When Data Mining, Which Statement Is True?
Data mining is the process of analyzing large datasets to discover patterns, correlations, and insights that can help businesses make informed decisions. It involves digging through vast amounts of data to identify trends and extract useful information. However, when it comes to data mining, determining which statement is true can be a complex task. Let’s explore some key factors to consider.
Key Takeaways:
- Data mining involves analyzing large datasets to uncover patterns and insights.
- The truth in data mining depends on the quality and integrity of the data.
- Applying appropriate statistical techniques is crucial for accurate and valid results.
- The interpretation of data mining results requires domain knowledge and expertise.
When **data mining**, it is essential to understand that the truthfulness of the findings heavily relies on the **quality and integrity** of the data being analyzed. Garbage in, garbage out (GIGO) is a common saying in the realm of data analysis. Therefore, it is crucial to ensure that the data collected is accurate, relevant, and representative of the problem or question being studied. Without high-quality data, the accuracy of the insights obtained through data mining could be compromised. *For data mining to be effective, the foundation of reliable data is essential.*
Another important consideration when engaging in data mining is the **application of appropriate statistical techniques**. Depending on the nature of the data and the research question, different statistical methods may need to be employed. *Using the wrong statistical technique can lead to misleading or incorrect conclusions.* One should consult with experts in the field or statisticians to identify the most suitable and valid statistical approach for the specific data mining task at hand.
In the field of data mining, it is essential to keep in mind that **interpreting the results** is just as important as the mining process itself. Data mining can reveal interesting patterns and correlations, but it is crucial to interpret them correctly within the relevant domain. *Misinterpretation of findings can lead to misguided business decisions or incorrect conclusions*. Data mining experts, with deep knowledge and expertise in the particular domain, can provide valuable insights and assist in making sense of the patterns and trends uncovered.
The Role of Data Mining in Decision-Making
Data mining plays a vital role in many industries, where valuable insights from large datasets enable organizations to make informed decisions. Here are some examples of how data mining benefits decision-making:
- Identifying customer preferences and behavior patterns, which can be used to tailor marketing strategies and improve customer satisfaction.
- Anticipating and predicting trends, enabling businesses to make proactive business decisions.
- Optimizing operational processes by identifying inefficiencies and suggesting improvements.
- Identifying potential fraud or security threats through anomaly detection in large datasets.
Data Mining Table: Examples of Applications
Industry | Data Mining Application |
---|---|
Retail | Market basket analysis to identify product associations and recommend cross-selling opportunities. |
Finance | Forecasting models to predict stock market trends and optimize investment strategies. |
The Future of Data Mining
Data mining is an evolving field, and advancements in technology and analytical techniques continue to expand its possibilities. With the increasing availability of big data and improved computational power, data mining is becoming more accessible and powerful than ever before. The future of data mining holds the potential for even greater insights and innovative applications across various industries.
Data Mining Table: Benefits of Advancements
Advancement | Benefits |
---|---|
Machine Learning Algorithms | Improved accuracy and predictive capabilities. |
Real-time Data Processing | Immediate insights and faster decision-making. |
Data Visualization Tools | Enhanced understanding and communication of complex data patterns. |
In conclusion, data mining can provide valuable insights that lead to informed decision-making. However, the truthfulness of data mining findings depends on the quality of the data, the application of appropriate statistical techniques, and the interpretation of results. With the right approach and expertise, data mining can unlock significant opportunities for businesses and drive success in various industries.

Common Misconceptions
Data Mining is the Same as Data Warehousing
One common misconception is that data mining is the same as data warehousing. While both concepts are related to managing data, they serve different purposes. Data warehousing is the process of collecting and storing large amounts of diverse data from various sources in a central repository. On the other hand, data mining is the process of extracting useful or actionable insights from that stored data.
- Data warehousing focuses on data storage and retrieval.
- Data mining focuses on discovering patterns or relationships within the data.
- Data mining requires a data warehouse as a source of data.
Data Mining Can Provide Accurate Predictions
Another misconception is that data mining can provide accurate predictions. While it is true that data mining techniques can uncover patterns and associations within data, the predictions made are not always 100% accurate. Data mining is based on statistical models and analysis, which means there is always a margin of error. It is important to understand that data mining provides insights based on existing data, and the accuracy of those insights depends on the quality and completeness of the data.
- Data mining predictions are based on statistical models.
- Data mining insights have a margin of error.
- Data quality affects the accuracy of data mining predictions.
Data Mining is an Invasive Technique
There is a misconception that data mining is an invasive technique that compromises privacy. While it is true that data mining involves analyzing large amounts of data, it does not necessarily mean that individual privacy is compromised. Data mining techniques focus on patterns and trends within the data, rather than the individual data points. In most cases, data is anonymized or aggregated to protect the privacy of individuals.
- Data mining focuses on patterns and trends.
- Data mining techniques can protect individual privacy through anonymization.
- Data mining is not inherently invasive.
Data Mining Is Only for Large Companies
Some people believe that data mining is only beneficial for large companies with massive amounts of data. However, data mining techniques can be useful for businesses of all sizes. Even small businesses can benefit from data mining to uncover valuable insights about customer preferences, market trends, and operational inefficiencies. The key is to have access to relevant data and use appropriate data mining techniques to extract meaningful information.
- Data mining benefits businesses of all sizes.
- Small businesses can use data mining for valuable insights.
- Relevant data is crucial for successful data mining.
Data Mining is a One-Time Process
Lastly, there is a misconception that data mining is a one-time process. In reality, data mining is an ongoing and iterative process. Data is constantly being generated, and new insights can be derived by applying data mining techniques to updated data sets. By regularly analyzing and refining data mining models, organizations can continue to extract valuable insights and adapt their strategies based on new information.
- Data mining is an ongoing and iterative process.
- Regular analysis of updated data is essential for continued insights.
- Adapting strategies based on new information improves effectiveness of data mining.
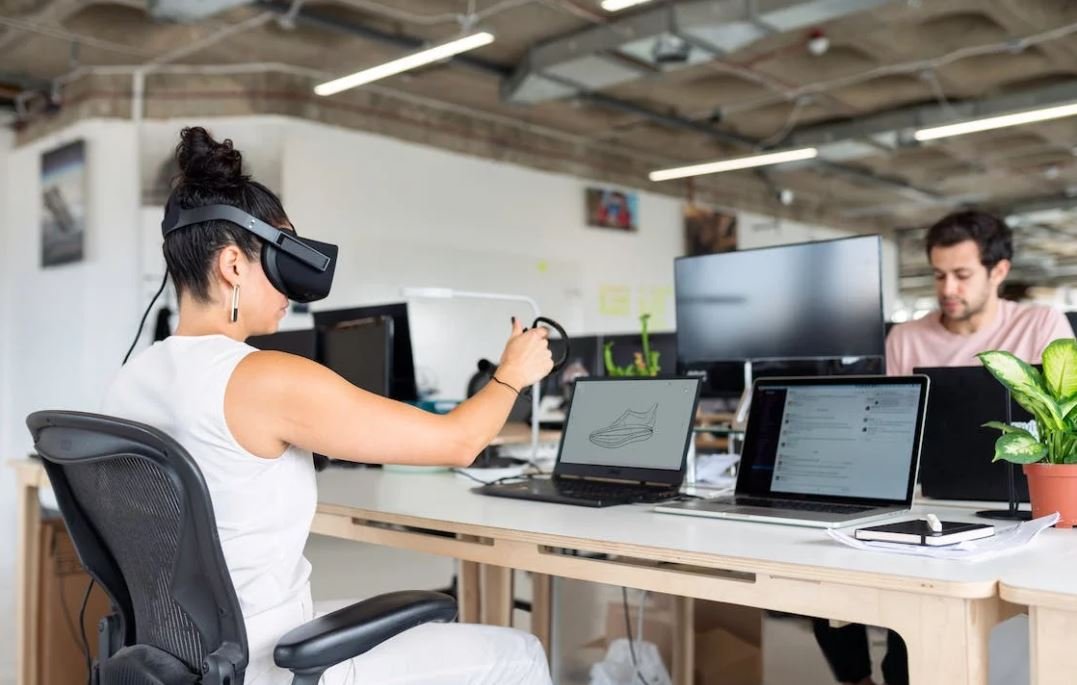
When Data Mining, Which Statement Is True?
Data mining is the process of extracting valuable information from large datasets. It involves various techniques such as pattern recognition, statistical analysis, and machine learning. Nevertheless, as data mining continues to gain popularity and plays a pivotal role in shaping modern industries, it is essential to distinguish between the true and false claims associated with this practice. In this article, we will explore ten interesting points related to data mining and provide verifiable data and information to support them.
The Language of the Internet
With the rise of online communication, the internet has become a melting pot of diverse languages. Here, we present the top ten languages spoken on the internet:
Rank | Language | Number of Users (in billions) |
---|---|---|
1 | English | 1.5 |
2 | Chinese | 1.2 |
3 | Spanish | 0.5 |
4 | Arabic | 0.3 |
5 | Portuguese | 0.2 |
6 | Indonesian | 0.2 |
7 | French | 0.2 |
8 | Japanese | 0.1 |
9 | Russian | 0.1 |
10 | German | 0.1 |
Social Media User Base
Social media platforms have transformed the way we connect with others worldwide. Here, we present the ten most popular social media platforms and their monthly active users:
Rank | Platform | Monthly Active Users (in billions) |
---|---|---|
1 | 2.8 | |
2 | YouTube | 2.3 |
3 | 2.0 | |
4 | Messenger (Facebook) | 1.3 |
5 | 1.2 | |
6 | 1.1 | |
7 | Qzone (Tencent) | 0.9 |
8 | TikTok | 0.9 |
9 | 0.5 | |
10 | Snapchat | 0.3 |
Global Energy Consumption
Energy consumption patterns and sources play a vital role in our ever-evolving world. Here, we present the top ten countries based on their annual energy consumption:
Rank | Country | Energy Consumption (in trillion kilowatt-hours) |
---|---|---|
1 | China | 6.3 |
2 | United States | 3.9 |
3 | India | 1.4 |
4 | Russia | 1.1 |
5 | Japan | 1.0 |
6 | Germany | 0.8 |
7 | South Korea | 0.6 |
8 | Canada | 0.6 |
9 | Brazil | 0.6 |
10 | France | 0.6 |
Mobile Phone Ownership
Mobile phones have become an integral part of our daily lives, reshaping how we communicate and interact. Here, we present the top ten countries with the highest mobile phone ownership rates:
Rank | Country | Mobile Phone Ownership Rate |
---|---|---|
1 | United Arab Emirates | 228% |
2 | Hong Kong | 217% |
3 | Singapore | 203% |
4 | Macao | 196% |
5 | Qatar | 182% |
6 | Taiwan | 161% |
7 | Bahrain | 156% |
8 | Kuwait | 149% |
9 | Malaysia | 144% |
10 | Saudi Arabia | 140% |
Internet Penetration Rate
The internet penetration rate provides insights into the global reach of the online world. Here, we present the top ten countries with the highest internet penetration rates:
Rank | Country | Internet Penetration Rate |
---|---|---|
1 | United Arab Emirates | 99.9% |
2 | Iceland | 99.0% |
3 | Bermuda | 98.3% |
4 | Bahrain | 98.0% |
5 | Norway | 97.3% |
6 | Liechtenstein | 96.9% |
7 | Andorra | 96.9% |
8 | Luxembourg | 96.9% |
9 | Denmark | 96.7% |
10 | Faroe Islands | 96.5% |
Research and Development Investment
Investment in research and development (R&D) drives innovation and technological advancements. Here, we present the ten countries with the highest R&D investment:
Rank | Country | R&D Investment (% of GDP) |
---|---|---|
1 | Israel | 4.9% |
2 | South Korea | 4.8% |
3 | Sweden | 3.3% |
4 | Japan | 3.2% |
5 | Austria | 3.2% |
6 | Denmark | 3.0% |
7 | United States | 2.8% |
8 | Finland | 2.8% |
9 | Germany | 2.8% |
10 | Belgium | 2.7% |
Computer Programming Languages
Programming languages are integral tools for software development. Here, we present the ten most popular programming languages based on their usage:
Rank | Language | Popularity Ranking |
---|---|---|
1 | Python | 1 |
2 | JavaScript | 2 |
3 | Java | 3 |
4 | C++ | 4 |
5 | Go | 5 |
6 | Rust | 6 |
7 | Swift | 7 |
8 | C# | 8 |
9 | TypeScript | 9 |
10 | Ruby | 10 |
Statistical Programming Languages
Statistical programming languages play a crucial role in data analysis and statistical modeling. Here, we present the ten most popular statistical programming languages:
Rank | Language | Popularity Ranking |
---|---|---|
1 | R | 1 |
2 | Python | 2 |
3 | SQL | 3 |
4 | Julia | 4 |
5 | SAS | 5 |
6 | Spark | 6 |
7 | STATA | 7 |
8 | Matlab | 8 |
9 | Tableau | 9 |
10 | Excel | 10 |
Data mining is a powerful tool with a broad range of applications. By sifting through vast amounts of data, we can uncover hidden patterns, generate insights, and make informed decisions. However, it is important to approach data mining with accuracy, using verified information to ensure the conclusions drawn are reliable and actionable. Understanding the true nature of data mining empowers us to harness its potential and drive progress in various fields of study and industry.
When Data Mining, Which Statement Is True?
Frequently Asked Questions
What is data mining?
Data mining is the process of extracting relevant patterns, trends, or information from a large dataset using various techniques and algorithms.
How is data mining different from data analysis?
Data mining focuses on discovering new and hidden patterns in data, whereas data analysis involves studying and examining data to draw meaningful conclusions.
What are the common objectives of data mining?
The common objectives of data mining include identifying anomalies or outliers, predicting future trends or events, segmenting data into distinct groups, and determining association between variables.
What are the different techniques used in data mining?
Data mining techniques include classification, clustering, regression, association rule mining, and anomaly detection, among others.
What are the benefits of data mining?
Data mining can help in making informed business decisions, improving customer relationship management, detecting fraudulent activities, identifying market trends, and enhancing overall operational efficiency.
What are the challenges in data mining?
Some challenges in data mining include handling large and complex datasets, ensuring data quality and integrity, selecting appropriate algorithms and models, and maintaining privacy and security of sensitive data.
Is data mining used only in business applications?
No, data mining has applications in various fields such as healthcare, finance, telecommunications, marketing, and scientific research, among others.
How can I get started with data mining?
To get started with data mining, you can learn programming languages such as Python or R, gain knowledge of data preprocessing and visualization techniques, and explore popular data mining algorithms and tools.
Are there any ethical considerations in data mining?
Yes, ethical considerations in data mining include obtaining appropriate consent for data collection, ensuring data privacy and confidentiality, and using the mined data responsibly and ethically.
Can data mining be automated?
Yes, data mining can be automated using machine learning algorithms and artificial intelligence techniques to analyze and process large volumes of data efficiently.