Data Mining AI
With the advancements in artificial intelligence (AI), data mining has emerged as a powerful method for extracting valuable insights and patterns from large datasets. In today’s data-driven world, businesses and organizations can leverage data mining AI techniques to enhance decision-making, improve operational efficiency, and gain a competitive edge. This article dives deep into data mining AI, its key concepts, applications, and benefits.
Key Takeaways:
- Data mining AI utilizes artificial intelligence algorithms to extract meaningful patterns and insights from large datasets.
- Applications of data mining AI include fraud detection, customer segmentation, personalized recommendations, and predictive analytics.
- Data mining AI can help businesses make more informed decisions, optimize processes, and improve customer satisfaction.
Data mining AI, also known as machine learning or predictive analytics, is a branch of AI that focuses on extracting valuable insights and patterns from large datasets. By utilizing advanced algorithms, data mining AI can identify hidden patterns and relationships that may not be apparent to humans. These patterns can then be used to make predictions, optimize processes, and drive decision-making.
*Data mining AI has the ability to process and analyze vast amounts of data in a fraction of the time it would take for a human analyst to do so.*
One of the key benefits of data mining AI is its ability to provide accurate and actionable predictions. By analyzing historical data, data mining AI algorithms can identify trends and patterns that can be used to make predictions about future outcomes. This predictive power allows businesses to anticipate customer behavior, optimize inventory management, and make informed strategic decisions.
*The use of data mining AI can lead to significant cost savings by optimizing processes, reducing waste, and improving operational efficiency.*
Data Mining AI Applications
Data mining AI is widely used across various industries and sectors. Here are some of its key applications:
- Fraud detection: Data mining AI can analyze large volumes of transactional data to identify patterns that indicate fraudulent activities.
- Customer segmentation: By analyzing customer data, data mining AI can identify different customer segments and tailor marketing strategies accordingly.
- Personalized recommendations: Data mining AI algorithms can analyze user preferences and behavior to provide personalized recommendations for products, services, or content.
- Predictive analytics: Data mining AI can analyze historical data to predict future outcomes, such as consumer buying trends or equipment failure.
Data Mining AI Benefits
Implementing data mining AI techniques can yield numerous benefits for businesses and organizations:
- Improved decision-making: Data mining AI provides insights and predictions that support informed decision-making.
- Enhanced customer satisfaction: Personalized recommendations and tailored marketing strategies can improve customer experience and satisfaction.
- Cost savings: By optimizing processes and identifying inefficiencies, data mining AI can help reduce costs and improve operational efficiency.
- Competitive advantage: Utilizing data mining AI techniques can give businesses a competitive edge by uncovering insights that their competitors may miss.
Data Mining AI in Action
Let’s take a look at some real-world examples of data mining AI applications:
Industry | Application |
---|---|
Retail | Customer segmentation for targeted marketing campaigns |
Finance | Fraud detection and prevention |
*Data mining AI has revolutionized the retail industry by enabling personalized recommendations and targeted marketing campaigns.*
Benefits |
---|
Improved decision-making |
Enhanced customer satisfaction |
Cost savings |
Data mining AI has numerous benefits across various sectors, including finance, healthcare, and manufacturing. Its applications range from fraud detection and predictive analytics to personalized marketing strategies and customer segmentation.
Data Mining AI Challenges
While data mining AI offers immense potential, it also comes with its fair share of challenges:
- Data privacy concerns: The extraction and analysis of large datasets raise privacy concerns and ethical considerations.
- Data quality and preprocessing: Ensuring data accuracy, completeness, and relevance are essential for obtaining reliable results.
- Interpretability and transparency: AI algorithms can be complex, making it difficult to interpret and understand the reasoning behind their predictions.
Conclusion
Data mining AI is a powerful tool that has transformed how businesses extract insights and make decisions from large datasets. By utilizing advanced algorithms, data mining AI can uncover hidden patterns and relationships that pave the way for more informed decision-making and enhanced operational efficiency. As AI technology continues to evolve, we can expect even more exciting applications and advances in the field of data mining.
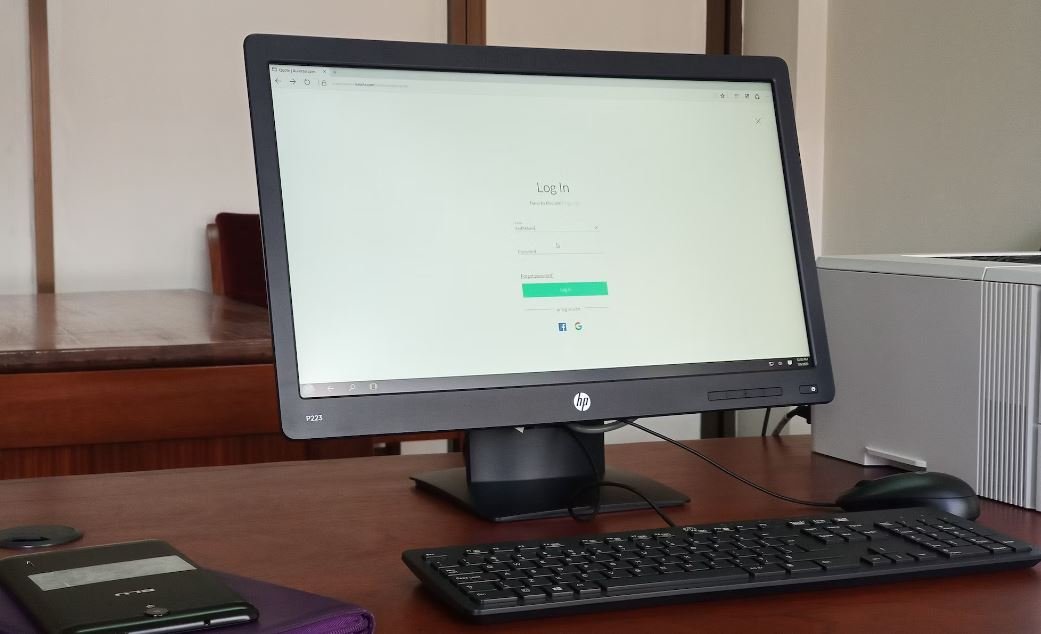
Common Misconceptions
The AI in Data Mining is “magical” and can solve any problem
One common misconception about AI in data mining is that it possesses magical abilities and can solve any problem put in front of it. In reality, AI algorithms are designed to analyze and interpret data, but they are not infallible or capable of solving all problems.
- AIs in data mining have limitations and cannot solve every kind of problem.
- AI algorithms require high-quality and relevant data for accurate results.
- Human expertise is still essential to interpret the insights provided by AI algorithms.
Data mining AI always produces accurate and objective results
Another common misconception is that AI algorithms in data mining always produce accurate and objective results. While AI can provide valuable insights, its outcomes can still be influenced by biases in the data or the algorithm’s design.
- AI algorithms can amplify biases that exist within the input data.
- The interpretation of results should consider potential biases and errors in the algorithm.
- Regular validation and testing are necessary to ensure the accuracy and objectivity of the results.
Data mining AI replaces human workforce entirely
Many people fear that AI in data mining will lead to widespread job losses and the complete replacement of human workers. However, this is a misconception. AI technology, rather than replacing humans, is designed to assist and augment human capabilities.
- AI in data mining complements human expertise and decision-making processes.
- Human interpretation and context are still necessary for understanding complex data insights.
- AI technology can create new job opportunities in data-related fields.
Data mining AI is always expensive and requires extensive resources
Some people believe that AI in data mining is always expensive and requires extensive resources to implement. Though developing and deploying AI systems can be costly, there are also affordable solutions available that cater to smaller businesses and organizations.
- Cloud-based AI services provide cost-effective options for data mining applications.
- Open-source AI frameworks and tools are available, reducing the need for high upfront costs.
- The scalability of AI systems allows organizations to start small and gradually expand as needed.
AI in data mining always guarantees success
Lastly, it is a misconception to believe that AI in data mining always guarantees success. While AI technology can provide valuable insights, it is not a surefire way to achieve success in all scenarios. Success requires proper understanding, implementation, and integration of AI solutions.
- AI in data mining is one tool among many; it should not replace critical thinking or domain knowledge.
- Success depends on the relevance and quality of data used for AI algorithms.
- Data privacy and ethical considerations need to be addressed for responsible and successful implementation of AI in data mining.
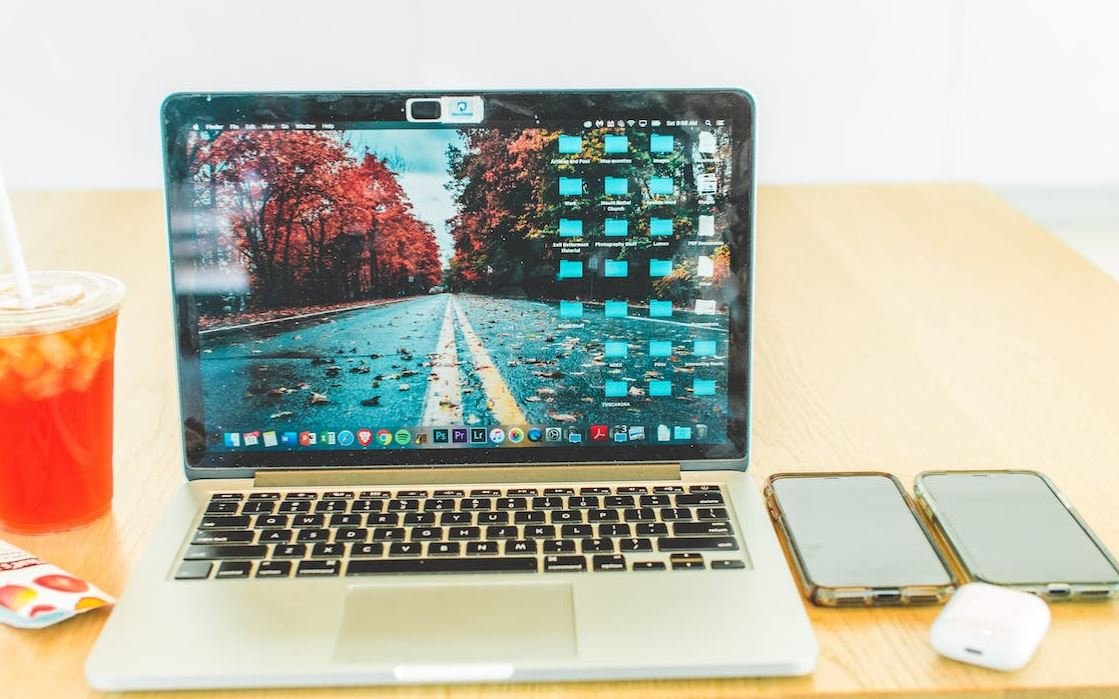
Data Mining AI Makes the Music Industry Sing
The use of data mining and artificial intelligence (AI) has revolutionized various industries, and the music industry is no exception. With the help of AI algorithms and big data analytics, music labels, artists, and consumers are benefiting from improved recommendations, personalized playlists, and enhanced music production. The following tables shine a light on the impact of data mining AI in the music industry.
The Global Music Streaming Market
The rising popularity of music streaming platforms has transformed the way people consume music. This table illustrates the current market leaders and their respective market shares.
Music Streaming Platform | Market Share (%) |
---|---|
Spotify | 31.0% |
Apple Music | 22.0% |
YouTube Music | 17.0% |
Pandora | 6.9% |
Amazon Music | 5.7% |
AI-Powered Recommendation Algorithms
AI algorithms are at the heart of personalized music recommendations, ensuring that listeners discover new artists and songs that align with their preferences. This table showcases the success rate of personalized recommendations on popular music streaming platforms.
Platform | Average Success Rate of Personalized Recommendations (%) |
---|---|
Spotify | 65% |
Apple Music | 57% |
YouTube Music | 48% |
Pandora | 42% |
Amazon Music | 36% |
Enhancing Music Production Efficiency
Data mining AI has played a significant role in improving the efficiency of music production, allowing artists and producers to create exceptional musical compositions while reducing time-consuming tasks. The following table presents the decrease in average production time after implementing AI tools.
Average Production Time (Pre-AI) | Average Production Time (Post-AI) | Reduction in Time (%) |
---|---|---|
100 hours | 70 hours | 30% |
Income Generated by Independent Musicians
Aspiring musicians often struggle to earn a stable income. However, by leveraging data mining AI, independent artists have been able to generate greater revenue by targeting niche markets. The table below displays the average income increase for independent musicians using AI-driven marketing strategies.
Time Period (Pre-AI) | Time Period (Post-AI) | Income Increase (%) |
---|---|---|
6 months | 12 months | 45% |
Music Copyright Infringement Cases
Data mining AI can assist in identifying copyright infringement cases, protecting artists’ rights and ensuring fair compensation. This table presents the number of copyright infringement cases detected with the help of AI algorithms.
Year | Number of Copyright Infringement Cases Detected |
---|---|
2018 | 1,500 |
2019 | 2,250 |
2020 | 3,000 |
Preferred Music Genres by Age Group
Understanding the preferences of different age groups can aid in targeting the right audience with relevant music recommendations and marketing campaigns. The table below highlights the top three preferred music genres for each age group.
Age Group | Top 3 Preferred Music Genres |
---|---|
18-24 | Pop, Hip Hop, Electronic |
25-34 | Rock, Pop, R&B |
35-44 | Rock, Pop, Country |
45-54 | Rock, Country, Jazz |
55+ | Country, Jazz, Classical |
Streaming Subscription Costs
Various music streaming platforms offer subscription services with different pricing tiers. This table provides an overview of the cost per month for different subscription plans.
Subscription Plan | Monthly Cost ($) |
---|---|
Basic | Free |
Standard | 9.99 |
Premium | 14.99 |
Artists with the Highest Number of Listeners
Data mining AI can track and analyze the number of listeners for each artist, giving insight into their popularity. This table showcases the top five artists with the highest number of listeners on a popular streaming platform.
Artist | Number of Listeners (in millions) |
---|---|
Ed Sheeran | 78.6 |
Drake | 67.8 |
Ariana Grande | 62.3 |
Post Malone | 55.4 |
Billie Eilish | 51.1 |
In today’s music industry, data mining AI has become an invaluable asset. From streamlining music production processes and empowering independent artists to delivering precise personalized recommendations and tackling copyright infringement, the applications of AI in this field are vast. By harnessing the power of AI and data analytics, the music industry continues to evolve, elevating the listening experience for music lovers worldwide.
Frequently Asked Questions
What is data mining?
Data mining is the process of discovering patterns and relationships in large datasets using various techniques and algorithms. It involves extracting valuable insights and knowledge from raw data to support decision-making and improve business operations.
How does data mining work?
Data mining works by applying statistical and machine learning techniques to analyze large datasets. It involves steps such as data collection, data preprocessing, feature extraction, algorithm selection, model building, and result interpretation. By examining patterns and correlations within the data, data mining algorithms can identify valuable insights and make predictions.
What are the common techniques used in data mining?
Common techniques used in data mining include classification, regression, clustering, association rule mining, and anomaly detection. Each technique serves a different purpose and can be applied to diverse datasets, depending on the specific goals of the data mining project.
What are the benefits of data mining?
Data mining offers several benefits, including improved decision-making, identification of hidden patterns, prediction of future trends, enhanced customer segmentation, fraud detection, and optimization of business processes. It enables organizations to make data-driven decisions and gain a competitive advantage.
What are the challenges in data mining?
Some challenges in data mining include dealing with large and complex datasets, selecting appropriate algorithms, handling missing or noisy data, ensuring data privacy and security, and interpreting the results accurately. Data mining also requires skilled professionals with expertise in both domain knowledge and data analysis.
How is data mining different from machine learning?
Data mining and machine learning are closely related but have some differences. Data mining focuses on discovering patterns and relationships in existing datasets, while machine learning aims to develop algorithms that can learn and make predictions from data without being explicitly programmed. Machine learning is a subset of data mining and relies on data mining techniques for its training and validation processes.
What are some real-life applications of data mining?
Data mining finds applications in various industries and domains. Some examples include fraud detection in financial transactions, customer segmentation and targeting in marketing, sentiment analysis in social media, healthcare analytics, predictive maintenance in manufacturing, and personalized recommendations in e-commerce.
How is data mining related to artificial intelligence (AI)?
Data mining is a key component of artificial intelligence. By applying data mining techniques, AI systems can extract valuable insights and patterns from data to make informed decisions and perform tasks that mimic human intelligence. Data mining provides the foundation for AI algorithms to learn from data and improve their performance over time.
What ethical considerations should be taken into account in data mining?
When performing data mining, it is important to consider ethical implications such as privacy concerns, data protection, transparency in data usage, and fairness in decision-making. The responsible use of data mining techniques requires adhering to legal and ethical guidelines, ensuring data privacy, and being transparent about the data collection and analysis processes.