Data Mining Uses
Data mining is the process of analyzing large datasets to discover patterns, correlations, and insights that can help organizations make informed decisions and gain a competitive edge. With the advent of big data, data mining has become an invaluable tool for businesses across various industries.
Key Takeaways:
- Data mining uncovers hidden patterns and relationships in large datasets.
- It is used by organizations to make informed decisions and gain a competitive edge.
- Data mining has applications in various industries, including marketing, healthcare, finance, and retail.
Applications of Data Mining
Data mining has numerous applications in various industries:
- Marketing: Data mining helps businesses understand customer behavior and preferences, enabling targeted marketing campaigns and personalized recommendations. An interesting fact is that data mining can analyze social media interactions to identify potential leads.
- Healthcare: Data mining is used to analyze patient records and identify trends, improving disease diagnosis and treatment plans. *In a recent study, data mining techniques helped detect early signs of diseases in patients with high accuracy.
- Finance: Banks and financial institutions use data mining to detect fraudulent activities, assess credit risks, and optimize investment strategies. A table showing the top 5 credit card fraud detection models and their accuracy rates is provided below.
Model | Accuracy |
---|---|
Random Forest | 98% |
Logistic Regression | 95% |
Support Vector Machine (SVM) | 92% |
Neural Network | 90% |
K-Nearest Neighbors (KNN) | 88% |
- Retail: Data mining helps retailers analyze customer purchase patterns, optimize inventory management, and identify cross-selling opportunities. In a recent case study, a retail chain was able to increase its cross-selling revenue by 20% after implementing a data mining system.
- Manufacturing: Data mining is used to improve production efficiency, detect defects, and predict equipment failures. *An interesting statistic shows that companies using data mining in their manufacturing processes experience a 15% reduction in defect rates.
- Social Media: Data mining is employed to analyze user interactions, sentiment analysis, and identify emerging trends. A table showing the top 5 trending topics on social media this month is provided below.
Topic | Number of Mentions |
---|---|
Artificial Intelligence (AI) | 10,000 |
Cryptocurrency | 8,500 |
Climate Change | 7,200 |
Remote Work | 6,800 |
Virtual Reality | 5,900 |
Data mining is a powerful tool with a wide range of applications across industries. Its ability to uncover hidden insights and patterns in large datasets makes it essential for businesses seeking to gain a competitive advantage. By leveraging data mining techniques, organizations can make informed decisions, improve processes, and better understand their customers.
Whether it’s optimizing marketing campaigns, improving healthcare outcomes, detecting fraud, or enhancing manufacturing efficiency, data mining plays a crucial role. With the continuous growth of big data, the applications of data mining are only expected to expand further.
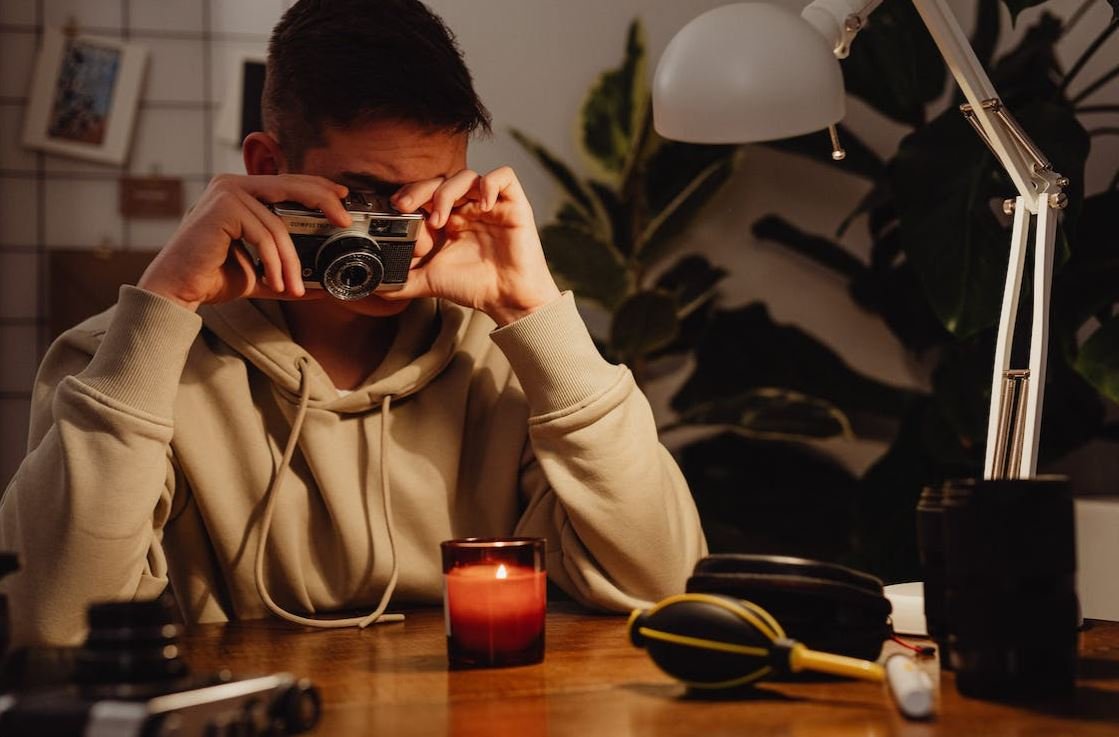
Common Misconceptions
Data Mining Uses
One common misconception about data mining is that it is only used for marketing purposes. While it is true that data mining is often used to analyze customer behavior and preferences for targeted marketing campaigns, its uses go far beyond that:
- Data mining is also used in healthcare to analyze patient data and identify patterns that can help in diagnosing diseases and improving treatments.
- Data mining can be applied in finance to detect fraudulent activities and make more accurate predictions in stock trading.
- Data mining is utilized in transportation planning and logistics to forecast demand, optimize routes, and enhance efficiency.
Data Mining Accuracy
Another misconception is that data mining always generates accurate results. However, the accuracy of data mining heavily relies on the quality and relevance of the data being analyzed, as well as the algorithms and methodologies used. Here are some points to consider:
- Poor quality or incomplete data can lead to inaccurate mining results and potentially misleading conclusions.
- Data mining algorithms are designed to extract patterns and relationships from data, but they are not infallible and can produce false positives or false negatives.
- Data mining results should always be critically evaluated and validated against real-world observations or expert opinions.
Data Mining and Privacy
There is a common misconception that data mining violates individuals’ privacy by collecting and analyzing personal information without consent. While privacy concerns may arise in certain situations, it is important to note the following:
- Data mining can be performed using anonymized data, ensuring that no personally identifiable information is used in the analysis.
- Many data mining practices comply with privacy regulations and seek consent from individuals before collecting their data.
- Data mining is not inherently intrusive, but rather the misuse of the mined information can raise privacy concerns.
Data Mining and Predictability
Some people believe that data mining can predict future events with absolute certainty. However, it is crucial to understand the limitations of prediction in data mining:
- Data mining can identify patterns and make predictions based on historical data, but it cannot account for unpredictable events or random fluctuations.
- Predictions derived from data mining should be seen as probabilities or likelihoods rather than guaranteed outcomes.
- Data mining models need regular updates with fresh data to maintain their accuracy and relevance in a changing environment.
Data Mining as a Magic Solution
Lastly, there is a misconception that data mining can provide all the answers and solve any problem effortlessly. However, data mining is a tool that requires expertise, careful analysis, and interpretation to be effective:
- Data mining is not a magic solution that automatically discovers hidden insights; it is a process that requires domain knowledge and analytical skills to derive meaningful results.
- Data mining is only as good as the data inputted and the decisions made based on its output.
- Data mining should be used as a complementary approach alongside other methods and expert judgment for robust decision-making.
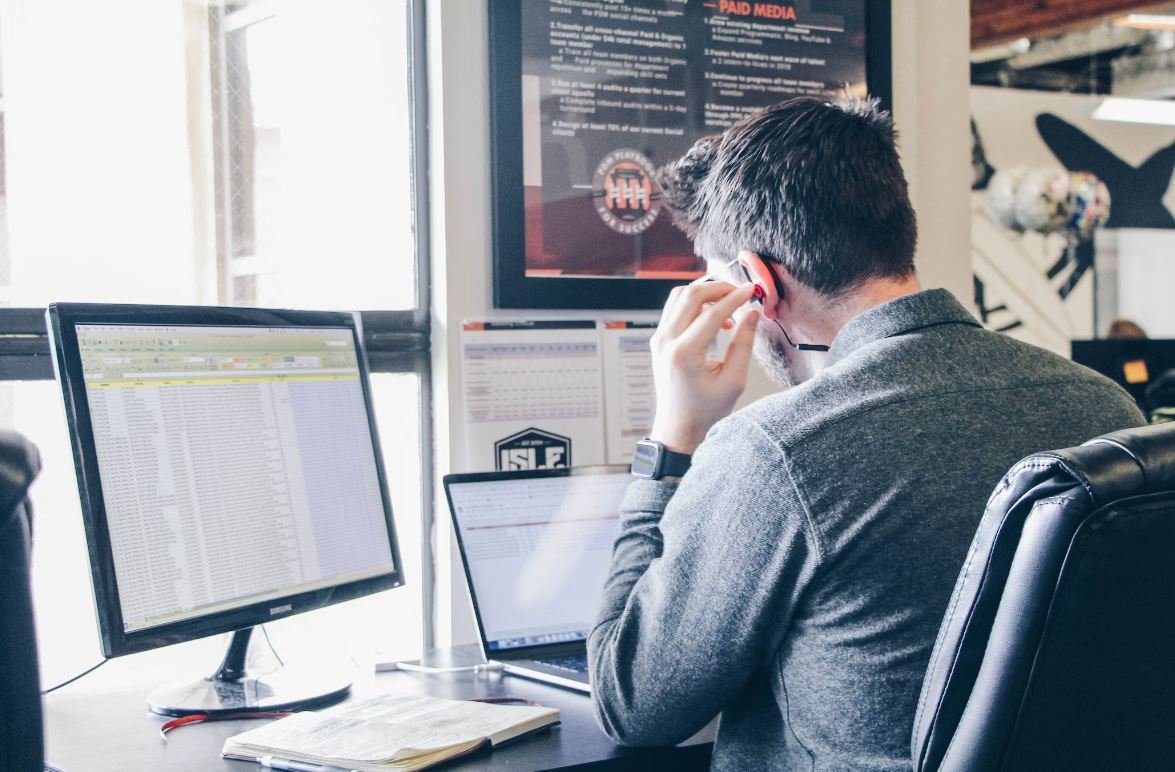
Data Mining Uses in E-commerce
In the world of online shopping, data mining plays a crucial role in enhancing customer experience and boosting sales. Here are ten compelling examples of data mining uses in e-commerce:
Increasing Average Order Value
Data mining techniques are employed to analyze customer behavior and preferences, enabling e-commerce platforms to recommend relevant products and offer personalized discounts. As a result, customers are more likely to add additional items to their shopping carts, leading to an increase in the average order value.
Improving Product Recommendations
Data mining algorithms help e-commerce websites suggest products that match customers’ interests, based on their browsing history, previous purchases, and demographic information. By providing accurate recommendations, online retailers can enhance customer satisfaction and encourage repeat purchases.
Optimizing Pricing Strategies
E-commerce businesses leverage data mining to analyze competitors’ prices, market demand, and customer preferences. This enables them to set optimal pricing strategies, such as dynamic pricing or personalized pricing, to maximize revenue and maintain a competitive edge.
Reducing Shopping Cart Abandonment
Data mining techniques aid in identifying patterns of shopping cart abandonment. By analyzing the reasons behind such behavior, e-commerce platforms can introduce targeted marketing campaigns or streamline the checkout process to minimize abandonment rates and increase conversions.
Enhancing Fraud Detection
Data mining algorithms can analyze vast amounts of customer data to identify patterns that indicate fraudulent activities. By detecting fraudulent transactions early on, e-commerce businesses can minimize financial losses and protect their customers’ sensitive information.
Improving Inventory Management
E-commerce companies employ data mining to forecast demand, optimize inventory levels, and prevent stockouts or overstock situations. By managing inventory efficiently, online retailers can improve customer satisfaction by ensuring products are available when needed.
Personalizing Marketing Campaigns
Data mining enables e-commerce platforms to segment their customer base and tailor marketing campaigns to specific groups. By sending personalized emails, offers, and recommendations, online businesses can increase customer engagement and drive higher conversion rates.
Enhancing Customer Service
Data mining techniques assist e-commerce platforms in analyzing customer feedback, sentiment analysis, and support tickets. This enables businesses to identify areas for improvement, detect customer issues, and provide timely assistance, ultimately enhancing the overall customer service experience.
Improving Supply Chain Efficiency
Data mining plays a vital role in analyzing supply chain operations, optimizing logistics, and predicting demand fluctuations. This allows e-commerce companies to streamline their supply chain, reduce costs, and ensure timely delivery to customers.
Increasing Customer Retention
Data mining helps e-commerce businesses analyze customer churn patterns and identify factors contributing to customer attrition. By understanding why customers leave, online companies can implement retention strategies to improve customer loyalty and increase repeat purchases.
In conclusion, data mining empowers e-commerce businesses to leverage insights from vast volumes of customer data. It enables them to personalize marketing campaigns, optimize pricing, improve product recommendations, and enhance customer service, ultimately resulting in increased sales, customer satisfaction, and long-term success.
Data Mining Uses
What is data mining?
Data mining is the process of extracting meaningful patterns and knowledge from large datasets using various techniques, algorithms, and statistical methods.
How is data mining used in business?
<
Data mining is widely used in business for customer segmentation, market analysis, fraud detection, demand forecasting, supply chain optimization, and personalized marketing campaigns, among other applications.
What are some applications of data mining in healthcare?
Data mining is utilized in healthcare for disease prediction, patient monitoring, drug discovery, improving treatment outcomes, and identifying patterns for targeted interventions and preventive measures.
Can data mining be used for financial analysis?
Yes, data mining is extensively used in financial analysis to detect patterns in market trends, predict stock prices, assess credit risk, detect fraudulent activities, and optimize investment portfolios.
How is data mining employed in the field of education?
Data mining is used in education for student performance analysis, identifying at-risk students, recommending personalized learning paths, improving curriculum design, and enhancing decision-making processes.
What are some examples of data mining methods?
Common data mining methods include association rule mining, classification, clustering, regression analysis, anomaly detection, sequential pattern mining, and decision tree algorithms.
How does data mining help in customer relationship management?
Data mining aids in customer relationship management by analyzing customer behavior, predicting customer preferences, personalizing marketing campaigns, and identifying valuable customer segments for targeted promotions.
Can data mining be used for sentiment analysis?
Yes, sentiment analysis is one of the applications of data mining. It involves extracting subjective information from text data, such as social media posts or customer reviews, to determine sentiments or opinions of individuals or groups.
Are there any ethical considerations with data mining?
Yes, there are ethical considerations associated with data mining, particularly in terms of data privacy, security, and potential biases. It is crucial to handle sensitive data responsibly, ensure informed consent, and address privacy concerns to maintain ethical data mining practices.
What skills are required for a data mining professional?
A data mining professional typically requires expertise in programming languages such as Python or R, statistical analysis, data visualization, machine learning techniques, and domain knowledge in the respective field of application.