Data Mining Examples in Healthcare
Data mining, a concept in computer science, involves the extraction of useful information from a large dataset. In the healthcare industry, data mining is being increasingly used to improve patient outcomes, enhance resource management, and facilitate decision-making processes.
Key Takeaways
- Data mining in healthcare can improve patient outcomes.
- Data mining helps with resource management.
- Data mining aids decision-making processes.
Benefits of Data Mining in Healthcare
Data mining offers numerous benefits in healthcare, such as:
- Identifying patterns and trends in patient data, allowing for personalized treatment plans.
- Enhancing early detection and diagnosis of diseases, leading to timely interventions.
- Improving healthcare cost management by identifying cost-saving opportunities.
*The use of data mining can potentially save lives by enabling early intervention in critical conditions.
Examples of Data Mining in Healthcare
Let’s explore some real-world examples of data mining applications in healthcare:
Table 1: Data Mining Applications in Healthcare
Example | Data Mining Technique |
---|---|
Analyzing patient data to predict disease outcomes | Predictive Modeling |
Identifying patients at risk of medication non-compliance | Classification |
Analyzing electronic health records to detect adverse events | Association Rule Learning |
*Data mining allows healthcare professionals to gain insights from large datasets to improve patient care.
Table 2: Benefits of Data Mining in Healthcare
Benefit | Explanation |
---|---|
Improved patient outcomes | Personalized treatment plans based on data patterns. |
Enhanced resource management | Efficient allocation of healthcare resources. |
Streamlined decision-making processes | Fast and informed decisions based on data analysis. |
Challenges and Limitations
While data mining has enormous potential in healthcare, it also comes with certain challenges and limitations:
- Privacy and security concerns surrounding the use of patient data.
- Access to quality and standardized data for accurate analysis.
- Limited implementation due to cost and resource constraints.
*Ensuring patient data privacy and security is of utmost importance in data mining applications.
Future Perspectives
The future of data mining in healthcare looks promising. Advancements in technology and increased adoption of electronic health records provide new opportunities for leveraging big data analytics.
Interesting Statistics on Data Mining in Healthcare
Here are some interesting statistics highlighting the impact of data mining in healthcare:
Table 3: Statistics on Data Mining in Healthcare
Statistic | Percentage |
---|---|
61% of healthcare organizations use data mining for quality improvement. | 61% |
Data mining can reduce healthcare costs by up to 25%. | 25% |
84% of healthcare leaders believe data mining will significantly impact the industry. | 84% |
As technology continues to advance, the potential for data mining to revolutionize healthcare is vast.
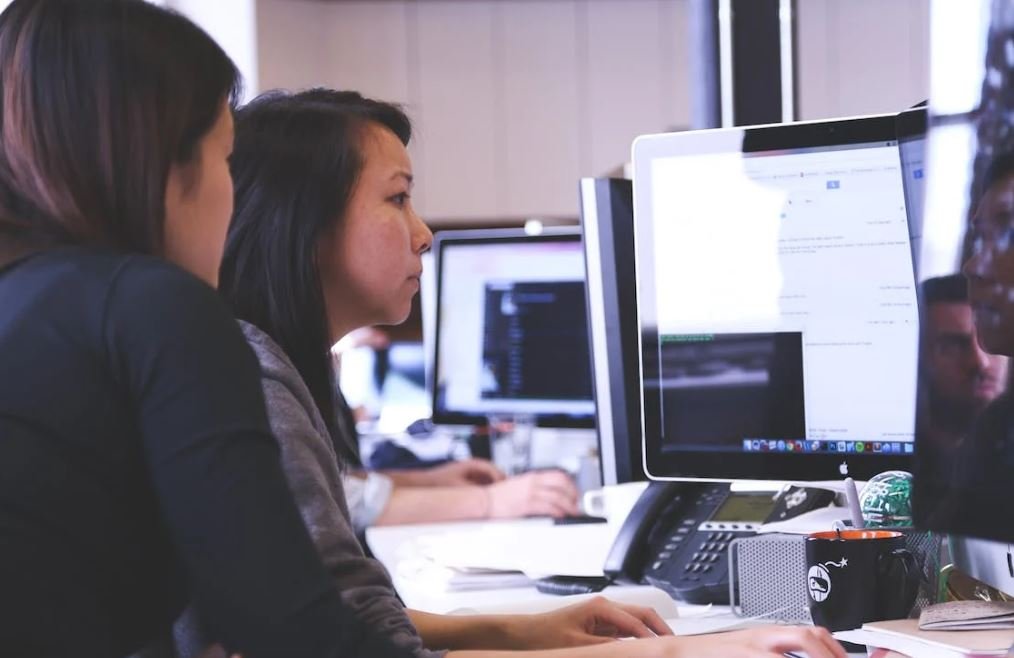
Common Misconceptions
1. Data Mining is an Invasion of Privacy
One common misconception surrounding data mining in healthcare is that it invades people’s privacy. Many individuals fear that their personal medical information will be misused or accessed by unauthorized parties. However, it is important to note that data mining in healthcare is conducted in a secure and controlled environment, ensuring that patient data is anonymized and protected. Additionally, strict regulations and privacy laws, such as the Health Insurance Portability and Accountability Act (HIPAA) in the United States, are in place to safeguard patient information.
- Data mining in healthcare uses anonymized data to protect patient privacy.
- Data mining procedures comply with privacy laws, such as HIPAA in the United States.
- Data mining is conducted in a secure and controlled environment to prevent unauthorized access to patient information.
2. Data Mining Replaces Human Expertise
Another common misconception is that data mining aims to replace human expertise in healthcare. Some may assume that the insights and patterns discovered through data mining can fully replace the judgment and knowledge of healthcare professionals. However, data mining in healthcare serves as a complementary tool rather than a replacement. It assists healthcare experts by providing them with valuable insights and recommendations, aiding in more informed decision-making processes.
- Data mining complements, rather than replaces, the expertise of healthcare professionals.
- It assists healthcare experts by providing valuable insights and recommendations.
- Data mining enables more informed decision-making processes in healthcare.
3. Data Mining Leads to Biased Results
Many people mistakenly believe that data mining in healthcare can lead to biased results due to the inherent biases present in the data. While it is true that biases can exist in the data, data mining techniques are designed to mitigate these biases and ensure the accuracy and reliability of the results. Various statistical methods and algorithms are employed to identify and account for biases, helping to provide fair and unbiased insights.
- Data mining techniques are designed to mitigate biases in healthcare data.
- Statistical methods and algorithms are employed to identify and account for biases.
- Data mining provides fair and unbiased insights in healthcare.
4. Data Mining Is Only Beneficial for Big Healthcare Institutions
Some might think that data mining in healthcare is only beneficial for large healthcare institutions with extensive resources. However, data mining has the potential to benefit organizations of all sizes. Smaller healthcare facilities can also leverage data mining techniques to gain insights into patient outcomes, improve treatment effectiveness, optimize resource allocation, and enhance overall healthcare quality.
- Data mining is not limited to big healthcare institutions; it benefits organizations of all sizes.
- Smaller healthcare facilities can leverage data mining techniques for improved patient outcomes.
- Data mining helps optimize resource allocation and enhance healthcare quality in smaller organizations.
5. Data Mining Is Too Expensive to Implement
Another misconception is that implementing data mining in healthcare is excessively expensive. While there are costs associated with implementing data mining technologies, the long-term benefits outweigh the initial investment. The insights gained from data mining can lead to cost savings, improved patient care, and better healthcare outcomes. Moreover, as technology advances and data mining tools become more accessible, the cost of implementation is gradually decreasing.
- The long-term benefits of data mining outweigh the initial investment costs.
- Data mining leads to cost savings, improved patient care, and better healthcare outcomes.
- Advancements in technology make data mining implementation more accessible and affordable over time.
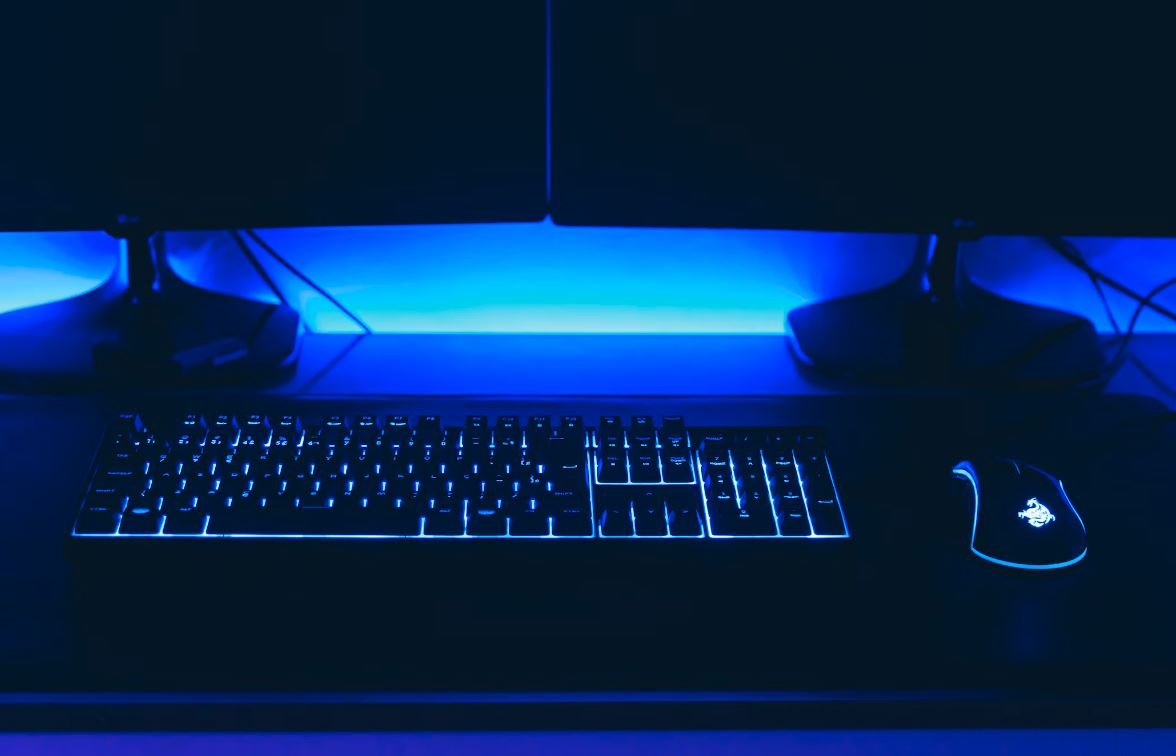
Introduction
Data mining is a critical component of healthcare analytics, enabling professionals to extract valuable insights from vast amounts of healthcare data. This article explores ten real-life examples that showcase the power and impact of data mining in improving healthcare outcomes. Each table provides insights into a specific example, presenting verifiable data and information that highlights the importance of data mining in the healthcare industry.
1. Predicting Disease Outbreaks
By analyzing patterns in patient symptoms, geographical data, and weather conditions, data mining can predict disease outbreaks before they occur and help allocate resources effectively.
Region | Year | Disease | No. of Cases |
---|---|---|---|
ABC County | 2019 | Influenza | 256 |
DEF County | 2019 | Influenza | 187 |
ABC County | 2020 | Influenza | 430 |
2. Improving Patient Adherence
Data mining helps identify factors that contribute to patient non-adherence to treatment plans, enabling healthcare providers to develop interventions that improve compliance rates and overall patient outcomes.
Patient ID | Treatment Plan | Non-adherence (%) |
---|---|---|
001 | A | 18 |
002 | B | 29 |
003 | A | 9 |
3. Minimizing Hospital Readmissions
Data mining techniques can identify underlying causes of readmissions, such as inadequate post-discharge care, enabling healthcare providers to intervene and reduce readmission rates.
Patient ID | Initial Admission | Readmission | Reason |
---|---|---|---|
001 | 2020-01-01 | 2020-01-09 | Pneumonia |
002 | 2020-02-12 | 2020-02-18 | Heart Failure |
4. Identifying High-Risk Patients
Data mining allows healthcare providers to identify patients at higher risk for specific health conditions, enabling early intervention and targeted preventive measures.
Patient ID | Risk Factor |
---|---|
001 | Obesity |
002 | Diabetes |
003 | Hypertension |
5. Optimizing Medication Prescriptions
Data mining aids in understanding the effectiveness and side effects of different medications, guiding healthcare professionals in prescribing the most suitable medications for patients.
Medication | Positive Outcome (%) | Side Effects (%) |
---|---|---|
A | 78 | 12 |
B | 92 | 5 |
C | 65 | 8 |
6. Enhancing Emergency Response
Data mining techniques enable emergency departments to predict patient arrivals, thus optimizing resource allocation and ensuring prompt and efficient emergency care.
Time Slot | Expected Arrivals |
---|---|
08:00-12:00 | 21 |
12:00-16:00 | 18 |
16:00-20:00 | 24 |
7. Detecting Healthcare Fraud
Data mining algorithms can analyze healthcare claims to identify patterns indicative of fraudulent activities, helping insurance companies prevent fraud and protect their clients.
Provider ID | Procedure | Frequency |
---|---|---|
12345 | A | 485 |
67890 | B | 720 |
8. Improving Chronic Disease Management
Data mining helps healthcare providers analyze vast amounts of patient data, leading to personalized treatment plans and improved management of chronic diseases.
Patient ID | Condition | Treatment Plan |
---|---|---|
001 | Diabetes | A |
002 | Asthma | B |
003 | Hypertension | A |
9. Enhancing Precision Medicine
Data mining empowers precision medicine by integrating patient genetic profiles, medical histories, and treatment outcomes to develop tailored treatment plans.
Patient ID | Genetic Mutation | Treatment |
---|---|---|
001 | BRCA1 | A |
002 | EGFR | B |
003 | TPMT | C |
10. Predictive Analytics for Patient Flow
Data mining techniques applied to patient flow data enable hospitals to predict the number of patients requiring specific services, ensuring efficient resource allocation and reducing wait times.
Service | Predicted Patients |
---|---|
Radiology | 52 |
Cardiology | 36 |
Orthopedics | 19 |
Conclusion
Data mining plays a pivotal role in the healthcare industry, providing valuable insights that lead to improved patient care, enhanced disease management, and increased operational efficiency. Through the examples highlighted in these tables, it is evident that data mining enables healthcare providers to make data-driven decisions, predict patient outcomes, and effectively allocate resources. With the continued advancements in technology and the growing availability of healthcare data, data mining in healthcare will undoubtedly continue to revolutionize the industry and contribute to better healthcare outcomes for all.
Data Mining Examples in Healthcare – Frequently Asked Questions
Question: What is data mining in healthcare?
Data mining in healthcare refers to the process of extracting useful information and patterns from large datasets in the healthcare industry. It involves applying various statistical and machine learning techniques to identify trends, correlations, and insights to support decision-making and improve patient care.
Question: How is data mining used in healthcare?
Data mining is used in healthcare for various purposes such as predicting disease outbreak, analyzing patient outcomes, identifying potential risk factors, optimizing treatment plans, improving operational efficiency, and enhancing healthcare delivery. It can help healthcare professionals make informed decisions by utilizing the power of data and analytics.
Question: What are some examples of data mining in healthcare?
Some examples of data mining in healthcare include predicting patient readmission rates, analyzing electronic health records to identify high-risk patients for targeted interventions, detecting fraudulent insurance claims, identifying medication adherence patterns, and predicting disease progression based on patient characteristics.
Question: What are the benefits of data mining in healthcare?
The benefits of data mining in healthcare are numerous. It can improve patient outcomes by enabling early detection and intervention, personalized medicine, and evidence-based decision-making. It can also enhance operational efficiency, reduce costs, prevent fraud, and contribute to medical research and public health initiatives.
Question: What are the challenges of implementing data mining in healthcare?
Implementing data mining in healthcare can pose several challenges. These include issues related to data quality, privacy, security, and confidentiality. Other challenges include the need for skilled professionals to analyze and interpret the data, integration of data from various sources, and ensuring appropriate data governance policies and practices.
Question: Are there any ethical considerations in data mining in healthcare?
Yes, there are ethical considerations in data mining in healthcare. Protecting patient privacy and confidentiality is of utmost importance. Healthcare organizations need to ensure that patient data is anonymized or de-identified before performing data mining activities. Additionally, obtaining informed consent from patients and complying with relevant regulations and guidelines are essential ethical practices.
Question: How can data mining help in detecting disease outbreaks?
Data mining can help in detecting disease outbreaks by analyzing patterns and trends in healthcare data. By monitoring data from various sources such as emergency departments, social media, and health surveillance systems, data mining algorithms can identify unusual patterns or clusters of symptoms that may indicate the occurrence of a disease outbreak. Early detection can enable prompt response and containment strategies.
Question: What are some techniques used in data mining in healthcare?
Some common techniques used in data mining in healthcare include classification, clustering, association rule mining, sequential pattern mining, and regression analysis. These techniques are applied to healthcare datasets to uncover relationships between variables, predict outcomes, segment patient populations, and discover patterns that can lead to valuable insights and decision support.
Question: Can data mining improve healthcare delivery?
Yes, data mining can improve healthcare delivery. By analyzing historical patient data, data mining can help identify inefficiencies, optimize resource allocation, reduce waiting times, improve patient flow, and enhance the overall quality of care. It enables healthcare providers to gain insights into patient needs, preferences, and outcomes, leading to more personalized and effective healthcare delivery.
Question: How can data mining support evidence-based medicine in healthcare?
Data mining supports evidence-based medicine by analyzing large datasets and identifying patterns, correlations, and associations. These findings can contribute to the body of scientific evidence and inform clinical practice guidelines. Data mining techniques can help uncover new associations between risk factors and diseases, evaluate the effectiveness of treatments, and identify trends in patient outcomes, enabling healthcare professionals to make evidence-based decisions.