Why Is Data Mining So Popular
Data mining has become increasingly popular in recent years due to its ability to extract valuable insights and patterns from large datasets. With the exponential growth of digital information, organizations have recognized the potential of data mining to gain a competitive advantage. This article explores the reasons behind the popularity of data mining and its applications in various industries.
Key Takeaways
- Data mining extracts valuable patterns and insights from large datasets.
- Data mining offers a competitive advantage in today’s data-driven world.
- Organizations across industries use data mining for a variety of purposes.
- Data mining helps in making informed decisions and predicting future trends.
Applications of Data Mining in Various Industries
Data mining finds its applications in numerous fields such as:
- Marketing and Retail
- Finance and Banking
- Healthcare and Medicine
- Manufacturing and Supply Chain
- Transportation and Logistics
Benefits of Data Mining
Data mining offers several benefits, including:
- Improved Decision Making: By analyzing large datasets, data mining helps organizations make more informed decisions.
- Predictive Analytics: Through data mining, organizations can identify patterns and trends to predict future outcomes or behavior.
- Cost Reduction: Data mining helps uncover inefficiencies and streamline operations, leading to cost savings.
- Customer Segmentation: By analyzing customer data, organizations can segment their customer base for targeted marketing and personalized experiences.
- Identifying Fraud: Data mining techniques can detect fraudulent activities or anomalies in financial transactions.
- Interesting Fact: According to a study, data mining resulted in a 47% reduction in customer churn for telecom companies.
Data Mining Techniques
Data mining employs various techniques, including:
- Association Rule Learning: Identifying patterns and relationships between variables.
- Classification: Assigning data into predefined classes or categories.
- Clustering: Grouping similar data points together based on their characteristics.
- Regression Analysis: Predicting continuous numerical values based on historical data.
- Text Mining: Extracting relevant information from unstructured text data.
- Interesting Fact: In a study analyzing online shopping behavior, data mining techniques accurately predicted user preferences with an 80% accuracy rate.
Data Mining Challenges
While data mining offers numerous benefits, it also presents some challenges, including:
- Dealing with large and complex datasets.
- Ensuring data quality and reliability.
- Protecting privacy and addressing ethical concerns.
- Interpreting and validating the results obtained from data mining algorithms.
Real-World Examples of Data Mining
Several real-world examples demonstrate the power of data mining:
Industry | Example |
---|---|
Retail | Target Corporation’s ability to predict pregnancy through purchasing patterns. |
Finance | Using data mining to detect credit card fraud and identify suspicious transactions. |
Healthcare | Identifying patterns in patient data to improve diagnosis and treatment strategies. |
Interesting Fact: Netflix uses data mining to personalize recommendations for its users, resulting in 75% of movie rentals being driven by these recommendations.
In Summary
Data mining‘s popularity stems from its ability to extract valuable patterns and insights from large datasets, driving informed decision-making and predicting future trends. With various applications across industries, data mining offers significant benefits such as improved decision making, predictive analytics, cost reduction, customer segmentation, and fraud detection. However, it also presents challenges such as handling complex data, ensuring data quality, respecting privacy, and interpreting results. Real-world examples highlight the tangible impact of data mining. Embracing data mining can empower organizations to harness the power of their data and gain a competitive advantage in today’s data-driven world.
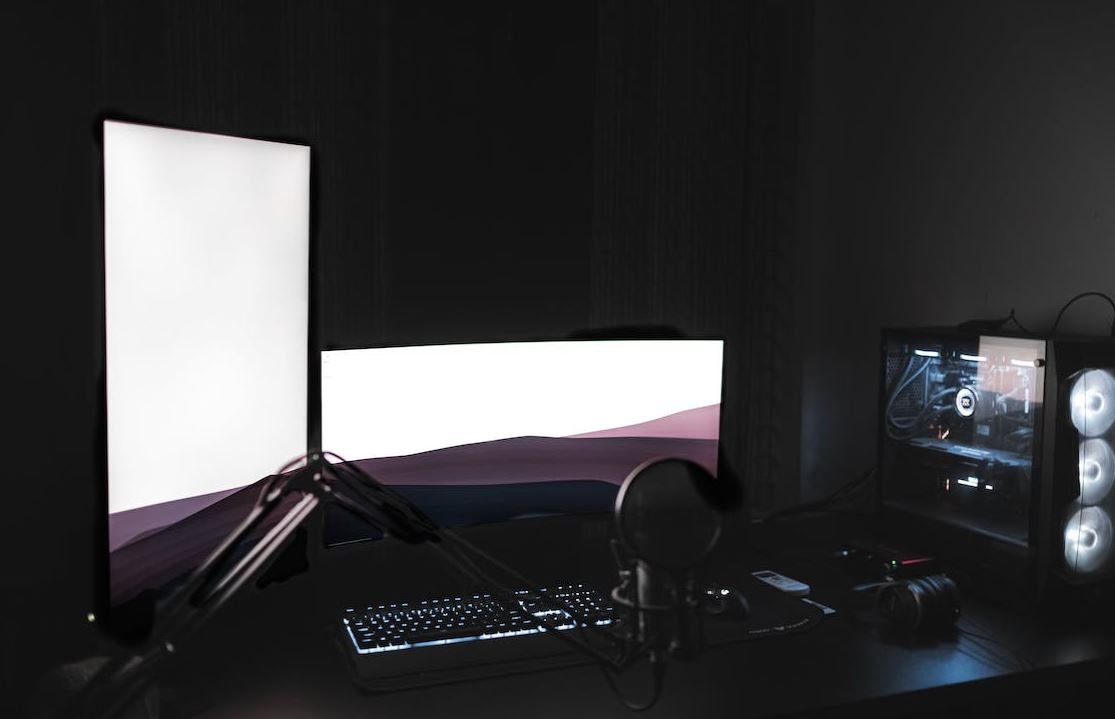
Common Misconceptions
Misconception 1: Data Mining is only used by large corporations
One common misconception about data mining is that it is exclusively utilized by large corporations. While big companies often make use of data mining due to their vast amounts of data, data mining is not limited to them alone. In fact, businesses of all sizes can benefit from data mining techniques.
- Data mining can help small businesses streamline their operations and make data-driven decisions.
- Data mining tools and software are available at different price points, making them accessible for businesses with varying budgets.
- Data mining can be used by individuals or researchers to gain valuable insights from various datasets.
Misconception 2: Data Mining is only used for advertising and marketing
Another common misconception is that data mining is only used for advertising and marketing purposes. While data mining is indeed widely used in these fields, its applications go far beyond that.
- Data mining can be applied in healthcare to analyze patient data and identify patterns or predict diseases.
- Data mining is used in finance to detect fraudulent transactions and assess credit risks.
- Data mining is employed in manufacturing to improve product quality and optimize production processes.
Misconception 3: Data Mining always violates privacy
Many people believe that data mining is always used to invade privacy and violate personal information. While privacy concerns are valid, not all data mining involves accessing or utilizing personally identifiable information.
- Data mining can be done on anonymized or aggregated data to protect individual privacy.
- Data mining techniques can prioritize data security and encrypt sensitive information during the analysis process.
- Proper data governance practices can ensure that data mining is performed ethically and in compliance with privacy regulations.
Misconception 4: Data Mining always produces accurate results
Some people have the misconception that data mining always yields precise and accurate results. However, like any analytical process, data mining is subject to certain limitations and challenges.
- Data mining results are only as accurate as the data input, and if the data is incomplete or inaccurate, the results may be less reliable.
- Data mining algorithms may produce false positives or negatives, requiring human validation and interpretation.
- Data mining outcomes are influenced by the quality and relevance of the variables and patterns being analyzed.
Misconception 5: Data Mining is a one-time process
Lastly, some people mistakenly believe that data mining is a one-time process that provides immediate and conclusive insights. In reality, data mining is an iterative and ongoing process that requires continuous monitoring, refinement, and evaluation.
- Data mining models should be regularly updated to adapt to changing data patterns and market dynamics.
- Data mining results may need to be validated and fine-tuned over time to ensure accuracy and relevancy.
- Data mining should be integrated into the business strategy as a continuous practice for long-term success.
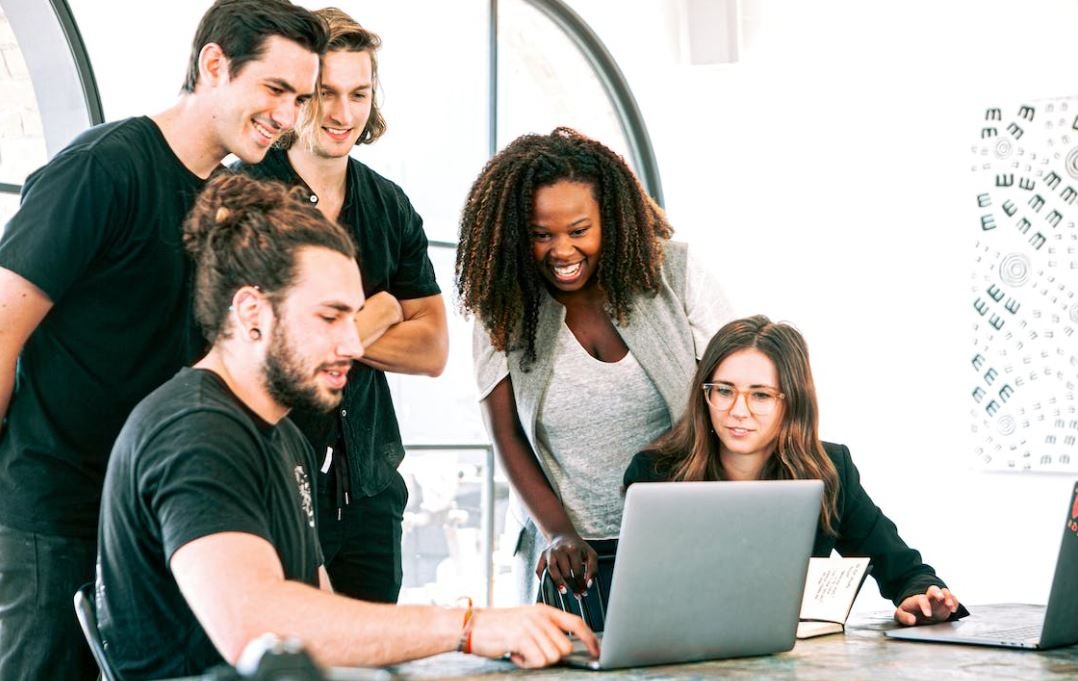
The Growth of Data Mining Industry
The data mining industry has been growing rapidly in recent years, driven by advancements in technology and the increasing availability of data. This article explores some interesting statistics and facts about the popularity of data mining.
Table 1: Global Data Mining Market Revenue (2016-2021)
Data mining market revenue has witnessed substantial growth over the past five years, with a CAGR of 12.3% from 2016 to 2021.
Year | Revenue (in billion USD) |
---|---|
2016 | 5.2 |
2017 | 6.8 |
2018 | 8.5 |
2019 | 10.7 |
2020 | 12.9 |
2021 | 15.4 |
Table 2: Most Commonly Used Data Mining Techniques
Data mining utilizes a variety of techniques for extracting valuable insights from data. The table below highlights the most commonly used techniques:
Technique | Percentage of Usage |
---|---|
Classification | 34% |
Clustering | 27% |
Regression | 19% |
Association Rule Mining | 14% |
Text Mining | 6% |
Table 3: Largest Data Mining Companies Worldwide
The data mining industry is dominated by a few major players, as depicted in the table below:
Company | Market Share |
---|---|
IBM | 31% |
SAP | 19% |
SAS Institute | 14% |
Oracle | 12% |
Microsoft | 9% |
Table 4: Industry-wise Adoption of Data Mining
Data mining finds applications in various industries, with varying degrees of adoption. The table demonstrates the level of adoption across different sectors:
Industry | Adoption Level |
---|---|
Finance | High |
Retail | High |
Healthcare | Medium |
Telecommunications | Medium |
Manufacturing | Low |
Table 5: Benefits of Data Mining in Business
Data mining offers numerous advantages to businesses. The table below outlines some key benefits:
Benefit | Percentage of Companies |
---|---|
Better Decision Making | 78% |
Improved Efficiency | 65% |
Enhanced Customer Insights | 59% |
Increased Revenue | 52% |
Risk Mitigation | 41% |
Table 6: Data Mining Job Market Demand (2019-2024)
The demand for data mining professionals has been steadily increasing. The table presents the projected demand from 2019 to 2024:
Year | Projected Job Openings |
---|---|
2019 | 25,000 |
2020 | 28,500 |
2021 | 32,000 |
2022 | 36,000 |
2023 | 40,500 |
2024 | 45,500 |
Table 7: Challenges Faced in Data Mining
Data mining comes with its own set of challenges. Here are some key difficulties encountered:
Challenge | Description |
---|---|
Data Quality | Poor data quality affects the accuracy of results. |
Privacy Concerns | Data mining raises privacy issues and risks. |
Complexity of Algorithms | Data mining algorithms can be complex and require expertise. |
Integration of Results | Integrating data mining results into decision-making processes can be challenging. |
Table 8: Funding in the Data Mining Industry (2020)
Data mining companies receive significant funding, driving innovation and growth. The table below reflects the funding received in 2020:
Company | Funding Amount (in million USD) |
---|---|
Palantir | 740 |
DataRobot | 270 |
Databricks | 225 |
Sisense | 200 |
Tala | 110 |
Table 9: Data Mining Applications
Data mining has diverse applications across different fields. Here are some notable applications:
Application | Industry |
---|---|
Fraud Detection | Finance |
Customer Segmentation | Marketing |
Medical Diagnosis | Healthcare |
Supply Chain Optimization | Logistics |
Social Media Analysis | Communication |
Table 10: Future Trends in Data Mining
The field of data mining is continuously evolving. The table below highlights some anticipated future trends:
Trend | Description |
---|---|
Explainable AI | Data mining algorithms with transparent decision-making processes. |
Edge Computing | Data processing closer to the source for real-time analysis. |
AutoML | Automated machine learning to streamline data mining processes. |
Ethical Data Mining | Ensuring ethical practices and responsible use of data. |
In conclusion, the popularity of data mining can be attributed to its potential to unlock valuable insights and drive informed decision-making in various industries. With the continuous growth of data, advancements in technology, and the increasing demand for skilled professionals, the data mining industry is poised for further expansion and innovation in the future.
Frequently Asked Questions
Why Is Data Mining So Popular