Data Mining Business
Data mining has become essential for businesses looking to leverage their vast amounts of data to gain valuable insights and make informed decisions. It is a process of discovering patterns and extracting useful information from large datasets, utilizing statistical and mathematical techniques. In this article, we will explore the benefits of data mining for businesses and how it can help drive success.
Key Takeaways
- Data mining helps businesses discover patterns and extract valuable insights from large datasets.
- Businesses can use data mining to improve decision-making, increase efficiency, and identify new opportunities.
- Data mining techniques include classification, regression, clustering, and association.
- Proper data preparation and understanding the context are key for successful data mining.
- With the advancements in technology, data mining is becoming more accessible and efficient for businesses of all sizes.
The Benefits of Data Mining in Business
Data mining provides businesses with a competitive edge by enabling them to uncover hidden patterns and insights within their data. *It helps businesses identify trends and make data-driven decisions.* By analyzing large datasets, businesses can gain a deeper understanding of customer behavior, preferences, and market trends, allowing them to tailor their products and services accordingly.
Moreover, data mining helps businesses increase operational efficiency by identifying inefficiencies and bottlenecks in their processes. By analyzing data from various sources, businesses can optimize workflows and streamline operations, leading to cost savings and improved productivity. *For example, a retail company can use data mining to identify the most profitable products and optimize their inventory management.*
Data Mining Techniques
There are various techniques employed in data mining, each serving a different purpose. These techniques include:
- Classification: This technique categorizes data into pre-defined classes based on their attributes. It helps businesses predict categorical variables, such as customer churn or fraud detection.
- Regression: Regression analysis examines the relationships between variables to predict numeric values. It is useful for forecasting sales, demand, or any other continuous variable.
- Clustering: Clustering groups similar data points together based on their characteristics. It is beneficial for market segmentation and identifying customer groups with similar traits.
- Association: Association analysis discovers relationships between variables and helps businesses uncover hidden patterns within their data. It is commonly used in recommendation systems and market basket analysis.
Data Mining in Action: Real-World Examples
Business | Data Mining Application |
---|---|
Online Retailer | Using clustering techniques to segment customers based on purchasing behavior, demographics, and browsing patterns. This allows the retailer to personalize marketing campaigns and improve customer satisfaction. |
Business | Data Mining Application |
---|---|
Insurance Company | Applying classification algorithms to identify potential fraudulent claims by analyzing historical claim data, patterns, and suspicious activities, enabling the company to reduce fraudulent payouts. |
Business | Data Mining Application |
---|---|
Manufacturing Company | Using regression analysis to forecast demand for different products based on historical sales data, market trends, and other external factors. This helps optimize production planning and inventory management. |
The Future of Data Mining in Business
Data mining plays a crucial role in the success of modern businesses, and its utilization is expected to grow in future years. With advancements in machine learning and artificial intelligence, businesses can now analyze data more efficiently and extract deeper insights. As technology continues to evolve, data mining will become even more accessible for businesses of all sizes, enabling them to make data-driven decisions and stay competitive in their respective industries.
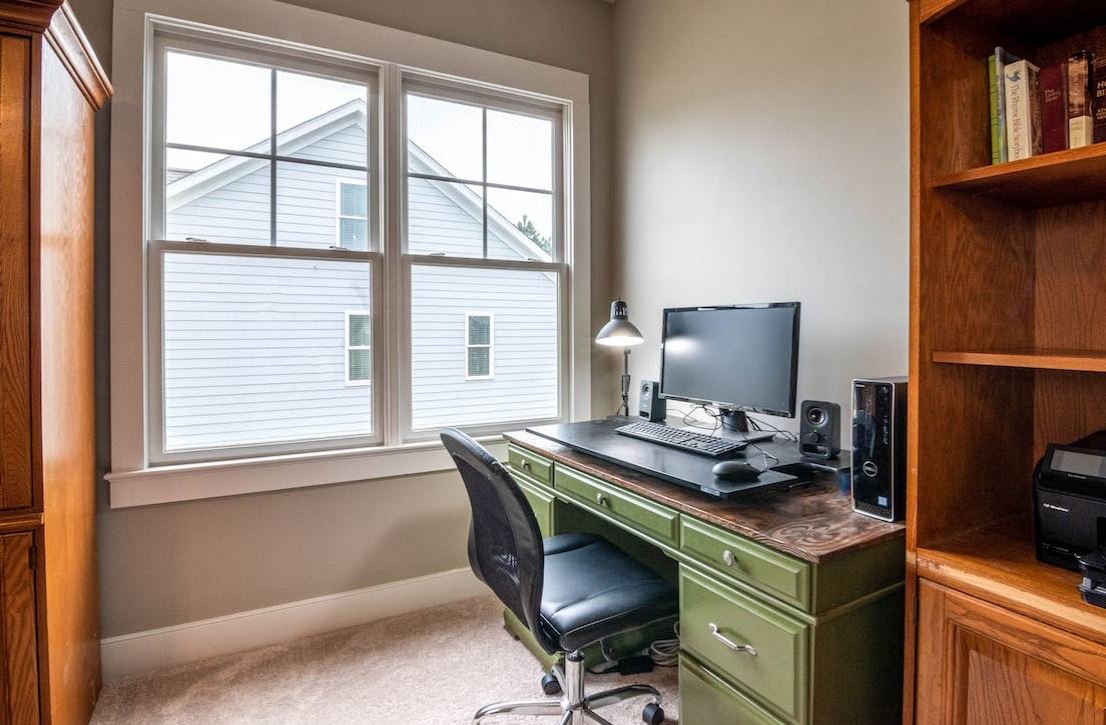
Data Mining Business
Common Misconceptions
There are several common misconceptions regarding data mining in the business world that can often lead to confusion and misinformation. By addressing these misconceptions, we can gain a better understanding of the true benefits and limitations of data mining.
- Data mining always results in immediate and accurate predictions.
- Data mining is only useful for large corporations.
- Data mining threatens privacy and security.
One common misconception is the belief that data mining always results in immediate and accurate predictions. While data mining techniques can certainly provide insights and patterns, it is important to understand that predictions are not always 100% accurate. There can be numerous variables and factors that influence the accuracy of predictions, and data mining should be viewed as a tool to assist decision-making rather than a fortune-telling mechanism.
- Data mining requires a significant investment in technology and resources.
- Data mining can replace human decision-making entirely.
- Data mining can only be used for sales and marketing purposes.
Another misconception is that data mining is only useful for large corporations with extensive resources. However, data mining techniques can be applied to businesses of all sizes. With advancements in technology and the availability of user-friendly data mining tools, smaller businesses can also leverage the power of data mining to gain insights, optimize operations, and make informed decisions.
- Data mining is only about collecting customer data.
- Data mining violates ethical standards.
- Data mining can provide absolute certainty in decision-making.
Some individuals believe that data mining primarily focuses on collecting customer data for sales and marketing purposes. While customer data is indeed one aspect of data mining, it involves much more than that. Data mining encompasses various techniques to analyze and discover patterns in different types of data, including financial data, operational data, and supply chain data, among others.
- Data mining can predict everything about the future.
- Data mining is a one-time process.
- Data mining always leads to actionable insights.
Lastly, a misconception that should be addressed is the idea that data mining can provide absolute certainty in decision-making. In reality, data mining provides insights based on patterns and historical data, but it does not guarantee accuracy in predicting the future. Additionally, data mining is not a one-time process; it requires continuous analysis and adaptation as business dynamics and data evolve.
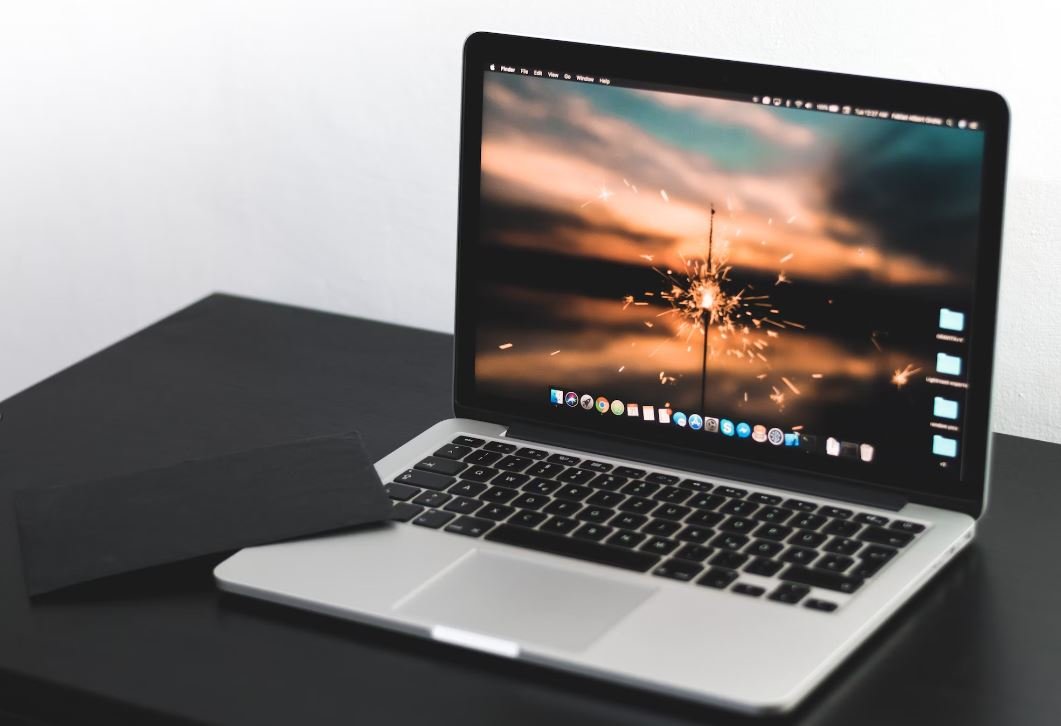
Data Breaches in the Last Decade
With the increasing reliance on technology, data breaches have become a significant concern for businesses and consumers alike. This table highlights some of the largest and most notable data breaches that have occurred in the past decade.
Year | Company | Type of Breach | Number of Records Compromised |
---|---|---|---|
2013 | Target | Payment Card Information Theft | 110 million |
2014 | eBay | Personal Information Theft | 145 million |
2015 | Anthem Inc. | Medical Records Theft | 78.8 million |
2016 | Yahoo | Email Account Information Theft | 500 million |
2017 | Equifax | Personal Information Theft | 147.9 million |
2018 | Marriott International | Booking Data Theft | 500 million |
2019 | Capital One | Financial Records Theft | 106 million |
2020 | Zoom | Video Meeting Privacy Breach | 500,000+ |
2021 | Personal Data Scraping | 533 million | |
2021 | SolarWinds | Supply Chain Attack | 18,000+ |
Data Mining Techniques for Improving Sales
In today’s competitive business landscape, data mining techniques can provide valuable insights for improving sales strategies. This table showcases some effective data mining techniques and their impact on sales growth.
Data Mining Technique | Description | Examples | Sales Impact |
---|---|---|---|
Market Basket Analysis | Identifies associations between products based on consumer purchasing behavior. | If a customer buys a loaf of bread, they are likely to purchase butter as well. | Increased cross-selling opportunities. |
Customer Segmentation | Divides customers into distinct groups based on various attributes and behaviors. | Demographic, psychographic, or purchase history segmentation. | More targeted marketing efforts and personalized recommendations. |
Regression Analysis | Examines the relationship between dependent and independent variables to predict sales. | Analyzing the impact of price, promotion, and competition on sales. | Optimized pricing and promotional strategies. |
Sentiment Analysis | Extracts opinions and emotions from text data to understand customer sentiment. | Analyzing product reviews or social media mentions. | Improved customer satisfaction and targeted product development. |
Churn Prediction | Forecasts customer churn or attrition based on historical data and customer behavior. | Identifying customers at risk of leaving based on their engagement patterns. | Improved customer retention strategies. |
Data Privacy Regulations Worldwide
As digital interactions increase, the need for data privacy regulations has become paramount. This table provides an overview of data privacy regulations implemented across different countries.
Country | Data Privacy Regulation | Year Enacted | Main Provisions |
---|---|---|---|
United States | California Consumer Privacy Act (CCPA) | 2018 | Right to know, delete, and opt-out of personal information collection. |
European Union | General Data Protection Regulation (GDPR) | 2018 | Consent-based data processing, data breach notification, and data portability. |
Canada | Personal Information Protection and Electronic Documents Act (PIPEDA) | 2000 | Consent-based data collection, use, and disclosure. |
Australia | Privacy Act | 1988 | Data breach notification, consent requirements, and privacy principles. |
India | Personal Data Protection (PDP) Bill | 2021 (proposed) | Data localization, consent management, and enhanced individual rights. |
Data Mining Tools Comparison
Data mining tools play a crucial role in analyzing vast amounts of data. This table compares and contrasts popular data mining tools based on various features and capabilities.
Data Mining Tool | License | Machine Learning algorithms | Visualization | Scalability |
---|---|---|---|---|
RapidMiner | Open Source/Commercial | Yes | Yes | High |
IBM SPSS Modeler | Commercial | Yes | Yes | High |
Weka | Open Source | Yes | Yes | Low-Medium |
KNIME | Open Source | Yes | Yes | High |
SAS Enterprise Miner | Commercial | Yes | Yes | High |
Data Mining Workflow Process
Data mining follows a systematic process to extract valuable information from large datasets. This table breaks down the stages of the data mining workflow and their key objectives.
Stage | Description | Key Objectives |
---|---|---|
Data Gathering | Collecting relevant data from multiple sources, such as databases or APIs. | Gather comprehensive and accurate data for analysis. |
Data Preprocessing | Cleaning, transforming, and reducing the dataset to improve analysis quality. | Remove noise, handle missing values, and normalize data. |
Data Exploration | Discovering patterns, relationships, and outliers in the dataset. | Identify meaningful insights and understand the data’s characteristics. |
Model Building | Constructing machine learning models to make predictions or classifications. | Create accurate models to solve specific business problems. |
Evaluation | Assessing the performance and validity of the models. | Measure the effectiveness of the models in predicting or classifying. |
Data Mining Challenges in Healthcare
Data mining in the healthcare industry presents unique challenges due to sensitive patient information. This table highlights key challenges and their impact on healthcare data mining.
Challenge | Description | Impact |
---|---|---|
Data Privacy | Protecting patient privacy and complying with data protection regulations. | Ensuring patient confidentiality without compromising analysis quality. |
Data Quality | Ensuring accuracy, completeness, and reliability of healthcare data. | Improved decision-making and prevention of errors or misdiagnosis. |
Data Integration | Integrating data from various sources and systems in a structured way. | Enable a holistic view for comprehensive analysis and insights. |
Data Sharing | Promoting secure and ethical data sharing between healthcare providers. | Facilitate collaboration and leverage shared knowledge for better outcomes. |
Data Interoperability | Ensuring compatibility and seamless exchange of data across different systems. | Efficient integration and secure data flow between healthcare applications. |
Data Mining Applications in Finance
Data mining has revolutionized the finance industry, enabling better risk assessment and fraud detection. This table demonstrates the diverse applications of data mining in finance.
Application | Description |
---|---|
Credit Scoring | Analyzing credit history and financial data to determine creditworthiness. |
Market Analysis | Identifying patterns and trends in financial markets for investment strategies. |
Fraud Detection | Identifying suspicious transactions and patterns indicative of fraud. |
Risk Management | Assessing and mitigating financial risks through predictive models. |
Algorithmic Trading | Automating trade execution based on predefined mathematical models. |
Data Mining Techniques for Customer Relationship Management
Data mining techniques have transformed customer relationship management strategies. This table explores various techniques and their impact on CRM.
Data Mining Technique | Description | CRM Impact |
---|---|---|
Customer Segmentation | Dividing customers into distinct groups based on behavior and characteristics. | Personalized marketing campaigns and tailored customer experiences. |
Churn Prediction | Forecasting which customers are likely to churn or discontinue their relationship. | Proactive retention strategies and targeted offers to prevent churn. |
Recommendation Engines | Suggesting products or services based on customer preferences and behavior. | Increased cross-selling and upselling opportunities. |
Sentiment Analysis | Extracting customer sentiment from text data to gauge satisfaction or dissatisfaction. | Improved customer service and timely issue resolution. |
Customer Lifetime Value | Predicting the future value of a customer throughout their relationship with the company. | Focusing on high-value customers and optimizing marketing efforts. |
Data Mining in Social Media Analysis
Social media platforms generate vast amounts of data that can be leveraged for valuable insights. This table showcases the applications of data mining in social media analysis.
Application | Description |
---|---|
Sentiment Analysis | Identifying the sentiment expressed in social media posts or comments. |
Influencer Identification | Identifying influential individuals who can impact the opinions and behaviors of others. |
Trend Analysis | Tracking and analyzing popular topics or trends in social media conversations. |
Social Network Analysis | Analyzing the relationships between individuals and their network structures. |
Customer Insights | Gaining insights into customer preferences, interests, and demographics. |
Conclusion
Data mining is a powerful tool for businesses across various sectors, enabling them to uncover valuable insights and make data-driven decisions. From analyzing data breaches to improving sales strategies, this article has illustrated the diverse applications of data mining in the business world. While challenges exist, such as data privacy and integration, the potential benefits of data mining are immense. By harnessing the power of data mining techniques and tools, organizations can gain a competitive edge and drive innovation in today’s data-driven landscape.
Frequently Asked Questions
What is data mining?
Data mining is the process of extracting patterns and valuable insights from large datasets. It involves using various statistical and machine learning techniques to analyze and interpret data, helping businesses make informed decisions and uncover hidden trends.
How is data mining beneficial for businesses?
Data mining can provide several benefits for businesses, such as identifying customer behavior patterns, improving marketing strategies, detecting fraud and anomalies, optimizing operations, and predicting future trends or outcomes. It enables businesses to make data-driven decisions and gain a competitive edge in the market.
What types of data can be mined?
Data mining can be performed on various types of data, including structured data (e.g., databases, spreadsheets), unstructured data (e.g., text documents, emails), semi-structured data (e.g., XML files), and even multimedia data (e.g., images, videos). The type of data and its quality greatly influence the effectiveness of data mining techniques.
What are some commonly used data mining techniques?
There are several commonly used data mining techniques, including classification, clustering, regression, association rule mining, anomaly detection, and text mining. Each technique has its own purpose and can be applied to different types of data mining tasks.
What are the ethical considerations in data mining?
When performing data mining, businesses need to consider ethical considerations such as privacy, consent, and data protection. It is important to ensure that sensitive and personal information is handled appropriately, and that the data mining process adheres to legal and regulatory requirements.
What tools or software can be used for data mining?
There are various tools and software available for data mining, such as Python libraries (e.g., Scikit-learn, TensorFlow), R programming language, RapidMiner, Weka, and KNIME. These tools provide functionalities for data preprocessing, model building, visualization, and evaluation.
How can businesses prepare data for data mining?
In order to prepare data for data mining, businesses need to perform data preprocessing tasks such as data cleaning, data integration, data transformation, and data reduction. This involves removing noise and redundant information, handling missing values, and transforming the data into a suitable format for analysis.
Are there any challenges in data mining?
Data mining faces several challenges, including dealing with high-dimensional and complex data, selecting appropriate data mining techniques, handling large datasets, ensuring data quality, and interpreting the results of data mining models accurately. Domain knowledge and expertise in statistical analysis are crucial to overcome these challenges.
How can businesses evaluate the performance of data mining models?
Businesses can evaluate the performance of data mining models using various evaluation metrics such as accuracy, precision, recall, F-measure, and ROC curves. Splitting the data into training and testing sets, cross-validation, and using validation techniques like holdout or k-fold validation can help assess the performance of the models.
How can data mining be applied in different industries?
Data mining can be applied in various industries such as finance, healthcare, retail, telecommunications, manufacturing, and transportation. In finance, it can be used for credit scoring and fraud detection, while in healthcare, it can help in disease prediction and treatment planning. The versatile nature of data mining makes it applicable across multiple sectors.