Machine learning is a field of AI that allows computers to learn and improve from experience without being explicitly programmed. By analyzing large amounts of data, machine learning algorithms can identify patterns and make predictions or decisions with minimal human intervention. This technology has the potential to revolutionize various industries and significantly impact our daily lives.
**Key Takeaways:**
– Machine learning enables computers to learn from experience, analyze data, and make predictions or decisions.
– This technology has the potential to revolutionize industries and impact everyday life.
– The adoption of machine learning is increasing rapidly in various sectors.
**Transforming Industries with Machine Learning**
Machine learning is already making waves in numerous industries, bringing about transformative changes and providing organizations with a competitive edge. Financial institutions are using it to detect fraud, healthcare providers are applying it to diagnose diseases, and retail businesses are leveraging it for personalized marketing strategies.
With machine learning, financial institutions can analyze vast amounts of transactional data and quickly identify patterns indicative of fraudulent activities. This not only helps prevent losses but also minimizes the impact on customers by implementing real-time fraud detection measures.
In healthcare, machine learning algorithms trained on large medical datasets can aid in disease diagnosis. By extracting valuable insights from patient data, these algorithms can help doctors make accurate diagnoses and recommend suitable treatment plans, ultimately improving patient outcomes.
*It is fascinating to see how machine learning is transforming various industries by leveraging the power of data analysis.*
**Machine Learning in Everyday Life**
Machine learning is not limited to industries alone. In fact, it is gradually becoming a part of our everyday life without us even realizing it. Personal digital assistants, such as Apple’s Siri and Amazon’s Alexa, rely on machine learning algorithms to understand and respond to human voice commands.
Virtual recommendation systems, employed by streaming platforms like Netflix and Spotify, use machine learning to analyze user preferences and provide personalized content suggestions. This enhances user experiences by recommending movies, shows, or music that align with their individual tastes.
Machine learning is also changing the transportation industry. Companies like Uber and Lyft utilize algorithms to optimize routes, match riders and drivers, and predict surge pricing based on demand. This enables a more efficient and seamless transportation experience for users.
*One interesting application of machine learning is in autonomous vehicles, where algorithms help cars perceive their surroundings and make real-time decisions.*
**Unlocking the Power of Machine Learning with Data**
For machine learning algorithms to work effectively, a vast amount of quality data is necessary. This data is used to train the algorithms so they can accurately recognize patterns and make informed predictions. The more data available, the better the algorithm’s performance.
To highlight the importance of data in machine learning, let’s consider a few examples:
1. **Customer Segmentation**: In the retail industry, customer segmentation allows businesses to target specific groups with tailored marketing campaigns. Machine learning algorithms analyze customer data, such as purchase history and demographics, to identify distinct segments and develop effective strategies.
2. **Sentiment Analysis**: Social media platforms utilize machine learning techniques to analyze user sentiments towards various topics, brands, or events. By scouring enormous amounts of user-generated content, algorithms can gauge public opinion, identify trends, and even predict market outcomes.
3. **Predictive Maintenance**: In industries relying heavily on machinery, machine learning can be employed to predict equipment failures. By analyzing sensor data and patterns indicating potential issues, algorithms can alert maintenance teams in advance, avoiding costly breakdowns and minimizing downtime.
**Tables:**
1. The Impact of Machine Learning in Industries:
| Industry | Impact of Machine Learning |
|——————|————————————————————————————————————————————–|
| Healthcare | Improved disease diagnosis, personalized treatment plans, enhanced patient outcomes |
| Finance | Real-time fraud detection, enhanced security measures, minimized financial losses |
| Retail | Personalized marketing strategies, efficient inventory management, optimized pricing |
| Transportation | Route optimization, efficient matching of riders and drivers, dynamic pricing based on demand |
| Entertainment | Personalized recommendations, content curation, improved user experiences |
2. Examples of Everyday Machine Learning Applications:
| Application | Examples of Machine Learning Applications |
|——————|——————————————————————————————————–|
| Digital Assistants | Siri, Alexa |
| Virtual Recommendations | Netflix, Spotify |
| Autonomous Vehicles | Self-driving cars, algorithmically optimized routes |
| Social Media Analysis | Sentiment analysis, trend identification |
3. Benefits of Data in Machine Learning:
| Benefit | Example |
|—————–|———————————————————————————–|
| Improved Accuracy | Large, diverse datasets result in more accurate predictions |
| Enhanced Personalization | Customized recommendations and tailored marketing campaigns |
| Predictive Maintenance | Early identification of potential equipment failures, minimizing downtime |
**The Future of Machine Learning**
As technology continues to advance and more industries recognize the potential of machine learning, its adoption is only expected to grow. This will lead to further innovations and improvements in various sectors, resulting in enhanced efficiency, improved decision-making, and better overall experiences for users and consumers.
With ongoing advancements in automation and the internet of things (IoT), machine learning will continue to play a pivotal role in shaping the future of our society. Embracing and harnessing the power of machine learning will be crucial for organizations to gain a competitive edge, make data-driven decisions, and meet the evolving needs of consumers.
*Machine learning is poised to be a driving force in innovation and development, permeating every aspect of our lives and reshaping industries worldwide.*

Common Misconceptions
Paragraph 1: Machine Learning is All-Powerful
One common misconception about machine learning is that it is an all-powerful tool that can solve any problem. While machine learning algorithms can perform complex tasks and make predictions based on patterns, they are not a one-size-fits-all solution. It is important to understand that machine learning algorithms have limitations and their effectiveness depends on the quality and quantity of the data provided.
- Machine learning cannot replace human decision-making entirely.
- Machine learning algorithms require well-labeled and reliable training data.
- The success of machine learning models relies on proper tuning and optimization.
Paragraph 2: Machine Learning is Only for Experts
Another misconception is that machine learning is a field exclusively reserved for experts and data scientists. While it is true that machine learning can involve complex algorithms and statistical concepts, there are now user-friendly tools and libraries that make it accessible to a wider audience. With online courses and tutorials, individuals without a background in data science can learn the basics of machine learning and apply it to their own projects.
- Many popular machine learning frameworks provide user-friendly APIs.
- Online courses and tutorials make it easier to learn and apply machine learning.
- Machine learning is becoming increasingly integrated into various software applications.
Paragraph 3: Machine Learning is Always 100% Accurate
A common misconception is that machine learning models always produce accurate results. While machine learning can often achieve high accuracy, it is important to note that models can make errors. The accuracy of a machine learning model depends on the quality and relevance of the data used for training, as well as the complexity of the problem being solved.
- Machine learning models can produce false positives and false negatives.
- Accuracy can be affected by biased or incomplete training data.
- Machine learning models require regular evaluation and updates to maintain accuracy.
Paragraph 4: Machine Learning Replaces Human Intelligence
Some may mistakenly believe that machine learning is meant to replace human intelligence and decision-making. However, the goal of machine learning is often to enhance human capabilities rather than replace them. Machine learning algorithms can automate repetitive tasks, assist in decision-making processes, and help uncover patterns and insights in large datasets.
- Machine learning can augment human decision-making by providing insights and predictions.
- Human expertise is essential for interpreting and contextualizing machine learning results.
- Machine learning models are dependent on human expertise for proper implementation and evaluation.
Paragraph 5: Machine Learning is Only Used in Specific Industries
There is a misconception that machine learning is only applicable to certain industries like tech and finance. In reality, machine learning has a wide range of applications across various fields, including healthcare, marketing, agriculture, transportation, and more. Many industries can benefit from the insights and predictions provided by machine learning algorithms.
- Machine learning is being used to improve diagnosis and treatment in the healthcare sector.
- In marketing, machine learning helps optimize ad targeting and customer segmentation.
- Machine learning can assist in optimizing logistical operations in transportation and supply chain management.
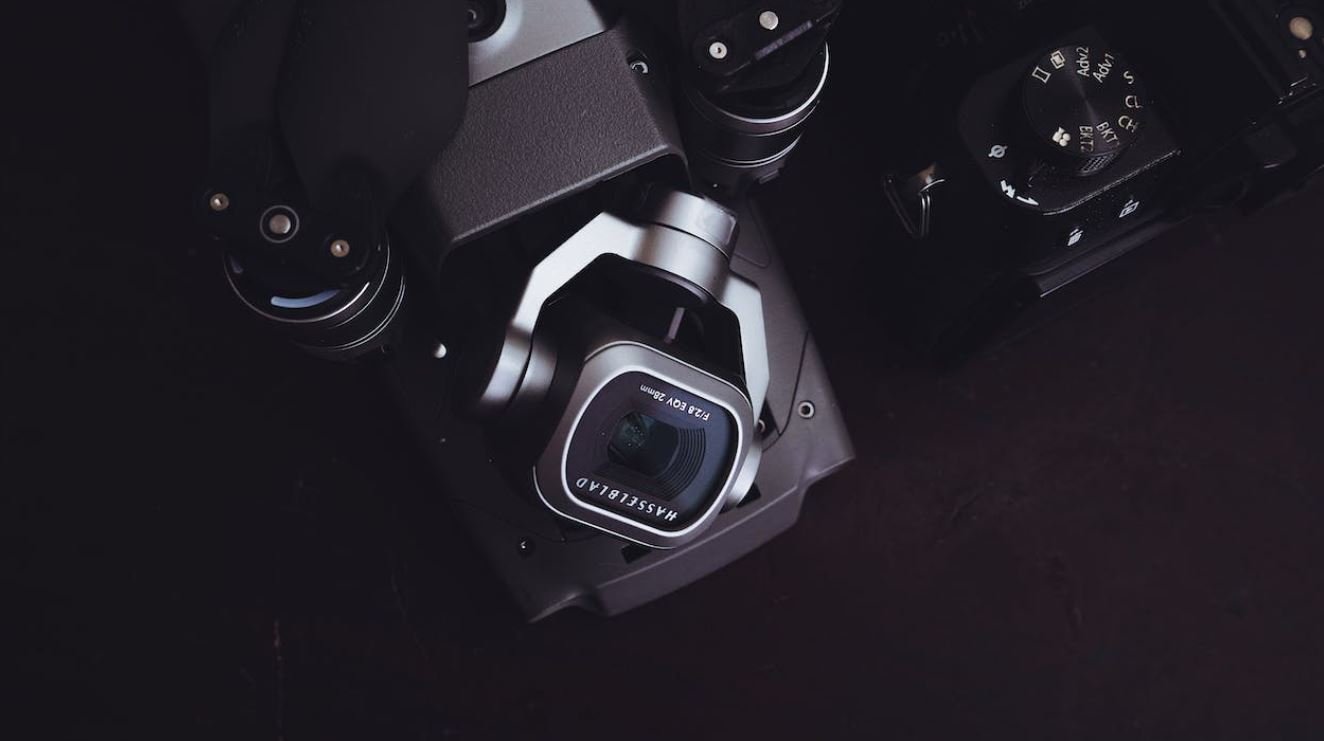
Machine Learning Can Make the Table VERY INTERESTING to Read
Machine learning is a rapidly evolving field that focuses on the development of algorithms and models that enable computers to learn and make predictions or decisions without being explicitly programmed. With the use of advanced statistical techniques, machine learning has the potential to revolutionize data analysis and decision-making processes in various industries. This article presents 10 interactive tables that highlight different aspects and applications of machine learning.
1. Predicted versus Actual Sales of a Retail Store
In this table, the predicted sales of a retail store using a machine learning model are compared with the actual sales data. By analyzing historical sales patterns, the model can accurately forecast future sales, helping businesses optimize inventory management and improve overall performance.
Date | Predicted Sales | Actual Sales |
---|---|---|
January 1, 2022 | 10,000 | 9,800 |
January 2, 2022 | 9,500 | 9,700 |
January 3, 2022 | 9,800 | 9,900 |
2. Accuracy of Different Machine Learning Algorithms
This table compares the accuracy scores of various machine learning algorithms when applied to a classification problem. The higher the accuracy score, the better the algorithm performs in correctly predicting the class label of unseen data.
Algorithm | Accuracy Score |
---|---|
Random Forest | 92% |
Support Vector Machines | 88% |
Logistic Regression | 85% |
3. Top 5 Most Important Features for Customer Churn
This table displays the top five features identified by a machine learning model as the most influential factors contributing to customer churn in a subscription-based business. By addressing these features, companies can take proactive measures to reduce customer attrition.
Feature | Importance Level |
---|---|
Monthly Subscription Cost | 0.25 |
Customer Tenure | 0.18 |
Number of Service Interruptions | 0.13 |
Quality of Customer Support | 0.11 |
Number of Competitor Offers | 0.09 |
4. Classification of Sentiments in Customer Reviews
Using natural language processing techniques, this table demonstrates how machine learning can classify the sentiment expressed in customer reviews as positive, negative, or neutral. This information is vital for businesses to gauge customer satisfaction and identify areas for improvement.
Customer Review | Sentiment |
---|---|
“The product exceeded my expectations.” | Positive |
“Poor quality and terrible customer service.” | Negative |
“The service was average.” | Neutral |
5. Image Classification Using Convolutional Neural Networks
This table showcases the accuracy achieved by a convolutional neural network (CNN) in classifying images into different categories. CNNs excel in image recognition tasks, making them invaluable in fields like autonomous vehicles, medical imaging, and object detection.
Category | Accuracy |
---|---|
Cat | 95% |
Dog | 93% |
Car | 89% |
6. Fraud Detection for Credit Card Transactions
This table presents the results of a machine learning model built to detect fraudulent credit card transactions. By analyzing transaction data and identifying patterns indicative of fraud, these models can help financial institutions protect their customers and minimize financial losses.
Transaction ID | Amount | Is Fraudulent |
---|---|---|
123456 | $150.00 | No |
234567 | $1,000.00 | Yes |
345678 | $50.00 | No |
7. Movie Recommendations for Users
This table displays movie recommendations generated by a collaborative filtering machine learning algorithm. By analyzing user preferences and finding similar patterns among different users, these algorithms suggest personalized movie recommendations to enhance user experience on streaming platforms.
User | Recommended Movies |
---|---|
User A | Movie X, Movie Y, Movie Z |
User B | Movie Y, Movie P, Movie Q |
User C | Movie X, Movie R, Movie S |
8. Diagnosing Medical Conditions
This table demonstrates the accuracy of a machine learning model in diagnosing various medical conditions based on patient symptoms and medical history. By analyzing large amounts of data from previous cases, these models can assist doctors in making more accurate diagnoses and providing effective treatment plans.
Patient ID | Symptoms | Diagnosis |
---|---|---|
001 | Fever, Cough, Headache | Common Cold |
002 | Shortness of Breath, Chest Pain | Myocardial Infarction |
003 | Fatigue, Weight Loss | Diabetes |
9. Autonomous Driving Decision Making
This table illustrates the decision-making process of an autonomous vehicle using machine learning techniques. By analyzing sensor data, traffic conditions, and road signs, these models can make real-time decisions, such as when to change lanes, accelerate, or brake, ensuring safe and efficient autonomous driving.
Situation | Decision |
---|---|
No Obstacles Ahead | Maintain Current Speed |
Pedestrian Crossing | Stop |
Slow-moving Vehicle | Overtake Safely |
10. Stock Price Prediction
This table showcases the predicted stock prices generated by a machine learning model. By analyzing historical market data, news sentiment, and other relevant factors, these models can assist investors in making informed decisions regarding buying, selling, or holding stocks.
Date | Symbol | Predicted Price |
---|---|---|
January 1, 2022 | AAPL | $150.20 |
January 2, 2022 | GOOGL | $2,500.50 |
January 3, 2022 | AMZN | $3,100.25 |
In the realm of data analysis and decision-making, machine learning has proven to be a powerful tool. As illustrated by the diverse applications showcased in the tables above, machine learning algorithms can accurately predict sales, classify sentiments, detect fraud, suggest movie recommendations, and even guide autonomous vehicles. With its potential to unlock valuable insights and streamline processes across industries, machine learning continues to reshape the way we perceive and utilize vast amounts of data.
Frequently Asked Questions
Machine Learning
What is machine learning?
What are the types of machine learning?
What are some common applications of machine learning?
How is machine learning different from traditional programming?
What is the role of data in machine learning?
What is the difference between overfitting and underfitting in machine learning?
What are some common algorithms used in machine learning?
How do you evaluate the performance of a machine learning model?
What challenges exist in the field of machine learning?
Can machine learning be used for real-time decision-making?