Data mining is a powerful tool used by businesses and organizations to extract valuable insights and patterns from large datasets. In today’s digital age, where massive amounts of data are generated every day, data mining plays a crucial role in making informed decisions and gaining a competitive edge. This article will explore the importance of data mining and how it can benefit various industries.
**Key Takeaways:**
1. Data mining is the process of discovering patterns and insights from large datasets.
2. It helps businesses make data-driven decisions and gain a competitive advantage.
3. Data mining is widely used across industries, including finance, healthcare, marketing, and more.
Data mining leverages a combination of statistical algorithms, machine learning techniques, and artificial intelligence to analyze vast amounts of data. By uncovering hidden patterns and relationships, organizations can make better predictions, optimize processes, and identify areas for improvement.
*One interesting use of data mining is in predictive analytics, where historical data is analyzed to forecast future outcomes.*
Data mining provides numerous benefits across various industries. Let’s explore some key applications:
**Applications of Data Mining:**
1. Financial Services:
– Fraud detection and prevention.
– Credit scoring and risk assessment.
– Market trend analysis.
2. Healthcare:
– Disease diagnosis and treatment recommendations.
– Patient monitoring and predictive analytics.
– Drug discovery and clinical research.
3. Marketing:
– Customer segmentation and targeting.
– Product recommendation engines.
– Churn analysis and customer retention strategies.
Table 1: Examples of Data Mining Applications
| Industry | Data Mining Applications |
|———————–|——————————————————|
| Financial Services | Fraud detection, credit scoring, market trend analysis |
| Healthcare | Disease diagnosis, patient monitoring, drug discovery |
| Marketing | Customer segmentation, product recommendation |
With the ability to handle large datasets and uncover hidden insights, data mining helps organizations gain a competitive advantage. The analysis and interpretation of data lead to improved decision-making, increased operational efficiency, and enhanced customer satisfaction.
Table 2: Benefits of Data Mining
| Benefit | Description |
|—————————————|————————————————————————————-|
| Improved decision-making | Data-based insights lead to informed decisions. |
| Increased operational efficiency | Identifying bottlenecks and optimizing processes. |
| Enhanced customer satisfaction | Understanding customer behavior and delivering personalized experiences. |
| Competitive advantage | Gaining insights that others may not have, leading to a competitive edge. |
The process of data mining involves several steps:
1. Data Collection: Gathering relevant data from various sources, such as databases, files, or the internet.
2. Data Preparation: Cleaning and transforming the raw data into a suitable format for analysis.
3. Data Exploration: Exploring the dataset to understand its characteristics and identify potential patterns.
4. Modeling: Applying statistical algorithms and machine learning techniques to discover patterns and relationships.
5. Evaluation: Assessing the effectiveness and accuracy of the models created during the modeling phase.
6. Deployment: Implementing the insights obtained from data mining into real-world applications.
*Exploring diverse datasets through data mining can often uncover unexpected relationships and patterns.*
Table 3: Data Mining Process Steps
| Step | Description |
|—————–|———————————————————————–|
| Data Collection | Gathering relevant data from various sources. |
| Data Preparation| Cleaning and transforming raw data into a usable format. |
| Data Exploration| Exploring the dataset to understand its characteristics and patterns. |
| Modeling | Applying algorithms to discover patterns and relationships. |
| Evaluation | Assessing the accuracy and effectiveness of the models created. |
| Deployment | Implementing the insights obtained from data mining. |
In conclusion, data mining plays a critical role in transforming large datasets into valuable insights. Its applications in finance, healthcare, marketing, and other industries are extensive, helping organizations make informed decisions, improve operations, and gain a competitive advantage. Harnessing the power of data mining allows businesses to thrive in today’s data-rich environment. Whether it’s identifying fraudulent activities, predicting market trends, or understanding customer behavior, data mining is an indispensable tool for unlocking the potential within data.
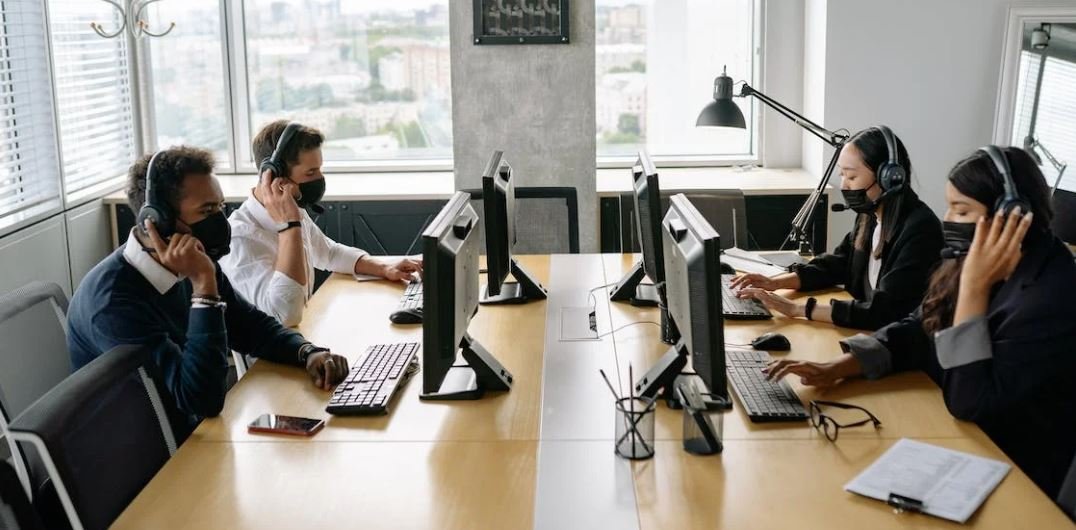
Common Misconceptions
Misconception 1: Data Mining is Similar to Data Collection
One common misconception about data mining is that it is the same as data collection. However, data collection refers to the process of gathering raw data, while data mining is the process of extracting valuable insights and patterns from the collected data.
- Data mining focuses on analyzing collected data.
- Data collection is a necessary step before data mining can occur.
- Data mining requires specific algorithms and techniques.
Misconception 2: Data Mining Violates Privacy
Another misconception is that data mining violates privacy. While it is true that data mining involves analyzing large amounts of data, it is done in a way that respects privacy regulations and standards.
- Data mining anonymizes and aggregates data to protect privacy.
- Privacy laws and ethical guidelines govern data mining practices.
- Data mining can provide valuable insights without compromising individual privacy.
Misconception 3: Data Mining is Reliable and Predictive
Many people mistakenly believe that data mining is always reliable and predictive. However, data mining is a scientific process that involves analyzing large datasets, and the accuracy of its predictions can vary based on numerous factors.
- Data mining outcomes are influenced by the quality and relevance of the dataset.
- Data mining predictions are probabilistic, not absolute.
- Data mining requires regular validation and refinement.
Misconception 4: Data Mining is Only Used by Large Companies
There is a perception that data mining is exclusive to large corporations. In reality, data mining techniques can be employed by businesses of all sizes and across different industries.
- Data mining tools and technologies are accessible to organizations of all scales.
- Data mining can help small businesses make informed decisions.
- Data mining can be applied in various sectors like healthcare, finance, and marketing.
Misconception 5: Data Mining is a One-time Process
Some individuals believe that data mining is a one-time process. However, data mining is an iterative process that involves continuous exploration and analysis of data to uncover new insights and refine existing models.
- Data mining requires regular updates and maintenance.
- Data mining can uncover hidden patterns over time as new data becomes available.
- Data mining helps organizations adapt to changing market trends.
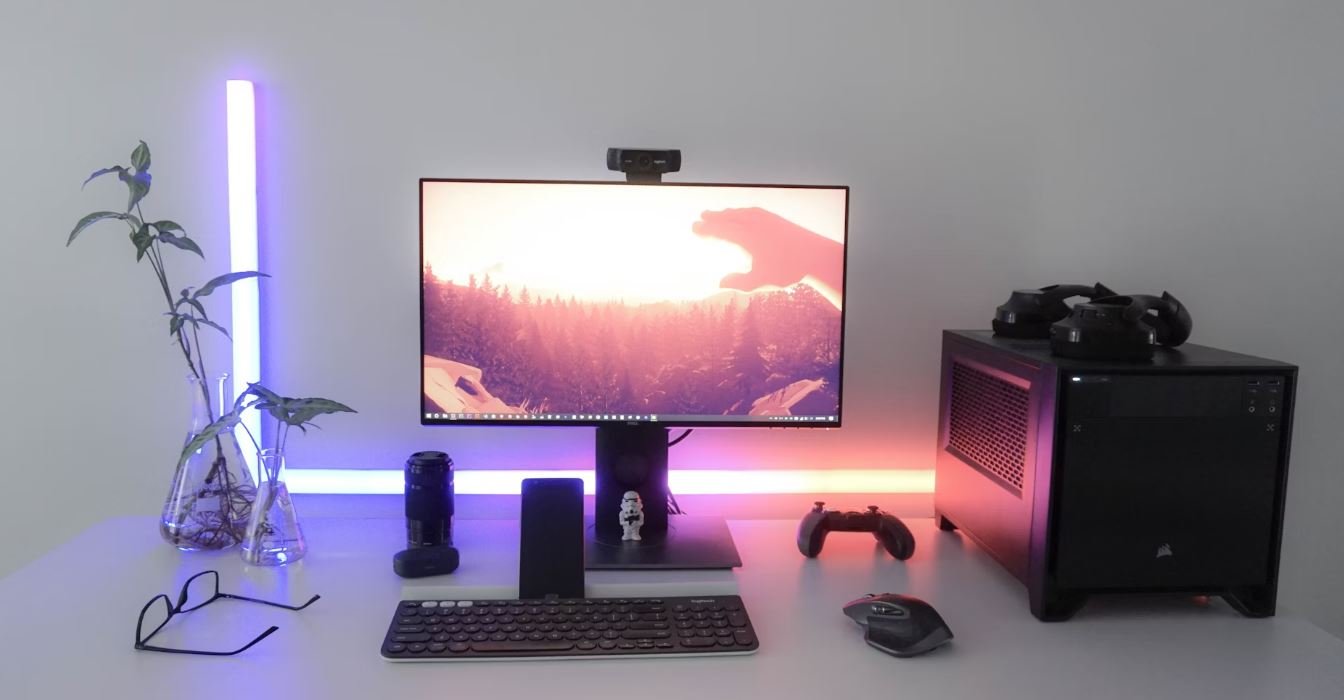
Importance of Data Mining in Business
Data mining refers to the process of discovering patterns, correlations, and insights from large datasets. It plays a crucial role in business decision-making by enabling organizations to extract valuable information that can improve operations, efficiency, and profitability. The following tables provide examples of how data mining impacts various aspects of a business.
Table: Customer Segmentation by Demographics
This table demonstrates how data mining can be used to segment customers based on their demographics. By analyzing data such as age, gender, income, and location, businesses can tailor their marketing strategies to different customer groups, improving customer satisfaction and increasing sales.
Segment | Age | Gender | Income | Location |
---|---|---|---|---|
Segment A | 25-35 | Male | $40,000-$60,000 | Urban |
Segment B | 35-45 | Female | $60,000-$80,000 | Rural |
Segment C | 18-24 | Male | $20,000-$40,000 | Suburban |
Table: Sales by Product Category
This table represents the sales performance of different product categories obtained through data mining. By analyzing these sales trends, businesses can identify which product categories are most popular and allocate resources accordingly to maximize revenue.
Product Category | 2018 Sales | 2019 Sales | 2020 Sales |
---|---|---|---|
Electronics | $500,000 | $600,000 | $700,000 |
Apparel | $200,000 | $250,000 | $300,000 |
Home Decor | $150,000 | $180,000 | $200,000 |
Table: Customer Churn Rate
This table displays the churn rate, representing the percentage of customers who stop using a company’s product or service over a given time period. By examining churn rates through data mining, businesses can identify factors that contribute to customer attrition and take proactive measures to retain customers and improve loyalty.
Year | Churn Rate |
---|---|
2018 | 15% |
2019 | 10% |
2020 | 8% |
Table: Product Recommendation Based on User Behavior
This table illustrates how data mining can be used to provide personalized product recommendations to customers. By analyzing user behavior such as browsing history, purchase patterns, and preferences, businesses can suggest relevant products, enhancing the overall customer experience and increasing sales.
User ID | Recommended Product |
---|---|
12345 | Product A |
56789 | Product B |
98765 | Product C |
Table: Fraud Detection in Financial Transactions
This table demonstrates how data mining is employed for fraud detection in financial transactions. By analyzing patterns and anomalies in transactional data, businesses can identify suspicious activities and prevent fraudulent transactions, safeguarding both customer interests and their own reputation.
Transaction ID | Customer | Amount | Status |
---|---|---|---|
1234 | John Doe | $500 | Approved |
5678 | Jane Smith | $1,000 | Denied |
9012 | Mike Johnson | $1,500 | Pending |
Table: Website Traffic by Source
This table showcases the different sources of website traffic obtained through data mining. By analyzing this data, businesses can determine which marketing channels drive the most traffic to their website and allocate resources effectively to maximize their online presence.
Source | Percentage of Traffic |
---|---|
Organic Search | 40% |
Social Media | 25% |
Referral | 20% |
Table: Employee Performance Ratings
This table presents employee performance ratings obtained through data mining. By analyzing various factors such as productivity, customer satisfaction, and attendance, businesses can assess individual employee performance, reward high performers, and provide necessary support for those in need.
Employee ID | Performance Rating |
---|---|
1001 | Excellent |
1002 | Good |
1003 | Needs Improvement |
Table: Inventory Management
This table represents inventory management data obtained through data mining. By analyzing factors such as demand patterns, lead times, and stock levels, businesses can optimize inventory management processes, reducing costs associated with overstocking or stockouts and ensuring efficient operations.
Product ID | Current Stock | Demand (monthly) | Lead Time (days) |
---|---|---|---|
001 | 100 | 50 | 5 |
002 | 75 | 100 | 10 |
003 | 50 | 30 | 3 |
Table: Social Media Sentiment Analysis
This table demonstrates how data mining can be utilized for sentiment analysis on social media platforms. By analyzing sentiments expressed by users towards a brand or product, businesses can gather valuable insights, understand customer satisfaction levels, and make data-driven decisions to enhance their reputation.
Post ID | Sentiment |
---|---|
1234 | Positive |
5678 | Negative |
9012 | Neutral |
Data mining revolutionizes the way businesses operate in today’s data-driven world. By harnessing the power of data and employing data mining techniques, organizations can gain valuable insights into various aspects of their business, including customer segmentation, sales performance, churn rate, fraud detection, website traffic, employee performance, inventory management, and social media sentiment. These insights empower businesses to make well-informed decisions, optimize processes, and effectively meet customer needs. Integrating data mining into business strategies enables companies to stay competitive and thrive in a rapidly evolving market.
Frequently Asked Questions
Why Data Mining
What is data mining?
Data mining refers to the process of discovering patterns and extracting useful information from large sets of data. It involves using various techniques and algorithms to identify trends, relationships, and anomalies within the data.
Why is data mining important?
Data mining plays a crucial role in various industries as it enables businesses to gain valuable insights and make informed decisions. It helps in identifying patterns, understanding customer behavior, improving marketing strategies, detecting fraud, optimizing operations, and much more.
What are the basic steps in data mining?
The basic steps in data mining typically include data collection, data preprocessing, exploratory data analysis, feature selection or extraction, model building and evaluation, and deployment of the model. These steps may vary depending on the specific data mining techniques and goals of the project.
What are some popular data mining techniques?
Some popular data mining techniques include association rule mining, classification, clustering, regression analysis, neural networks, decision trees, and genetic algorithms. Each technique has its own usage and benefits, depending on the type of data and the desired outcomes.
What are the challenges in data mining?
Data mining faces challenges such as dealing with large and complex datasets, ensuring data quality, handling missing or noisy data, protecting privacy and security, choosing appropriate algorithms, and interpreting the results accurately. Overcoming these challenges requires expertise and careful consideration of various factors.
What are the ethical considerations in data mining?
Ethical considerations in data mining include ensuring privacy protection, obtaining informed consent when collecting and analyzing personal data, avoiding discrimination or bias in decision-making based on the results, and being transparent about the data sources and methodologies used. Adhering to ethical standards is crucial to maintain trust and avoid potential harm.
What are the potential applications of data mining?
Data mining has applications in various fields including marketing, finance, healthcare, telecommunications, e-commerce, social media analysis, fraud detection, customer relationship management, and more. It can be used to improve business processes, optimize resource allocation, enhance customer experiences, and drive innovation.
What skills are needed for data mining?
Data mining requires a combination of technical skills and domain knowledge. Proficiency in programming languages such as Python or R, understanding of statistical concepts, familiarity with data manipulation and visualization techniques, and critical thinking abilities are important in data mining. Additionally, knowledge of the specific industry or field being analyzed is beneficial.
What are the limitations of data mining?
Data mining has limitations such as the potential for false discoveries or overfitting, reliance on quality and availability of data, difficulties in interpreting results, the need for domain expertise, and the time and computational resources required for analysis. It is important to consider these limitations when interpreting the findings of a data mining project.
How is data mining related to machine learning?
Data mining and machine learning are closely related fields. Data mining involves extracting patterns and insights from data, while machine learning focuses on using algorithms and models to enable computers to learn and make predictions or decisions without being explicitly programmed. Machine learning techniques are often utilized within data mining projects to create predictive models and uncover hidden patterns.