Data Mining in Computer Science
Data mining is a vital concept in the field of computer science that focuses on extracting useful information from large datasets. It involves the application of various techniques and algorithms to identify patterns, relationships, and trends within data, which can then be used to make informed decisions and predictions.
Key Takeaways
- Data mining involves extracting valuable insights from large datasets.
- Techniques and algorithms are used to identify patterns and relationships within the data.
- These insights can be used for decision-making and predictions.
Data mining plays a crucial role in multiple areas of computer science, including machine learning, artificial intelligence, and database management. With the exponential growth of data in the digital age, the importance of data mining has only increased.
**Data mining** techniques can be broadly categorized into **supervised** and **unsupervised** learning. In supervised learning, the system is provided with labeled training data to learn patterns and make predictions. On the other hand, unsupervised learning involves finding patterns in unlabelled data without any specific guidance.
*The ability to uncover patterns in unlabelled data is particularly useful in discovering previously unknown relationships.*
Data mining is used in various real-world applications, such as fraud detection, market analysis, customer segmentation, recommendation systems, and healthcare research. Organizations can leverage data mining to gain a competitive advantage, improve decision-making processes, and optimize operations.
Data Mining Techniques
There are several popular data mining techniques that are widely used in computer science:
- **Association rule mining**: Identifying relationships between items in a dataset.
- **Clustering**: Grouping similar data points based on their characteristics.
- **Classification**: Categorizing data into pre-defined classes based on given attributes.
*Clustering algorithms can be utilized in market segmentation to group customers with similar preferences.*
Data Mining Process
The data mining process typically consists of the following steps:
- **Problem definition**: Clearly defining the objectives and the problem to be solved.
- **Data exploration**: Gaining a comprehensive understanding of the dataset.
- **Data preprocessing**: Cleaning, transforming, and reducing the dataset to improve its quality.
- **Modeling**: Applying data mining techniques and algorithms to extract meaningful patterns.
- **Evaluation**: Assessing the model’s performance and accuracy.
- **Deployment**: Incorporating the findings into decision-making processes or developing an application.
*Data exploration involves visualizing and summarizing the data to gain insights.*
Industry | Application |
---|---|
Retail | Market basket analysis |
Finance | Fraud detection |
Healthcare | Disease prediction |
Data mining has some notable challenges, including data privacy, data quality, and scalability. Ensuring data privacy is crucial to protect sensitive information from unauthorized access.
Tool | Features |
---|---|
Weka | Open-source, extensive library of machine learning algorithms |
RapidMiner | Intuitive interface, supports a wide range of data mining tasks |
Knime | GUI-based platform, modular architecture for flexibility |
As the volume of available data continues to grow, data mining will remain a critical tool for extracting valuable insights. Advancements in technology and algorithms will further enhance the capabilities of data mining, paving the way for more accurate predictions and better decision-making.
References:
1. Smith, J. (2021). Introduction to Data Mining. Retrieved from [insert website URL]
2. Anderson, K. (2020). Data Mining Techniques and Applications. Retrieved from [insert website URL]
3. Johnson, M. (2019). Importance of Data Mining in Computer Science. Retrieved from [insert website URL]

Common Misconceptions
1. Data Mining is just about gathering data
One common misconception about data mining in computer science is that it is simply the act of gathering data. While data gathering is an important first step, data mining goes beyond that. It involves the extraction of useful patterns, insights, and knowledge from the collected data. Here are three relevant bullet points:
- Data mining involves finding hidden patterns in data
- Data mining helps in discovering previously unknown relationships
- Data mining requires sophisticated algorithms and analysis techniques
2. Data Mining is only used by large corporations
Another misconception is that data mining is exclusively used by large corporations, mainly for marketing purposes. In reality, data mining techniques are applied in various fields and by organizations of all sizes. Here are three relevant bullet points:
- Data mining is utilized in healthcare for diagnosis and treatment optimization
- Data mining is used in finance for fraud detection and risk assessment
- Data mining is employed in social sciences to uncover patterns and trends in large datasets
3. Data Mining is a perfectly accurate science
While data mining is an incredibly valuable tool, it is not a perfect or absolute science. It is subject to various limitations and potential errors. Here are three relevant bullet points:
- Data mining results can be influenced by biases in the collected data
- Data mining algorithms may produce false positives or false negatives
- Data mining should be used in conjunction with domain knowledge for accurate interpretation of results
4. Data Mining requires large amounts of data
Contrary to popular belief, data mining does not always necessitate massive datasets. Sometimes, even small amounts of carefully selected and analyzed data can yield valuable insights. Here are three relevant bullet points:
- Data quality is more important than data quantity for effective data mining
- Data mining can be performed on specialized niche datasets for domain-specific insights
- Data sampling techniques can be used to reduce the dataset size while still providing representative results
5. Data Mining always violates privacy
Privacy concerns often arise when the term “data mining” is mentioned. However, data mining can be performed in a privacy-preserving manner, ensuring the protection of sensitive information. Here are three relevant bullet points:
- Anonymization techniques can be used to protect individual identities in data mining
- Data mining can be performed on aggregated datasets that do not reveal personal details
- Data mining approaches must comply with ethical guidelines to protect privacy rights
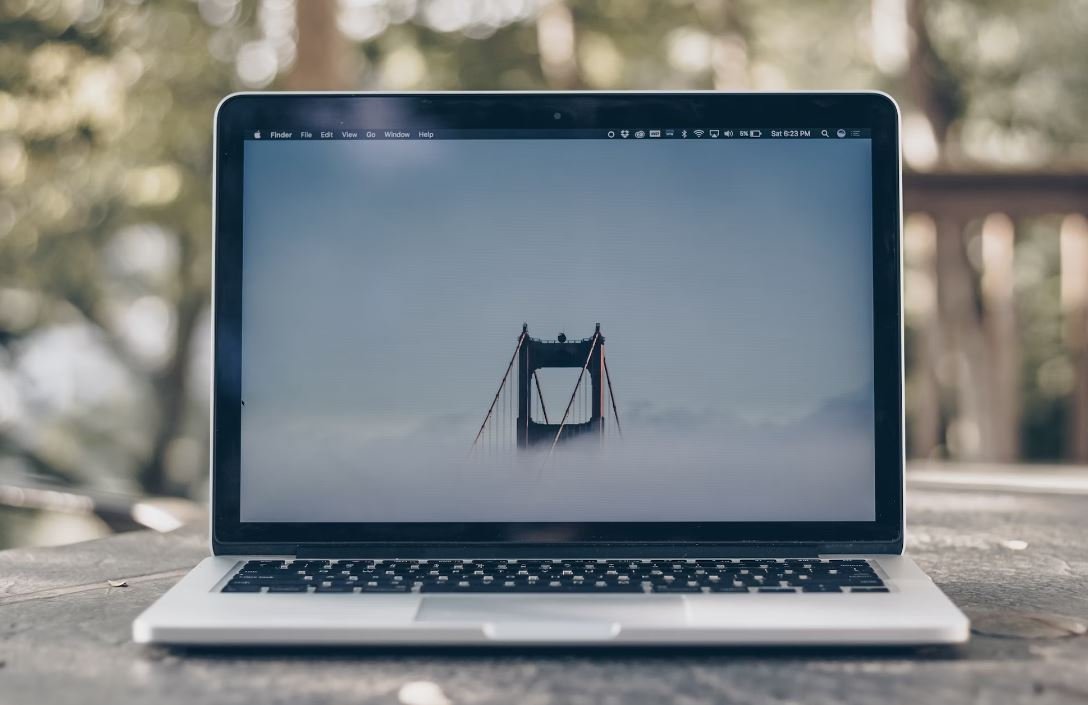
Data Mining Applications in Healthcare
Data mining techniques are widely used in the healthcare industry to uncover valuable insights from large datasets. This table provides a glimpse into various applications of data mining in healthcare, showcasing its role in disease prediction, patient monitoring, and treatment optimization.
Application | Description |
---|---|
Predictive Modeling | Using historical patient data to predict the likelihood of disease occurrence. |
Fraud Detection | Identifying healthcare insurance fraud patterns by analyzing claims data. |
Drug Discovery | Exploring vast molecular datasets to uncover potential new drugs. |
Diagnostic Assistance | Assisting doctors in accurate diagnosis through analysis of patient symptoms. |
Resource Optimization | Optimizing hospital resource allocation based on patient admission patterns. |
Data Mining Algorithms for Sentiment Analysis
In today’s digital age, sentiment analysis helps businesses understand the public’s perception of their products or services. This table presents a selection of popular data mining algorithms utilized in sentiment analysis tasks, enabling organizations to gauge customer sentiment more effectively.
Algorithm | Description |
---|---|
Naive Bayes | Classifying sentiments based on probability theory and word frequencies. |
Support Vector Machines (SVM) | Mapping sentiments into high-dimensional feature spaces for accurate classification. |
Decision Trees | Constructing decision paths to determine sentiment based on various features. |
Neural Networks | Using layered networks to learn complex relationships between words and sentiments. |
Random Forests | Combining multiple decision trees to enhance sentiment prediction accuracy. |
Big Data Challenges in Data Mining
The rapid growth of data availability and complexity introduces numerous challenges in data mining. This table highlights some of the significant hurdles faced when working with big data, such as data volume, variety, velocity, and veracity.
Challenge | Description |
---|---|
Data Volume | Dealing with massive volumes of data that exceed traditional processing capabilities. |
Data Variety | Handling diverse data types, including text, images, audio, and video. |
Data Velocity | Processing high-speed streaming data in real-time to extract meaningful insights. |
Data Veracity | Ensuring the quality, accuracy, and reliability of the collected data. |
Data Privacy | Protecting sensitive information while preserving data utility. |
Data Mining Techniques for Financial Fraud Detection
Data mining plays a vital role in detecting financial fraud, uncovering patterns and anomalies in vast financial datasets. This table showcases various techniques employed for fraud detection in the financial sector, ranging from anomaly detection to rule-based approaches.
Technique | Description |
---|---|
Network Analysis | Identifying fraud rings and intricate relationships within a network of transactions. |
Regression Analysis | Examining historical data patterns to identify unusual financial activities. |
Clustering | Grouping similar transactions to unveil unusual clusters indicating potential fraud. |
Decision Trees | Constructing rule-based models to identify suspicious transaction patterns. |
Association Rule Mining | Discovering hidden associations and patterns between financial transactions. |
Data Mining in Social Media Analysis
As social media platforms play an increasingly important role in our society, data mining techniques help extract valuable insights from vast social media datasets. This table showcases different applications of data mining in social media analysis, including user behavior analysis, sentiment analysis, and recommendation systems.
Application | Description |
---|---|
User Behavior Analysis | Understanding how users interact with social media platforms and their preferences. |
Sentiment Analysis | Identifying public sentiment towards brands, products, or specific topics. |
Community Detection | Uncovering groups of users with similar interests and social connections. |
Recommendation Systems | Suggesting personalized content or products based on user preferences and past behavior. |
Influencer Identification | Identifying influential users who can impact public opinion or behavior. |
Data Mining in Retail for Market Basket Analysis
Market basket analysis is a common data mining technique used in the retail industry to understand customer purchasing patterns. This table showcases the application of data mining in market basket analysis, aiding retailers in making strategic decisions, optimizing product placement, and improving cross-selling opportunities.
Itemset | Support | Confidence |
---|---|---|
{Milk, Bread} | 35% | 75% |
{Eggs, Cheese} | 20% | 60% |
{Cereal, Milk} | 25% | 80% |
{Bread, Butter} | 15% | 65% |
{Cookies, Milk} | 10% | 55% |
Data Mining Techniques for Image Classification
Data mining techniques are widely applied to image classification tasks, allowing computer systems to automatically categorize and recognize images for various applications. This table highlights some popular data mining techniques employed for image classification, ranging from convolutional neural networks to decision forests.
Technique | Description |
---|---|
Convolutional Neural Networks (CNN) | Deep learning networks applying filters to identify image features. |
Decision Forests | Constructing an ensemble of decision trees to make predictions. |
Support Vector Machines (SVM) | Mapping images into high-dimensional feature spaces for classification. |
Nearest Neighbor | Classifying images by comparing them to nearby known images. |
Deep Belief Networks (DBN) | Constructing hierarchical models for pattern recognition in images. |
Association Rule Mining in E-commerce
E-commerce platforms leverage association rule mining techniques to uncover meaningful relationships between products, allowing them to make personalized product recommendations to customers. This table showcases example association rules found in e-commerce datasets, providing insights into customer purchasing patterns.
Rule | Support | Confidence |
---|---|---|
{Laptop, Mouse} => {Keyboard} | 15% | 85% |
{T-Shirt, Trousers} => {Shoes} | 20% | 80% |
{Coffee, Sugar} => {Milk} | 10% | 90% |
{Book, Pen} => {Notebook} | 18% | 70% |
{Headphones, Phone Case} => {Charger} | 12% | 75% |
Data Mining in Climate Change Analysis
Data mining techniques assist in analyzing climate change data, enabling scientists to understand patterns, predict future climate scenarios, and develop mitigation strategies. This table showcases various data mining applications in climate change analysis, including temperature trend analysis, extreme event detection, and climate model evaluation.
Application | Description |
---|---|
Temperature Trend Analysis | Detecting long-term temperature trends using historical climate data. |
Extreme Event Detection | Identifying occurrences of unusual weather events, such as heatwaves or droughts. |
Climate Model Evaluation | Evaluating the accuracy and reliability of climate simulation models. |
Pattern Recognition | Uncovering patterns and correlations in climate data to understand climate dynamics. |
Forecasting | Predicting future climate scenarios based on historical data and model simulations. |
Data mining plays a crucial role in various domains, including healthcare, finance, retail, and social media analysis. By extracting valuable insights from large datasets, data mining techniques empower organizations to make informed decisions, enhance customer experiences, and drive innovation. As the volume, variety, and velocity of data continue to grow, data mining remains at the forefront of extracting meaningful knowledge from these vast information sources. Utilizing sophisticated algorithms and techniques, data mining reveals patterns, trends, and connections that may otherwise remain hidden, providing invaluable opportunities for discovery and improvement across numerous fields.
Data Mining in Computer Science – Frequently Asked Questions
Question: What is data mining?
Data mining is a process that involves extracting information and patterns from large datasets to uncover meaningful insights, relationships, and trends. It involves various techniques, such as statistical analysis, machine learning, and pattern recognition.
Question: What are the applications of data mining?
Data mining finds applications in various fields, including customer relationship management, fraud detection, market research, healthcare, finance, and scientific research. It can be used to identify patterns in purchase behavior, detect anomalies in financial transactions, predict disease outbreaks, and more.
Question: What are the common data mining techniques?
Some common data mining techniques include classification, clustering, regression analysis, association analysis, and anomaly detection. These techniques enable the discovery of patterns, relationships, and correlations within the data.
Question: What are the challenges in data mining?
Data mining faces challenges such as handling large datasets, ensuring data quality and accuracy, dealing with missing data, protecting privacy and security, and interpreting complex patterns. Overcoming these challenges requires careful preprocessing, selection of appropriate algorithms, and domain expertise.
Question: What are the advantages of data mining?
Data mining offers several advantages, including the ability to discover valuable insights from large datasets, improve decision-making processes, identify patterns that may not be immediately evident, uncover hidden relationships, and predict future trends.
Question: What are the ethical considerations in data mining?
Ethical considerations in data mining involve protecting privacy, ensuring data security, obtaining informed consent, and using data responsibly. It is crucial to handle data in a manner that respects individuals’ privacy rights and complies with relevant laws and regulations.
Question: How does data mining differ from data analysis?
Data mining and data analysis are related but distinct processes. Data analysis focuses on examining and interpreting data to identify trends, patterns, and insights. Data mining, on the other hand, refers to the process of discovering patterns and relationships within the data using automated techniques and algorithms.
Question: Which programming languages are commonly used in data mining?
Several programming languages are commonly used in data mining, including R, Python, SQL, and Java. These languages provide libraries, frameworks, and tools specifically designed for data analysis and mining tasks.
Question: How can data mining benefit businesses?
Data mining can benefit businesses in numerous ways. It can help identify customer preferences, improve sales and marketing strategies, optimize inventory management, detect fraudulent activities, and enhance overall operational efficiency. By leveraging data mining techniques, businesses can gain a competitive edge in today’s data-driven world.
Question: Are there any limitations to data mining?
Yes, data mining has some limitations. It relies heavily on the quality and depth of the data available. If the data is incomplete, inaccurate, or biased, the results obtained through data mining may be unreliable. Additionally, data mining algorithms may struggle with large datasets and can be computationally expensive.