Data Mining Risks
As more and more organizations gather and analyze vast amounts of data, the risks associated with data mining become increasingly important to understand. Data mining, often used for market research, fraud detection, and decision-making purposes, entails extracting patterns and knowledge from large datasets. However, the process can also lead to privacy breaches, discrimination concerns, and inaccurate predictions.
Key Takeaways:
- Data mining involves extracting patterns and knowledge from large datasets.
- There are potential risks associated with data mining such as privacy breaches and discrimination concerns.
- Inaccurate predictions can occur as a result of faulty data or flawed algorithms.
Data mining poses a potential threat to privacy, as it involves analyzing sensitive personal information. Individuals are increasingly cautious about sharing their data due to fears of identity theft, data breaches, and unauthorized access. Even when data is anonymized, it can still be re-identified by combining it with external datasets, creating a privacy risk.
It is essential for organizations to ensure proper data anonymization techniques are in place to protect individuals’ privacy.
Data mining processes can unintentionally lead to discriminatory outcomes. When algorithms are trained on biased datasets or use discriminatory attributes, they may perpetuate existing biases or create new ones. For example, an algorithm used in the hiring process may negatively impact certain groups if it is trained on historical data that reflects past discrimination.
Data mining projects need to be carefully monitored and audited to identify and mitigate any biases that may arise.
Inaccurate predictions can arise from flawed data or faulty algorithms. If there are errors or omissions in the dataset used for training, the resulting predictions may be unreliable or misleading. Faulty algorithms can also lead to poor predictions if they are not designed properly or if they are applied to inappropriate contexts.
Data validation processes should be implemented to ensure the quality and accuracy of the data used in data mining projects.
Examples of Data Mining Risks:
To illustrate the potential risks associated with data mining, consider the following examples:
Risk | Example |
---|---|
Privacy Breaches | Healthcare provider accidentally exposes patients’ medical records, leading to potential identity theft. |
Discrimination | Financial institution denies loan applications based on race or gender, due to biases in their data analysis methods. |
Inaccurate Predictions | A retail store sends a promotional offer to a customer who had recently passed away, as their data had not been updated. |
In order to mitigate the risks associated with data mining, organizations must prioritize ethical and responsible data practices. This includes obtaining informed consent from individuals whose data is being used, promoting transparency in algorithms and data usage, and regularly evaluating and updating models and processes to address biases and inaccuracies.
Best Practices to Address Data Mining Risks:
- Implement strict data anonymization techniques to protect individuals’ privacy.
- Audit and monitor data mining projects to identify and mitigate any biases.
- Validate and ensure the quality of data used in data mining processes.
- Promote ethical data practices by obtaining informed consent and being transparent about data usage.
- Evaluate and update algorithms and models regularly to address biases and inaccuracies.
While data mining can offer valuable insights and benefits, it is crucial to be aware of the associated risks. By understanding and effectively managing these risks, organizations can harness the power of data mining while minimizing potential negative impacts.
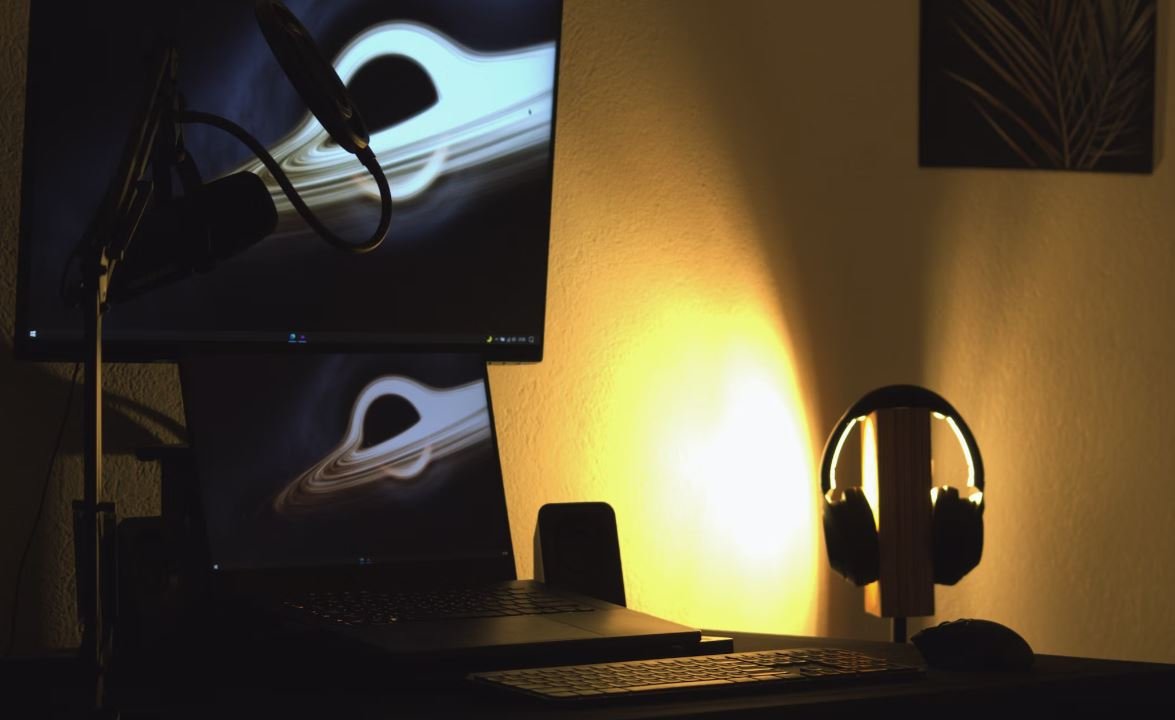
Common Misconceptions
Misconception 1: Data mining is always invasive
One common misconception about data mining is that it is inherently invasive and a violation of privacy. While it is true that data mining can potentially collect sensitive information, not all data mining activities are meant to invade privacy. Many organizations use data mining techniques to analyze patterns and trends in data without the intention of identifying individuals. It is important to understand that data mining can be done ethically and responsibly.
- Data mining can be used for anonymized market research.
- Data mining can help identify trends and patterns for product development.
- Data mining can benefit society by improving healthcare and public safety.
Misconception 2: Data mining is always accurate
Another common misconception is that data mining is always accurate and infallible. While data mining algorithms are powerful tools, they are not immune to errors and limitations. Inaccurate or incomplete data can lead to misleading results. It is crucial to understand the limitations of data mining and to validate the results before making important decisions based solely on the outcomes of data mining.
- Data mining can result in false positives and false negatives.
- Data mining accuracy depends on the quality of the data being analyzed.
- Data mining should be used as a tool to support decision-making rather than the sole basis for decision-making.
Misconception 3: Data mining is always used for malicious purposes
There is a misconception that data mining is always used for malicious purposes such as identity theft or surveillance. While data mining techniques can be misused, it is important to note that data mining has many legitimate applications that benefit society. For example, data mining can be used to identify patterns of fraudulent behavior and prevent financial losses.
- Data mining can be used in healthcare to identify patterns of disease outbreaks.
- Data mining can assist in detecting and preventing credit card fraud.
- Data mining can help improve customer relationship management by analyzing customer behavior.
Misconception 4: Data mining is a threat to job security
Some individuals may have the misconception that data mining poses a threat to job security, as it is often associated with automation and the potential to replace human labor. However, data mining is a tool that complements human expertise rather than replacing it entirely. Data mining requires skilled professionals to analyze, interpret, and apply the results to solve complex problems.
- Data mining creates new job opportunities in fields such as data analysis and data science.
- Data mining can enhance productivity and efficiency in various industries.
- Data mining can free up human resources from repetitive tasks to focus on higher-value work.
Misconception 5: Data mining is only for large organizations
Lastly, it is a common misconception that data mining is exclusive to large organizations with vast amounts of data. While large organizations may have more data to analyze, data mining techniques can be applied to data sets of any size. Even small businesses can benefit from data mining by gaining insights into customer preferences, optimizing their operations, and making informed decisions.
- Data mining can help small businesses identify market opportunities and target their marketing efforts.
- Data mining can assist in optimizing inventory management for better cost control.
- Data mining can help small organizations personalize their offerings to improve customer satisfaction.
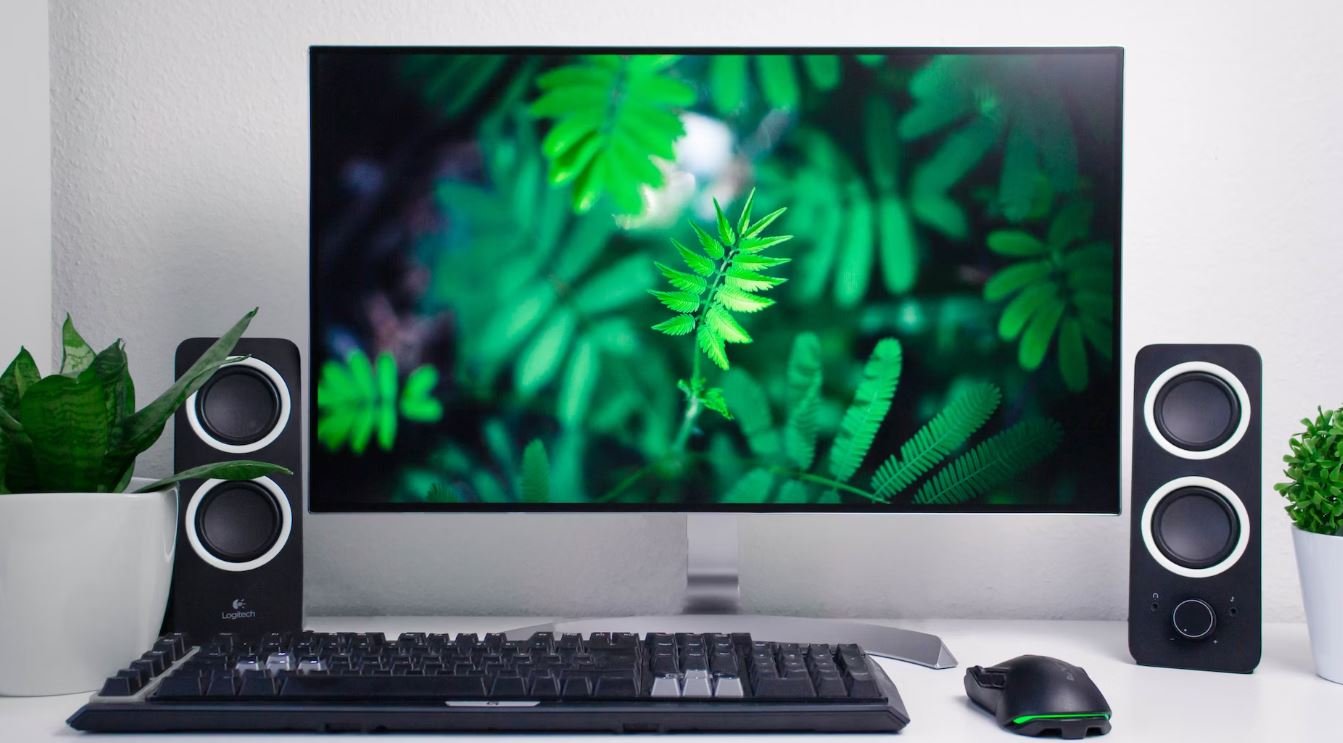
Data Breach Statistics
The table below shows the number of reported data breaches from 2015 to 2020, highlighting the increasing trend of cyber threats.
Year | Number of Data Breaches |
---|---|
2015 | 781 |
2016 | 1,093 |
2017 | 1,579 |
2018 | 1,244 |
2019 | 1,473 |
2020 | 1,001 |
Data Mining Techniques Used by Companies
The following table displays the most commonly utilized data mining techniques by companies engaged in extracting meaningful insights from large datasets.
Data Mining Technique | Percentage of Companies |
---|---|
Cluster Analysis | 64% |
Decision Trees | 56% |
Regression Analysis | 48% |
Neural Networks | 42% |
Association Rules | 38% |
Data Mining Challenges Faced by Businesses
This table outlines the main challenges encountered by businesses when embracing data mining practices.
Challenge | Frequency |
---|---|
Data Quality | 86% |
Privacy Concerns | 79% |
Data Protection Regulations | 72% |
Insufficient Skills | 68% |
Integration of Data Sources | 61% |
Impact of Data Mining on Personal Privacy
This table demonstrates the effects of data mining on personal privacy by illustrating the types of information commonly extracted.
Data Extracted | Percentage of Instances |
---|---|
Email Addresses | 76% |
Phone Numbers | 63% |
Addresses | 58% |
Demographic Information | 45% |
Internet Browsing History | 37% |
Data Mining in the Healthcare Industry
The table below showcases data mining applications within the healthcare industry, offering valuable insights for patient care and treatment.
Data Mining Application | Benefit |
---|---|
Disease Prediction | Enhanced early detection |
Drug-Drug Interaction Analysis | Safer medication practices |
Medical Image Analysis | Improved diagnostic accuracy |
Healthcare Fraud Detection | Reduction in fraudulent claims |
Patient Segmentation | Personalized treatment plans |
Data Mining and E-Commerce
The table presents how data mining is applied in the e-commerce sector, resulting in enhanced customer experiences and improved sales.
Data Mining Application | Benefit |
---|---|
Market Basket Analysis | Optimized product recommendations |
User Behavior Analysis | Personalized shopping experiences |
Price Optimization | Increased revenue and profitability |
Churn Prediction | Improved customer retention |
Ad Campaign Optimization | Better targeting and ROI |
Legal Issues Surrounding Data Mining
This table highlights the legal concerns and issues that arise when conducting data mining activities.
Legal Issue | Description |
---|---|
Data Ownership | Confusion over who owns collected data |
Data Sharing | Legal restrictions on sharing data with third parties |
Consent and Privacy | Ensuring compliance with privacy regulations |
Data Security | Protecting data from unauthorized access or breaches |
Algorithm Bias | Addressing biases and discrimination in algorithms |
Data Mining Ethical Considerations
The table below outlines the ethical factors that must be taken into account when engaging in data mining activities.
Ethical Consideration | Description |
---|---|
Transparency | Keeping individuals aware of how their data is used |
Data Anonymization | Protecting individual privacy through anonymization techniques |
Fairness and Bias | Avoiding discrimination and biases in data analysis |
Data Accuracy | Maintaining the accuracy and reliability of data |
Accountability | Assuming responsibility for the consequences of data mining |
Conclusion
Data mining brings valuable insights and opportunities for various industries, allowing businesses to make data-driven decisions. However, the increasing frequency of data breaches, privacy concerns, legal issues, and ethical considerations remind us of the risks involved. It is crucial for organizations to prioritize data security, adhere to privacy regulations, and conduct data mining in a responsible and transparent manner. By balancing the rewards of data mining with the protection of individual privacy and ethical practices, businesses can harness its potential while mitigating associated risks.
Frequently Asked Questions
What are the risks associated with data mining?
Data mining involves certain risks that organizations need to be aware of. These risks include privacy breaches, data leakage, biased decision-making, legal and ethical implications, and security vulnerabilities.
What are the privacy risks in data mining?
Data mining poses privacy risks as it involves collecting and analyzing large amounts of data, often from multiple sources. There is a risk of exposing sensitive personal information if proper security measures are not in place. Individuals may also feel their privacy has been violated if their data is used without their knowledge or consent.
How can data leakage occur in data mining?
Data leakage can occur in data mining when sensitive information is unintentionally or intentionally disclosed to unauthorized parties. This can happen through insecure data storage, weak access controls, or even insider threats. Proper data protection protocols are essential to prevent data leakage.
What are the risks of biased decision-making in data mining?
Data mining algorithms can inadvertently introduce biases if the data used for training is biased. This can result in automated decisions that discriminate against certain individuals or groups. It is crucial to ensure fairness and mitigate bias in data mining models.
What legal and ethical implications are associated with data mining?
Data mining raises legal and ethical concerns such as compliance with data protection regulations, ensuring informed consent for data collection, and respecting data subjects’ rights. Organizations must handle data mining activities in a responsible and transparent manner to avoid legal and ethical repercussions.
How can security vulnerabilities be a risk in data mining?
Data mining involves handling large volumes of data, which can make organizations vulnerable to security breaches. Malicious actors may attempt to gain unauthorized access to the data, leading to data theft or other cyberattacks. Implementing robust security measures is crucial to protect against such vulnerabilities.
What steps can organizations take to minimize data mining risks?
Organizations can take several steps to minimize data mining risks. They should implement strong data protection measures, including encryption and access controls. Regular security audits and updates can help identify and address vulnerabilities. Additionally, organizations should prioritize ethical considerations and ensure compliance with relevant regulations.
Who is responsible for managing data mining risks?
Managing data mining risks is a shared responsibility within organizations. It involves collaboration between data scientists, IT professionals, legal teams, and stakeholders. Leadership should provide guidance and allocate necessary resources to effectively manage and mitigate the risks associated with data mining.
How can individuals protect their privacy in the era of data mining?
Individuals can take steps to protect their privacy in the era of data mining. They can be cautious about sharing personal information online, regularly review and update their privacy settings on websites and social media platforms, and exercise their rights regarding data collection and sharing. Being aware of privacy risks and staying informed about data protection practices is important.
Are there any specific industries more prone to data mining risks?
Data mining risks are present in various industries that heavily rely on data analytics, such as finance, healthcare, marketing, and technology. However, any organization that collects and analyzes data is susceptible to these risks, regardless of the industry.