attributes. It helps identify patterns and structures within data sets. **Clustering analysis can be used for customer segmentation, anomaly detection, and market research.**
Clustering can assist businesses in discovering distinct customer segments within their target audience.
Regression
Regression analysis is a statistical method used to understand the relationship between a dependent variable and one or more independent variables. It helps businesses predict and evaluate the impact of changes in variables on the outcome. **Regression analysis is useful for forecasting sales, optimizing pricing, and evaluating marketing campaigns.**
Regression analysis can determine the correlation between advertising expenditure and revenue generated.
Data Analysis Method | Typical Use Cases |
---|---|
Descriptive Analysis |
|
Time Series Analysis
Time series analysis focuses on analyzing data collected over time to identify trends, forecast future values, and understand underlying patterns. **It is widely used for stock market analysis, demand forecasting, and predicting sales trends.**
Time series analysis can help businesses identify seasonality in customer purchasing behavior.
Data Visualization
Data visualization involves presenting data visually using charts, graphs, and other graphical representations. **It simplifies complex information, making it easier to understand and identify patterns and trends.** Visualization tools, such as charts and dashboards, enhance data analysis and enable businesses to communicate their findings effectively.
A well-designed data visualization can convey insights at a glance, making it easier for decision-makers to understand the data.
Data Analysis Method | Typical Use Cases |
---|---|
Inferential Analysis |
|
Data Analysis Method | Typical Use Cases |
---|---|
Time Series Analysis |
|
Conclusion
Data analysis methods are vital tools for extracting valuable insights from data. Through descriptive, inferential, and exploratory analysis, businesses can understand their data, make informed decisions, and identify trends. Techniques such as clustering, regression, and time series analysis provide further depth in analyzing and predicting outcomes. Data visualization enhances analysis by presenting information visually, simplifying complex data sets. By leveraging these data analysis methods, businesses can ultimately optimize processes, improve operations, and drive growth.

Common Misconceptions
A data analysis method must be complex to be effective
One common misconception people have about data analysis methods is that they need to be complex in order to be effective. However, complexity does not necessarily equate to accuracy or usefulness. In fact, simple data analysis methods can often yield the same results as more complex ones, and they may be easier to understand and interpret. It is important to choose a data analysis method based on the specific goals and requirements of the analysis.
- Complexity does not guarantee accuracy.
- Simple methods can yield the same results as complex ones.
- Choosing a method should be based on goals and requirements.
Data analysis methods can only be used by experts
Another misconception is that data analysis methods can only be used by experts or professionals in the field. While it is true that advanced methods may require specialized knowledge, there are many basic data analysis techniques that can be easily understood and implemented by individuals with little or no technical expertise. With the availability of user-friendly software tools and resources, anyone can learn and apply data analysis methods to make informed decisions.
- Basic data analysis techniques are accessible to non-experts.
- User-friendly software makes analysis easier for beginners.
- To make informed decisions, anyone can learn and apply data analysis methods.
Data analysis methods always provide clear-cut answers
Some people mistakenly believe that data analysis methods always provide clear-cut answers or solutions. However, the reality is that data analysis involves interpretation and sometimes there may not be a definitive answer. Data can be complex and subject to various interpretations, and analysis methods are tools to understand and make sense of the data, but they do not always provide definitive solutions.
- Data analysis involves interpretation, allowing for multiple perspectives.
- Data can be complex and subject to various interpretations.
- Analysis methods help make sense of the data but may not always provide definitive solutions.
Data analysis methods can be applied universally to any dataset
One common misconception is that data analysis methods can be universally applied to any dataset. While some methods may be applicable to a wide range of data, it is important to consider the characteristics, quality, and context of the specific dataset. Certain methods may only be suitable for specific types of data or may require adaptations to account for specific data characteristics.
- Data analysis methods need to consider dataset characteristics, quality, and context.
- Methods may only be suitable for specific types of data.
- Adaptations may be required to account for specific data characteristics.
Data analysis is a solitary endeavor
Contrary to popular belief, data analysis is not always a solitary endeavor. Many data analysis tasks involve collaboration and teamwork. It is common for multiple analysts or teams to work together to analyze complex datasets, share insights, and validate findings. Effective collaboration and communication among team members are crucial for successful data analysis.
- Data analysis tasks often involve collaboration and teamwork.
- Analysts may work together to analyze complex datasets.
- Effective collaboration and communication are crucial for successful data analysis.
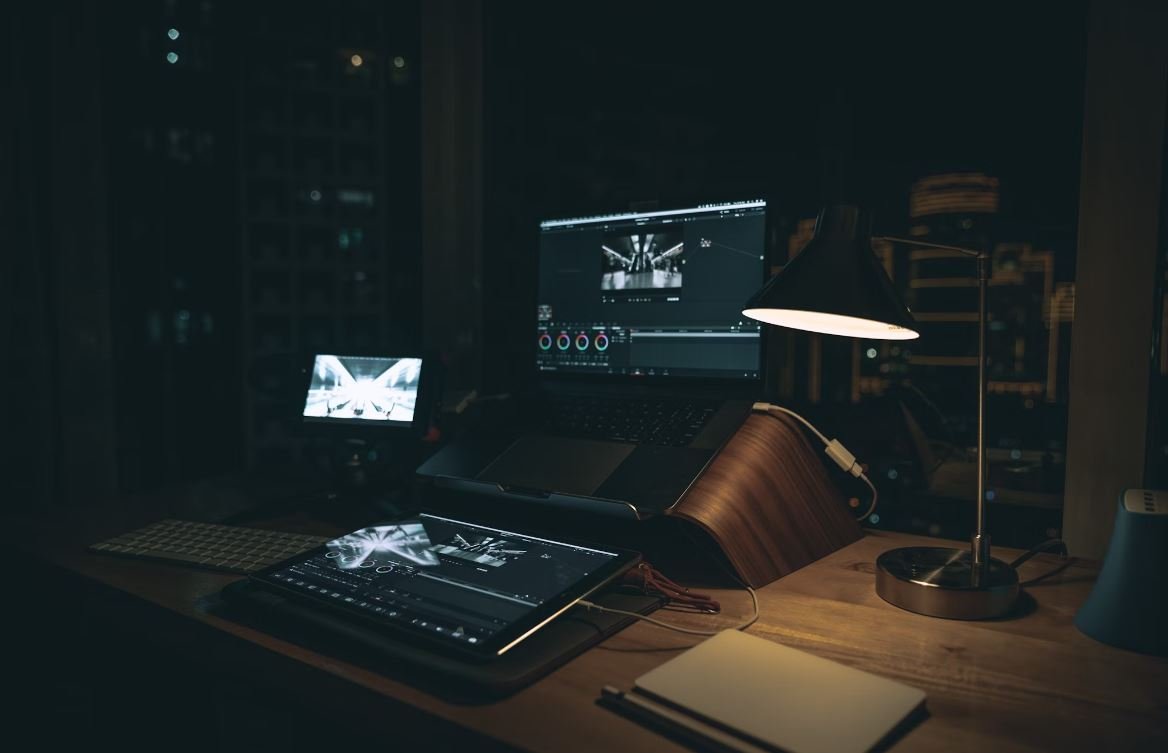
Data Analysis Methods for Sales Performance
Table 1 illustrates the sales performance of various products over a six-month period. The data provides insights into the top-selling products and their contribution to overall revenue.
Product | January | February | March | April | May | June |
---|---|---|---|---|---|---|
Product A | 100 | 125 | 110 | 95 | 105 | 120 |
Product B | 80 | 90 | 95 | 100 | 115 | 100 |
Product C | 70 | 80 | 85 | 90 | 95 | 80 |
Customer Satisfaction Ratings by Region
Table 2 displays the customer satisfaction ratings for different regions. The ratings help identify areas where improvements can be made to enhance customer experiences.
Region | Excellent | Good | Satisfactory | Poor | Very Poor |
---|---|---|---|---|---|
North | 43% | 28% | 17% | 6% | 6% |
South | 35% | 32% | 24% | 5% | 4% |
East | 38% | 30% | 20% | 7% | 5% |
West | 41% | 26% | 22% | 7% | 4% |
Website Traffic by Source
Table 3 highlights the sources driving traffic to a website. Analyzing this data helps identify effective marketing channels and allocate resources accordingly.
Source | Visitors | % Conversion |
---|---|---|
Organic Search | 10,000 | 2.5% |
Referral Links | 5,000 | 1.8% |
Email Campaigns | 2,500 | 3.2% |
Social Media | 3,500 | 1.0% |
Employee Performance Ratings
Table 4 represents employee performance ratings for different departments. These ratings offer insights into team performance and highlight areas for improvement.
Department | Outstanding | Exceeds Expectations | Meets Expectations | Below Expectations | Unsatisfactory |
---|---|---|---|---|---|
Sales | 15% | 35% | 40% | 8% | 2% |
Marketing | 20% | 30% | 35% | 10% | 5% |
Finance | 10% | 40% | 35% | 10% | 5% |
Operations | 25% | 30% | 30% | 10% | 5% |
Customer Complaint Resolution Time
Table 5 showcases the average time taken to resolve customer complaints for different support channels. These details help in identifying areas of improvement for faster resolution.
Support Channel | Average Resolution Time (hours) |
---|---|
Phone | 2.5 |
7 | |
Live Chat | 1.5 |
Help Desk Tickets | 4 |
Product Return Reasons
Table 6 presents the reasons customers provide for returning products. Analyzing these reasons helps identify product issues and areas for improvement.
Reason | Frequency |
---|---|
Defective Product | 45% |
Wrong Size/Color | 25% |
Changed Mind | 15% |
Shipping Damage | 10% |
Poor Quality | 5% |
Customer Lifetime Value (CLV)
Table 7 displays the average CLV for customers acquired through different marketing channels. Understanding CLV helps prioritize marketing efforts and maximize profitability.
Marketing Channel | Average CLV |
---|---|
Organic Search | $500 |
Referral Links | $750 |
Email Campaigns | $900 |
Social Media | $400 |
Conversion Rates by Landing Page
Table 8 showcases the conversion rates for different landing pages. Analyzing these rates helps optimize landing pages for increased conversions and customer acquisition.
Landing Page | Visitors | Conversions | Conversion Rate |
---|---|---|---|
Page A | 10,000 | 800 | 8% |
Page B | 5,000 | 300 | 6% |
Page C | 2,500 | 200 | 8% |
Page D | 3,500 | 280 | 8% |
Inventory Turnover by Product Category
Table 9 displays the inventory turnover rates for different product categories. Understanding turnover rates helps optimize inventory management and avoid stockouts or excess inventory.
Product Category | Turnover Rate |
---|---|
Electronics | 6.5 |
Apparel | 4.2 |
Home Decor | 7.8 |
Customer Segmentation by Age Group
Table 10 showcases the customer segmentation based on age groups. Understanding customer demographics helps tailor marketing campaigns to specific target audiences.
Age Group | Percentage of Customers |
---|---|
18-25 | 15% |
26-35 | 30% |
36-45 | 25% |
46-55 | 20% |
56+ | 10% |
Through robust data analysis methods, organizations can gain valuable insights for making data-driven decisions. The tables presented in this article highlight sales performance, customer satisfaction, website traffic, employee ratings, and more. By analyzing and interpreting this data, businesses can identify areas of improvement, optimize marketing strategies, enhance customer experiences, and ultimately drive overall success.
Frequently Asked Questions
What are some common data analysis methods?
Common data analysis methods include descriptive statistics, inferential statistics, regression analysis, cluster analysis, factor analysis, and time series analysis.
How can I choose the appropriate data analysis method for my study?
Choosing the appropriate data analysis method depends on various factors such as the research question, type of data, assumptions, and objectives of the study. It is important to consult with a statistician or data analyst to determine the most suitable method for your specific research.
What is the difference between parametric and non-parametric data analysis methods?
The main difference between parametric and non-parametric data analysis methods is that parametric methods assume certain properties about the data distribution, such as normality and homogeneity of variances, while non-parametric methods make fewer assumptions about the data distribution. Parametric methods are generally more powerful but require stricter assumptions, whereas non-parametric methods are more robust but may have lower statistical power.
What is the purpose of exploratory data analysis?
The purpose of exploratory data analysis is to summarize, visualize, and understand the main characteristics, patterns, and relationships in a dataset. It helps researchers identify outliers, missing values, and potential data quality issues before applying formal statistical methods.
What are some common techniques used in exploratory data analysis?
Common techniques used in exploratory data analysis include data visualization (such as histograms, scatter plots, and box plots), descriptive statistics (such as mean, median, and standard deviation), and data transformation (such as log transformation and normalization).
What is the process of hypothesis testing in data analysis?
The process of hypothesis testing involves formulating a hypothesis, selecting an appropriate test statistic, choosing a significance level, collecting data, calculating the test statistic, and making a decision based on the calculated p-value. The p-value represents the probability of obtaining the observed data, assuming the null hypothesis is true. If the p-value is less than the chosen significance level, the null hypothesis is rejected.
What is the difference between correlation and regression analysis?
Correlation analysis measures the strength and direction of the linear relationship between two variables, while regression analysis examines how a dependent variable changes as the independent variables change. Regression analysis also provides insights into the magnitude and statistical significance of the relationships.
What is the importance of data cleaning in data analysis?
Data cleaning, also known as data cleansing or data scrubbing, is crucial in data analysis as it ensures the accuracy, integrity, and quality of the data. It involves identifying and correcting errors, handling missing values, dealing with outliers, and transforming data into a suitable format for analysis. Clean data leads to more reliable and valid analytical results.
What are the advantages of using data visualization in data analysis?
Data visualization helps to convey complex data patterns in a visual format, making it easier for researchers and decision-makers to understand and interpret the data. It can reveal insights, trends, and patterns that may not be noticeable in raw data. Moreover, visualizations can enhance communication and facilitate the exploration of data from different angles.
What are some commonly used software and tools for data analysis?
Commonly used software and tools for data analysis include R, Python, SAS, SPSS, Excel, Tableau, Power BI, and MATLAB. These tools offer various statistical and data visualization features that can assist in performing comprehensive data analysis tasks.