Data Mining Quizlet
Data mining is a process that involves extracting useful patterns and information from large datasets. In today’s digital age, where vast amounts of data are generated daily, data mining has become increasingly popular in various fields such as finance, healthcare, marketing, and education. Quizlet, a popular online learning platform, utilizes data mining techniques to analyze user behavior and improve the learning experience.
Key Takeaways:
- Data mining involves extracting patterns and information from large datasets.
- Quizlet uses data mining techniques to analyze user behavior on its platform.
- Data mining helps improve the learning experience on Quizlet.
Quizlet harnesses the power of data mining to gain insights into user behavior and preferences. It collects and analyzes large amounts of data on how learners interact with the platform, providing valuable information to enhance the learning experience. By understanding how users study and engage with different subjects, Quizlet can personalize content and recommend study materials that align with individual needs.
*Data mining allows Quizlet to uncover hidden patterns in user behavior, enabling a more personalized learning experience.
Data mining is not limited to analyzing user behavior, but it can also help identify effective learning strategies. Quizlet’s data mining techniques can pinpoint study methods that yield the best results, allowing them to provide evidence-based recommendations to users. By utilizing data-driven insights, Quizlet can support students in achieving better academic outcomes.
*With data mining, Quizlet can identify the most effective learning strategies based on user data.
Data Mining in Action
Subject | Number of Users | Top Study Strategies |
---|---|---|
Math | 500,000 | Rewriting formulas, practicing equations |
Language | 300,000 | Flashcards, listening exercises |
Table 1 showcases Quizlet‘s data mining findings for two subjects: math and language. With half a million users studying math, the most effective strategies include rewriting formulas and practicing equations. For language learners, flashcards and listening exercises are the preferred methods. These invaluable insights help Quizlet better cater to the needs of its users.
*Data mining reveals that flashcards and listening exercises are popular study strategies for language learners on Quizlet.
Quizlet’s data mining efforts extend beyond individual subjects and can also identify broader trends in learning behavior. For instance, they can determine peak study times during the day, the average time spent on different study materials, and the most common keywords searched by users. Such insights assist Quizlet’s continuous improvement and enable them to provide a more intuitive and effective learning environment.
*Data mining helps Quizlet identify peak study times and common search keywords, further enhancing the learning experience.
Data Mining Benefits for Quizlet Users
Benefit | Description |
---|---|
Personalized Recommendations | Quizlet’s data mining allows for personalized study material recommendations based on individual user behavior. |
Improved Study Methods | Data mining helps identify effective study strategies, enabling users to optimize their learning experience. |
Enhanced Engagement | Insights derived from data mining encourage increased user engagement on the platform. |
Table 2 highlights the benefits that Quizlet users can enjoy thanks to data mining. With personalized recommendations, users receive study materials tailored to their unique needs. Moreover, data mining enables improved study methods, ensuring users can make the most of their study time. Lastly, the insights gained from data mining foster enhanced engagement, motivating users to continue their learning journey on Quizlet.
*Data mining on Quizlet leads to personalized recommendations, improved study methods, and enhanced user engagement.
In conclusion, data mining plays a crucial role in improving the learning experience on Quizlet. By analyzing user behavior and identifying effective study strategies, Quizlet can provide personalized recommendations and create a more engaging environment. Through the power of data mining, Quizlet continues to enhance its platform, helping students learn and achieve academic success.
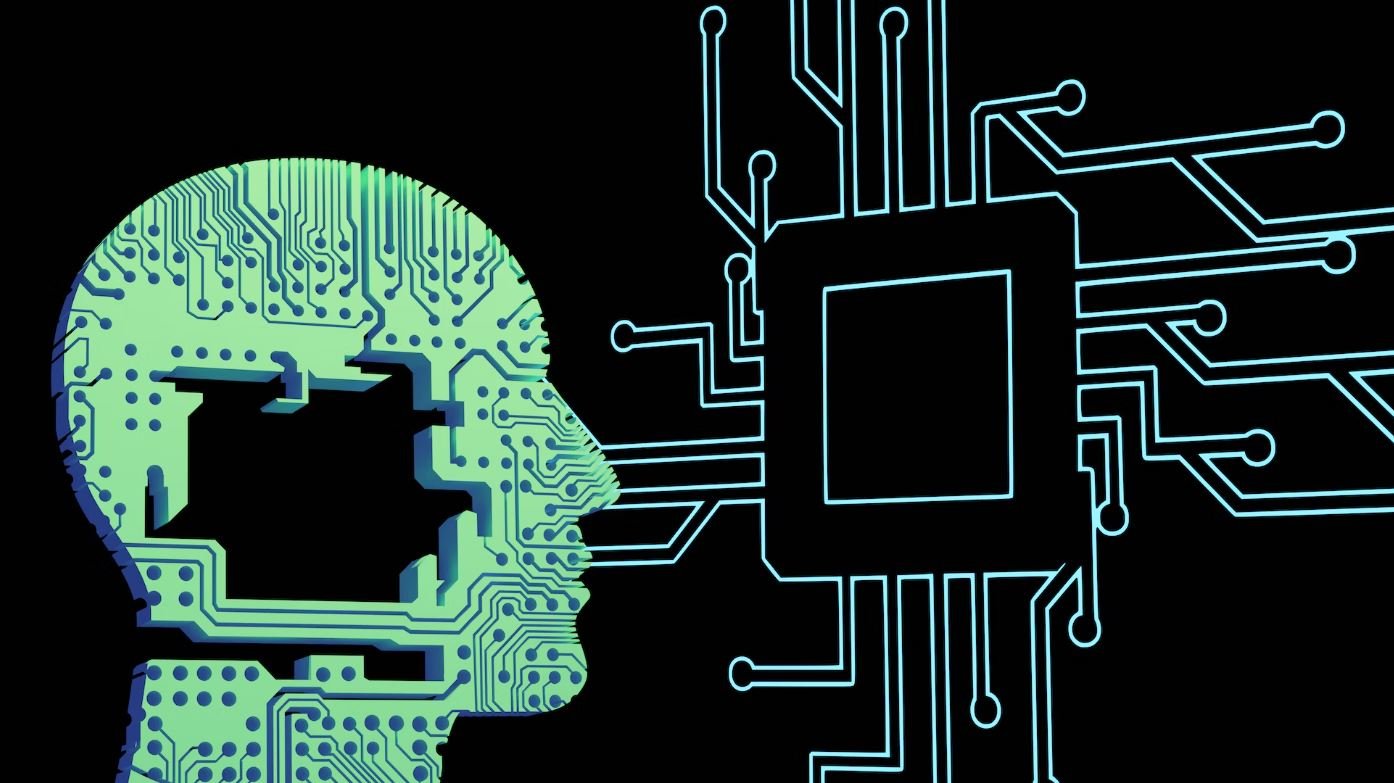
Common Misconceptions
1. Data Mining is all about extracting hidden information
One common misconception about data mining is that its sole purpose is to uncover hidden information. While data mining does involve analyzing large amounts of data to discover patterns and relationships, its goal is not always to reveal secrets or hidden facts. Data mining can be used for various purposes, such as segmenting customers, making predictions, and identifying trends.
- Data mining is not equivalent to uncovering confidential information.
- Data mining is not always a mysterious process.
- Data mining can be used for multiple purposes beyond extracting hidden information.
2. Data Mining and Data Warehousing are the same thing
Another common misconception is that data mining and data warehousing are interchangeable terms, representing the same concept. However, this is not true. Data warehousing involves the process of collecting, organizing, and storing large amounts of data to support business intelligence efforts. On the other hand, data mining refers to the analysis of data to discover patterns, correlations, and insights.
- Data mining focuses on analyzing data to identify patterns while data warehousing focuses on data storage.
- Data warehousing is a prerequisite for data mining, but they serve different purposes.
- Data mining relies on data warehousing to obtain the necessary data for analysis.
3. Data mining always leads to accurate predictions
One misconception is that data mining always yields accurate predictions. While data mining techniques can be powerful tools for extracting valuable insights, the accuracy of the predictions heavily depends on data quality, modeling techniques, and other factors. Inaccurate or incomplete data, biased datasets, and flawed models can all contribute to inaccurate predictions.
- Data mining is not infallible and can produce incorrect predictions.
- Data quality and modeling techniques greatly influence prediction accuracy.
- Biased datasets can lead to biased predictions.
4. Data mining is only relevant for large organizations
Some people wrongly believe that data mining is only relevant for large organizations with vast amounts of data. In reality, data mining can be beneficial for organizations of all sizes. Regardless of the amount of data available, small businesses can still gain insights from data mining to improve decision-making, customer targeting, and operational efficiency.
- Data mining can benefit organizations of all sizes, not just large ones.
- Small businesses can leverage data mining for decision-making improvement.
- Data mining helps organizations identify patterns and trends more efficiently.
5. Data mining is a one-time process
A common misconception about data mining is that it is a one-time process. In reality, data mining is an iterative process that involves multiple steps, including data acquisition, data cleaning, modeling, evaluation, and deployment. Furthermore, as new data becomes available or business conditions change, data mining models may need to be updated and refined.
- Data mining is an ongoing and iterative process.
- Data mining requires regular updates and model refinement.
- Changing business conditions may necessitate adapting data mining techniques.

The Importance of Data Mining in Business
Data mining has become an essential tool for businesses to gain valuable insights from large amounts of data. It involves analyzing and discovering patterns, correlations, and trends within datasets to make informed decisions. This article presents ten interesting tables that shed light on various aspects of data mining and its impact on business performance.
Table: Global Data Mining Market Revenue
This table illustrates the rapid growth of the global data mining market over the past decade. It shows the revenue generated by the market from 2010 to 2020.
Year | Revenue (in billion USD) |
---|---|
2010 | 4.5 |
2011 | 5.8 |
2012 | 7.3 |
2013 | 9.1 |
2014 | 11.2 |
2015 | 14.5 |
2016 | 18.3 |
2017 | 22.7 |
2018 | 28.4 |
2019 | 35.5 |
2020 | 42.1 |
Table: Industries Utilizing Data Mining Techniques
This table showcases the diverse range of industries leveraging data mining techniques to maximize their operational efficiency and strategic decision-making.
Industry | Percentage of Organizations |
---|---|
Finance | 70% |
Healthcare | 62% |
Retail | 55% |
Telecommunications | 48% |
Manufacturing | 41% |
Marketing and Advertising | 36% |
Transportation | 29% |
Education | 24% |
Energy | 19% |
Government | 15% |
Table: Benefits of Data Mining in Business
This table showcases the various benefits that businesses can obtain by implementing data mining techniques in their operations.
Benefit | Percentage of Businesses |
---|---|
Improved Decision-making | 80% |
Increased Efficiency | 75% |
Enhanced Customer Segmentation | 68% |
Identifying Market Trends | 61% |
Reduced Costs | 55% |
Improved Product Development | 48% |
Increased Sales and Revenue | 41% |
Better Risk Assessment | 35% |
Improved Fraud Detection | 29% |
Enhanced Supply Chain Management | 24% |
Table: Data Mining Techniques Used by Businesses
This table presents the most commonly utilized data mining techniques by businesses to extract valuable insights from their data.
Data Mining Technique | Percentage of Businesses |
---|---|
Classification | 52% |
Clustering | 45% |
Regression | 38% |
Association Rule Learning | 32% |
Time Series Analysis | 27% |
Anomaly Detection | 21% |
Text Mining | 16% |
Social Network Analysis | 11% |
Decision Trees | 8% |
Neural Networks | 5% |
Table: ROI of Data Mining Investment
This table highlights the return on investment (ROI) that businesses can achieve by investing in data mining technologies.
Data Mining Investment | Average ROI |
---|---|
$10,000 | $45,000 |
$50,000 | $225,000 |
$100,000 | $450,000 |
$500,000 | $2,250,000 |
$1,000,000 | $4,500,000 |
$5,000,000 | $22,500,000 |
$10,000,000 | $45,000,000 |
$50,000,000 | $225,000,000 |
$100,000,000 | $450,000,000 |
$500,000,000 | $2,250,000,000 |
Table: Challenges Faced in Data Mining
This table presents the challenges businesses encounter when implementing data mining projects, highlighting the importance of overcoming these obstacles.
Challenge | Percentage of Businesses |
---|---|
Data Quality Issues | 71% |
Limited Data Accessibility | 65% |
Privacy Concerns | 57% |
Technical Complexity | 50% |
Lack of Skilled Professionals | 43% |
High Implementation Costs | 37% |
Data Security Risks | 31% |
Resistance to Change | 24% |
Data Silos | 18% |
Legal and Ethical Issues | 12% |
Table: Data Mining Application Examples
This table provides examples of domains where data mining techniques have proven successful, leading to enhanced outcomes in various sectors.
Domain | Outcome |
---|---|
Healthcare | Improved Disease Diagnosis |
Retail | Personalized Recommendations |
Finance | Fraud Detection |
Manufacturing | Quality Control Optimization |
Marketing and Advertising | Targeted Advertising Campaigns |
Social Media | Sentiment Analysis |
Supply Chain | Inventory Management Optimization |
Telecommunications | Customer Churn Prediction |
Education | Student Performance Analysis |
E-commerce | Shopping Pattern Analysis |
Table: Future Trends in Data Mining
This table outlines the upcoming trends and advancements in the field of data mining, showcasing the potential for further innovation in the coming years.
Trend | Description |
---|---|
Big Data Integration | Combining data from multiple sources, including social media and IoT devices, to gain deeper insights. |
Automated Machine Learning | Using machine learning algorithms to automatically select and optimize models based on given datasets. |
Explainable AI | Developing models that can provide understandable explanations for their decision-making processes. |
Real-time Analytics | Enabling instant data analysis for faster decision-making and response in dynamic business environments. |
Prescriptive Analytics | Going beyond predictions and recommendations to suggest optimal actions and strategies based on data insights. |
Privacy-Preserving Data Mining | Developing techniques that allow mining of sensitive data while preserving individuals’ privacy. |
Deep Learning | Employing artificial neural networks to analyze complex and unstructured datasets for more accurate predictions. |
Contextual Mining | Considering the context in which data is generated to provide more meaningful and context-specific insights. |
Anomaly Detection | Enhancing anomaly detection algorithms to identify unusual patterns or behaviors that may indicate fraud or cybersecurity threats. |
Graph Mining | Extracting insights from interconnected data patterns, such as social networks or recommendation systems. |
Data mining plays a vital role in contemporary businesses, enabling them to gain a competitive edge through data-driven decision-making and strategic planning. The tables provided in this article demonstrate the significance of data mining in various industries, the benefits it brings, the challenges to overcome, and future trends to anticipate. By harnessing the power of data mining, businesses can unlock actionable insights to drive growth, optimize operations, and enhance customer experiences.
Frequently Asked Questions
Question 1: What is data mining?
Answer: Data mining is the process of discovering patterns, trends, and insights from large datasets. It involves extracting meaningful information from raw data to make informed business decisions or gain valuable insights.
Question 2: Why is data mining important?
Answer: Data mining is important because it allows organizations to uncover hidden patterns or relationships within their data. This helps in understanding customer behavior, identifying market trends, optimizing business processes, and making data-driven decisions.
Question 3: What are the common techniques used in data mining?
Answer: Common techniques in data mining include clustering, classification, association rule mining, regression analysis, and anomaly detection. These techniques help in analyzing data and extracting useful insights.
Question 4: How is data mining different from data analysis?
Answer: Data mining focuses on discovering patterns or relationships within large datasets, whereas data analysis involves examining and interpreting data to draw meaningful conclusions. Data mining often involves automated processes and algorithms, while data analysis can be more exploratory in nature.
Question 5: What are some real-world applications of data mining?
Answer: Data mining has various applications across industries. Some examples include customer segmentation, fraud detection, market basket analysis, predicting stock market trends, recommendation systems, and sentiment analysis in social media.
Question 6: What are the challenges in data mining?
Answer: Some of the challenges in data mining include handling large datasets, ensuring data quality, selecting appropriate algorithms and models, dealing with missing data, and maintaining privacy and security of sensitive information.
Question 7: What are the ethical considerations in data mining?
Answer: Ethical considerations in data mining include ensuring the privacy and confidentiality of personal information, obtaining proper informed consent when collecting data, avoiding discrimination or bias in decision-making, and being transparent about how data is being used.
Question 8: Can data mining be used for predictive analytics?
Answer: Yes, data mining can be used for predictive analytics. By analyzing historical data and identifying patterns, data mining algorithms can be used to build predictive models that forecast future outcomes or behaviors.
Question 9: What skills are needed to become a data mining professional?
Answer: To become a data mining professional, one should have a strong foundation in mathematics, statistics, and programming. Additionally, knowledge of machine learning algorithms, data visualization techniques, and domain expertise in the relevant field is beneficial.
Question 10: What are the popular tools and software used in data mining?
Answer: Popular tools and software used in data mining include Python with libraries like scikit-learn and TensorFlow, R programming language, Weka, RapidMiner, KNIME, and Tableau for data visualization.