Data Mining Examples
Data mining is the process of extracting patterns and relationships from large datasets, with the goal of discovering meaningful insights and making informed decisions. This practice is widely used in various industries, including marketing, finance, healthcare, and more. In this article, we will explore several data mining examples that showcase the power and importance of this technique.
Key Takeaways:
- Data mining is crucial for extracting valuable insights from large datasets.
- It is widely used across industries such as marketing, finance, and healthcare.
- By uncovering patterns and relationships, data mining enables informed decision-making.
1. Customer Segmentation:
One major application of data mining is customer segmentation, where companies divide their customer base into distinct groups based on common characteristics and behaviors. Using data mining techniques, businesses can identify clusters of customers with similar preferences, allowing them to tailor their marketing strategies and offerings accordingly. *Segmenting customers provides a deeper understanding of their needs and preferences, ultimately leading to enhanced customer satisfaction and increased sales.*
2. Fraud Detection:
Data mining is instrumental in fraud detection and prevention. By analyzing historical transactional data, such as credit card purchases or insurance claims, companies can identify fraudulent patterns and potential red flags. Data mining algorithms can detect anomalies and unusual patterns, enabling organizations to flag suspicious activities and take appropriate preventive measures. *This allows businesses to save significant amounts of money by minimizing financial losses caused by fraud.*
3. Predictive Analytics:
Predictive analytics is an essential part of data mining, where historical data and statistical models are used to make predictions about future events or behavior. By analyzing patterns and trends, businesses can forecast upcoming trends, estimate customer churn rates, optimize inventory management, and predict market demand. *This enables businesses to proactively respond to changes and make data-driven strategic decisions with confidence.*
Tables:
Example | Data Mining Application |
---|---|
Web browsing history | Targeted advertising |
Customer purchase data | Recommendation systems |
Data Mining Technique | Industry |
---|---|
Association rule mining | Retail |
Decision trees | Healthcare |
Data Mining Benefit | Example |
---|---|
Cost savings | Fraud detection |
Improved customer targeting | Customer segmentation |
Summary:
Data mining plays a critical role in various industries by enabling organizations to extract insights from large datasets. Through customer segmentation, fraud detection, and predictive analytics, businesses can make data-driven decisions and gain a competitive edge. Leveraging data mining techniques and tools empowers companies to uncover valuable patterns and relationships, optimizing their operations and enhancing customer experiences. Embrace the power of data mining and unlock the hidden potential of your data today!
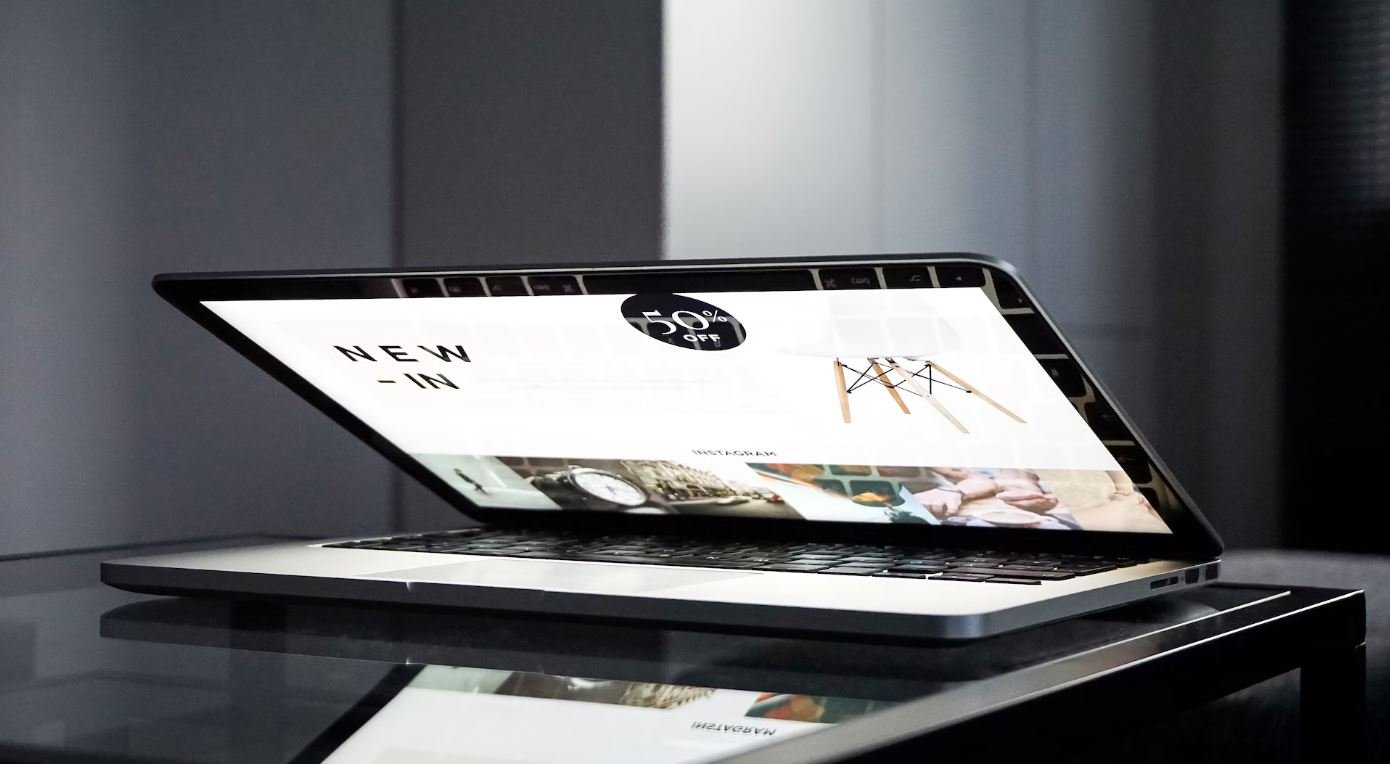
Common Misconceptions
Misconception 1: Data mining is only used by large corporations
One common misconception about data mining is that it is only utilized by large corporations. However, this is far from the truth. Data mining techniques can be applied by businesses of any size, including small and medium enterprises (SMEs), as well as individuals.
- Data mining can be beneficial for SMEs in identifying customer trends and preferences, helping them make more informed business decisions.
- Data mining tools and software are often affordable and accessible to businesses and individuals alike.
- With the rise of cloud computing, even individuals can leverage data mining techniques to analyze and gain insights from their personal data.
Misconception 2: Data mining is the same as data analysis
Another misconception is that data mining is synonymous with data analysis. While data analysis is often a part of data mining, they are not the same thing. Data mining is a more specific process that involves discovering patterns, relationships, or insights from large datasets, while data analysis refers to the broader examination and interpretation of data.
- Data mining requires the use of advanced algorithms and techniques to extract meaningful information from vast amounts of data.
- Data analysis encompasses a wide range of techniques, including statistical analysis, visualization, and descriptive analytics.
- Data mining is typically used for predictive analytics, uncovering hidden patterns and making predictions based on historical data.
Misconception 3: Data mining is used to invade privacy
One of the most prevalent misconceptions about data mining is that it is solely used to invade privacy. While data mining can potentially be misused for unethical purposes, such as unauthorized access to personal information, the field itself is not inherently invasive.
- Data mining can be used for legitimate purposes, such as improving customer experiences and personalizing recommendations in e-commerce.
- Data mining techniques can help in detecting and preventing fraud and identifying potential security threats.
- Data mining practices are often regulated by privacy laws and policies, ensuring the protection of individuals’ privacy rights.
Misconception 4: Data mining is only applicable in business-related fields
Many people mistakenly believe that data mining is only relevant in business-related fields. However, data mining techniques have applications in various domains beyond commerce.
- Data mining can be utilized in healthcare to identify patterns in patient data, leading to improved diagnoses and treatment plans.
- Data mining is valuable in scientific research, assisting in identifying correlations and patterns that can lead to new discoveries.
- Data mining techniques can be applied in social media analysis, helping to understand user behavior and trends.
Misconception 5: Data mining guarantees accurate predictions
Lastly, a widespread misconception about data mining is that it always guarantees accurate predictions. While data mining can provide valuable insights and predictions, there are various factors that can influence the accuracy of the results.
- Data quality plays a crucial role in the accuracy of data mining predictions.
- Incomplete or biased data can lead to inaccurate predictions or misinterpretation of results.
- Data mining models are based on assumptions and simplifications, and their predictions are probabilistic in nature.
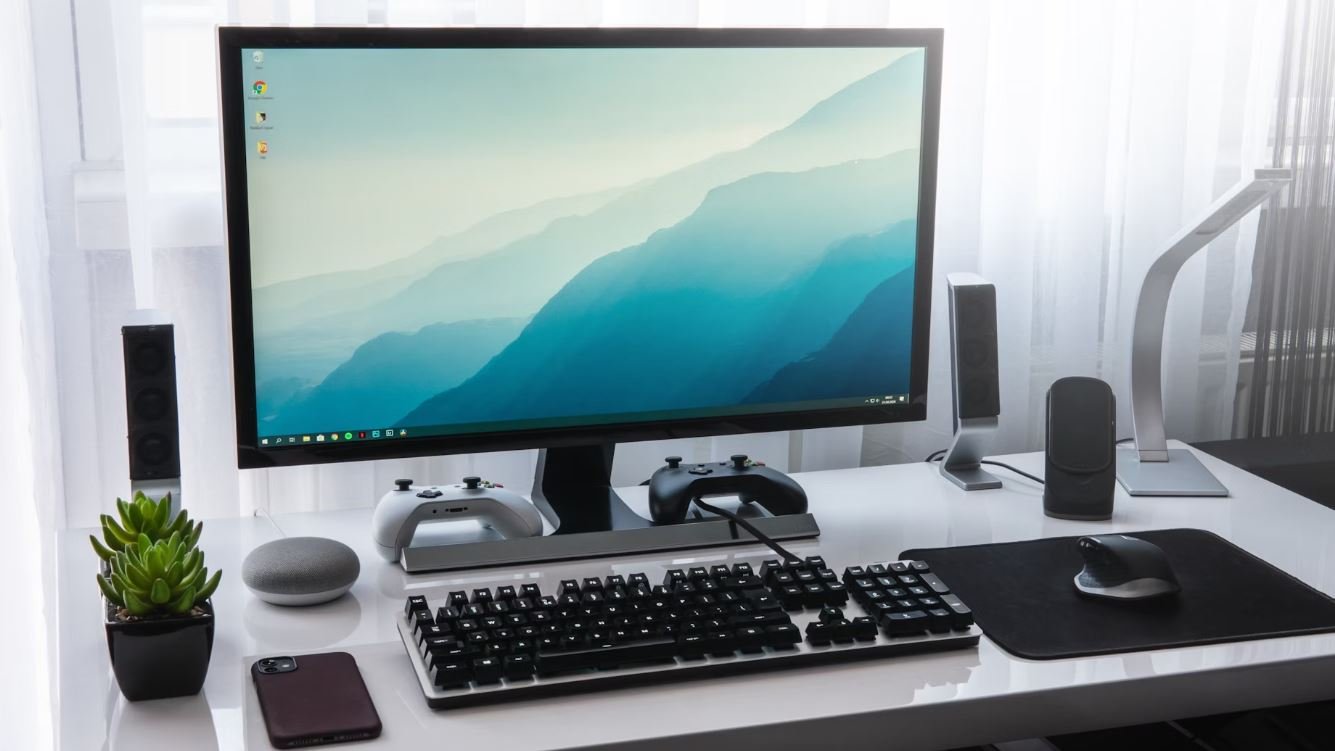
Data Mining Examples: Analyzing Patterns and Unveiling Insights
Data mining is a powerful technique that enables organizations to discover hidden patterns, trends, and insights from vast amounts of data. By applying various algorithms and statistical models, valuable knowledge can be extracted and utilized for decision-making purposes. In this article, we explore ten fascinating examples of data mining, shedding light on diverse fields and uncovering intriguing information. Each table below represents a different scenario wherein data mining has been employed to extract meaningful insights.
Unraveling Consumer Preferences in Online Shopping
Online retailers gather immense data about their customers, enabling them to understand preferences and tailor their offerings. By mining transactional data, these insights can drive targeted marketing strategies and personalized recommendations, enhancing the overall shopping experience.
Forecasting Stock Market Trends using Historical Data
Investors and traders often rely on data mining techniques to analyze historical stock market data. By identifying patterns in past performance and capturing market trends, predictions about future stock prices can be made. Such insights enable informed decision-making and potentially maximize profits.
Understanding Customer Churn in the Telecommunications Industry
Telecom companies face the challenge of minimizing customer churn, which can have a significant impact on their bottom line. By mining customer data, such as call duration, billing information, and service usage, companies can identify patterns and factors that contribute to customer churn, allowing them to implement retention strategies effectively.
Optimizing Energy Consumption in Smart Grids
Data mining is instrumental in optimizing energy consumption and load management in smart grids. By analyzing historical data on energy usage, patterns and trends can be identified, enabling efficient energy distribution, reducing waste, and enhancing overall grid performance.
Enhancing Fraud Detection in Financial Transactions
Financial institutions leverage data mining techniques to detect instances of fraudulent activities, such as credit card fraud or money laundering. By analyzing transactional data and identifying patterns that deviate from regular behavior, suspicious activities can be pinpointed, allowing timely intervention and prevention.
Improving Medical Diagnosis and Treatment
Data mining plays a crucial role in healthcare by analyzing patient data and medical records to identify patterns and extract insights. This allows healthcare professionals to improve diagnosis accuracy, predict disease outcomes, and recommend personalized treatments based on historical data.
Enhancing Road Safety through Traffic Pattern Analysis
Data mining techniques can contribute to improved road safety by analyzing traffic patterns and accident data. By identifying factors contributing to accidents, such as weather conditions, road design, and traffic congestion, targeted measures can be implemented to reduce accidents and enhance overall road safety.
Personalized News Recommendations based on User Behavior
Online news platforms utilize data mining to analyze user behavior and preferences. By examining browsing history, read articles, and interactions, personalized news recommendations can be generated. This enhances user engagement and satisfaction while providing them with relevant and interesting content.
Efficient Resource Allocation in Supply Chain Management
Supply chain management can benefit from data mining techniques to optimize resource allocation and logistics planning. By analyzing data such as demand patterns, transportation costs, and inventory levels, organizations can make informed decisions about procurement, inventory management, and distribution strategies.
Improving Customer Satisfaction through Sentiment Analysis
Data mining is used to extract insights from customer feedback and sentiment analysis. By analyzing customer reviews, social media posts, and comments, organizations can identify prevailing sentiments, enabling them to address issues and trends that impact customer satisfaction and loyalty.
In this article, we have highlighted ten compelling examples of data mining and its impact across various industries. From unraveling consumer preferences and enhancing road safety to improving medical diagnosis and optimizing resource allocation, data mining empowers organizations to unveil valuable insights, enhancing decision-making and driving positive outcomes. As the era of big data continues to evolve, the role of data mining becomes increasingly significant in unlocking the full potential of data.
Frequently Asked Questions
What is data mining?
Data mining is a process of discovering patterns and extracting valuable information from large datasets to solve business problems or gain insights.
How is data mining different from data analysis?
Data mining involves the use of automated algorithms and machine learning techniques to discover patterns or relationships in data. Data analysis, on the other hand, encompasses a broader range of techniques that involve examining, cleaning, transforming, and modeling data.
What are some real-life examples of data mining?
Data mining is used in various industries and applications. Some examples include customer segmentation and profiling for marketing campaigns, fraud detection in financial transactions, recommendation systems in e-commerce, predictive maintenance in manufacturing, and sentiment analysis in social media.
What are the steps involved in the data mining process?
The data mining process typically involves several steps: data collection, data preprocessing, data transformation, pattern discovery, pattern evaluation, and deployment. Each step plays a vital role in extracting meaningful insights from the data.
What are the main challenges in data mining?
Some of the challenges in data mining include handling large datasets, dealing with missing or noisy data, choosing the right data mining techniques, interpreting and validating the results, and ensuring data privacy and security.
What are some commonly used data mining techniques?
There are several commonly used data mining techniques, including classification, clustering, association rule mining, regression analysis, time series analysis, anomaly detection, and text mining. The choice of technique depends on the nature of the data and the problem at hand.
How do businesses benefit from data mining?
Data mining enables businesses to gain valuable insights from their data, which can be used to improve decision-making, identify market trends, optimize processes, detect anomalies or fraud, enhance customer targeting and personalization, and increase overall efficiency and productivity.
What are the ethical considerations in data mining?
Data mining raises ethical concerns such as privacy, consent, data bias, and the responsible use of collected data. Organizations must ensure they comply with relevant regulations, obtain informed consent, protect individuals’ privacy, and use data in a transparent and ethical manner.
What skills are required to perform data mining?
Data mining requires a combination of skills, including a solid understanding of statistics and mathematics, proficiency in programming and data manipulation, familiarity with data mining tools and techniques, and critical thinking and problem-solving abilities.
What is the future of data mining?
The future of data mining looks promising, with advancements in artificial intelligence and machine learning. As more data becomes available and technology improves, data mining is expected to play a crucial role in various domains, including healthcare, finance, transportation, and smart cities.