Data Mining Quality
Data mining is the process of extracting knowledge and valuable information from large data sets. In the era of big data, organizations are increasingly relying on data mining to gain insights and make informed decisions. However, ensuring the quality of the data being mined is crucial to the success and accuracy of the results obtained.
Key Takeaways:
- Data mining involves extracting knowledge from large data sets.
- Quality of data being mined is essential for accurate results.
**Quality data** is crucial when it comes to data mining. It ensures that the results obtained are reliable, consistent, and accurate. **Quality data** refers to data that is clean, complete, consistent, and relevant.
*Data mining* algorithms rely on patterns and relationships found in the data to make predictions and draw conclusions. It is important to note that **quality data** leads to more accurate and reliable predictions, whereas poor quality data can lead to erroneous results.
The Importance of Data Quality
Data quality plays a significant role in the success of data mining efforts. **High-quality data** ensures that the outcomes are trustable and can be used with confidence. On the other hand, **poor quality data** introduces errors and biases into the analysis, resulting in unreliable predictions and misleading insights.
*Data inconsistencies* can arise due to various reasons, such as data entry errors, duplicate records, and missing values. These inconsistencies can impact the accuracy of data mining models. **Ensuring data accuracy** is key to obtaining meaningful and actionable insights.
Metrics for Evaluating Data Quality
Several metrics can be used to evaluate the quality of data used for data mining. **Completeness** measures the extent to which data is missing, while **consistency** assesses the degree of data conformity within and across datasets. **Validity** checks if the data conforms to predefined rules and standards. **Accuracy** determines the correctness of data, while **timeliness** evaluates the relevance and currency of the data.
*Outlier detection* is an essential aspect of data quality assessment. Identifying and handling outliers is crucial as these values can significantly impact the findings of data mining. Adopting appropriate **data cleansing techniques** is vital to ensure accurate results.
Metric | Description |
---|---|
Completeness | The degree to which data is missing. |
Consistency | The conformity of data within and across datasets. |
Validity | Assessment of data conformity to predefined rules/standards. |
Data Mining Techniques for Improving Data Quality
Data mining techniques can be employed to improve data quality before performing actual analysis. **Data preprocessing** involves several steps, such as data cleaning, data integration, data transformation, and data reduction. These techniques help in enhancing the quality and reliability of the data used for data mining.
*Entity resolution* is a subtask of data preprocessing that involves identifying and merging records that refer to the same real-world entity. This technique helps in reducing duplicate records and improving data quality.
Furthermore, **data profiling** allows organizations to gain insights into the quality of their data by analyzing its characteristics, distributions, and patterns. With data profiling, businesses can identify data quality issues and take necessary actions to rectify them.
Conclusion
Ensuring data quality is paramount for successful and reliable data mining. Organizations should prioritize maintaining *high-quality data* to generate accurate predictions and obtain meaningful insights. By employing appropriate techniques and metrics for evaluating and improving data quality, businesses can enhance the effectiveness and credibility of their data mining efforts.
Technique | Description |
---|---|
Data Preprocessing | Steps to enhance data quality before analysis. |
Entity Resolution | Identifying and merging duplicate records. |
Data Profiling | Analysis of data characteristics, distributions, and patterns. |
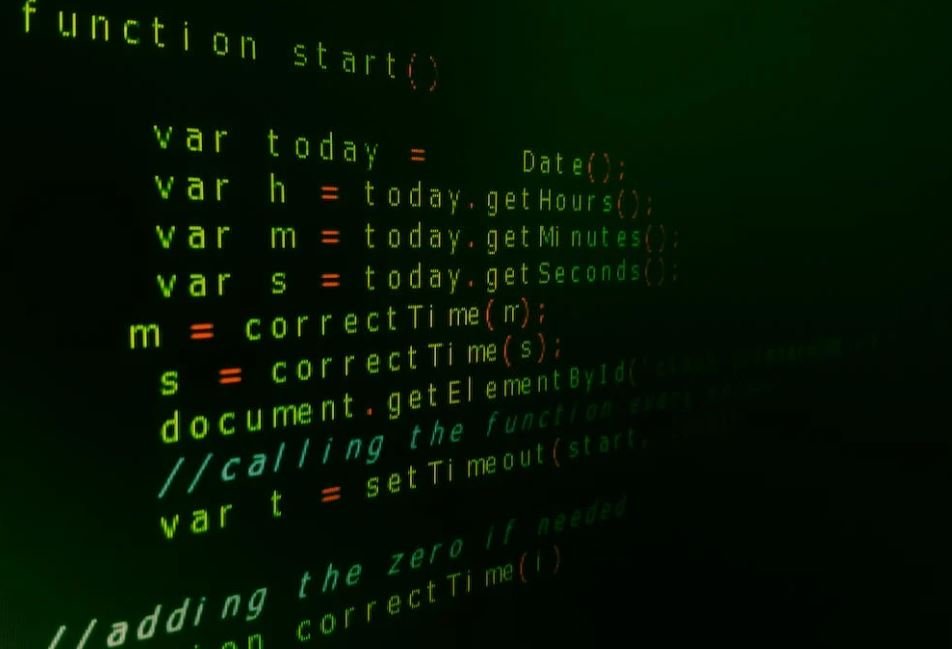
Common Misconceptions
Misconception 1: Data mining is only used for collecting personal information
One common misconception about data mining is that it is solely used for collecting personal information in an invasive way. However, data mining is not exclusively focused on collecting personal data, but rather on extracting patterns and insights from large datasets.
- Data mining involves analyzing various types of data, such as customer behavior, market trends, and even scientific research.
- Data mining helps businesses make informed decisions by uncovering hidden patterns and relationships in data.
- Data mining can be used in fields such as healthcare to improve patient care and treatment planning.
Misconception 2: Data mining always violates privacy
Another misconception is that data mining automatically violates individuals’ privacy and exposes personal information. However, data mining can be done in a responsible manner that protects privacy rights and maintains confidentiality.
- Sensitive personal information should be anonymized or encrypted before conducting data mining to ensure privacy preservation.
- Data storage and access should be regulated to prevent unauthorized use of personal data.
- Data mining projects should adhere to ethical and legal guidelines to respect individuals’ privacy rights.
Misconception 3: Data mining can replace human judgment
Some people believe that data mining algorithms alone can replace human judgment and decision-making. However, data mining is a tool that assists humans in making better-informed decisions rather than replacing human cognition entirely.
- Data mining results need to be interpreted and contextualized by experts to avoid misinterpretation or blind reliance on automated insights.
- Human judgment is essential in considering ethical implications, biases, and critical factors that may not be captured by data mining algorithms.
- Data mining should be seen as a complement to human expertise, enabling more informed decision-making processes.
Misconception 4: Data mining always leads to valuable insights
Some individuals mistakenly assume that data mining always uncovers valuable insights. However, data mining is a complex process, and not all analyses lead to meaningful or actionable results.
- Data mining may uncover patterns that are statistically significant but irrelevant to the specific problem or objective.
- Poor data quality or incomplete datasets can negatively impact the accuracy and reliability of data mining results.
- Data mining requires careful validation and verification of findings to ensure the credibility and usefulness of the insights obtained.
Misconception 5: Larger datasets always yield better results
There is a common misconception that larger datasets always lead to better data mining results. While large datasets can offer more opportunities for discovering patterns, size alone does not guarantee superior insights.
- Data mining techniques should focus on relevant data that is directly related to the problem being investigated, rather than blindly analyzing vast amounts of irrelevant information.
- Data quality and suitability for the specific analysis are more critical than simply having a large volume of data.
- Data pre-processing techniques, such as feature selection and dimensionality reduction, can enhance the effectiveness of data mining algorithms when dealing with large datasets.
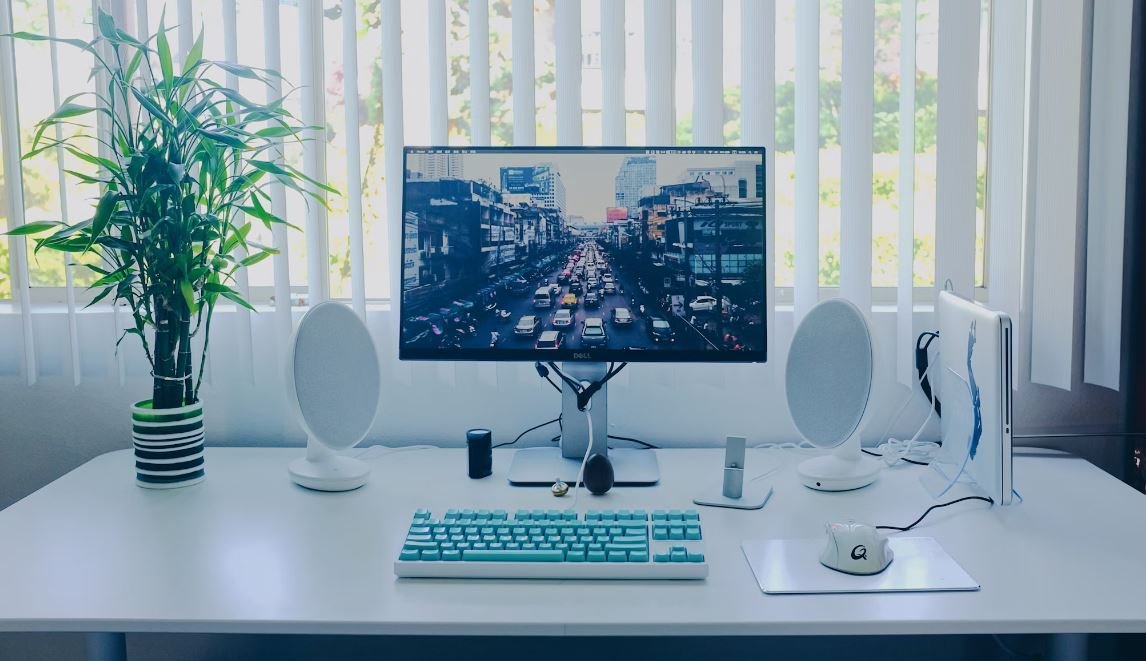
Data Mining in E-commerce
Table showing the growth of e-commerce sales from 2015 to 2020 in billions of dollars:
Year | Global E-commerce Sales |
---|---|
2015 | $1.548 |
2016 | $1.915 |
2017 | $2.304 |
2018 | $2.842 |
2019 | $3.535 |
2020 | $4.206 |
Data Mining in Healthcare
Table showing the number of new cancer cases and cancer deaths in the United States in 2019:
Cancer Type | New Cases | Cancer Deaths |
---|---|---|
Lung & Bronchus | 228,820 | 135,720 |
Breast | 268,600 | 41,760 |
Colorectal | 145,600 | 51,020 |
Prostate | 191,930 | 33,330 |
Pancreas | 57,600 | 47,050 |
Data Mining in Finance
Table showing the top 5 countries with the highest GDP in 2020 (in trillion US dollars):
Country | GDP |
---|---|
United States | $21.43 |
China | $14.34 |
Japan | $5.15 |
Germany | $3.86 |
United Kingdom | $2.83 |
Data Mining in Marketing
Table showing the average conversion rates for various marketing channels:
Marketing Channel | Average Conversion Rate |
---|---|
Email Marketing | 4.29% |
Organic Search | 2.95% |
Social Media | 1.95% |
Display Ads | 0.77% |
Affiliate Marketing | 0.54% |
Data Mining in Education
Table showing the average SAT scores by race/ethnicity:
Race/Ethnicity | Reading and Writing | Math | Total |
---|---|---|---|
Asian | 557 | 618 | 1175 |
White | 528 | 536 | 1064 |
Hispanic/Latino | 477 | 485 | 962 |
African American | 483 | 462 | 945 |
American Indian/Alaska Native | 486 | 480 | 966 |
Data Mining in Social Media
Table showing the number of monthly active users (MAU) on popular social media platforms:
Social Media Platform | MAU (in millions) |
---|---|
2,740 | |
YouTube | 2,291 |
2,000 | |
Facebook Messenger | 1,300 |
1,074 |
Data Mining in Politics
Table showing voter turnout in recent presidential elections:
Presidential Election | Voter Turnout (%) |
---|---|
2012 | 57.5 |
2016 | 55.7 |
2020 | 66.7 |
Data Mining in Sports
Table showing the world records in track and field events:
Event | Record (Men) | Record (Women) |
---|---|---|
100m Sprint | 9.58 seconds | 10.49 seconds |
200m Sprint | 19.19 seconds | 21.34 seconds |
High Jump | 2.45 meters | 2.09 meters |
Long Jump | 8.95 meters | 7.52 meters |
Shot Put | 23.12 meters | 22.63 meters |
Data Mining in Transportation
Table showing the busiest airports in the world by passenger traffic in 2019:
City | Country | Passenger Traffic (in millions) |
---|---|---|
Atlanta | United States | 110.5 |
Beijing | China | 100.9 |
Dubai | United Arab Emirates | 89.1 |
Los Angeles | United States | 88.1 |
Tokyo | Japan | 87.1 |
Conclusion
Data mining has become an essential tool across various domains, including e-commerce, healthcare, finance, marketing, education, social media, politics, sports, and transportation. By extracting valuable insights from large datasets, businesses are able to make informed decisions, improve customer experiences, and drive growth. The tables presented in this article highlight key data points within these sectors, demonstrating the power of data mining and its impact on decision-making processes. As technology advances and data availability continues to grow, data mining will undoubtedly play an even more significant role in shaping our future. Embracing this technology can lead to innovation and substantial improvements in various fields, ultimately benefiting individuals and society as a whole.
Frequently Asked Questions
What is data mining?
Data mining is the process of discovering patterns and information from large datasets using various computational techniques. It involves extracting valuable insights and knowledge from raw data, allowing businesses and organizations to make informed decisions based on the patterns and relationships found in the data.
Why is data mining important?
Data mining plays a crucial role in various industries and domains. It helps businesses understand customer preferences, improve marketing campaigns, detect fraudulent activities, optimize operations, and make data-driven decisions. By uncovering hidden patterns and trends in data, data mining provides valuable insights that can lead to increased efficiency, profitability, and competitive advantage.
What are some common data mining techniques?
There are several common data mining techniques used to extract meaningful information from large datasets. These include classification, clustering, regression analysis, association rule learning, anomaly detection, and prediction. Each technique serves a specific purpose and can be applied differently based on the nature of the data and the objective of the analysis.
How does data mining differ from data analysis?
While data mining and data analysis are closely related, they have distinct differences. Data analysis focuses on examining datasets to discover patterns, trends, and insights. It involves exploring the data using statistical methods, visualization techniques, and descriptive analytics. On the other hand, data mining specifically refers to the process of using computational algorithms to extract valuable information and patterns from the data.
What are the challenges of data mining?
Data mining presents several challenges that researchers and practitioners need to address. Some of the common challenges include data quality issues, data preprocessing and transformation, selecting appropriate algorithms and techniques, handling large datasets, dealing with noise and outliers, privacy and security concerns, and interpretability and validation of results.
What are some real-world applications of data mining?
Data mining has found applications in various industries and domains. Some common real-world applications include customer segmentation and targeting, fraud detection in financial transactions, recommendation systems in e-commerce, predictive maintenance in manufacturing, healthcare data analysis for disease prediction, sentiment analysis in social media, and market basket analysis for retail sales optimization.
What ethical considerations are associated with data mining?
Data mining raises several ethical considerations that need to be taken into account. Privacy is a significant concern, as the analysis of personal data can potentially infringe on individuals’ privacy rights. It is important to ensure that proper consent and anonymization measures are implemented. Fairness and non-discrimination are also important ethical considerations, as biased data or algorithms can lead to discriminatory outcomes.
How can data mining be used for fraud detection?
Data mining techniques can be employed to detect fraudulent activities in various domains, including finance, insurance, and e-commerce. By analyzing patterns and anomalies in transactional data, data mining algorithms can identify suspicious activities such as fraudulent credit card transactions or insurance claims. These techniques can help save businesses significant financial losses and protect consumers from fraud.
What are the future trends in data mining?
The field of data mining continues to evolve with advancements in technology and data availability. Some of the future trends in data mining include the integration of artificial intelligence and machine learning techniques, enhanced visualization and interactive analytics, handling and analyzing unstructured data such as text and images, and the application of data mining in emerging fields such as Internet of Things (IoT) and Big Data analytics.